探索我的亚马逊作者页面,获取多种书籍选择。 在 Medium 上关注我以获取更多见解和更新!非常感谢您的支持。
释放 Python 多线程和多处理功能的强大功能,显着提高应用程序的速度和效率。本指南揭示了有效利用这些功能的八项基本技术。
线程在 I/O 密集型操作方面表现出色。 Python 的 threading
模块为线程管理提供了一个用户友好的界面。 以下是同时下载多个文件的方法:
import threading import requests def download_file(url): response = requests.get(url) filename = url.split('/')[-1] with open(filename, 'wb') as f: f.write(response.content) print(f"Downloaded {filename}") urls = ['http://example.com/file1.txt', 'http://example.com/file2.txt', 'http://example.com/file3.txt'] threads = [] for url in urls: thread = threading.Thread(target=download_file, args=(url,)) threads.append(thread) thread.start() for thread in threads: thread.join() print("All downloads complete")
此代码将每个下载分配给一个单独的线程,从而实现同时执行。
对于 CPU 密集型任务,由于 Python 的全局解释器锁 (GIL),multiprocessing
模块更加优越。 多处理创建独立的进程,每个进程都有自己的内存空间和 GIL,避免了 GIL 的限制。 这是并行计算的示例:
import multiprocessing def calculate_square(number): return number * number if __name__ == '__main__': numbers = range(10) with multiprocessing.Pool() as pool: results = pool.map(calculate_square, numbers) print(results)
这利用进程池来有效地分配计算。
concurrent.futures
模块为异步任务执行提供了更高级别的抽象,与线程和进程无缝协作。 这是使用 ThreadPoolExecutor
的示例:
from concurrent.futures import ThreadPoolExecutor import time def worker(n): print(f"Worker {n} starting") time.sleep(2) print(f"Worker {n} finished") with ThreadPoolExecutor(max_workers=3) as executor: executor.map(worker, range(5)) print("All workers complete")
这将创建一个线程池来管理五个工作任务。
对于异步 I/O,asyncio
模块大放异彩,可以使用协程实现高效的异步编程。这是一个例子:
import asyncio import aiohttp async def fetch_url(url): async with aiohttp.ClientSession() as session: async with session.get(url) as response: return await response.text() async def main(): urls = ['http://example.com', 'http://example.org', 'http://example.net'] tasks = [fetch_url(url) for url in urls] results = await asyncio.gather(*tasks) for url, result in zip(urls, results): print(f"Content length of {url}: {len(result)}") asyncio.run(main())
这可以有效地同时从多个 URL 获取内容。
进程之间的数据共享需要特定的工具。 multiprocessing
模块为共享内存提供了类似 Value
的机制:
from multiprocessing import Process, Value import time def increment(counter): for _ in range(100): with counter.get_lock(): counter.value += 1 time.sleep(0.01) if __name__ == '__main__': counter = Value('i', 0) processes = [Process(target=increment, args=(counter,)) for _ in range(4)] for p in processes: p.start() for p in processes: p.join() print(f"Final counter value: {counter.value}")
这展示了跨多个进程的安全计数器增量。
线程同步可防止多个线程访问共享资源时出现竞争情况。 Python 提供了同步原语,例如 Lock
:
import threading class Counter: def __init__(self): self.count = 0 self.lock = threading.Lock() def increment(self): with self.lock: self.count += 1 def worker(counter, num_increments): for _ in range(num_increments): counter.increment() counter = Counter() threads = [] for _ in range(5): thread = threading.Thread(target=worker, args=(counter, 100000)) threads.append(thread) thread.start() for thread in threads: thread.join() print(f"Final count: {counter.count}")
此示例使用锁来确保原子计数器增量。
ProcessPoolExecutor
非常适合 CPU 密集型任务。 这是查找素数的示例:
