


World models provide a way to train reinforcement learning agents in a safe and sample-efficient manner. Recently, world models have mainly operated on discrete latent variable sequences to simulate environmental dynamics.
However, this method of compressing into compact discrete representations may ignore visual details that are important for reinforcement learning. On the other hand, diffusion models have become the dominant method for image generation, posing challenges to discrete latent models.
To promote this paradigm shift, researchers from the University of Geneva, the University of Edinburgh, and Microsoft Research jointly proposed a reinforcement learning agent trained in a diffuse world model—DIAMOND (DIffusion As a Model Of eNvironment Dreams).
- ##Paper address: https://arxiv .org/abs/2405.12399
- Project address: https://github.com/eloialonso/diamond
- Paper title: Diffusion for World Modeling: Visual Details Matter in Atari
In the Atari 100k benchmark, DIAMOND+ achieved an average Human Normalized Score (HNS) of 1.46. This means that an agent trained in the world model can be fully trained at the SOTA level of an agent trained in the world model. This study provides a stability analysis to illustrate that DIAMOND design choices are necessary to ensure the long-term efficient stability of the diffusive world model.
In addition to the benefit of operating in image space, it enables the diffuse world model to become a direct representation of the environment, thus providing a deeper understanding of the world model and agent behavior. In particular, the study found that performance improvements in certain games result from better modeling of key visual details.
Method Introduction
