There is my solutions to tackle the disk spaces shortage problem I described in the previous post. The core principle of the solution is to reduce the number of output records at Mapper stage; the method I used is Filter , adding a filter,
There is my solutions to tackle the disk spaces shortage problem I described in the previous post. The core principle of the solution is to reduce the number of output records at Mapper stage; the method I used is Filter, adding a filter, which I will explain later, to decrease the output records of Mapper, which in turn significantly decrease the Mapper’s Spill records, and fundamentally decrease the disk space usages. After applying the filter, with 30,661 records. some 200MB data set as inputs, the total Spill Records is 25,471,725, and it only takes about 509MB disk spaces!
Followed Filter
And now I’m going to reveal what’s kinda Filter it looks like, and how did I accomplish that filter. The true face of the FILTER is called Followed Filter, it filters users from computing co-followed combinations if their followed number does not satisfy a certain number, called Followed Threshold.
Followed Filter is used to reduce the co-followed combinations at Mapper stage. Say we set the followed threshold to 100, meaning users who doesn’t own 100 fans(be followed by 100 other users) will be ignored during co-followed combinations computing stage(to get the actual number of the threshold we need analyze statistics of user’s followed number of our data set).
Reason
Choosing followed filter is reasonable because how many user follows is a metric of user’s popularity/famousness.
HOW
In order to accomplish it, we need:
First, counting user’s followed number among our data set, which needs a new MapReduce Job;
Second, choosing a followed threshold after analyze the statistics perspective of followed number data set got in first step;
Third, using DistrbutedCache of Hadoop to cache users who satisfy the filter to all Mappers;
Forth, adding followed filter to Mapper class, only users satisfy filter condition will be passed into co-followed combination computing phrase;
Fifth, adding co-followed filter/threshold in Reducer side if necessary.
Outcomes
Here is the Hadoop Job Summary, after applying the followed filter with followed threshold of 1000, that means only users who are followed by 1000 users will have the opportunity to co-followed combinations, compared with the Job Summary in my previous post, most all metrics have significant improvements:
Counter | Map | Reduce | Total |
Bytes Written | 0 | 1,798,185 | 1,798,185 |
Bytes Read | 203,401,876 | 0 | 203,401,876 |
FILE_BYTES_READ | 405,219,906 | 52,107,486 | 457,327,392 |
HDFS_BYTES_READ | 203,402,751 | 0 | 203,402,751 |
FILE_BYTES_WRITTEN | 457,707,759 | 52,161,704 | 509,869,463 |
HDFS_BYTES_WRITTEN | 0 | 1,798,185 | 1,798,185 |
Reduce input groups | 0 | 373,680 | 373,680 |
Map output materialized bytes | 52,107,522 | 0 | 52,107,522 |
Combine output records | 22,202,756 | 0 | 22,202,756 |
Map input records | 30,661 | 0 | 30,661 |
Reduce shuffle bytes | 0 | 52,107,522 | 52,107,522 |
Physical memory (bytes) snapshot | 2,646,589,440 | 116,408,320 | 2,762,997,760 |
Reduce output records | 0 | 373,680 | 373,680 |
Spilled Records | 22,866,351 | 2,605,374 | 25,471,725 |
Map output bytes | 2,115,139,050 | 0 | 2,115,139,050 |
Total committed heap usage (bytes) | 2,813,853,696 | 84,738,048 | 2,898,591,744 |
CPU time spent (ms) | 5,766,680 | 11,210 | 5,777,890 |
Virtual memory (bytes) snapshot | 9,600,737,280 | 1,375,002,624 | 10,975,739,904 |
SPLIT_RAW_BYTES | 875 | 0 | 875 |
Map output records | 117,507,725 | 0 | 117,507,725 |
Combine input records | 137,105,107 | 0 | 137,105,107 |
Reduce input records | 0 | 2,605,374 | 2,605,374 |
P.S.
Frankly Speaking, chances are I am on the wrong way to Hadoop Programming, since I’m palying Pesudo Distribution Hadoop
with my personal computer, which has 4 CUPs and 4G RAM, in real Hadoop Cluster disk spaces might never be a trouble, and all the tuning work I have done may turn into meaningless efforts. Before the Followed Filter, I also did some Hadoop tuning like customed Writable class, RawComparator, block size and io.sort.mb, etc.
---EOF---
原文地址:Adding Filter in Hadoop Mapper Class, 感谢原作者分享。
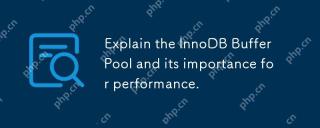
InnoDBBufferPool reduces disk I/O by caching data and indexing pages, improving database performance. Its working principle includes: 1. Data reading: Read data from BufferPool; 2. Data writing: After modifying the data, write to BufferPool and refresh it to disk regularly; 3. Cache management: Use the LRU algorithm to manage cache pages; 4. Reading mechanism: Load adjacent data pages in advance. By sizing the BufferPool and using multiple instances, database performance can be optimized.
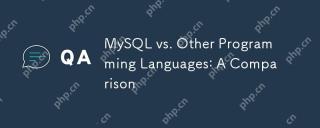
Compared with other programming languages, MySQL is mainly used to store and manage data, while other languages such as Python, Java, and C are used for logical processing and application development. MySQL is known for its high performance, scalability and cross-platform support, suitable for data management needs, while other languages have advantages in their respective fields such as data analytics, enterprise applications, and system programming.
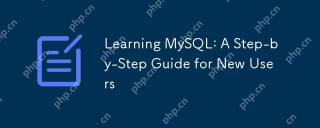
MySQL is worth learning because it is a powerful open source database management system suitable for data storage, management and analysis. 1) MySQL is a relational database that uses SQL to operate data and is suitable for structured data management. 2) The SQL language is the key to interacting with MySQL and supports CRUD operations. 3) The working principle of MySQL includes client/server architecture, storage engine and query optimizer. 4) Basic usage includes creating databases and tables, and advanced usage involves joining tables using JOIN. 5) Common errors include syntax errors and permission issues, and debugging skills include checking syntax and using EXPLAIN commands. 6) Performance optimization involves the use of indexes, optimization of SQL statements and regular maintenance of databases.
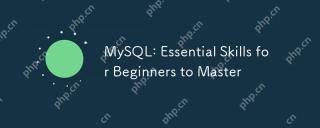
MySQL is suitable for beginners to learn database skills. 1. Install MySQL server and client tools. 2. Understand basic SQL queries, such as SELECT. 3. Master data operations: create tables, insert, update, and delete data. 4. Learn advanced skills: subquery and window functions. 5. Debugging and optimization: Check syntax, use indexes, avoid SELECT*, and use LIMIT.
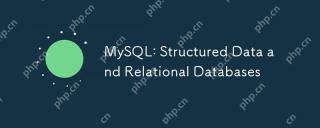
MySQL efficiently manages structured data through table structure and SQL query, and implements inter-table relationships through foreign keys. 1. Define the data format and type when creating a table. 2. Use foreign keys to establish relationships between tables. 3. Improve performance through indexing and query optimization. 4. Regularly backup and monitor databases to ensure data security and performance optimization.
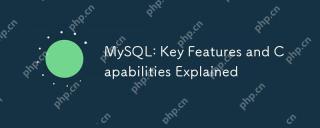
MySQL is an open source relational database management system that is widely used in Web development. Its key features include: 1. Supports multiple storage engines, such as InnoDB and MyISAM, suitable for different scenarios; 2. Provides master-slave replication functions to facilitate load balancing and data backup; 3. Improve query efficiency through query optimization and index use.
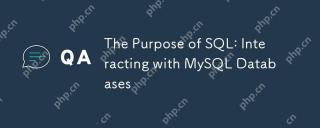
SQL is used to interact with MySQL database to realize data addition, deletion, modification, inspection and database design. 1) SQL performs data operations through SELECT, INSERT, UPDATE, DELETE statements; 2) Use CREATE, ALTER, DROP statements for database design and management; 3) Complex queries and data analysis are implemented through SQL to improve business decision-making efficiency.
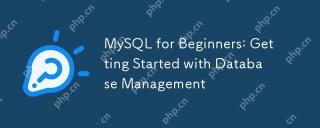
The basic operations of MySQL include creating databases, tables, and using SQL to perform CRUD operations on data. 1. Create a database: CREATEDATABASEmy_first_db; 2. Create a table: CREATETABLEbooks(idINTAUTO_INCREMENTPRIMARYKEY, titleVARCHAR(100)NOTNULL, authorVARCHAR(100)NOTNULL, published_yearINT); 3. Insert data: INSERTINTObooks(title, author, published_year)VA


