


Editor | Radish Skin
Protein complex structure prediction plays an important role in drug development, antibody design and other applications. However, due to the limited prediction accuracy, the prediction results are often inconsistent with the experimental results.
A research team from Peking University, Changping Laboratory, and Harvard University proposed ColabDock, a general framework that employs deep learning structural prediction models to integrate experimental constraints of different forms and sources without further large-scale retraining Or fine-tuning.
ColabDock outperforms HADDOCK and ClusPro using AlphaFold2 as a structure prediction model, not only in complex structure predictions with simulated residues and surface constraints, but also in structure predictions with NMR chemical shift perturbations and covalent labeling in this way.
Also, it can help antibody-antigen interface prediction by simulating interface scan limitations.
The study was titled "Integrated structure prediction of protein–protein docking with experimental restraints using ColabDock" and was published in "Nature Machine Intelligence" on August 5, 2024.
Protein docking provides important structural information for understanding biological mechanisms. Although deep models have developed rapidly in protein structure prediction, most models perform predictions in a free-docking manner, which may lead to inconsistencies between experimental constraints and predicted structures.
In order to solve this problem, research teams from Peking University, Changping Laboratory and other institutions proposed a general framework for constrained complex conformation prediction - ColabDock, which is a general protein-protein docking guided by sparse experimental constraints frame.
Through gradient backpropagation, this method effectively integrates experimentally constrained priors and the energy landscape of data-driven protein structure prediction models, automatically searching for conformations that satisfy both while tolerating conflicts or ambiguities in constraints.
ColabDock can leverage different forms and sources of experimental constraints without further extensive retraining or fine-tuning.
The framework contains two stages: generation stage and prediction stage.