Next, this article introduces DIAMOND, a reinforcement learning agent trained in a diffusion world model. Specifically, we base this on the drift and diffusion coefficients f and g introduced in Section 2.2, which correspond to a specific choice of diffusion paradigm. Furthermore, this study also chose the EDM formulation based on Karras et al.
First define a disturbance kernel, , where is a real-valued function related to the diffusion time, called the noise schedule. This corresponds to setting the drift and diffusion coefficients to
and
.
Then use the network preprocessing introduced by Karras et al. (2022), and parameterize in formula (5) as noise observations and neural network predictions Weighted sum of values:
includes all condition variables.
Preprocessor selection. Choose preprocessors and
to maintain unit variance of network inputs and outputs at any noise level
.
is the empirical conversion of noise level,
is given by
and the standard deviation of the data distribution
, the formula is
Combined with formula 5 and 6. Get the training target:
##This study uses standard U-Net 2D to construct the vector field, and retain a buffer containing the past L observations and actions to condition the model. Next they concatenated these past observations channel-wise with the next noisy observation and fed the actions into the residual block of U-Net via an adaptive group normalization layer. As discussed in Section 2.3 and Appendix A, there are many possible sampling methods to generate the next observation from a trained diffusion model. While the code base released by the study supports multiple sampling schemes, the study found that Euler methods are effective without requiring additional NFE (number of function evaluations) and avoiding the unnecessary complexity of higher-order samplers or random sampling. Effective.
Experiment
