随着大型语言模型(LLM)技术日渐成熟,提示工程(Prompt Engineering)变得越来越重要。一些研究机构发布了 LLM 提示工程指南,包括微软、OpenAI 等等。
最近,Meta 提供了一份交互式提示工程指南,专门针对他们的 Llama 2 开源模型。这份指南涵盖了使用 Llama 2 进行快速工程和最佳实践的知识。
以下是这份指南的核心内容。
Llama 模型
2023 年,Meta 推出了 Llama 、Llama 2 模型。较小的模型部署和运行成本较低,而更大的模型能力更强。
Llama 2 系列模型参数规模如下:
Code Llama 是一个以代码为中心的 LLM,建立在 Llama 2 的基础上,也有各种参数规模和微调变体:
部署 LLM
LLM 可以通过多种方式部署和访问,包括:
自托管(Self-hosting):使用本地硬件来运行推理,例如使用 llama.cpp 在 Macbook Pro 上运行 Llama 2。优势:自托管最适合有隐私 / 安全需要的情况,或者您拥有足够的 GPU。
云托管:依靠云提供商来部署托管特定模型的实例,例如通过 AWS、Azure、GCP 等云提供商来运行 Llama 2。优势:云托管是最适合自定义模型及其运行时的方式。
托管 API:通过 API 直接调用 LLM。有许多公司提供 Llama 2 推理 API,包括 AWS Bedrock、Replicate、Anyscale、Together 等。优势:托管 API 是总体上最简单的选择。
托管 API
托管 API 通常有两个主要端点(endpoint):
1. completion:生成对给定 prompt 的响应。
2. chat_completion:生成消息列表中的下一条消息,为聊天机器人等用例提供更明确的指令和上下文。
token
LLM 以称为 token 的块的形式来处理输入和输出,每个模型都有自己的 tokenization 方案。比如下面这句话:
Our destiny is written in the stars.
Llama 2 的 tokenization 为 ["our", "dest", "iny", "is", "writing", "in", "the", "stars"]。考虑 API 定价和内部行为(例如超参数)时,token 显得尤为重要。每个模型都有一个 prompt 不能超过的最大上下文长度,Llama 2 是 4096 个 token,而 Code Llama 是 100K 个 token。
Notebook 设置
作为示例,我们使用 Replicate 调用 Llama 2 chat,并使用 LangChain 轻松设置 chat completion API。
首先安装先决条件:
pip install langchain replicate
from typing import Dict, Listfrom langchain.llms import Replicatefrom langchain.memory import ChatMessageHistoryfrom langchain.schema.messages import get_buffer_stringimport os# Get a free API key from https://replicate.com/account/api-tokensos.environ ["REPLICATE_API_TOKEN"] = "YOUR_KEY_HERE"LLAMA2_70B_CHAT = "meta/llama-2-70b-chat:2d19859030ff705a87c746f7e96eea03aefb71f166725aee39692f1476566d48"LLAMA2_13B_CHAT = "meta/llama-2-13b-chat:f4e2de70d66816a838a89eeeb621910adffb0dd0baba3976c96980970978018d"# We'll default to the smaller 13B model for speed; change to LLAMA2_70B_CHAT for more advanced (but slower) generationsDEFAULT_MODEL = LLAMA2_13B_CHATdef completion (prompt: str,model: str = DEFAULT_MODEL,temperature: float = 0.6,top_p: float = 0.9,) -> str:llm = Replicate (model=model,model_kwargs={"temperature": temperature,"top_p": top_p, "max_new_tokens": 1000})return llm (prompt)def chat_completion (messages: List [Dict],model = DEFAULT_MODEL,temperature: float = 0.6,top_p: float = 0.9,) -> str:history = ChatMessageHistory ()for message in messages:if message ["role"] == "user":history.add_user_message (message ["content"])elif message ["role"] == "assistant":history.add_ai_message (message ["content"])else:raise Exception ("Unknown role")return completion (get_buffer_string (history.messages,human_prefix="USER",ai_prefix="ASSISTANT",),model,temperature,top_p,)def assistant (content: str):return { "role": "assistant", "content": content }def user (content: str):return { "role": "user", "content": content }def complete_and_print (prompt: str, model: str = DEFAULT_MODEL):print (f'==============\n {prompt}\n==============')response = completion (prompt, model)print (response, end='\n\n')
Completion API
complete_and_print ("The typical color of the sky is:")
complete_and_print ("which model version are you?")
