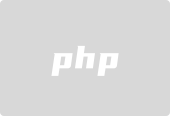
Editor | KX
Generative deep learning is reshaping drug design. Chemical language models (CLMs), which generate molecules as strings of molecules, are particularly important for this process.
Recently, researchers from Eindhoven University of Technology in the Netherlands introduced a latest deep learning architecture (S4) into de novo drug design.
The Structured State Space Sequence (S4) model has excellent performance in learning the global properties of the sequence, so can S4 advance chemical language modeling designed from scratch?
To provide answers, the researchers systematically benchmarked S4 against state-of-the-art CLM on a range of drug discovery tasks, such as the identification of bioactive compounds and the design of drug-like molecules and natural products. S4 has the superior ability to explore a variety of scaffolds while learning complex molecular properties.
Finally, 8 out of 10 molecules designed by S4 were predicted to be highly active by molecular dynamics simulations when applied prospectively to kinase inhibitors.
In summary, S4 has great potential in chemical language modeling, especially in capturing biological activities and complex molecular properties. This is the first time that a state-space model has been applied to a molecular task.
Relevant research was titled "Chemical language modeling with structured state space sequence models" and was published in "Nature Communications" on July 22.
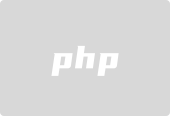
Paper link: https://www.nature.com/articles/s41467-024-50469-9
Designing molecules with desired properties from scratch is a "needle in a haystack" problem. The chemical universe, containing up to 10^60 small molecules, remains largely unknown.
Generative deep learning can produce the desired molecules without hand-designed rules, allowing for a time-saving and low-cost way to explore the chemical universe. In particular, CLM has produced experimentally validated bioactive designs and stands out as a powerful molecular generator.
CLM uses algorithms developed for sequence processing to learn the "language of chemistry", i.e. how to generate molecules that are chemically valid (syntax) and have the desired properties (semantics). This is achieved by representing molecular structures as string symbols, such as the Simplified Molecular Input Line Entry System (SMILES). These molecule strings are then used for model training and subsequent generation of molecules in text form.
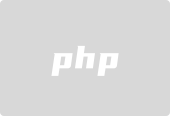
Illustration: Key concepts of structured state space sequence (S4) models for chemical language modeling. (Source: Paper)
CLM Architecture:
- Long Short-Term Memory (LSTM) model
- Transformer Architecture
Structured State Space Sequence Model (S4):
- Rapidly developing new member
- Excellent in audio, image and text generation
-
Has a "dual nature":
- Train on the entire input sequence to learn complex global properties
- Generate one string element at a time
Applications:
Design of drug-like molecules and natural products:
- Researchers benchmarked S4 against state-of-the-art CLM
- such as the design of drug-like molecules and natural products
- First, Analyzed the ability of S4 to design drug-like small molecules (SMILES length less than 100 tokens) extracted from the ChEMBL database
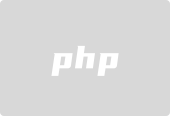
1. All CLMs generated more than 91% of valid molecules, 91% of unique molecules, and 81% of new molecules.
- S4 designs the most efficient, unique, and novel molecules by generating more new molecules than the baseline (approximately 4000 to over 12,000), and shows good ability to learn the "chemical grammar" of SMILES strings.
- The potential of S4 compared to existing de novo design methods is further confirmed on the MOSES benchmark, where S4 consistently ranks among the best performing deep learning methods.
- S4 is also further tested against more challenging molecular entities than drug-like molecules.
- To this end, researchers evaluated its ability to engineer natural products (NPs).
- Compared with synthetic small molecules, NPs tend to have more complex molecular structures and ring systems, as well as a greater proportion of sp3 hybridized carbon atoms and chiral centers.
- These features correspond to longer SMILES sequences on average, with more long-range dependencies, and make natural products challenging test cases for CLM.
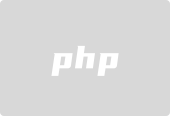
All CLMs can design natural products, but their performance is lower compared to drug-like molecules. S4 designs have the highest number of effective molecules, with about 6000 to 12,000 more molecules than S4 (7-13% better), while LSTM has the highest novelty, with about 2000 more molecules (2%) than S4.
Finally, the training and generation speed of CLM architectures when increasing the SMILES length was also analyzed to test their practical applicability when designing larger molecules such as natural products. The analysis highlights that due to its dual nature, S4 is as fast as GPT during training (both ~1.3x faster than LSTM) and fastest in terms of generation. This further advocates the introduction of S4 as an efficient method for molecular design, offering “the best of both worlds” compared to GPT and LSTM.
Prospective de novo design
Researchers using S4 conducted a prospective in silico study focused on designing inhibitors of mitogen-activated protein kinase 1 (MAPK1), a relevant target for tumor therapy. The putative biological activity of the design was then evaluated by molecular dynamics (MD). 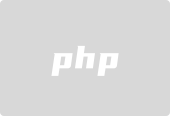
Illustration: Prospective de novo design of putative MAPK1 inhibitors using S4. (Source: paper) The S4 model was fine-tuned and then the last five epochs of the fine-tuned model were used to generate 256K molecules. Designs were ranked and filtered by log-likelihood score and scaffold similarity to the training set, and the 10 highest-scoring molecules were further characterized using MD simulations.
8 out of 10 designs were predicted to be bioactive against the intended targets by MD, with predicted affinities comparable to or higher than the closest fine-tuned molecules, these results further confirm the potential of S4 for de novo drug design.
Opportunities for Molecule S4 In summary, this study is the first to introduce state space models into chemical language modeling, focusing on structured state spaces (S4). The unique dual nature of S4, including convolution and loop generation during training, makes it particularly suitable for de novo design starting from SMILES strings.
Researchers conducted a systematic comparison with GPT and LSTM on various drug discovery tasks, revealing the advantages of S4: Although loop generation (LSTM and S4) is superior in learning chemical grammar and exploring various scaffolds, it is not effective for the entire Ensemble learning of SMILES sequences (GPT and S4) performs well in capturing certain complex properties such as biological activity.
S4 has a dual nature, “the best of both worlds”: it performs as well as or better than LSTM in designing efficient and diverse molecules, and systematically outperforms baselines in capturing complex molecular properties while maintaining computational efficiency. The application of
S4 in MAPK1 inhibition has been validated by MD simulations, further demonstrating its potential for designing potent bioactive molecules. In the future, researchers will prospectively combine S4 with wet lab experiments to enhance its impact in the field.
There are many aspects of S4 yet to be explored in molecular science, such as its potential in longer sequences (e.g. macrocyclic peptide and protein sequences) and other molecular tasks (e.g. organic reaction planning and structure-based drug design).
In the future, the application of S4 in molecular discovery will continue to increase and may replace widely used chemical language models such as LSTM and GPT.
The above is the detailed content of 'The best of both worlds', designing molecules from scratch, deep learning architecture S4 for chemical language modeling. For more information, please follow other related articles on the PHP Chinese website!