


Protein glycosylation is a post-translational modification of proteins by sugar groups, which plays an important role in various physiological and pathological functions of cells.
Glycoproteomics is the study of protein glycosylation within the proteome, using liquid chromatography coupled with tandem mass spectrometry (MS/MS) technology to obtain combined information on glycosylation sites, glycosylation levels and sugar structures. .
However, current database search methods for glycoproteomics often have difficulty determining glycan structures due to the limited occurrence of structure-determining ions. Although spectral search methods can exploit fragmentation intensity to facilitate structural identification of glycopeptides, difficulties in spectral library construction hinder their application.
In the latest study, researchers from Fudan University proposed DeepGP, a hybrid deep learning framework based on Transformer and graph neural networks, for predicting MS/MS spectra and retention times (RT) of glycopeptides.
Two graph neural network modules are used to capture branched sugar structures and predict sugar ion strengths respectively. Additionally, a pre-training strategy was implemented to alleviate the shortage of glycoproteomic data.
The research was titled "Deep learning prediction of glycopeptide tandem mass spectra powers glycoproteomics" and was published in "Nature Machine Intelligence" on July 30, 2024.
During the glycosylation process, sugar molecules are attached to the side chains of specific amino acid residues, resulting in structural heterogeneity, resulting in the diversity of glycopeptide isomers and increasing the difficulty of identification.
Liquid chromatography tandem mass spectrometry (LC-MS/MS) is the primary technique to identify glycopeptides by fragment ions and molecular weight combined with RT. Mass-to-charge ratio (m/z) alone is not enough to determine the sugar structure, so scientists use spectral matching methods to improve identification sensitivity. However, constructing glycopeptide MS/MS spectral libraries is costly and complex.
In recent years, deep learning has made progress in peptide MS/MS spectrum prediction. However, the relatively small number of current glycopeptidomics data sets and the lack of standardized protocols for generating glycopeptide mass spectrometry data limits the availability of suitable data for deep learning model training.
To this end, researchers from Fudan University propose DeepGP, a deep learning-based hybrid end-to-end framework for complete N-glycopeptide MS/MS spectra and RT prediction. The deep learning framework consists of a pre-trained Transformer module and two graph neural network (GNN) modules.
DeepGP model
- accepts glycopeptides as input
-
Encoded glycopeptide features:
- Glycostructure
- Amino acid sequence
- PTM type
- PTM position
- Precursor charge state
-
The sugar structure is embedded through GNN, converting the glycopeptide into a graph:
- Node: Monosaccharide
Illustration: Distinguishing similar glycan compositions on synthetic data sets based on DeepGP. (Source: Paper)
Two GNN modules capture sugar structure and predict sugar ion intensity
Researchers evaluated three GNN architectures, including graph convolutional network (GCN), graph isomorphism network (GIN) and graph attention network (GAT) for sugar intercalation and B/Y ion intensity prediction.
GCN utilizes convolution operations to obtain node representations and implements a message passing protocol to aggregate the representations of adjacent nodes; GIN performs well in graph isomorphism tests; GAT incorporates an attention mechanism to enable the model to focus on the most relevant parts of the input.
Experimental results show that GCN performs best in the sugar embedding task, while GIN performs well in the B/Y ion intensity prediction task, so GCN and GIN were selected for corresponding analysis.
Illustration: DeepGP performance in MS/MS prediction. (Source: paper) - Node: Monosaccharide
Pre-training strategy to alleviate the shortage of glycoproteomics data
DeepGP uses a large amount of unlabeled natural language data for pre-training, similar to models such as BERT. Pre-training enables the model to have a knowledge base before formal training, thereby enhancing its performance in dealing with small-scale annotated data.
Testing on multiple biological data sets
연구원들은 마우스와 인간 샘플 데이터 세트를 사용하여 MS/MS 및 RT 예측에서 DeepGP의 높은 정확도를 입증했습니다.
합성 및 생물학적 데이터 세트에 대한 DeepGP의 포괄적인 벤치마킹은 유사한 글리칸을 구별하는 데 있어 DeepGP의 효율성을 검증합니다. 데이터베이스 검색과 결합된 DeepGP는 글리코펩타이드 검출 감도를 향상시킵니다.