Chat Completion 模型提供了与 LLM 互动的额外结构,将结构化消息对象数组而不是单个文本发送到 LLM。此消息列表为 LLM 提供了一些可以继续进行的「背景」或「历史」信息。
通常,每条消息都包含角色和内容:
具有系统角色的消息用于开发人员向 LLM 提供核心指令。
具有用户角色的消息通常是人工提供的消息。
具有助手角色的消息通常由 LLM 生成。
response = chat_completion (messages=[user ("My favorite color is blue."),assistant ("That's great to hear!"),user ("What is my favorite color?"),])print (response)# "Sure, I can help you with that! Your favorite color is blue."
LLM 超参数
LLM API 通常会采用影响输出的创造性和确定性的参数。在每一步中,LLM 都会生成 token 及其概率的列表。可能性最小的 token 会从列表中「剪切」(基于 top_p),然后从剩余候选者中随机(温度参数 temperature)选择一个 token。换句话说:top_p 控制生成中词汇的广度,温度控制词汇的随机性,温度参数 temperature 为 0 会产生几乎确定的结果。
def print_tuned_completion (temperature: float, top_p: float):response = completion ("Write a haiku about llamas", temperature=temperature, top_p=top_p)print (f'[temperature: {temperature} | top_p: {top_p}]\n {response.strip ()}\n')print_tuned_completion (0.01, 0.01)print_tuned_completion (0.01, 0.01)# These two generations are highly likely to be the sameprint_tuned_completion (1.0, 1.0)print_tuned_completion (1.0, 1.0)# These two generations are highly likely to be different
prompt 技巧
详细、明确的指令会比开放式 prompt 产生更好的结果:
complete_and_print (prompt="Describe quantum physics in one short sentence of no more than 12 words")# Returns a succinct explanation of quantum physics that mentions particles and states existing simultaneously.
我们可以给定使用规则和限制,以给出明确的指令。
- 风格化,例如:
- 向我解释一下这一点,就像儿童教育网络节目中教授小学生一样;
- 我是一名软件工程师,使用大型语言模型进行摘要。用 250 字概括以下文字;
- 像私家侦探一样一步步追查案件,给出你的答案。
- 格式化
使用要点;
以 JSON 对象形式返回;
使用较少的技术术语并用于工作交流中。
- 限制
- 仅使用学术论文;
- 切勿提供 2020 年之前的来源;
- 如果你不知道答案,就说你不知道。
以下是给出明确指令的例子:
complete_and_print ("Explain the latest advances in large language models to me.")# More likely to cite sources from 2017complete_and_print ("Explain the latest advances in large language models to me. Always cite your sources. Never cite sources older than 2020.")# Gives more specific advances and only cites sources from 2020
零样本 prompting
一些大型语言模型(例如 Llama 2)能够遵循指令并产生响应,而无需事先看过任务示例。没有示例的 prompting 称为「零样本 prompting(zero-shot prompting)」。例如:
complete_and_print ("Text: This was the best movie I've ever seen! \n The sentiment of the text is:")# Returns positive sentimentcomplete_and_print ("Text: The director was trying too hard. \n The sentiment of the text is:")# Returns negative sentiment
少样本 prompting
添加所需输出的具体示例通常会产生更加准确、一致的输出。这种方法称为「少样本 prompting(few-shot prompting)」。例如:
def sentiment (text):response = chat_completion (messages=[user ("You are a sentiment classifier. For each message, give the percentage of positive/netural/negative."),user ("I liked it"),assistant ("70% positive 30% neutral 0% negative"),user ("It could be better"),assistant ("0% positive 50% neutral 50% negative"),user ("It's fine"),assistant ("25% positive 50% neutral 25% negative"),user (text),])return responsedef print_sentiment (text):print (f'INPUT: {text}')print (sentiment (text))print_sentiment ("I thought it was okay")# More likely to return a balanced mix of positive, neutral, and negativeprint_sentiment ("I loved it!")# More likely to return 100% positiveprint_sentiment ("Terrible service 0/10")# More likely to return 100% negative
Role Prompting
Llama 2 在指定角色时通常会给出更一致的响应,角色为 LLM 提供了所需答案类型的背景信息。
例如,让 Llama 2 对使用 PyTorch 的利弊问题创建更有针对性的技术回答:
complete_and_print ("Explain the pros and cons of using PyTorch.")# More likely to explain the pros and cons of PyTorch covers general areas like documentation, the PyTorch community, and mentions a steep learning curvecomplete_and_print ("Your role is a machine learning expert who gives highly technical advice to senior engineers who work with complicated datasets. Explain the pros and cons of using PyTorch.")# Often results in more technical benefits and drawbacks that provide more technical details on how model layers