In the generation phase, ColabDock uses ColabDesign, a protein design framework developed based on AlphaFold2. Input sequence profiles are optimized in logit space to guide structure prediction models to generate complex structures based on given experimental constraints and templates while maximizing pLDDT and pAE measurements.
In the prediction phase, the structure is predicted based on the generated complex structure and the given template. For each target, ColabDock performs multiple runs and generates different conformations. The final conformation was selected by a ranked support vector machine (SVM) algorithm.
Robust performance
As a proof of concept, the researchers adopted AlphaFold2 as a structure prediction model in ColabDock. Of course, other data-driven deep learning models can also be used here, such as RoseTTAFold2 and AF-Multimer.
The researchers tested ColabDock on synthetic data sets and several types of experimental constraints, including NMR chemical shift perturbation (CSP), covalent labeling (CL), and simulated deep mutation scanning (DMS).
Illustration: ColabDock’s performance on the validation set. (Source: Paper)
ColabDock evaluates two types of constraints, namely 1v1 and MvN constraints. The former is at the residue-residue level and examples include constraints from XL-MS. The latter is at the interface level and is relevant for NMR and CL experiments.
Test results on synthetic data sets show that ColabDock achieves satisfactory performance. Furthermore, as expected, the performance of ColabDock improves as the number of constraints increases.
Even with few constraints, ColabDock outperforms AF-Multimer on benchmark data sets and the same frame settings, and converges to fewer conformations when more constraints are provided, demonstrating the effective application of additional information.
Illustration: Comparing ColabDock, HADDOCK and ClusPro on the benchmark set. (Source: paper)
Compared with HADDOCK and ClusPro, ColabDock performs better when the constraint quality is higher. On both experimental datasets, ColabDock still outperforms HADDOCK and ClusPro regardless of the number and quality of constraints provided.
Illustration: Performance and constraint analysis of ColabDock on CSP set. (Source: paper)
