Artificial Intelligence (AI) has become an essential part of modern software development, powering applications in areas like data analysis, automation, and machine learning. While many AI frameworks and libraries exist for languages like Python, Java remains a powerful, versatile language for creating robust, scalable AI applications. In this blog, we’ll explore how to build AI applications in Java with hands-on examples and a step-by-step guide.
Why Use Java for AI Development?
While Python dominates the AI landscape due to its simplicity and powerful libraries, Java offers several advantages:
- Performance: Java applications are known for their performance and scalability, which makes it ideal for large-scale AI applications.
- Wide Adoption: Many enterprises use Java, and existing systems often need AI integration.
- Cross-Platform: Java's platform-independent nature makes it easy to run AI models on different systems.
- Rich Ecosystem: Java boasts a wealth of libraries for AI, including Deeplearning4j, Weka, and Apache Mahout.
Let’s dive into how you can implement AI solutions using Java, focusing on machine learning and neural networks.
Setting Up Java for AI
To build AI applications in Java, you need to set up your development environment with appropriate libraries. Some popular libraries include:
- Deeplearning4j (DL4J): A popular open-source, distributed deep-learning library for Java.
- Weka: A collection of machine learning algorithms for data mining.
- Apache Mahout: A scalable machine learning library for clustering, classification, and collaborative filtering.
For this tutorial, we'll focus on Deeplearning4j because of its powerful deep learning capabilities and ease of use in Java.
Installing Deeplearning4j
To install Deeplearning4j, you’ll first need to set up a new Maven project in your favorite Integrated Development Environment (IDE) like IntelliJ IDEA or Eclipse.
Create a Maven Project: Open IntelliJ IDEA (or another IDE), create a new Maven project, and name it something like JavaAIExample.
Add Dependencies: Open the pom.xml file in your project and add the following dependencies for Deeplearning4j and ND4J (Numerical computing library for Java):
<dependencies> <dependency> <groupid>org.deeplearning4j</groupid> <artifactid>deeplearning4j-core</artifactid> <version>1.0.0-beta7</version> </dependency> <dependency> <groupid>org.nd4j</groupid> <artifactid>nd4j-native-platform</artifactid> <version>1.0.0-beta7</version> </dependency> </dependencies>
Once these dependencies are in place, Maven will download the necessary libraries for Deeplearning4j.
Building a Simple AI Model in Java
Let’s walk through creating a simple neural network using Deeplearning4j. We’ll build a basic model to classify handwritten digits from the MNIST dataset.
Step 1: Load the Data
Deeplearning4j provides built-in support for loading the MNIST dataset. Here’s how to load it into your project:
import org.deeplearning4j.datasets.iterator.impl.MnistDataSetIterator; import org.nd4j.linalg.dataset.api.iterator.DataSetIterator; public class MnistExample { public static void main(String[] args) throws Exception { int batchSize = 128; int outputClasses = 10; DataSetIterator mnistTrain = new MnistDataSetIterator(batchSize, true, 12345); DataSetIterator mnistTest = new MnistDataSetIterator(batchSize, false, 12345); } }
Step 2: Define the Neural Network Configuration
Next, we’ll set up a basic neural network with one hidden layer. You can customize the number of layers and neurons depending on your requirements.
import org.deeplearning4j.nn.conf.MultiLayerConfiguration; import org.deeplearning4j.nn.conf.NeuralNetConfiguration; import org.deeplearning4j.nn.conf.layers.DenseLayer; import org.deeplearning4j.nn.conf.layers.OutputLayer; import org.deeplearning4j.nn.multilayer.MultiLayerNetwork; import org.deeplearning4j.optimize.listeners.ScoreIterationListener; import org.nd4j.linalg.activations.Activation; import org.nd4j.linalg.learning.config.Adam; import org.nd4j.linalg.lossfunctions.LossFunctions; public class MnistExample { public static void main(String[] args) throws Exception { // Configuration of the neural network MultiLayerConfiguration config = new NeuralNetConfiguration.Builder() .seed(123) .updater(new Adam(0.001)) .list() .layer(new DenseLayer.Builder() .nIn(28 * 28) // Input layer size (28x28 pixels) .nOut(1000) // Number of neurons in the hidden layer .activation(Activation.RELU) .build()) .layer(new OutputLayer.Builder(LossFunctions.LossFunction.NEGATIVELOGLIKELIHOOD) .nIn(1000) .nOut(10) // 10 output classes (digits 0-9) .activation(Activation.SOFTMAX) .build()) .build(); MultiLayerNetwork model = new MultiLayerNetwork(config); model.init(); model.setListeners(new ScoreIterationListener(10)); // Output score every 10 iterations } }
Step 3: Train the Model
Now, we’ll train the model using the MNIST training dataset and evaluate its performance.
import org.nd4j.linalg.dataset.api.iterator.DataSetIterator; import org.nd4j.linalg.dataset.api.DataSet; import org.deeplearning4j.eval.Evaluation; public class MnistExample { public static void main(String[] args) throws Exception { // (Setup code here) // Training the model for (int i = 0; i <h3> Step 4: Run the Model </h3> <p>Compile and run your Java application. After the training is complete, the console will display the evaluation metrics, including accuracy and precision.</p> <hr> <h2> Conclusion </h2> <p>Creating AI applications in Java may not be as popular as in Python, but it offers significant advantages for enterprise-grade, scalable AI systems. In this tutorial, we demonstrated how to set up a Java environment for AI development using Deeplearning4j, load data, configure a neural network, and train and evaluate the model.</p> <p>Java's performance, coupled with libraries like Deeplearning4j, allows developers to integrate AI into their systems seamlessly. Whether you are working on real-time applications or large-scale data-driven solutions, Java remains a powerful choice for building AI systems.</p> <hr> <h3> Further Reading: </h3>
- Deeplearning4j Documentation
- Java Machine Learning Library (Java-ML)
- Apache Mahout
With Java, you have the tools and libraries to bring the power of AI to your applications. Happy coding!
The above is the detailed content of Building Smart AI Apps in Java. For more information, please follow other related articles on the PHP Chinese website!
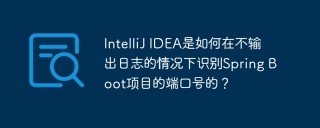
Start Spring using IntelliJIDEAUltimate version...
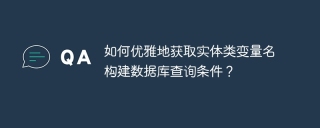
When using MyBatis-Plus or other ORM frameworks for database operations, it is often necessary to construct query conditions based on the attribute name of the entity class. If you manually every time...
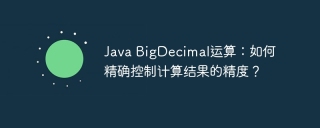
Java...
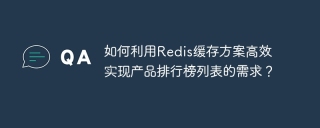
How does the Redis caching solution realize the requirements of product ranking list? During the development process, we often need to deal with the requirements of rankings, such as displaying a...
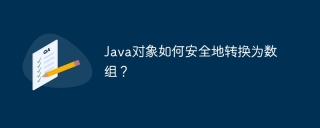
Conversion of Java Objects and Arrays: In-depth discussion of the risks and correct methods of cast type conversion Many Java beginners will encounter the conversion of an object into an array...
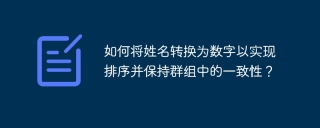
Solutions to convert names to numbers to implement sorting In many application scenarios, users may need to sort in groups, especially in one...
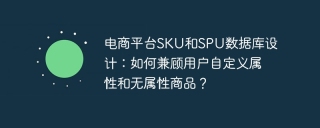
Detailed explanation of the design of SKU and SPU tables on e-commerce platforms This article will discuss the database design issues of SKU and SPU in e-commerce platforms, especially how to deal with user-defined sales...
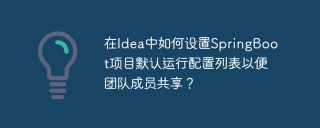
How to set the SpringBoot project default run configuration list in Idea using IntelliJ...