思维链
简单地添加一个「鼓励逐步思考」的短语可以显著提高大型语言模型执行复杂推理的能力(Wei et al. (2022)),这种方法称为 CoT 或思维链 prompting:
complete_and_print ("Who lived longer Elvis Presley or Mozart?")# Often gives incorrect answer of "Mozart"complete_and_print ("Who lived longer Elvis Presley or Mozart? Let's think through this carefully, step by step.")# Gives the correct answer "Elvis"
自洽性(Self-Consistency)
LLM 是概率性的,因此即使使用思维链,一次生成也可能会产生不正确的结果。自洽性通过从多次生成中选择最常见的答案来提高准确性(以更高的计算成本为代价):
import refrom statistics import modedef gen_answer ():response = completion ("John found that the average of 15 numbers is 40.""If 10 is added to each number then the mean of the numbers is?""Report the answer surrounded by three backticks, for example:```123```",model = LLAMA2_70B_CHAT)match = re.search (r'```(\d+)```', response)if match is None:return Nonereturn match.group (1)answers = [gen_answer () for i in range (5)]print (f"Answers: {answers}\n",f"Final answer: {mode (answers)}",)# Sample runs of Llama-2-70B (all correct):# [50, 50, 750, 50, 50]-> 50# [130, 10, 750, 50, 50] -> 50# [50, None, 10, 50, 50] -> 50
检索增强生成
有时我们可能希望在应用程序中使用事实知识,那么可以从开箱即用(即仅使用模型权重)的大模型中提取常见事实:
complete_and_print ("What is the capital of the California?", model = LLAMA2_70B_CHAT)# Gives the correct answer "Sacramento"
然而,LLM 往往无法可靠地检索更具体的事实或私人信息。模型要么声明它不知道,要么幻想出一个错误的答案:
complete_and_print ("What was the temperature in Menlo Park on December 12th, 2023?")# "I'm just an AI, I don't have access to real-time weather data or historical weather records."complete_and_print ("What time is my dinner reservation on Saturday and what should I wear?")# "I'm not able to access your personal information [..] I can provide some general guidance"
检索增强生成(RAG)是指在 prompt 中包含从外部数据库检索的信息(Lewis et al. (2020))。RAG 是将事实纳入 LLM 应用的有效方法,并且比微调更经济实惠,微调可能成本高昂并对基础模型的功能产生负面影响。
MENLO_PARK_TEMPS = {"2023-12-11": "52 degrees Fahrenheit","2023-12-12": "51 degrees Fahrenheit","2023-12-13": "51 degrees Fahrenheit",}def prompt_with_rag (retrived_info, question):complete_and_print (f"Given the following information: '{retrived_info}', respond to: '{question}'")def ask_for_temperature (day):temp_on_day = MENLO_PARK_TEMPS.get (day) or "unknown temperature"prompt_with_rag (f"The temperature in Menlo Park was {temp_on_day} on {day}'",# Retrieved factf"What is the temperature in Menlo Park on {day}?",# User question)ask_for_temperature ("2023-12-12")# "Sure! The temperature in Menlo Park on 2023-12-12 was 51 degrees Fahrenheit."ask_for_temperature ("2023-07-18")# "I'm not able to provide the temperature in Menlo Park on 2023-07-18 as the information provided states that the temperature was unknown."
程序辅助语言模型
LLM 本质上不擅长执行计算,例如:
complete_and_print ("""Calculate the answer to the following math problem:((-5 + 93 * 4 - 0) * (4^4 + -7 + 0 * 5))""")# Gives incorrect answers like 92448, 92648, 95463
Gao et al. (2022) 提出「程序辅助语言模型(Program-aided Language Models,PAL)」的概念。虽然 LLM 不擅长算术,但它们非常擅长代码生成。PAL 通过指示 LLM 编写代码来解决计算任务。
complete_and_print ("""# Python code to calculate: ((-5 + 93 * 4 - 0) * (4^4 + -7 + 0 * 5))""",model="meta/codellama-34b:67942fd0f55b66da802218a19a8f0e1d73095473674061a6ea19f2dc8c053152")
# The following code was generated by Code Llama 34B:num1 = (-5 + 93 * 4 - 0)num2 = (4**4 + -7 + 0 * 5)answer = num1 * num2print (answer)
以上是Meta官方的Prompt工程指南:Llama 2这样用更高效的详细内容。更多信息请关注PHP中文网其他相关文章!