To fully evaluate DIAMOND, the study used the well-established Atari 100k benchmark, which included 26 games, using For testing the broad capabilities of an agent. For each game, the agent was only allowed 100k actions in the environment, which is roughly equivalent to 2 hours of human game time, to learn to play the game before being evaluated. For reference, an Atari agent without constraints is typically trained for 50 million steps, which corresponds to a 500-fold increase in experience. The researchers trained DIAMOND from scratch on each game using 5 random seeds. Each run used approximately 12GB of VRAM and took approximately 2.9 days on a single Nvidia RTX 4090 (1.03 GPU years total).
Table 1 compares different scores for training agents on the world model:
In order to study the stability of diffusion variables, this study analyzed the imagined trajectory generated by autoregression, as shown in Figure 3 below:
The study found that some situations require an iterative solver to drive the sampling process to a specific mode, such as the boxing game shown in Figure 4:
As shown in Figure 5, compared with the trajectories imagined by IRIS, the trajectories imagined by DIAMOND generally have higher visual quality and are more consistent with the real environment.
Interested readers can read the original text of the paper to learn more about the research content.
The above is the detailed content of The world model also spreads! The trained agent turns out to be pretty good. For more information, please follow other related articles on the PHP Chinese website!
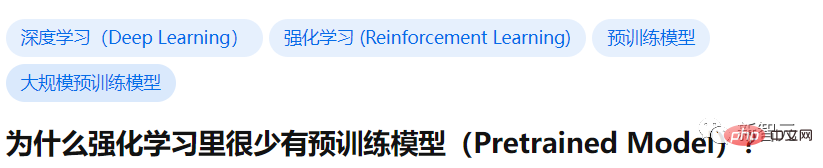
在当下的序列建模任务上,Transformer可谓是最强大的神经网络架构,并且经过预训练的Transformer模型可以将prompt作为条件或上下文学习(in-context learning)适应不同的下游任务。大型预训练Transformer模型的泛化能力已经在多个领域得到验证,如文本补全、语言理解、图像生成等等。从去年开始,已经有相关工作证明,通过将离线强化学习(offline RL)视为一个序列预测问题,那么模型就可以从离线数据中学习策略。但目前的方法要么是从不包含学习的数据中学习策略
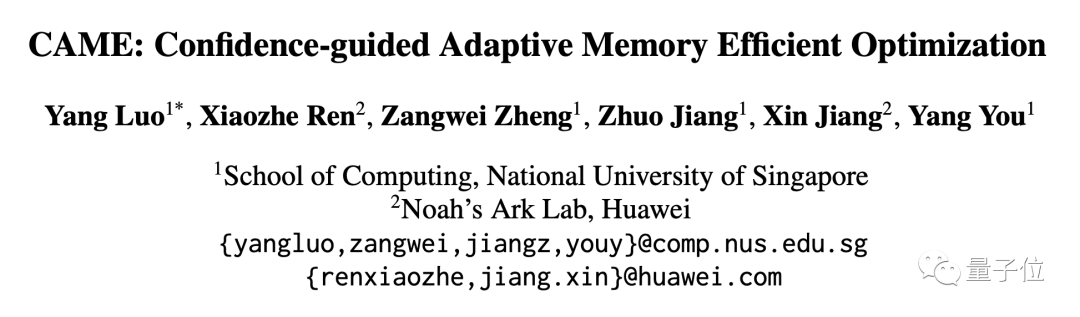
