计算机科学中良好的问题解决很大程度上依赖于高效的算法,例如贪婪最佳优先搜索(GBFS)。 GBFS 已经确立了作为寻路或优化问题的最佳解决方法的可信度。因此,我们在本文中深入讨论 GBFS,同时探索其使用 C++ 的实现方法。
语法
void greedyBestFirstSearch(Graph graph, Node startNode, Node goalNode);
算法
贪心最佳优先搜索算法旨在找到图中从给定起始节点到目标节点的路径。以下是该算法的一般步骤 -
初始化一个空的优先级队列。
将起始节点放入优先级队列。
创建一个空集来跟踪访问过的节点。
当优先级队列不为空时 -
将优先级最高的节点从队列中出列。
如果出队的节点是目标节点,则算法终止,并找到路径。
否则,将出队节点标记为已访问。
将出队节点的所有未访问的邻居节点放入优先级队列中。
如果优先级队列在到达目标节点之前变空,则不存在路径。
方法一:基于欧氏距离的启发式函数
示例
#include <iostream> #include <queue> #include <cmath> #include <vector> #include <unordered_set> using namespace std; // Structure to represent a node in the graph struct Node { int x, y; // Coordinates of the node int cost; // Cost to reach this node }; // Euclidean distance heuristic function double euclideanDistance(int x1, int y1, int x2, int y2) { return sqrt(pow((x1 - x2), 2) + pow((y1 - y2), 2)); } // Custom comparison function for nodes in the priority queue struct NodeCompare { bool operator()(const Node& node1, const Node& node2) const { return node1.cost > node2.cost; } }; // Greedy Best-First Search function void greedyBestFirstSearch(vector<vector<int>>& graph, Node start, Node goal) { int rows = graph.size(); int cols = graph[0].size(); // Priority queue for nodes to be explored priority_queue<Node, vector<Node>, NodeCompare> pq; // Visited nodes set unordered_set<int> visited; // Add the start node to the priority queue pq.push(start); while (!pq.empty()) { // Get the node with the lowest cost Node current = pq.top(); pq.pop(); // Check if the current node is the goal node if (current.x == goal.x && current.y == goal.y) { cout << "Goal node reached!" << endl; return; } // Mark the current node as visited int nodeId = current.x * cols + current.y; visited.insert(nodeId); // Explore the neighboring nodes int dx[] = {-1, 1, 0, 0}; // Possible x-direction movements int dy[] = {0, 0, -1, 1}; // Possible y-direction movements for (int i = 0; i < 4; i++) { int newX = current.x + dx[i]; int newY = current.y + dy[i]; // Check if the neighboring node is within the graph boundaries if (newX >= 0 && newX < rows && newY >= 0 && newY < cols) { // Calculate the heuristic value for the neighboring node double heuristicValue = euclideanDistance(newX, newY, goal.x, goal.y); // Check if the neighboring node has not been visited if (visited.find(newX * cols + newY) == visited.end()) { // Create a new node for the neighboring position Node neighbor; neighbor.x = newX; neighbor.y = newY; neighbor.cost = current.cost + graph[newX][newY]; // Add the neighboring node to the priority queue pq.push(neighbor); } } } } cout << "Goal node not reachable!" << endl; } int main() { // Example graph represented as a 2D vector vector<vector<int>> graph = { {3, 5, 1, 2}, {1, 3, 2, 4}, {5, 2, 6, 7}, {4, 3, 1, 2} }; Node start; start.x = 0; // Starting x-coordinate start.y = 0; // Starting y-coordinate start.cost = 0; // Cost to reach the starting node Node goal; goal.x = 3; // Goal x-coordinate goal.y = 3; // Goal y-coordinate // Run Greedy Best-First Search algorithm greedyBestFirstSearch(graph, start, goal); return 0; }
输出
Goal node reached!
说明
这段代码包含两个关键元素。首先,它包含 Graph 类的定义,该类表示使用邻接表的图结构。
其次,它引入了 CompareEuclideanDistance - 一个自定义比较器,用于通过使用欧几里德距离公式估计节点与目标节点的距离来评估节点。
greedyBestFirstSearch 函数实现贪婪最佳优先搜索算法。它使用优先级队列根据节点的启发值来存储节点。
该算法首先将起始节点放入优先级队列中。
在每次迭代中,它将最高优先级的节点出队并检查它是否是目标节点。
如果找到目标节点,则会显示“路径已找到!”消息被打印。否则,算法将出队的节点标记为已访问,并将其未访问的相邻节点放入队列。
如果优先级队列变空而没有找到目标节点,则会显示“不存在路径!”消息已打印。
main函数通过创建图、定义起始节点和目标节点以及调用greedyBestFirstSearch函数演示了算法的用法。
方法2:基于曼哈顿距离的启发式函数
我们解决此问题的策略需要使用依赖于曼哈顿距离概念的启发式函数。这种距离度量有时称为出租车距离,涉及将节点之间的水平和垂直距离相加。
示例
