The AIxiv column is a column where this site publishes academic and technical content. In the past few years, the AIxiv column of this site has received more than 2,000 reports, covering top laboratories from major universities and companies around the world, effectively promoting academic exchanges and dissemination. If you have excellent work that you want to share, please feel free to contribute or contact us for reporting. Submission email: liyazhou@jiqizhixin.com; zhaoyunfeng@jiqizhixin.com
The first authors of this article are Qian Cheng and He Bingxiang, undergraduate students in the Department of Computer Science, Tsinghua University. Both are members of THUNLP. Qian Cheng's main research interests are tool learning and large model-driven agents. He is about to study for a PhD at UIUC. He Bingxiang's main research interests are large model alignment and security, and he will soon study for a PhD at Tsinghua University. The corresponding authors of this article are Cong Xin and Lin Yankai, and the supervisor is Associate Professor Liu Zhiyuan. Today, with the rapid development of artificial intelligence, we are constantly exploring the intelligence of machines, but we often ignore how these intelligent agents deeply understand us - their creators. Every interaction, every word, every action we humans have in life is filled with intention and emotion. But the real challenge is: how are these implicit intentions captured, parsed, and reacted to by the agent? Traditional intelligent agents respond quickly to explicit commands, but they often fail to understand complex human implicit intentions. In recent years, language models such as GPT and LLaMA have demonstrated amazing capabilities in solving complex tasks. However, although the agents with them as their core are good at formulating strategies and executing tasks, they rarely take into account robust user interaction strategies. The tasks given by users are usually vague and short, which requires the agent to not only understand our literal requests, but also to see through our implicit intentions. Therefore, for a new generation of intelligent agents to be implemented and used by the public, it needs to be human-centered, focusing not only on the accuracy of task execution, but also on how to establish a more natural, smooth and rich relationship with humans. Insightful communication style. In order to make up for this lack, a joint team from Tsinghua University, Renmin University, and Tencent recently proposed a new intelligent agent interaction design plan. This work first introduces Intention-in-Interaction (IN3), a new benchmark that aims to understand users' implicit intentions through explicit interactions with users. Using Mistral-7B as the framework and based on IN3 training, Mistral-Interact can proactively evaluate the ambiguity of tasks, query user intentions, and refine them into actionable goals before launching downstream agent task execution. After embedding the model into the XAgent framework, the article conducts a comprehensive evaluation of the fully stateful agent system. The results show that this solution has outstanding performance in identifying ambiguous user tasks, recovering and summarizing key missing information, setting accurate and necessary agent execution goals, and reducing the use of redundant tools. This innovative method not only fills the gap in the interaction between intelligent agents and users, truly putting humans at the center of intelligent agent design, but also means that we are taking a step closer to the goal of designing intelligent agents that are more in line with human intentions. 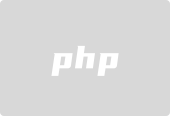
- Paper title: Tell Me More! Towards Implicit User Intention Understanding of Language Model Driven Agents
- Paper link: https://arxiv.org/abs/2402.09205
- Code repository: https ://github.com/HBX-hbx/Mistral-Interact
- Open source model: https://huggingface.co/hbx/Mistral-Interact
- Open source dataset: https://huggingface.co/ datasets/hbx/IN3
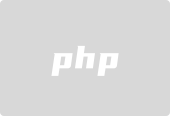
Comparison of fuzzy task and clear task execution Current agent benchmarks often assume that a given task is clear , and does not consider user intent understanding as an important aspect of evaluation. In view of the incompleteness of evaluation indicators, this work developed the Intention-in-Interaction (IN3) benchmark, which aims to evaluate the interactive capabilities of agents through clear task ambiguity judgment and user intention understanding. IN3 benchmark data construction process
As shown in the figure above, the seed task based on human writing is Step 1 , the model iteratively generates new tasks to enhance the dataset while sampling from the dataset as new examples for the next round of generation (Step 2). After this Self-Instruct generation method, the ambiguity, missing details of each task, the importance of each detail, and potential options are manually annotated (Step 3).
Mistral-Interact training process Since large language models are at the core of agent design, this work first conducted a preliminary study to evaluate the implicit performance of current open source and closed source models in the interaction process. Formula intention understanding ability.
Specifically, the article randomly selects ten tasks from IN3, applies them to test LLaMA-2-7B-Chat, Mistral-7B-Instruct-v0.2 and GPT-4 and indicates these modelsi ) determine the ambiguity of the task, ii) ask the user for missing details when the task is ambiguous, iii) summarize the detailed user task. Better, but still lacking understanding of human intent problem. In contrast, GPT-4 is closest to human intention in terms of task ambiguity and important missing details. At the same time, preliminary exploration also shows that in order to further improve the agent's ability to understand implicit intentions in interaction, simple prompt engineering is not enough. It is necessary to further train based on the current open source model to achieve the goal of The degree of application of intelligent agents.