논문 링크:
https://www.nature.com/articles/s42256-024-00875-x
The above is the detailed content of New method of glycoproteomics, Fudan developed a hybrid end-to-end framework based on Transformer and GNN, published in Nature sub-journal. For more information, please follow other related articles on the PHP Chinese website!
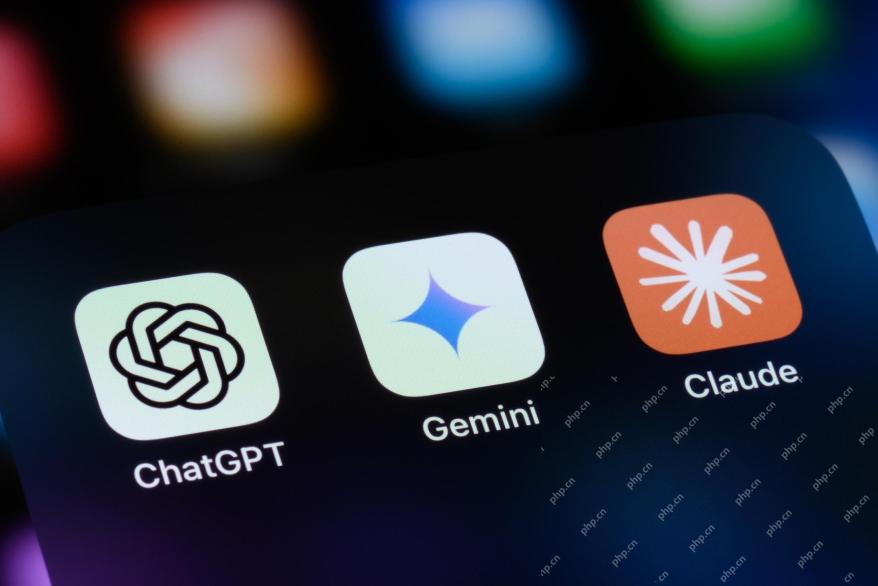
The unchecked internal deployment of advanced AI systems poses significant risks, according to a new report from Apollo Research. This lack of oversight, prevalent among major AI firms, allows for potential catastrophic outcomes, ranging from uncont
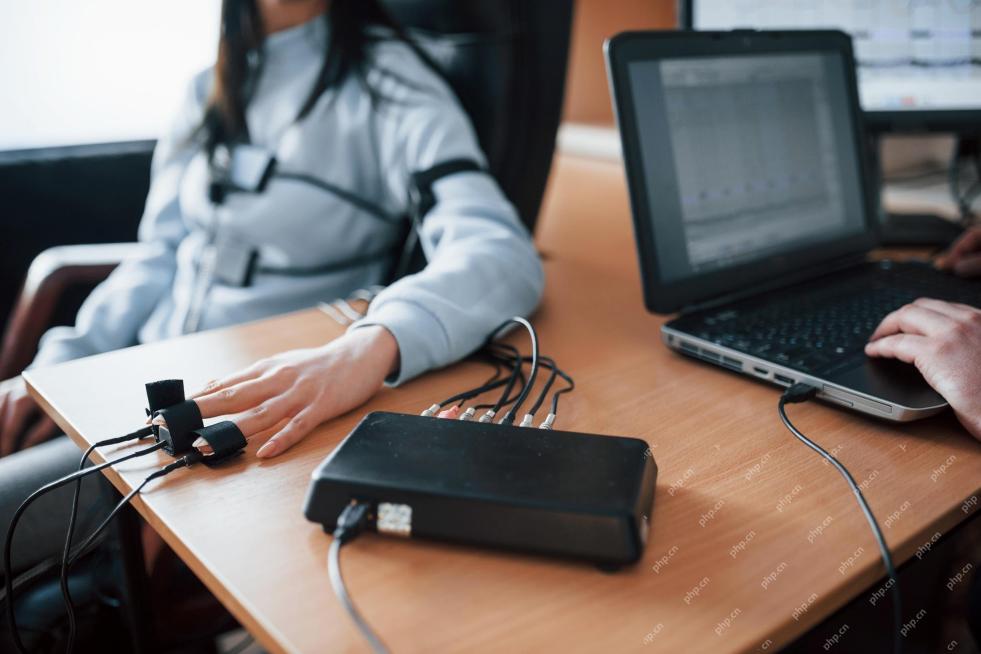
Traditional lie detectors are outdated. Relying on the pointer connected by the wristband, a lie detector that prints out the subject's vital signs and physical reactions is not accurate in identifying lies. This is why lie detection results are not usually adopted by the court, although it has