Hot AI Tools

Undresser.AI Undress
AI-powered app for creating realistic nude photos

AI Clothes Remover
Online AI tool for removing clothes from photos.

Undress AI Tool
Undress images for free

Clothoff.io
AI clothes remover

Video Face Swap
Swap faces in any video effortlessly with our completely free AI face swap tool!

Hot Article

Hot Tools

mPDF
mPDF is a PHP library that can generate PDF files from UTF-8 encoded HTML. The original author, Ian Back, wrote mPDF to output PDF files "on the fly" from his website and handle different languages. It is slower than original scripts like HTML2FPDF and produces larger files when using Unicode fonts, but supports CSS styles etc. and has a lot of enhancements. Supports almost all languages, including RTL (Arabic and Hebrew) and CJK (Chinese, Japanese and Korean). Supports nested block-level elements (such as P, DIV),

SecLists
SecLists is the ultimate security tester's companion. It is a collection of various types of lists that are frequently used during security assessments, all in one place. SecLists helps make security testing more efficient and productive by conveniently providing all the lists a security tester might need. List types include usernames, passwords, URLs, fuzzing payloads, sensitive data patterns, web shells, and more. The tester can simply pull this repository onto a new test machine and he will have access to every type of list he needs.
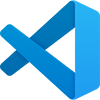
VSCode Windows 64-bit Download
A free and powerful IDE editor launched by Microsoft
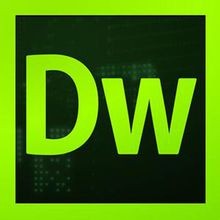
Dreamweaver CS6
Visual web development tools

MantisBT
Mantis is an easy-to-deploy web-based defect tracking tool designed to aid in product defect tracking. It requires PHP, MySQL and a web server. Check out our demo and hosting services.