![无法使用chatgpt!解释可以立即测试的原因和解决方案[最新2025]](https://img.php.cn/upload/article/001/242/473/174717025174979.jpg?x-oss-process=image/resize,p_40)
ChatGPT无法访问?本文提供多种实用解决方案!许多用户在日常使用ChatGPT时,可能会遇到无法访问或响应缓慢等问题。本文将根据不同情况,逐步指导您解决这些问题。 ChatGPT无法访问的原因及初步排查 首先,我们需要确定问题是出在OpenAI服务器端,还是用户自身网络或设备问题。 请按照以下步骤进行排查: 步骤1:检查OpenAI官方状态 访问OpenAI Status页面 (status.openai.com),查看ChatGPT服务是否正常运行。如果显示红色或黄色警报,则表示Open
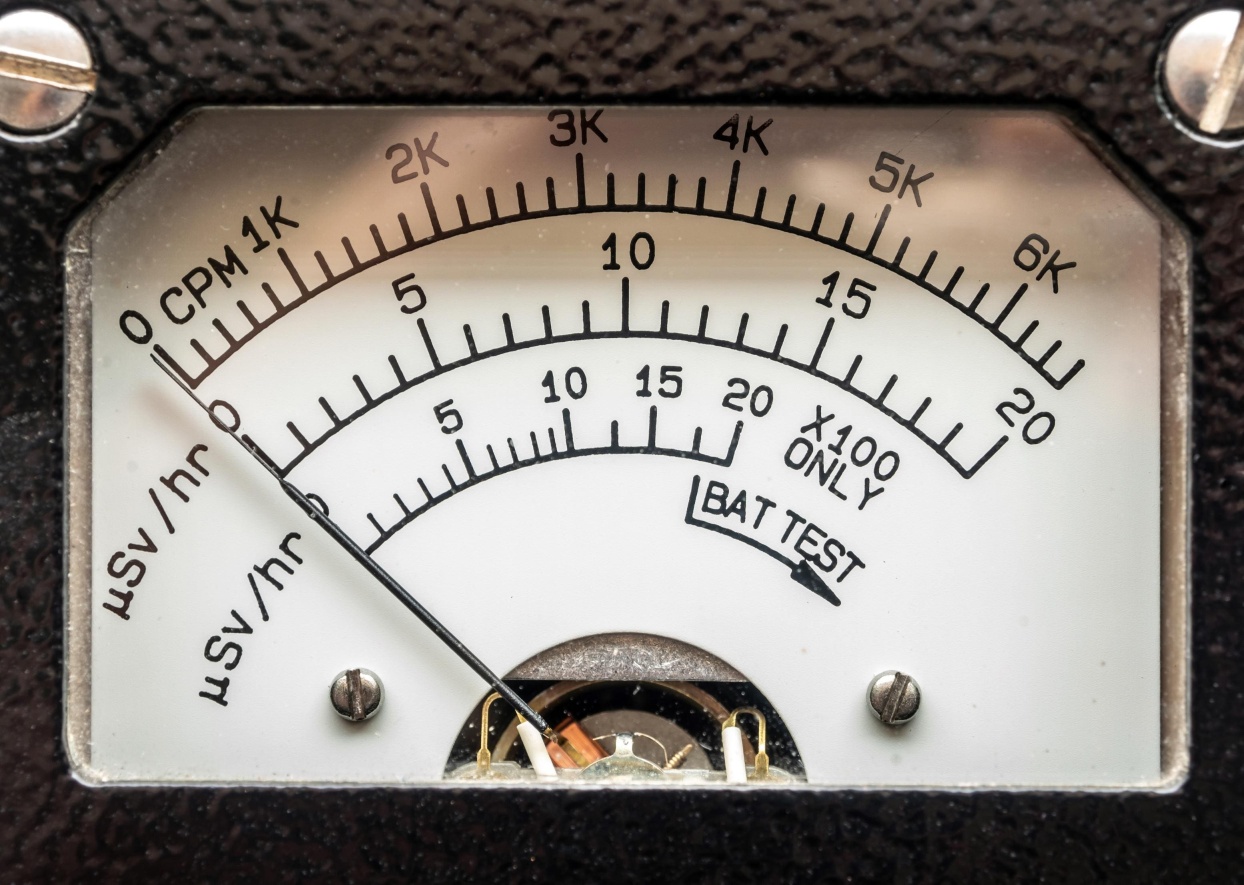
2025年5月10日,麻省理工学院物理学家Max Tegmark告诉《卫报》,AI实验室应在释放人工超级智能之前模仿Oppenheimer的三位一体测试演算。 “我的评估是'康普顿常数',这是一场比赛的可能性
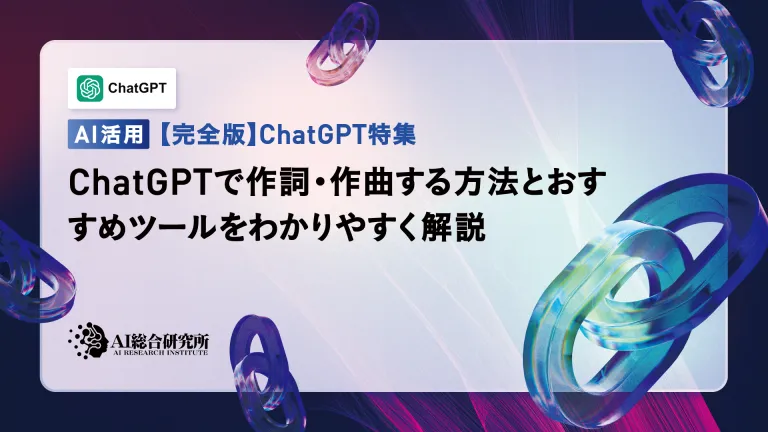
AI音乐创作技术日新月异,本文将以ChatGPT等AI模型为例,详细讲解如何利用AI辅助音乐创作,并辅以实际案例进行说明。我们将分别介绍如何通过SunoAI、Hugging Face上的AI jukebox以及Python的Music21库进行音乐创作。 