led to many innocent people being jailed. In contrast, artificial intelligence is a powerful data engine, and its working principle is to observe all aspects. This means that scientists can apply artificial intelligence to applications seeking truth through a variety of ways. One approach is to analyze the vital sign responses of the person being interrogated like a lie detector, but with a more detailed and precise comparative analysis. Another approach is to use linguistic markup to analyze what people actually say and use logic and reasoning. As the saying goes, one lie breeds another lie, and eventually
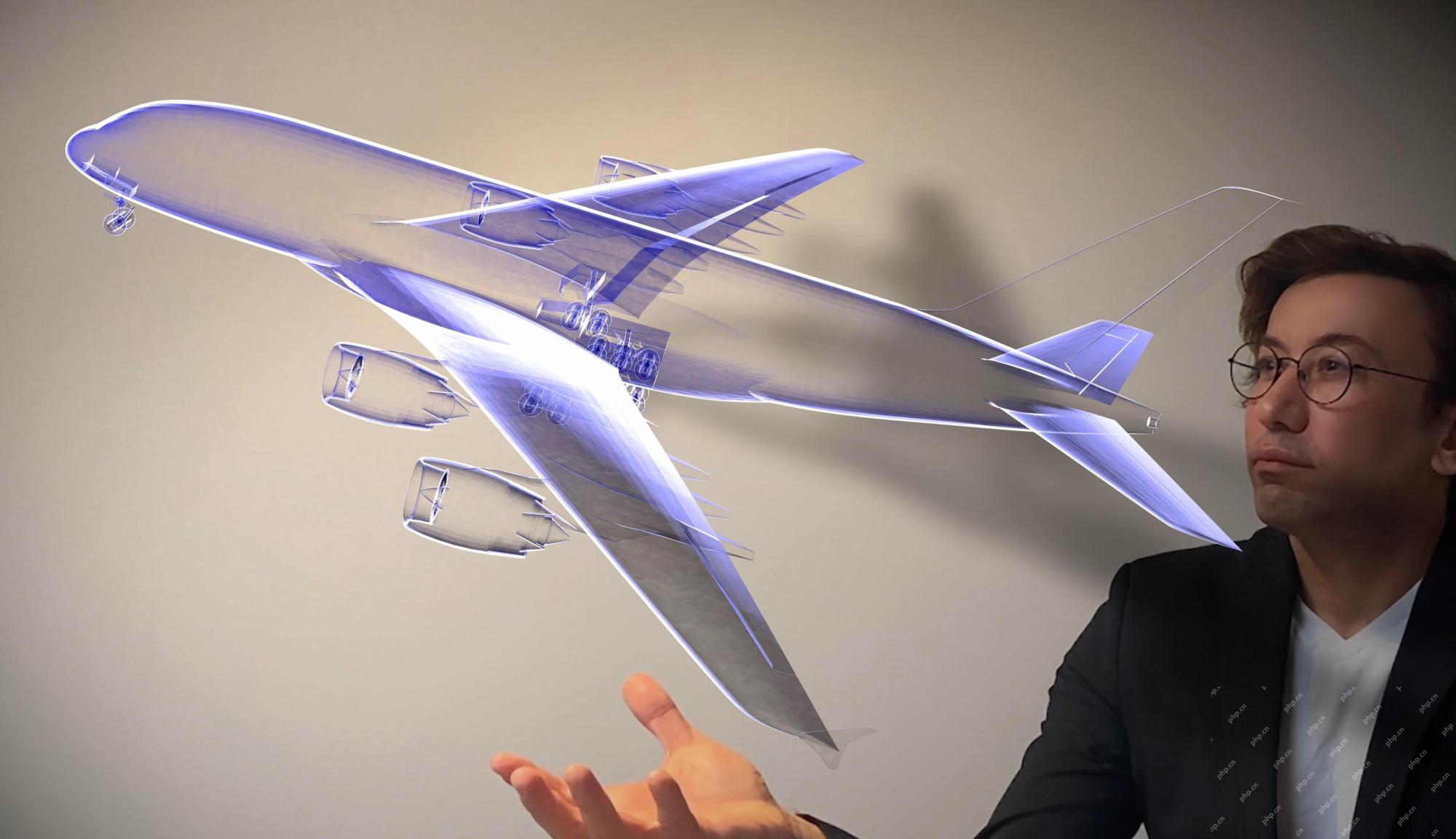
The aerospace industry, a pioneer of innovation, is leveraging AI to tackle its most intricate challenges. Modern aviation's increasing complexity necessitates AI's automation and real-time intelligence capabilities for enhanced safety, reduced oper
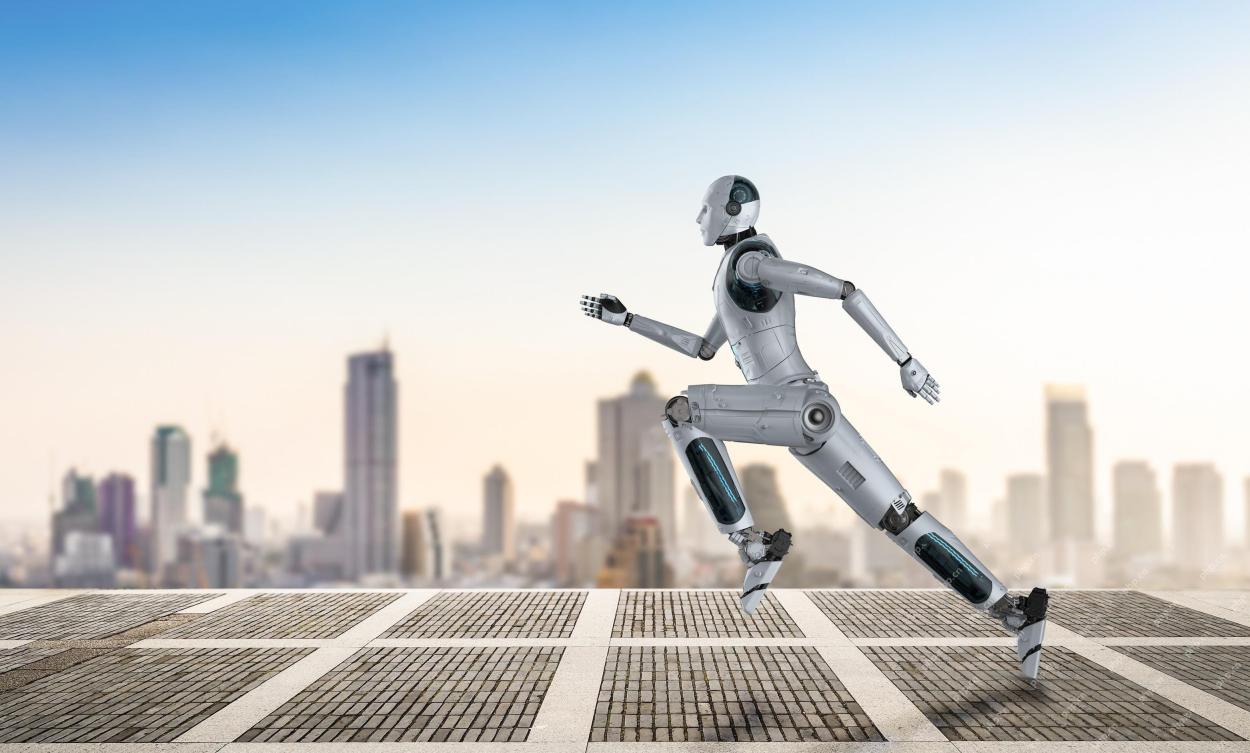