优化器在大语言模型的训练中占据了大量内存资源。现在有一种新的优化方式,在性能保持不变的情况下将内存消耗降低了一半。该成果由新加坡国立大学打造,在ACL会议上获得了杰出论文奖,并已经投入了实际应用。图片随着大语言模型不断增加的参数量,训练时的内存消耗问题更为严峻。研究团队提出了CAME优化器,在减少内存消耗的同时,拥有与Adam相同的性能。图片CAME优化器在多个常用的大规模语言模型的预训练上取得了相同甚至超越Adam优化器的训练表现,并对大batch预训练场景显示出更强的鲁棒性。进一步地,通过C
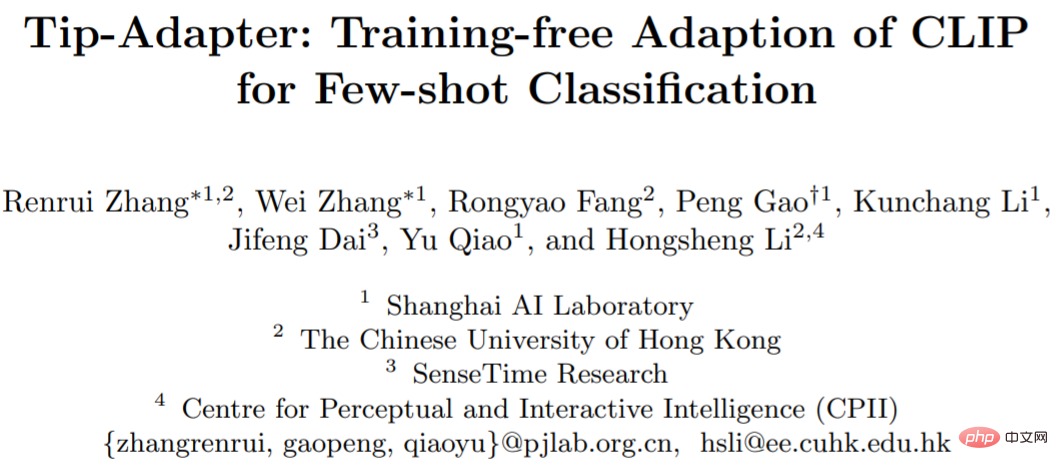
论文链接:https://arxiv.org/pdf/2207.09519.pdf代码链接:https://github.com/gaopengcuhk/Tip-Adapter一.研究背景对比性图像语言预训练模型(CLIP)在近期展现出了强大的视觉领域迁移能力,可以在一个全新的下游数据集上进行 zero-shot 图像识别。为了进一步提升 CLIP 的迁移性能,现有方法使用了 few-shot 的设置,例如 CoOp 和 CLIP-Adapter,即提供了少量下游数据集的训练数据,使得 CLIP
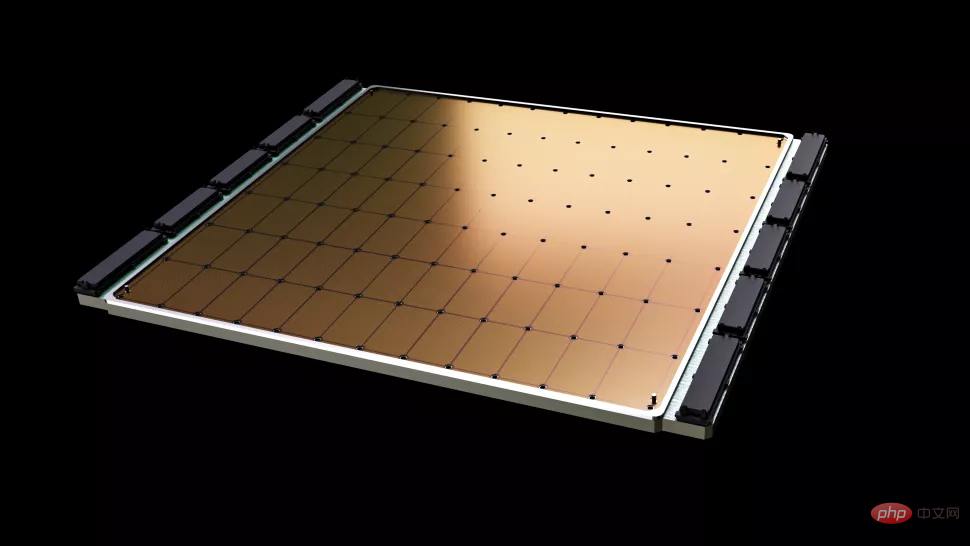
本周,芯片创业公司Cerebras宣布了一个里程碑式的新进展:在单个计算设备中训练了超过百亿参数的NLP(自然语言处理)人工智能模型。由Cerebras训练的AI模型体量达到了前所未有的200亿参数,所有这些都无需横跨多个加速器扩展工作负载。这项工作足以满足目前网络上最火的文本到图像AI生成模型——OpenAI的120亿参数大模型DALL-E。Cerebras新工作中最重要的一点是对基础设施和软件复杂性的要求降低了。这家公司提供的芯片WaferScaleEngine-
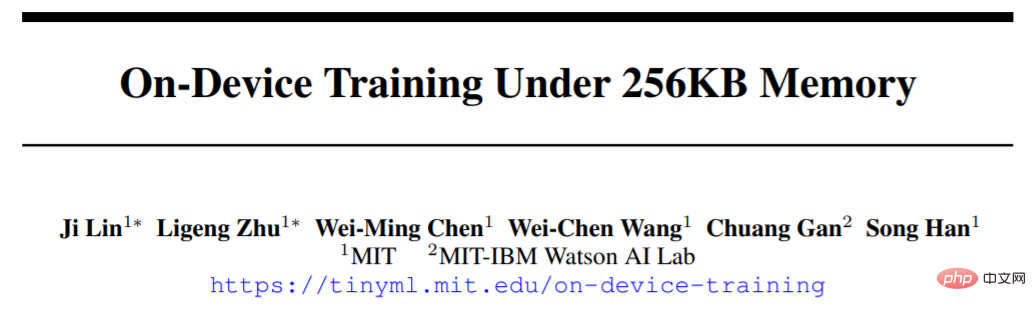
说到神经网络训练,大家的第一印象都是 GPU + 服务器 + 云平台。传统的训练由于其巨大的内存开销,往往是云端进行训练而边缘平台仅负责推理。然而,这样的设计使得 AI 模型很难适应新的数据:毕竟现实世界是一个动态的,变化的,发展的场景,一次训练怎么能覆盖所有场景呢?为了使得模型能够不断的适应新数据,我们能否在边缘进行训练(on-device training),使设备不断的自我学习?在这项工作中,我们仅用了不到 256KB 内存就实现了设备上的训练,开销不到 PyTorch 的 1/1000,
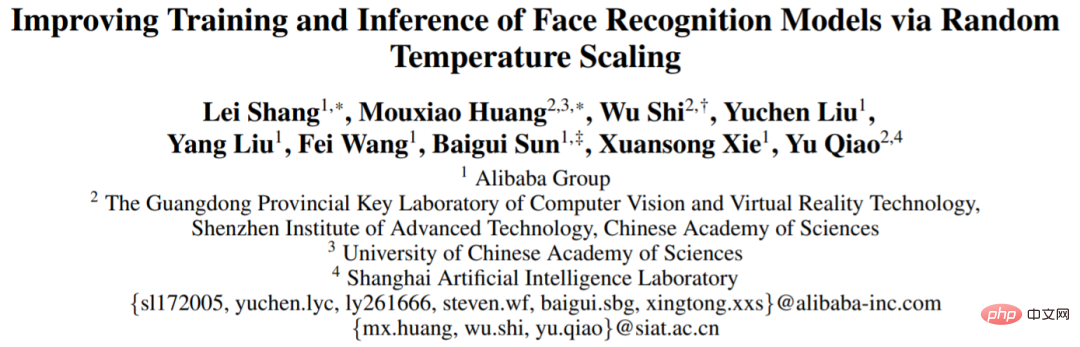
本文介绍被机器学习顶级国际会议AAAI2023接收的论文《ImprovingTrainingandInferenceofFaceRecognitionModelsviaRandomTemperatureScaling》。论文创新性地从概率视角出发,对分类损失函数中的温度调节参数和分类不确定度的内在关系进行分析,揭示了分类损失函数的温度调节因子是服从Gumbel分布的不确定度变量的尺度系数。从而提出一个新的被叫做RTS的训练框架对特征抽取的可靠性进行建模。基于RTS
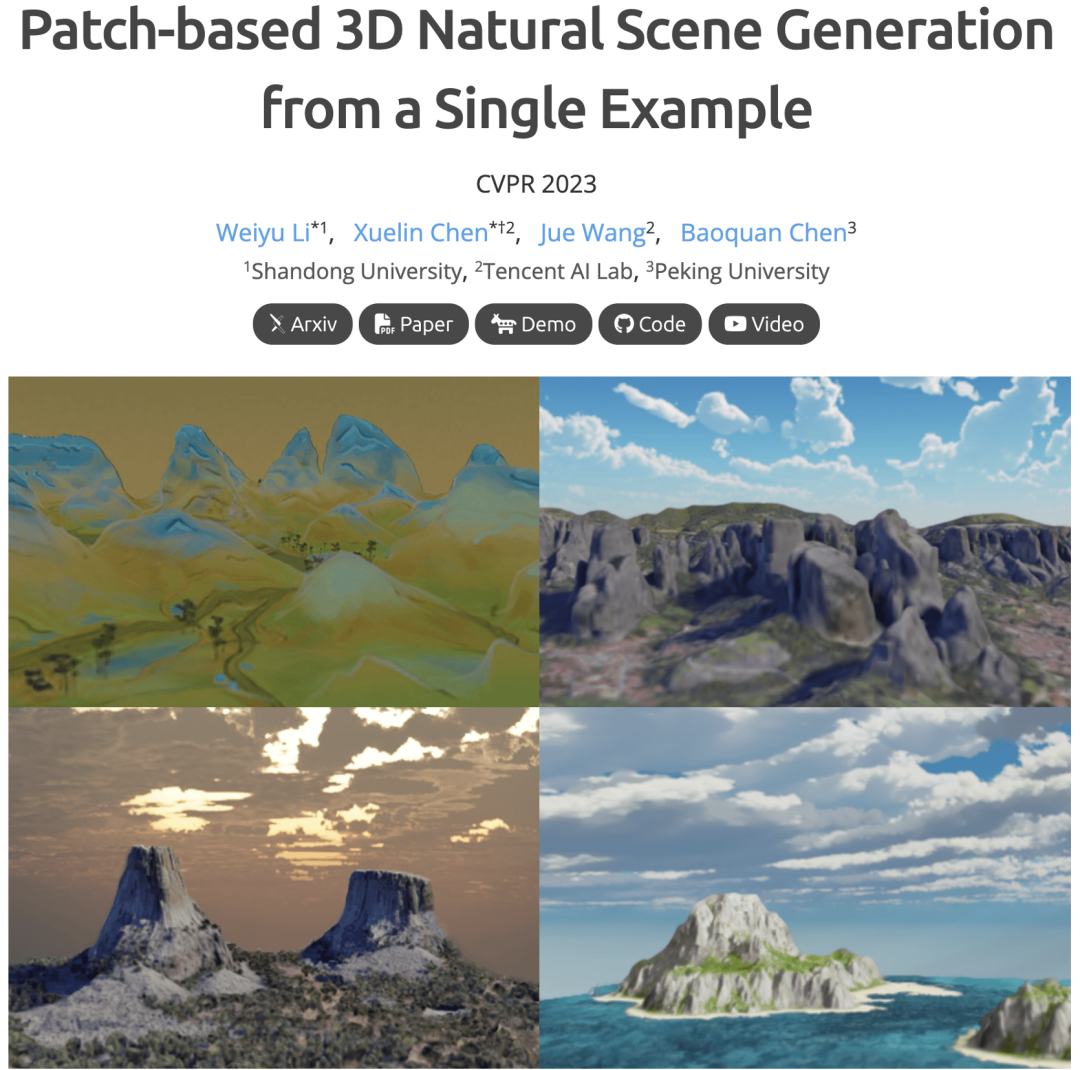
多样高质的三维场景生成结果论文地址:https://arxiv.org/abs/2304.12670项目主页:http://weiyuli.xyz/Sin3DGen/引言使用人工智能辅助内容生成(AIGC)在图像生成领域涌现出大量的工作,从早期的变分自编码器(VAE),到生成对抗网络(GAN),再到最近大红大紫的扩散模型(DiffusionModel),模型的生成能力飞速提升。以StableDiffusion,Midjourney等为代表的模型在生成具有高真实感图像方面取得了前所未有的成果。同时
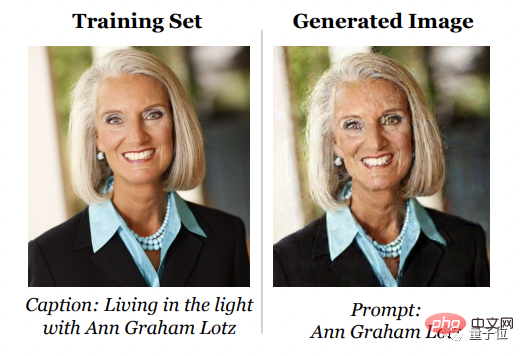
本文经AI新媒体量子位(公众号ID:QbitAI)授权转载,转载请联系出处。AI绘画侵权,实锤了!最新研究表明,扩散模型会牢牢记住训练集中的样本,并在生成时“依葫芦画瓢”。也就是说,像Stable Diffusion生成的AI画作里,每一笔背后都可能隐藏着一次侵权事件。不仅如此,经过研究对比,扩散模型从训练样本中“抄袭”的能力是GAN的2倍,且生成效果越好的扩散模型,记住训练样本的能力越强。这项研究来自Google、DeepMind和UC伯克利组成的团队。论文中还有另一个糟糕的消息,那就是针对这