通过这些技术,每个人都能轻松创作原创音乐。但需注意,AI生成内容的版权问题不容忽视,使用时务必谨慎。 让我们一起探索AI在音乐领域的无限可能! OpenAI最新AI代理“OpenAI Deep Research”介绍: [ChatGPT]Ope
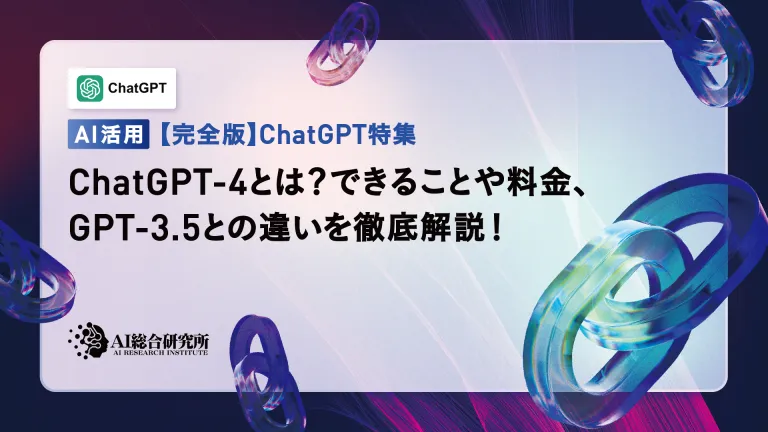
ChatGPT-4的出现,极大地拓展了AI应用的可能性。相较于GPT-3.5,ChatGPT-4有了显着提升,它具备强大的语境理解能力,还能识别和生成图像,堪称万能的AI助手。在提高商业效率、辅助创作等诸多领域,它都展现出巨大的潜力。然而,与此同时,我们也必须注意其使用上的注意事项。 本文将详细解读ChatGPT-4的特性,并介绍针对不同场景的有效使用方法。文中包含充分利用最新AI技术的技巧,敬请参考。 OpenAI发布的最新AI代理,“OpenAI Deep Research”详情请点击下方链
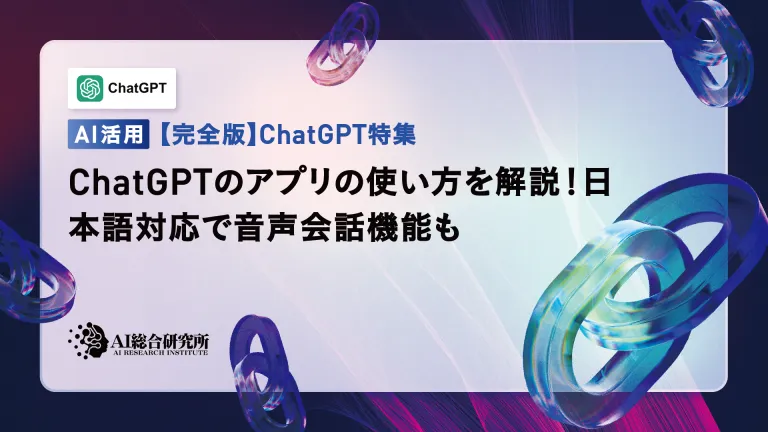
CHATGPT应用程序:与AI助手释放您的创造力!初学者指南 ChatGpt应用程序是一位创新的AI助手,可处理各种任务,包括写作,翻译和答案。它是一种具有无限可能性的工具,可用于创意活动和信息收集。 在本文中,我们将以一种易于理解的方式解释初学者,从如何安装chatgpt智能手机应用程序到语音输入功能和插件等应用程序所独有的功能,以及在使用该应用时要牢记的要点。我们还将仔细研究插件限制和设备对设备配置同步