Finally, the researchers evaluated the performance of different docking methods on the antibody-antigen data set. ColabDock predicted a much higher proportion of medium or higher quality structures than HADDOCK and ClusPro.
Illustration: Comparison of ColabDock, HADDOCK and ClusPro on the antibody-antigen benchmark set. (Source: paper)
This shows that ColabDock has potential application value in antibody design. Moreover, ColabDock still shows comparable or even better performance than AF-Multimer on the newly released unbiased dataset.
Limitations and Conclusion
ColabDock also has some limitations. Currently, ColabDock can only accept distances smaller than 22 Å, which is determined by the upper limit of the distance map in AlphaFold2. This limitation renders the model applicable to only a small subset of XL-MS reagents.
Without fragment-based optimization, ColabDock can only process complexes of less than 1,200 residues on an NVIDIA A100 graphics processing unit (GPU) due to limited memory.
In addition, this method can be very time-consuming, especially for large protein complexes. Using the bfloat16 floating point format version of AlphaFold2 is expected to help save memory and speed up calculations.
I believe that in the future, after researchers iteratively optimize it, as a unified framework, ColabDock will be able to help bridge the gap between experimental and computational protein science.
Paper link:https://www.nature.com/articles/s42256-024-00873-z
The above is the detailed content of Nature sub-journal, Peking University team's general AI framework conducts comprehensive structure prediction for protein-protein docking, bridging the gap between experiment and calculation. For more information, please follow other related articles on the PHP Chinese website!
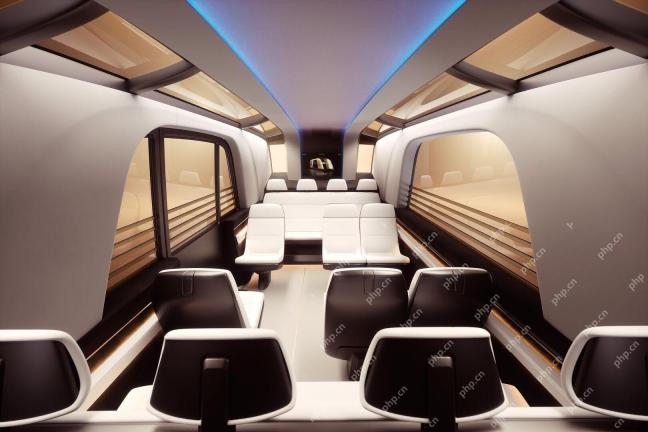
Since 2008, I've championed the shared-ride van—initially dubbed the "robotjitney," later the "vansit"—as the future of urban transportation. I foresee these vehicles as the 21st century's next-generation transit solution, surpas
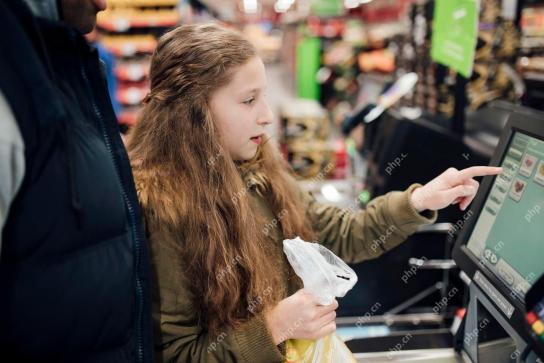
Revolutionizing the Checkout Experience Sam's Club's innovative "Just Go" system builds on its existing AI-powered "Scan & Go" technology, allowing members to scan purchases via the Sam's Club app during their shopping trip.