The rapid development of robotics has brought us a fascinating case study. The N2 robot from Noetix weighs over 40 pounds and is 3 feet tall and is said to be able to backflip. Unitree's G1 robot weighs about twice the size of the N2 and is about 4 feet tall. There are also many smaller humanoid robots participating in the competition, and there is even a robot that is driven forward by a fan. Data interpretation The half marathon attracted more than 12,000 spectators, but only 21 humanoid robots participated. Although the government pointed out that the participating robots conducted "intensive training" before the competition, not all robots completed the entire competition. Champion - Tiangong Ult developed by Beijing Humanoid Robot Innovation Center
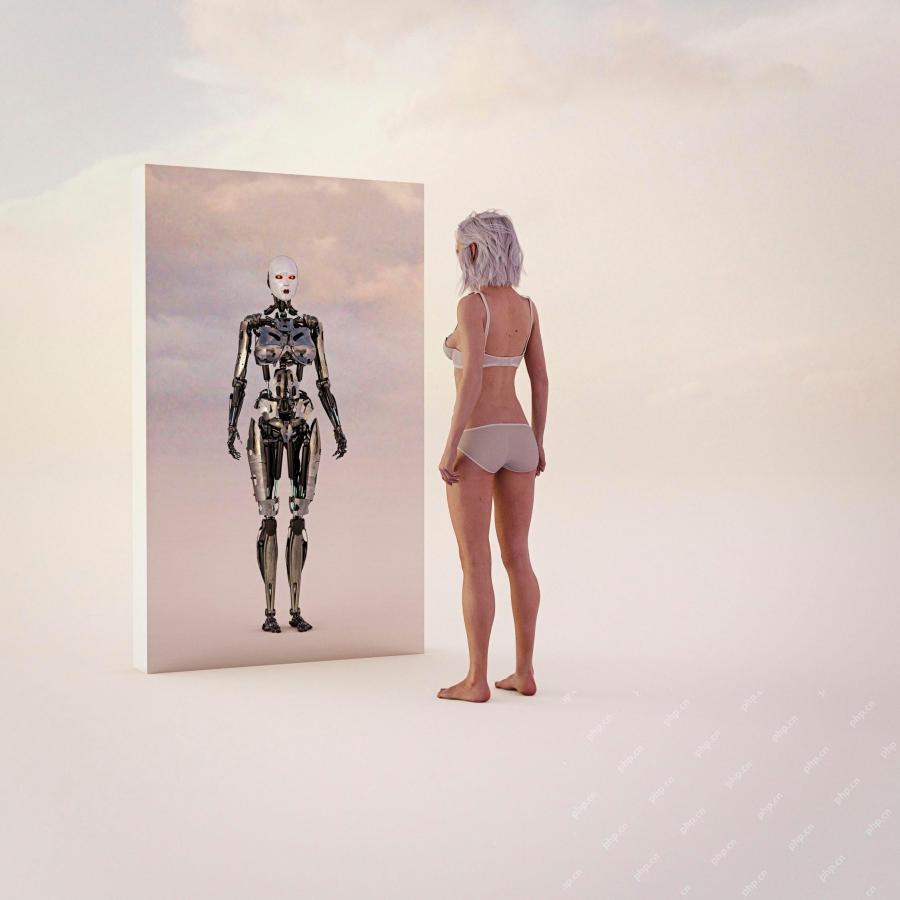
Artificial intelligence, in its current form, isn't truly intelligent; it's adept at mimicking and refining existing data. We're not creating artificial intelligence, but rather artificial inference—machines that process information, while humans su