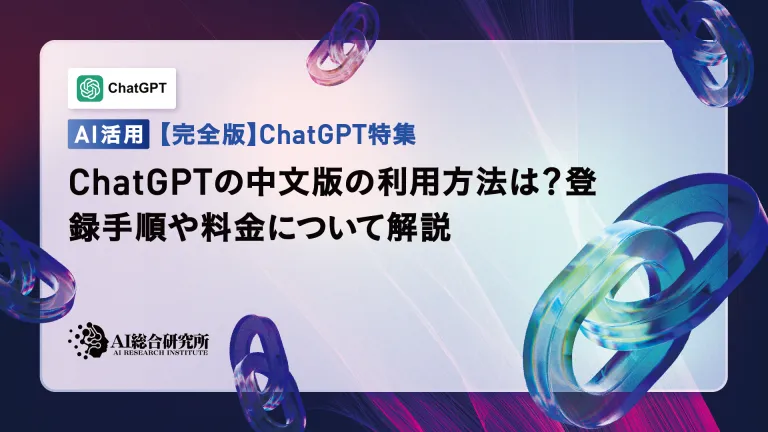
ChatGPT中文版:解锁中文AI对话新体验 ChatGPT风靡全球,您知道它也提供中文版本吗?这款强大的AI工具不仅支持日常对话,还能处理专业内容,并兼容简体中文和繁体中文。无论是中国地区的使用者,还是正在学习中文的朋友,都能从中受益。 本文将详细介绍ChatGPT中文版的使用方法,包括账户设置、中文提示词输入、过滤器的使用、以及不同套餐的选择,并分析潜在风险及应对策略。此外,我们还将对比ChatGPT中文版和其他中文AI工具,帮助您更好地了解其优势和应用场景。 OpenAI最新发布的AI智能
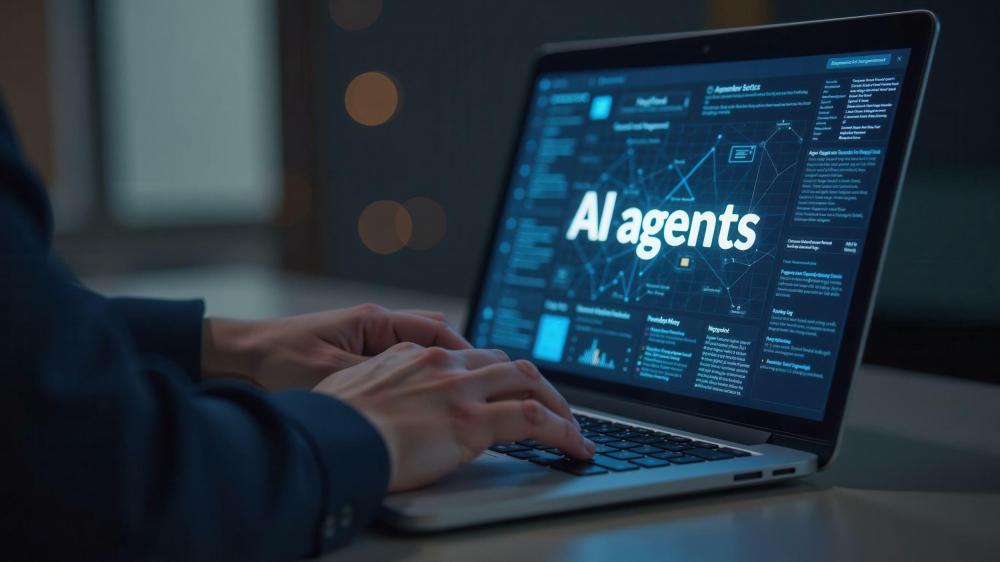
这些可以将其视为生成AI领域的下一个飞跃,这为我们提供了Chatgpt和其他大型语言模型聊天机器人。他们可以代表我们采取行动,而不是简单地回答问题或产生信息
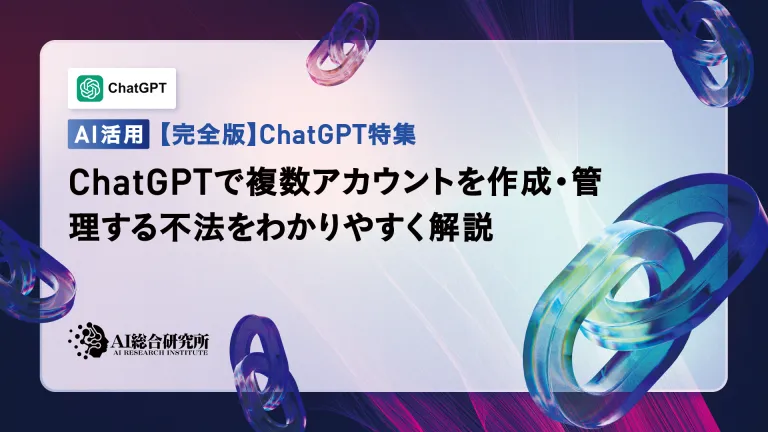
使用chatgpt有效的多个帐户管理技术|关于如何使用商业和私人生活的详尽解释! Chatgpt在各种情况下都使用,但是有些人可能担心管理多个帐户。本文将详细解释如何为ChatGpt创建多个帐户,使用时该怎么做以及如何安全有效地操作它。我们还介绍了重要的一点,例如业务和私人使用差异,并遵守OpenAI的使用条款,并提供指南,以帮助您安全地利用多个帐户。 Openai


热AI工具

Undresser.AI Undress
人工智能驱动的应用程序,用于创建逼真的裸体照片

AI Clothes Remover