#include <iostream> #include <queue> #include <cmath> #include <vector> #include <unordered_set> using namespace std; // Structure to represent a node in the graph struct Node { int x, y; // Coordinates of the node int cost; // Cost to reach this node }; // Manhattan distance heuristic function int manhattanDistance(int x1, int y1, int x2, int y2) { return abs(x1 - x2) + abs(y1 - y2); } // Custom comparison function for nodes in the priority queue struct NodeCompare { bool operator()(const Node& node1, const Node& node2) const { return node1.cost > node2.cost; } }; // Greedy Best-First Search function void greedyBestFirstSearch(vector<vector<int>>& graph, Node start, Node goal) { int rows = graph.size(); int cols = graph[0].size(); // Priority queue for nodes to be explored priority_queue<Node, vector<Node>, NodeCompare> pq; // Visited nodes set unordered_set<int> visited; // Add the start node to the priority queue pq.push(start); while (!pq.empty()) { // Get the node with the lowest cost Node current = pq.top(); pq.pop(); // Check if the current node is the goal node if (current.x == goal.x && current.y == goal.y) { cout << "Goal node reached!" << endl; return; } // Mark the current node as visited int nodeId = current.x * cols + current.y; visited.insert(nodeId); // Explore the neighboring nodes int dx[] = {-1, 1, 0, 0}; // Possible x-direction movements int dy[] = {0, 0, -1, 1}; // Possible y-direction movements for (int i = 0; i < 4; i++) { int newX = current.x + dx[i]; int newY = current.y + dy[i]; // Check if the neighboring node is within the graph boundaries if (newX >= 0 && newX < rows && newY >= 0 && newY < cols) { // Calculate the heuristic value for the neighboring node int heuristicValue = manhattanDistance(newX, newY, goal.x, goal.y); // Check if the neighboring node has not been visited if (visited.find(newX * cols + newY) == visited.end()) { // Create a new node for the neighboring position Node neighbor; neighbor.x = newX; neighbor.y = newY; neighbor.cost = current.cost + graph[newX][newY]; // Add the neighboring node to the priority queue pq.push(neighbor); } } } } cout << "Goal node not reachable!" << endl; } int main() { // Example graph represented as a 2D vector vector<vector<int>> graph = { {3, 5, 1, 2}, {1, 3, 2, 4}, {5, 2, 6, 7}, {4, 3, 1, 2} }; Node start; start.x = 0; // Starting x-coordinate start.y = 0; // Starting y-coordinate start.cost = 0; // Cost to reach the starting node Node goal; goal.x = 3; // Goal x-coordinate goal.y = 3; // Goal y-coordinate // Run Greedy Best-First Search algorithm greedyBestFirstSearch(graph, start, goal); return 0; }
输出
Goal node reached!
说明
该代码遵循与方法 1 类似的结构,但使用自定义比较器 CompareManhattanDistance,该比较器使用曼哈顿距离公式根据到目标节点的估计距离来比较节点。
greedyBestFirstSearch 函数使用曼哈顿距离启发式实现贪婪最佳优先搜索算法。
main函数演示了算法的使用,创建一个图,定义起始节点和目标节点,并调用greedyBestFirstSearch函数。
结论
在本文中,我们探讨了贪婪最佳优先搜索算法及其在 C++ 中的实现。通过采用这些方法,程序员可以有效地找到图中的路径并解决优化问题。启发式函数的选择,例如欧氏距离或曼哈顿距离,可以显着影响算法在不同场景下的性能。
以上是贪婪最佳优先搜索算法(Greedy Best-First Search Algorithm)在C++中的实现的详细内容。更多信息请关注PHP中文网其他相关文章!
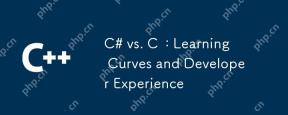
C#和C 的学习曲线和开发者体验有显着差异。 1)C#的学习曲线较平缓,适合快速开发和企业级应用。 2)C 的学习曲线较陡峭,适用于高性能和低级控制的场景。
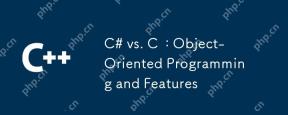
C#和C 在面向对象编程(OOP)中的实现方式和特性上有显着差异。 1)C#的类定义和语法更为简洁,支持如LINQ等高级特性。 2)C 提供更细粒度的控制,适用于系统编程和高性能需求。两者各有优势,选择应基于具体应用场景。
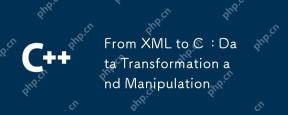
从XML转换到C 并进行数据操作可以通过以下步骤实现:1)使用tinyxml2库解析XML文件,2)将数据映射到C 的数据结构中,3)使用C 标准库如std::vector进行数据操作。通过这些步骤,可以高效地处理和操作从XML转换过来的数据。
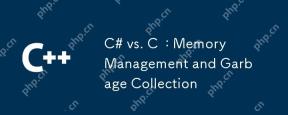
C#使用自动垃圾回收机制,而C 采用手动内存管理。1.C#的垃圾回收器自动管理内存,减少内存泄漏风险,但可能导致性能下降。2.C 提供灵活的内存控制,适合需要精细管理的应用,但需谨慎处理以避免内存泄漏。