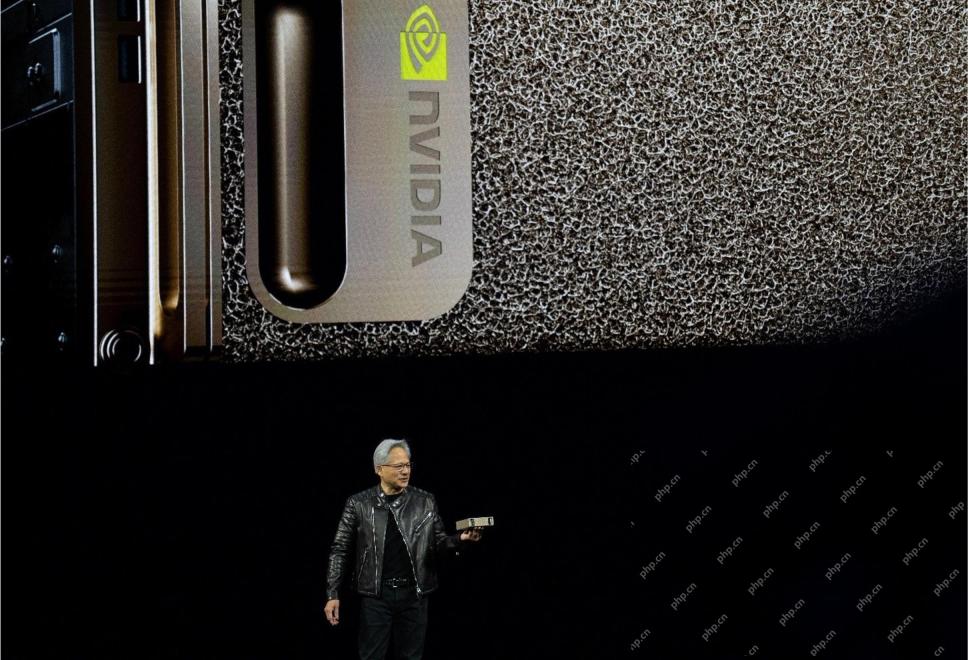
Nvidia's Enhanced Predictability and New Product Lineup at GTC 2025 Nvidia, a key player in AI infrastructure, is focusing on increased predictability for its clients. This involves consistent product delivery, meeting performance expectations, and
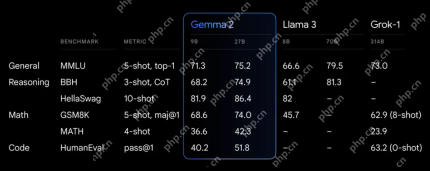
Google's Gemma 2: A Powerful, Efficient Language Model Google's Gemma family of language models, celebrated for efficiency and performance, has expanded with the arrival of Gemma 2. This latest release comprises two models: a 27-billion parameter ver
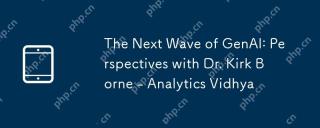
This Leading with Data episode features Dr. Kirk Borne, a leading data scientist, astrophysicist, and TEDx speaker. A renowned expert in big data, AI, and machine learning, Dr. Borne offers invaluable insights into the current state and future traje
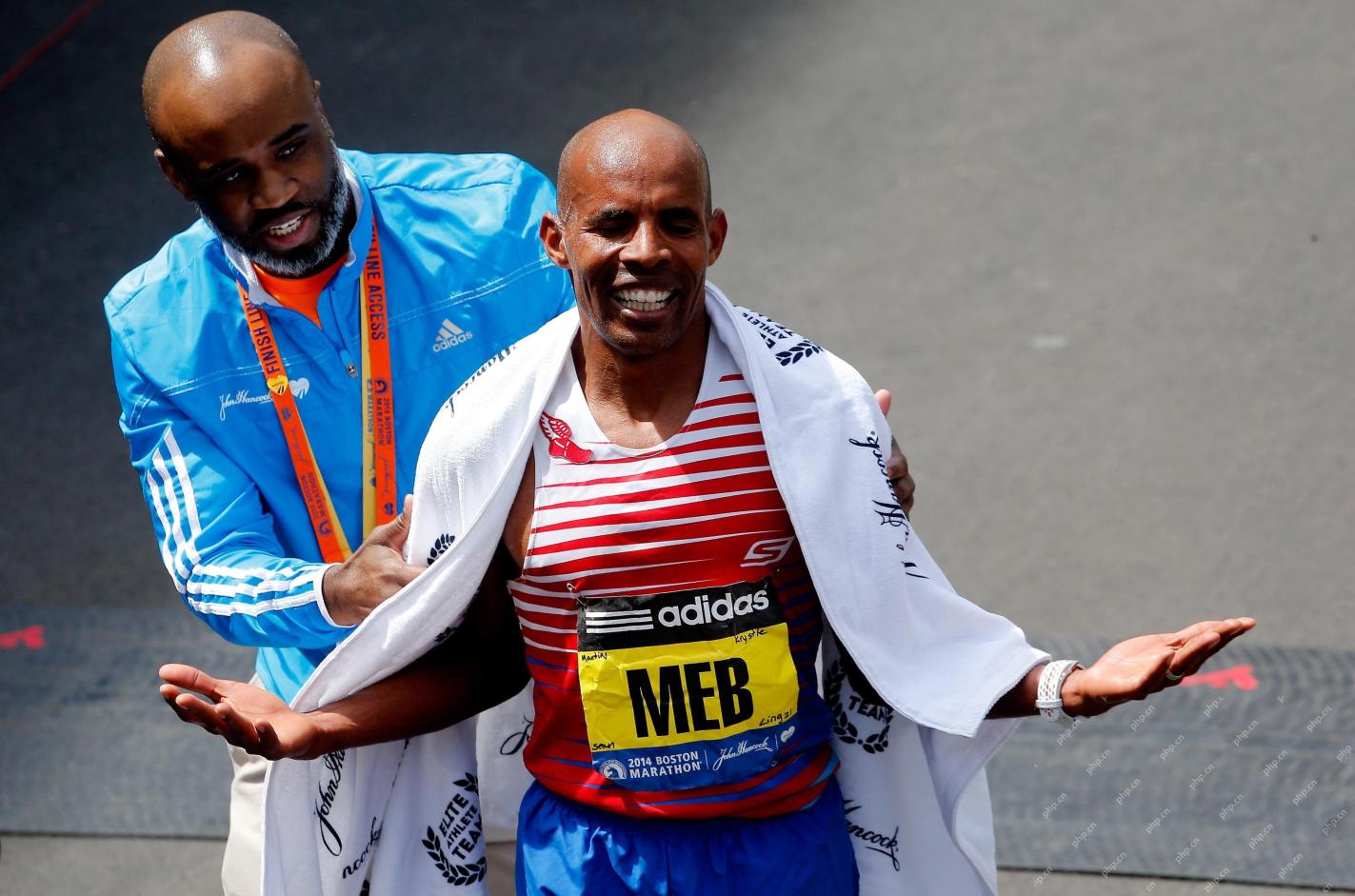
There were some very insightful perspectives in this speech—background information about engineering that showed us why artificial intelligence is so good at supporting people’s physical exercise. I will outline a core idea from each contributor’s perspective to demonstrate three design aspects that are an important part of our exploration of the application of artificial intelligence in sports. Edge devices and raw personal data This idea about artificial intelligence actually contains two components—one related to where we place large language models and the other is related to the differences between our human language and the language that our vital signs “express” when measured in real time. Alexander Amini knows a lot about running and tennis, but he still
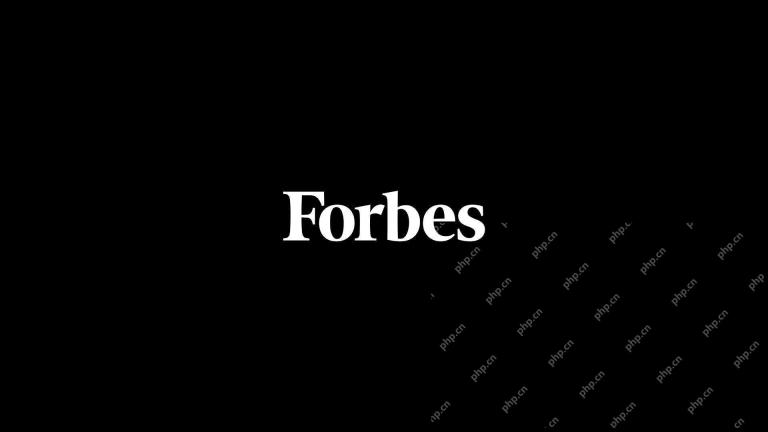
Caterpillar's Chief Information Officer and Senior Vice President of IT, Jamie Engstrom, leads a global team of over 2,200 IT professionals across 28 countries. With 26 years at Caterpillar, including four and a half years in her current role, Engst
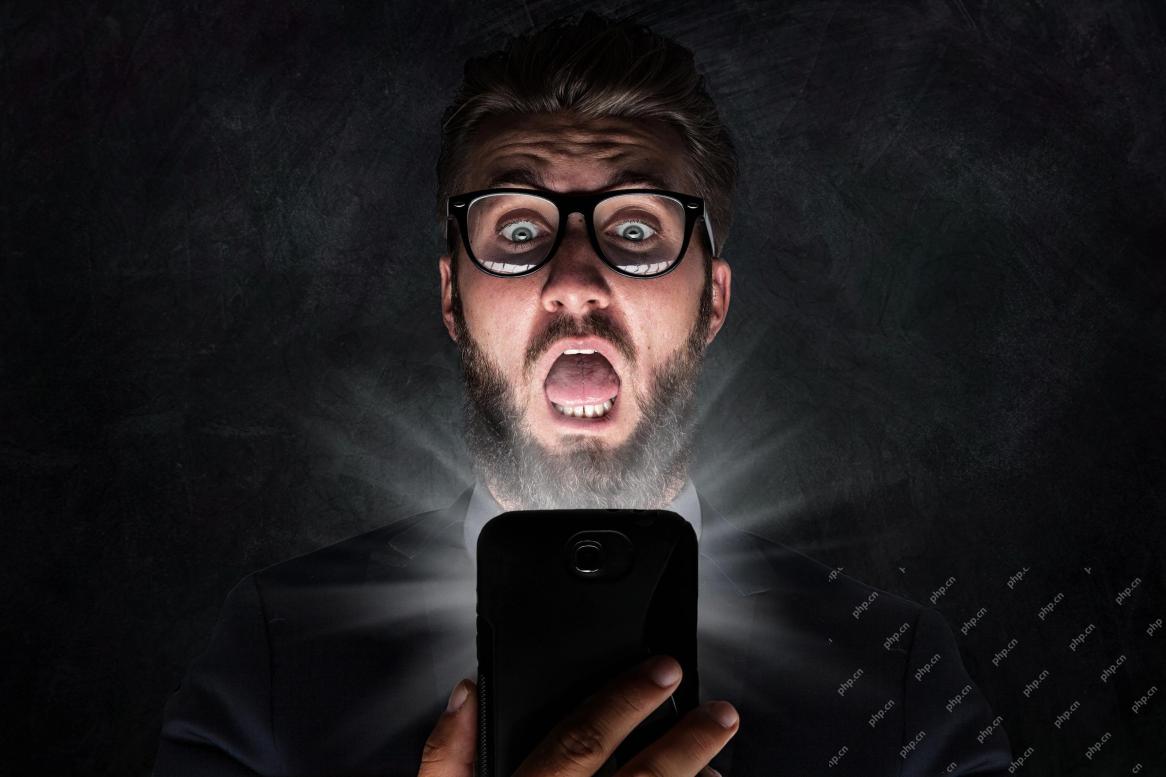
Google Photos' New Ultra HDR Tool: A Quick Guide Enhance your photos with Google Photos' new Ultra HDR tool, transforming standard images into vibrant, high-dynamic-range masterpieces. Ideal for social media, this tool boosts the impact of any photo,