from concurrent.futures import ProcessPoolExecutor import math def is_prime(n): if n <= 1: return False if n <= 3: return True if n % 2 == 0 or n % 3 == 0: return False i = 5 while i * i <= n: if n % i == 0 or n % (i + 2) == 0: return False i += 6 return True if __name__ == '__main__': numbers = range(100000) with ProcessPoolExecutor() as executor: results = list(executor.map(is_prime, numbers)) print(sum(results))
这将素数检查分布在多个进程中。
在多线程和多处理之间进行选择取决于任务。 I/O 密集型任务受益于多线程,而 CPU 密集型任务通常需要多处理才能实现真正的并行性。 负载平衡和任务依赖性是并行处理中的关键考虑因素。 处理共享资源时,适当的同步机制至关重要。 性能比较因任务和系统而异。 在数据处理和科学计算中,多重处理非常有效。 对于 Web 应用程序,asyncio
提供了并发连接的高效处理。 Python 多样化的并行处理工具使开发人员能够创建高性能应用程序。
101本书
101 Books 是一家由人工智能驱动的出版社,由作家 Aarav Joshi 共同创立,提供价格实惠、高质量的书籍 — 有些价格低至 4 美元.
在亚马逊上探索我们的Golang Clean Code书籍。 搜索 Aarav Joshi 查找更多图书和特别折扣!
我们的其他项目
探索我们的其他项目:投资者中心(英语、西班牙语、德语)、智能生活、时代与回响、令人费解的奥秘、印度教、精英Dev 和 JS 学校。
在 Medium 上关注我们
通过 Medium 与我们联系:Tech Koala Insights、Epochs & Echoes World、Investor Central Medium、Puzzling Mysteries Medium、 科学与时代媒介,以及现代印度教。
以上是用于多线程和多处理的python技术:提高您的应用程序性能的详细内容。更多信息请关注PHP中文网其他相关文章!
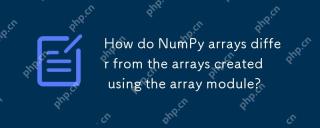
numpyArraysareAreBetterFornumericalialoperations andmulti-demensionaldata,而learthearrayModuleSutableforbasic,内存效率段
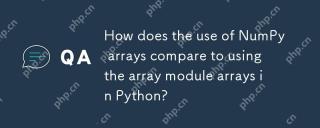
numpyArraySareAreBetterForHeAvyNumericalComputing,而lelethearRayModulesiutable-usemoblemory-connerage-inderabledsswithSimpleDatateTypes.1)NumpyArsofferVerverVerverVerverVersAtility andPerformanceForlargedForlargedAtatasetSetsAtsAndAtasEndCompleXoper.2)
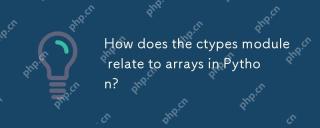
ctypesallowscreatingingangandmanipulatingc-stylarraysinpython.1)usectypestoInterfacewithClibrariesForperfermance.2)createc-stylec-stylec-stylarraysfornumericalcomputations.3)passarraystocfunctions foreforfunctionsforeffortions.however.however,However,HoweverofiousofmemoryManageManiverage,Pressiveo,Pressivero
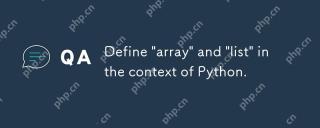
Inpython,一个“列表” isaversatile,mutableSequencethatCanholdMixedDatateTypes,而“阵列” isamorememory-效率,均质sepersequeSequeSequeReDencErequiringElements.1)
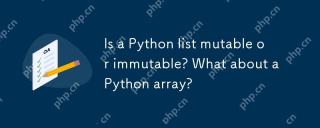
pythonlistsandArraysareBothable.1)列表Sareflexibleandsupportereceneousdatabutarelessmory-Memory-Empefficity.2)ArraysareMoremoremoremoreMemoremorememorememorememoremorememogeneSdatabutlesserversEversementime,defteringcorcttypecrecttypececeDepeceDyusagetoagetoavoavoiDerrors。
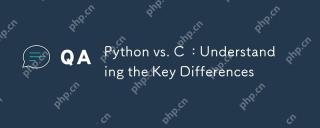
Python和C 各有优势,选择应基于项目需求。1)Python适合快速开发和数据处理,因其简洁语法和动态类型。2)C 适用于高性能和系统编程,因其静态类型和手动内存管理。
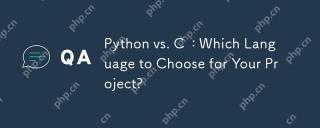
选择Python还是C 取决于项目需求:1)如果需要快速开发、数据处理和原型设计,选择Python;2)如果需要高性能、低延迟和接近硬件的控制,选择C 。
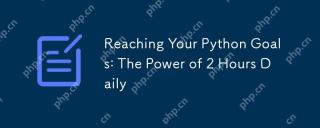
通过每天投入2小时的Python学习,可以有效提升编程技能。1.学习新知识:阅读文档或观看教程。2.实践:编写代码和完成练习。3.复习:巩固所学内容。4.项目实践:应用所学于实际项目中。这样的结构化学习计划能帮助你系统掌握Python并实现职业目标。


热AI工具

Undresser.AI Undress
人工智能驱动的应用程序,用于创建逼真的裸体照片

AI Clothes Remover
用于从照片中去除衣服的在线人工智能工具。

Undress AI Tool
免费脱衣服图片

Clothoff.io
AI脱衣机

Video Face Swap
使用我们完全免费的人工智能换脸工具轻松在任何视频中换脸!

热门文章

热工具

螳螂BT
Mantis是一个易于部署的基于Web的缺陷跟踪工具,用于帮助产品缺陷跟踪。它需要PHP、MySQL和一个Web服务器。请查看我们的演示和托管服务。

禅工作室 13.0.1
功能强大的PHP集成开发环境
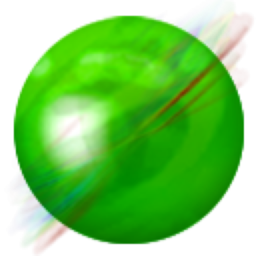
ZendStudio 13.5.1 Mac
功能强大的PHP集成开发环境

SublimeText3汉化版
中文版,非常好用

Atom编辑器mac版下载
最流行的的开源编辑器