C 在现代编程中仍然具有重要相关性。1)高性能和硬件直接操作能力使其在游戏开发、嵌入式系统和高性能计算等领域占据首选地位。2)丰富的编程范式和现代特性如智能指针和模板编程增强了其灵活性和效率,尽管学习曲线陡峭,但其强大功能使其在今天的编程生态中依然重要。
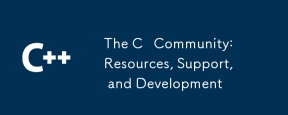
C 学习者和开发者可以从StackOverflow、Reddit的r/cpp社区、Coursera和edX的课程、GitHub上的开源项目、专业咨询服务以及CppCon等会议中获得资源和支持。1.StackOverflow提供技术问题的解答;2.Reddit的r/cpp社区分享最新资讯;3.Coursera和edX提供正式的C 课程;4.GitHub上的开源项目如LLVM和Boost提升技能;5.专业咨询服务如JetBrains和Perforce提供技术支持;6.CppCon等会议有助于职业

C#适合需要高开发效率和跨平台支持的项目,而C 适用于需要高性能和底层控制的应用。1)C#简化开发,提供垃圾回收和丰富类库,适合企业级应用。2)C 允许直接内存操作,适用于游戏开发和高性能计算。
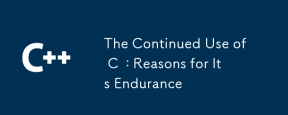
C 持续使用的理由包括其高性能、广泛应用和不断演进的特性。1)高效性能:通过直接操作内存和硬件,C 在系统编程和高性能计算中表现出色。2)广泛应用:在游戏开发、嵌入式系统等领域大放异彩。3)不断演进:自1983年发布以来,C 持续增加新特性,保持其竞争力。


热AI工具

Undresser.AI Undress
人工智能驱动的应用程序,用于创建逼真的裸体照片

AI Clothes Remover
用于从照片中去除衣服的在线人工智能工具。

Undress AI Tool
免费脱衣服图片

Clothoff.io
AI脱衣机

AI Hentai Generator
免费生成ai无尽的。

热门文章

热工具
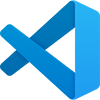
VSCode Windows 64位 下载
微软推出的免费、功能强大的一款IDE编辑器

记事本++7.3.1
好用且免费的代码编辑器

MinGW - 适用于 Windows 的极简 GNU
这个项目正在迁移到osdn.net/projects/mingw的过程中,你可以继续在那里关注我们。MinGW:GNU编译器集合(GCC)的本地Windows移植版本,可自由分发的导入库和用于构建本地Windows应用程序的头文件;包括对MSVC运行时的扩展,以支持C99功能。MinGW的所有软件都可以在64位Windows平台上运行。
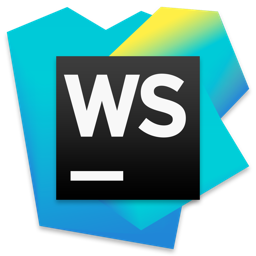
WebStorm Mac版
好用的JavaScript开发工具

SublimeText3 Linux新版
SublimeText3 Linux最新版