The construction process of training data (IN3 conversation records) Referring to the figure above, according to IN3's annotation of task ambiguity, missing details and potential options, the article is used in the process of constructing conversation records several strategies (orange box), which includes: the construction of a clear initial reasoning chain, the construction of a query with suggested options, the construction of different user response tones, and the construction of a clear summary of the reasoning chain. These dialogue construction strategies will better stimulate the query and reasoning capabilities of the target model. Comprehensive evaluation of agent interaction capabilitiesAn agent’s implicit intention understanding ability can be evaluated directly through user interaction or indirectly through the agent performing downstream tasks. Among them, user interaction focuses on intention understanding itself, while task execution focuses on the ultimate goal of intention understanding, which is to enhance the agent's ability to handle tasks. Therefore, in order to comprehensively evaluate the interactive agent design, the article divides the experiment into two parts: i) Instruction Understanding: Evaluating the agent’s intention understanding ability during user interaction; ii) Instruction Execution : Evaluate the task execution performance of the agent after integrating the interaction model. Instruction understanding does not involve any real-time agent execution, so the article directly evaluates the performance of different language models during the interaction process to determine their interaction capabilities as an upstream module in agent design. The results are as follows Show: 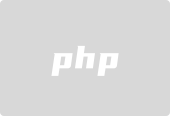
The instructions understand the test results. Among them, the arrow represents the higher the score/ lower the capability. It performs best on indicators such as judging task ambiguity and coverage of missing details, and can make clear and comprehensive summaries based on detailed user intentions. Compared with other open source models, Mistral-Interact can provide more reasonable options for asking for missing details in fuzzy tasks, the query method is more friendly, and its performance is comparable to GPT-4.
In terms of instruction execution, in order to evaluate the effectiveness of implicit intention understanding for agent task execution, the article integrates Mistral-Interact as an upstream interaction module into the XAgent framework for testing. Among them, XAgent can interact in environments such as network search, code execution, command line and file system. Instruction execution test results (ST stands for subtask, MS stands for milestone) 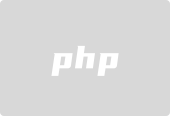
Quantitative evaluation results show that integrating Mistral-Interact helps: i) avoid setting unnecessary goals during the execution process, ii) make the execution process of the agent more consistent with detailed user intentions, and iii) reduce unnecessary tool calls and promote the efficiency of agent tool usage. Agent Interaction Case AnalysisIn terms of command understanding, in order to further demonstrate the robustness of Mistral-Interact in different dialogue scenarios, the article also provides three case analyses. Case studies of Mistral-Interact and users in different scenarios Case A shows the impact of different user tones and conversation styles on Mistral-Interact. The article found that regardless of whether the user's answer was short or detailed, enthusiastic or cold, or even contained spelling errors, Mistral-Interact was able to accurately understand and provide an appropriate response, proving its robustness. In case B, it was tested whether Mistral-Interact can continue to ask questions and guide the conversation back on track when the user shows an uncooperative attitude. The results showed that even when users avoided questions, they were still able to effectively redirect the conversation. In case C it can be observed that Mistral-Interact can incorporate additional information provided by the user into the summary, which is not explicitly asked by the interaction model. This shows that when the model's query cannot fully cover missing details or the user has specific requirements, the model is still able to reasonably and comprehensively summarize all user intentions, making it more user-friendly. In order to more clearly illustrate the role of Mistral-Interact in terms of instruction execution, a comparative case study is provided in the figure below. 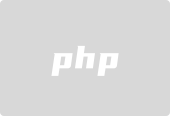
The text in light red can be found when the user’s target is blurred. XAgent cannot accurately set subtasks that accurately reflect user needs. According to the text marked purple, it can be found that XAgent often sets some unnecessary subtasks. These are because the user's task is too vague to perform, and the agent tends to fabricate unnecessary details, which is inconsistent with the user's true intention. In contrast, clear task goals enable XAgent to formulate more specific subtasks after active interaction with Mistral-Interact. The text marked green in the figure demonstrates this consistency. At the same time, the agent execution process becomes simpler and the number of tool calls is reduced. All of these reflect a more efficient agent execution process. We are standing at a new starting point, ready to witness a new chapter of human-machine collaboration, mutual understanding and learning. Intelligent agents will soon no longer be cold information processors, but empathetic partners, capable of deeply understanding our needs and desires that may not be initially clearly expressed through delicate interactive experiences. This revolution in human-centered intelligent agent design will reveal infinite possibilities in interaction, making intelligent agents truly an indispensable help in our lives. The above is the detailed content of Open and closed source model 'Chaos': Let's see which agent can best glimpse human beings' true intentions. For more information, please follow other related articles on the PHP Chinese website!