As I delved into my week two learning at Lux Tech Academy, we were introduced to Python as a beginner language. Here is an overview of an introduction to Python as a data analytics tool. I've gone through numerous videos of data analysts and most of them recommend using Python for data analysis, why? Python is a popular programming language for data analytics as it offers versatility, flexibility, vast libraries, and visualization capabilities to work with large datasets. It's also suitable for data analytics as it blends well with BI tools and databases.
Advantages of Python in Data Analytics
Python is a preferred choice in data analytics since:
- It has a syntax that is simple and easy to learn.
- Numerous libraries such as NumPy, Pandas, Seaborn, SciPy, and Matplotlib aid in data analysis.
- The available libraries such as Matplotlib and Seaborn help in data visualization, aiding in understanding data trends and patterns.
- It is flexible and works well with various data sources and databases, allowing for analysis.
- It helps to deploy analytical solutions into production using frameworks such as Django and Flask. This helps to apply theoretical concepts to the real business world.
Below are some of the ways in which Python is applied in data analytics
- Data Wrangling What exactly is data wrangling? This is the process of gathering raw data, assessing and cleaning it to make it useful. Python is used to import data from various sources, handle missing values, and process large datasets. This helps to reshape data for analysis. Pandas Library is mostly used for data wrangling and manipulation. To use pandas, an environment has to be available i.e., Vs Code or Jupyter Notebook. If pandas isn't installed, run the command:
Pip install pandas
Then run:
import pandas as pd
From there, load a dataset into a pandas data frame.
Exploratory Data Analysis (EDA)
Python helps in data exploration and visualization using libraries like Matplotlib, Seaborn, and Pandas. Coding with Python helps to understand data distribution and relationships using descriptive statistics, pivot tables etc.
Machine Learning
Python offers libraries that aid in machine learning such as Scikit-Learn and Tensor Flow.
How do data analysts use Python in every day life?
Importing data
Various Python libraries such as NumPy, Pandas, BeautifulSoup help in data importation from various sources such as: Csv files and excel spreadsheets, SQL databases, Web APIs and scraping HTML/XML pages and cloud storage. The choice of a library to be used depends on the type and structure of data.
EDA in Python
In the real world, data often contain errors such as missing values and sometimes can be inaccurate thus the need for some cleaning. Exploratory Data Analysis in Python helps in data cleaning by: Identifying anomalies, handling missing data, checking and fixing data types, transforming raw data into a useful and reliable set for analysis.
Transforming data for insights
After cleaning data, python enables various transformation like Pivot tables, concatenating datasets and changing data types and formats.
Descriptive Analysis using Python
SciPy Library has descriptive and inferential statistical functions whereas Matplotlib and Seaborn aid in data visualization.
Python is an interesting language and I can attest it's beginner-friendly. Feel free to leave any question/comment in the comment section.
The above is the detailed content of Introduction to Python as a Data Analytics Tool. For more information, please follow other related articles on the PHP Chinese website!
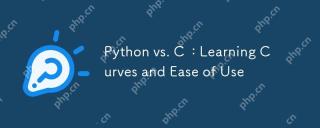
Python is easier to learn and use, while C is more powerful but complex. 1. Python syntax is concise and suitable for beginners. Dynamic typing and automatic memory management make it easy to use, but may cause runtime errors. 2.C provides low-level control and advanced features, suitable for high-performance applications, but has a high learning threshold and requires manual memory and type safety management.
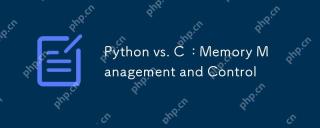
Python and C have significant differences in memory management and control. 1. Python uses automatic memory management, based on reference counting and garbage collection, simplifying the work of programmers. 2.C requires manual management of memory, providing more control but increasing complexity and error risk. Which language to choose should be based on project requirements and team technology stack.
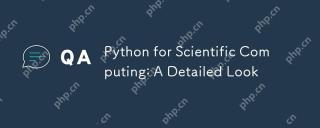
Python's applications in scientific computing include data analysis, machine learning, numerical simulation and visualization. 1.Numpy provides efficient multi-dimensional arrays and mathematical functions. 2. SciPy extends Numpy functionality and provides optimization and linear algebra tools. 3. Pandas is used for data processing and analysis. 4.Matplotlib is used to generate various graphs and visual results.
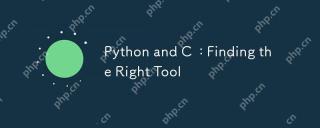
Whether to choose Python or C depends on project requirements: 1) Python is suitable for rapid development, data science, and scripting because of its concise syntax and rich libraries; 2) C is suitable for scenarios that require high performance and underlying control, such as system programming and game development, because of its compilation and manual memory management.
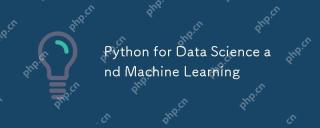
Python is widely used in data science and machine learning, mainly relying on its simplicity and a powerful library ecosystem. 1) Pandas is used for data processing and analysis, 2) Numpy provides efficient numerical calculations, and 3) Scikit-learn is used for machine learning model construction and optimization, these libraries make Python an ideal tool for data science and machine learning.
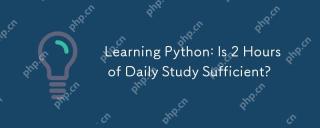
Is it enough to learn Python for two hours a day? It depends on your goals and learning methods. 1) Develop a clear learning plan, 2) Select appropriate learning resources and methods, 3) Practice and review and consolidate hands-on practice and review and consolidate, and you can gradually master the basic knowledge and advanced functions of Python during this period.
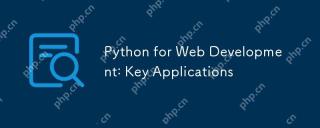
Key applications of Python in web development include the use of Django and Flask frameworks, API development, data analysis and visualization, machine learning and AI, and performance optimization. 1. Django and Flask framework: Django is suitable for rapid development of complex applications, and Flask is suitable for small or highly customized projects. 2. API development: Use Flask or DjangoRESTFramework to build RESTfulAPI. 3. Data analysis and visualization: Use Python to process data and display it through the web interface. 4. Machine Learning and AI: Python is used to build intelligent web applications. 5. Performance optimization: optimized through asynchronous programming, caching and code
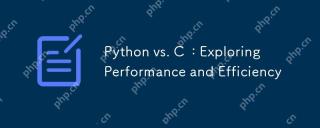
Python is better than C in development efficiency, but C is higher in execution performance. 1. Python's concise syntax and rich libraries improve development efficiency. 2.C's compilation-type characteristics and hardware control improve execution performance. When making a choice, you need to weigh the development speed and execution efficiency based on project needs.