Hot AI Tools

Undresser.AI Undress
AI-powered app for creating realistic nude photos

AI Clothes Remover
Online AI tool for removing clothes from photos.

Undress AI Tool
Undress images for free

Clothoff.io
AI clothes remover

Video Face Swap
Swap faces in any video effortlessly with our completely free AI face swap tool!

Hot Article

Hot Tools

Atom editor mac version download
The most popular open source editor

SublimeText3 English version
Recommended: Win version, supports code prompts!

mPDF
mPDF is a PHP library that can generate PDF files from UTF-8 encoded HTML. The original author, Ian Back, wrote mPDF to output PDF files "on the fly" from his website and handle different languages. It is slower than original scripts like HTML2FPDF and produces larger files when using Unicode fonts, but supports CSS styles etc. and has a lot of enhancements. Supports almost all languages, including RTL (Arabic and Hebrew) and CJK (Chinese, Japanese and Korean). Supports nested block-level elements (such as P, DIV),

DVWA
Damn Vulnerable Web App (DVWA) is a PHP/MySQL web application that is very vulnerable. Its main goals are to be an aid for security professionals to test their skills and tools in a legal environment, to help web developers better understand the process of securing web applications, and to help teachers/students teach/learn in a classroom environment Web application security. The goal of DVWA is to practice some of the most common web vulnerabilities through a simple and straightforward interface, with varying degrees of difficulty. Please note that this software

MinGW - Minimalist GNU for Windows
This project is in the process of being migrated to osdn.net/projects/mingw, you can continue to follow us there. MinGW: A native Windows port of the GNU Compiler Collection (GCC), freely distributable import libraries and header files for building native Windows applications; includes extensions to the MSVC runtime to support C99 functionality. All MinGW software can run on 64-bit Windows platforms.