Hot AI Tools

Undresser.AI Undress
AI-powered app for creating realistic nude photos

AI Clothes Remover
Online AI tool for removing clothes from photos.

Undress AI Tool
Undress images for free

Clothoff.io
AI clothes remover

Video Face Swap
Swap faces in any video effortlessly with our completely free AI face swap tool!

Hot Article

Hot Tools

SublimeText3 English version
Recommended: Win version, supports code prompts!

mPDF
mPDF is a PHP library that can generate PDF files from UTF-8 encoded HTML. The original author, Ian Back, wrote mPDF to output PDF files "on the fly" from his website and handle different languages. It is slower than original scripts like HTML2FPDF and produces larger files when using Unicode fonts, but supports CSS styles etc. and has a lot of enhancements. Supports almost all languages, including RTL (Arabic and Hebrew) and CJK (Chinese, Japanese and Korean). Supports nested block-level elements (such as P, DIV),
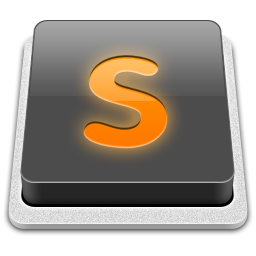
SublimeText3 Mac version
God-level code editing software (SublimeText3)

MinGW - Minimalist GNU for Windows
This project is in the process of being migrated to osdn.net/projects/mingw, you can continue to follow us there. MinGW: A native Windows port of the GNU Compiler Collection (GCC), freely distributable import libraries and header files for building native Windows applications; includes extensions to the MSVC runtime to support C99 functionality. All MinGW software can run on 64-bit Windows platforms.

Atom editor mac version download
The most popular open source editor