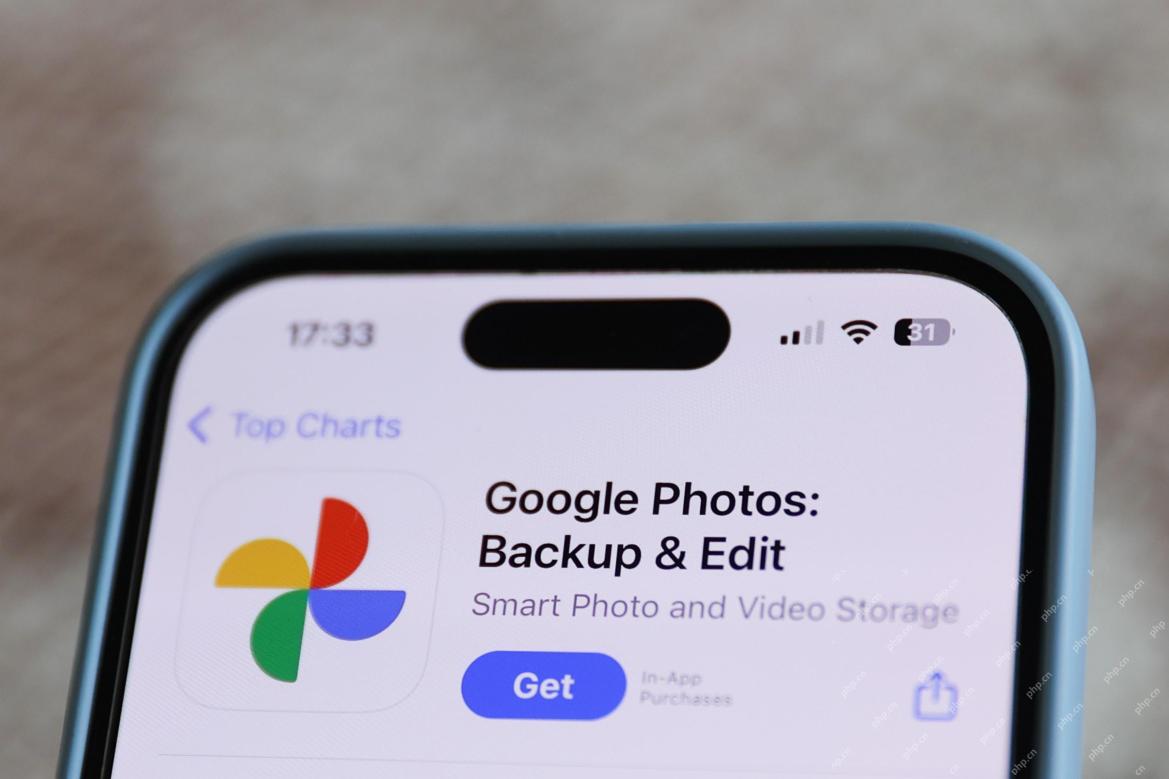
A report found that an updated interface was hidden in the code for Google Photos Android version 7.26, and each time you view a photo, a row of newly detected face thumbnails are displayed at the bottom of the screen. The new facial thumbnails are missing name tags, so I suspect you need to click on them individually to see more information about each detected person. For now, this feature provides no information other than those people that Google Photos has found in your images. This feature is not available yet, so we don't know how Google will use it accurately. Google can use thumbnails to speed up finding more photos of selected people, or may be used for other purposes, such as selecting the individual to edit. Let's wait and see. As for now
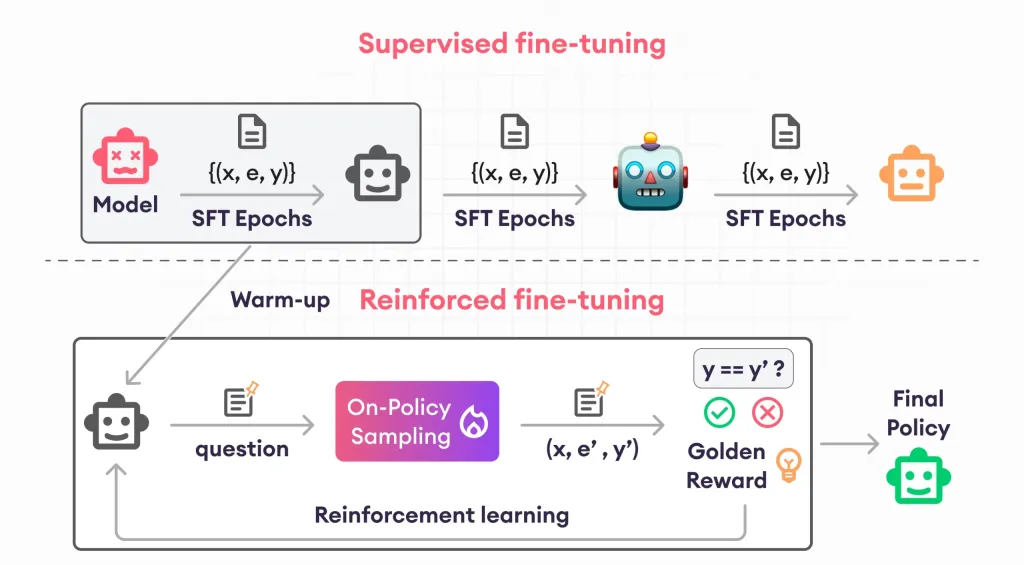