Hot AI Tools

Undresser.AI Undress
AI-powered app for creating realistic nude photos

AI Clothes Remover
Online AI tool for removing clothes from photos.

Undress AI Tool
Undress images for free

Clothoff.io
AI clothes remover

Video Face Swap
Swap faces in any video effortlessly with our completely free AI face swap tool!

Hot Article

Hot Tools

MinGW - Minimalist GNU for Windows
This project is in the process of being migrated to osdn.net/projects/mingw, you can continue to follow us there. MinGW: A native Windows port of the GNU Compiler Collection (GCC), freely distributable import libraries and header files for building native Windows applications; includes extensions to the MSVC runtime to support C99 functionality. All MinGW software can run on 64-bit Windows platforms.
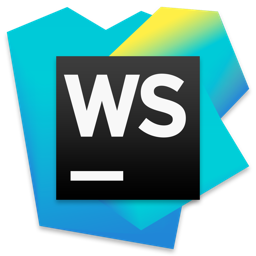
WebStorm Mac version
Useful JavaScript development tools

mPDF
mPDF is a PHP library that can generate PDF files from UTF-8 encoded HTML. The original author, Ian Back, wrote mPDF to output PDF files "on the fly" from his website and handle different languages. It is slower than original scripts like HTML2FPDF and produces larger files when using Unicode fonts, but supports CSS styles etc. and has a lot of enhancements. Supports almost all languages, including RTL (Arabic and Hebrew) and CJK (Chinese, Japanese and Korean). Supports nested block-level elements (such as P, DIV),

DVWA
Damn Vulnerable Web App (DVWA) is a PHP/MySQL web application that is very vulnerable. Its main goals are to be an aid for security professionals to test their skills and tools in a legal environment, to help web developers better understand the process of securing web applications, and to help teachers/students teach/learn in a classroom environment Web application security. The goal of DVWA is to practice some of the most common web vulnerabilities through a simple and straightforward interface, with varying degrees of difficulty. Please note that this software

SecLists
SecLists is the ultimate security tester's companion. It is a collection of various types of lists that are frequently used during security assessments, all in one place. SecLists helps make security testing more efficient and productive by conveniently providing all the lists a security tester might need. List types include usernames, passwords, URLs, fuzzing payloads, sensitive data patterns, web shells, and more. The tester can simply pull this repository onto a new test machine and he will have access to every type of list he needs.