Hot AI Tools

Undresser.AI Undress
AI-powered app for creating realistic nude photos

AI Clothes Remover
Online AI tool for removing clothes from photos.

Undress AI Tool
Undress images for free

Clothoff.io
AI clothes remover

AI Hentai Generator
Generate AI Hentai for free.

Hot Article

Hot Tools

DVWA
Damn Vulnerable Web App (DVWA) is a PHP/MySQL web application that is very vulnerable. Its main goals are to be an aid for security professionals to test their skills and tools in a legal environment, to help web developers better understand the process of securing web applications, and to help teachers/students teach/learn in a classroom environment Web application security. The goal of DVWA is to practice some of the most common web vulnerabilities through a simple and straightforward interface, with varying degrees of difficulty. Please note that this software

Atom editor mac version download
The most popular open source editor
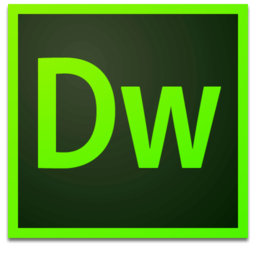
Dreamweaver Mac version
Visual web development tools

PhpStorm Mac version
The latest (2018.2.1) professional PHP integrated development tool

SecLists
SecLists is the ultimate security tester's companion. It is a collection of various types of lists that are frequently used during security assessments, all in one place. SecLists helps make security testing more efficient and productive by conveniently providing all the lists a security tester might need. List types include usernames, passwords, URLs, fuzzing payloads, sensitive data patterns, web shells, and more. The tester can simply pull this repository onto a new test machine and he will have access to every type of list he needs.