用于从照片中去除衣服的在线人工智能工具。

Undress AI Tool
免费脱衣服图片

Clothoff.io
AI脱衣机

Video Face Swap
使用我们完全免费的人工智能换脸工具轻松在任何视频中换脸!

热门文章

热工具

MinGW - 适用于 Windows 的极简 GNU
这个项目正在迁移到osdn.net/projects/mingw的过程中,你可以继续在那里关注我们。MinGW:GNU编译器集合(GCC)的本地Windows移植版本,可自由分发的导入库和用于构建本地Windows应用程序的头文件;包括对MSVC运行时的扩展,以支持C99功能。MinGW的所有软件都可以在64位Windows平台上运行。

禅工作室 13.0.1
功能强大的PHP集成开发环境
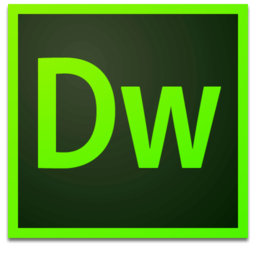
Dreamweaver Mac版
视觉化网页开发工具

DVWA
Damn Vulnerable Web App (DVWA) 是一个PHP/MySQL的Web应用程序,非常容易受到攻击。它的主要目标是成为安全专业人员在合法环境中测试自己的技能和工具的辅助工具,帮助Web开发人员更好地理解保护Web应用程序的过程,并帮助教师/学生在课堂环境中教授/学习Web应用程序安全。DVWA的目标是通过简单直接的界面练习一些最常见的Web漏洞,难度各不相同。请注意,该软件中
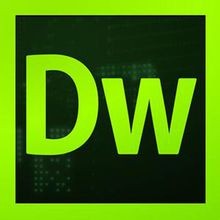
Dreamweaver CS6
视觉化网页开发工具