Reinforcement finetuning has shaken up AI development by teaching models to adjust based on human feedback. It blends supervised learning foundations with reward-based updates to make them safer, more accurate, and genuinely help
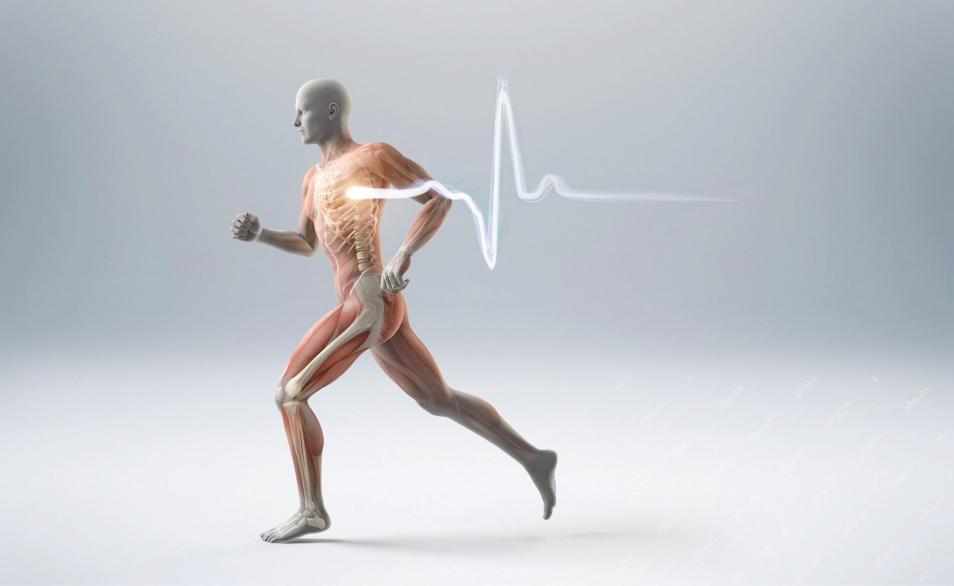
Scientists have extensively studied human and simpler neural networks (like those in C. elegans) to understand their functionality. However, a crucial question arises: how do we adapt our own neural networks to work effectively alongside novel AI s


Hot AI Tools

Undresser.AI Undress
AI-powered app for creating realistic nude photos

AI Clothes Remover
Online AI tool for removing clothes from photos.

Undress AI Tool
Undress images for free

Clothoff.io
AI clothes remover

Video Face Swap
Swap faces in any video effortlessly with our completely free AI face swap tool!

Hot Article

Hot Tools

SublimeText3 Chinese version
Chinese version, very easy to use

mPDF
mPDF is a PHP library that can generate PDF files from UTF-8 encoded HTML. The original author, Ian Back, wrote mPDF to output PDF files "on the fly" from his website and handle different languages. It is slower than original scripts like HTML2FPDF and produces larger files when using Unicode fonts, but supports CSS styles etc. and has a lot of enhancements. Supports almost all languages, including RTL (Arabic and Hebrew) and CJK (Chinese, Japanese and Korean). Supports nested block-level elements (such as P, DIV),
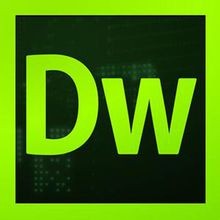
Dreamweaver CS6
Visual web development tools
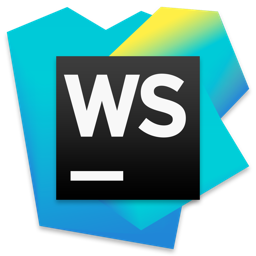
WebStorm Mac version
Useful JavaScript development tools

Safe Exam Browser
Safe Exam Browser is a secure browser environment for taking online exams securely. This software turns any computer into a secure workstation. It controls access to any utility and prevents students from using unauthorized resources.
