Chainlit:可扩展的对话式人工智能框架
Chainlit 是一个开源异步 Python 框架,旨在构建强大且可扩展的对话式 AI 应用程序。 它提供了灵活的基础,允许开发人员无缝集成外部 API、自定义逻辑和本地模型。
本教程演示了 Chainlit 中的两种检索增强生成 (RAG) 实现:
- 利用 OpenAI 助手上传文档。
- 将 llama_index 与本地文档文件夹结合使用。
本地 Chainlit 设置
虚拟环境
创建虚拟环境:
mkdir chainlit && cd chainlit python3 -m venv venv source venv/bin/activate
安装依赖项
安装所需的包并保存依赖项:
pip install chainlit pip install llama_index # For implementation #2 pip install openai pip freeze > requirements.txt
测试 Chainlit
启动Chainlit:
chainlit hello
访问占位符https://www.php.cn/link/2674cea93e3214abce13e072a2dc2ca5
Upsun 部署
Git 初始化
初始化 Git 存储库:
git init .
创建.gitignore
文件:
<code>.env database/** data/** storage/** .chainlit venv __pycache__</code>
Upsun 项目创建
使用 CLI 创建 Upsun 项目(按照提示操作)。 Upsun 将自动配置远程存储库。
配置
Chainlit 的 Upsun 配置示例:
applications: chainlit: source: root: "/" type: "python:3.11" mounts: "/database": source: "storage" source_path: "database" ".files": source: "storage" source_path: "files" "__pycache__": source: "storage" source_path: "pycache" ".chainlit": source: "storage" source_path: ".chainlit" web: commands: start: "chainlit run app.py --port $PORT --host 0.0.0.0" upstream: socket_family: tcp locations: "/": passthru: true "/public": passthru: true build: flavor: none hooks: build: | set -eux pip install -r requirements.txt deploy: | set -eux # post_deploy: | routes: "https://{default}/": type: upstream upstream: "chainlit:http" "https://www.{default}": type: redirect to: "https://{default}/"
通过 Upsun CLI 设置 OPENAI_API_KEY
环境变量:
upsun variable:create env:OPENAI_API_KEY --value=sk-proj[...]
部署
提交并部署:
git add . git commit -m "First chainlit example" upsun push
查看部署状态。 成功部署将显示 Chainlit 在您的主环境中运行。
实现一:OpenAI助手及上传文件
此实现使用 OpenAI 助手来处理上传的文档。
辅助创作
在 OpenAI 平台上创建一个新的 OpenAI 助手。设置系统指令,选择模型(带有文本响应格式),并保持较低的温度(例如0.10)。 复制助手 ID (asst_[xxx]
) 并将其设置为环境变量:
upsun variable:create env:OPENAI_ASSISTANT_ID --value=asst_[...]
内容上传
将您的文档(首选 Markdown)上传到助手。 OpenAI 将创建一个矢量存储。
助理逻辑(app.py)
将 app.py
内容替换为提供的代码。 关键部分:@cl.on_chat_start
创建一个新的 OpenAI 线程,@cl.on_message
将用户消息发送到该线程并流式传输响应。
提交并部署更改。测试助手。
实现2:OpenAI llama_index
此实现使用 llama_index 进行本地知识管理,并使用 OpenAI 进行响应生成。
创建分支
创建一个新分支:
mkdir chainlit && cd chainlit python3 -m venv venv source venv/bin/activate
文件夹创建和安装
创建 data
和 storage
文件夹。将安装添加到 Upsun 配置中。
app.py 更新
使用提供的 llama_index 代码更新 app.py
。 此代码加载文档,创建 VectorStoreIndex,并使用它通过 OpenAI 回答查询。
部署新环境并上传data
文件夹。测试应用程序。
奖励:身份验证
使用 SQLite 数据库添加身份验证。
数据库设置
创建一个 database
文件夹并将挂载添加到 Upsun 配置中。为数据库路径创建环境变量:
pip install chainlit pip install llama_index # For implementation #2 pip install openai pip freeze > requirements.txt
身份验证逻辑 (app.py)
使用 app.py
将身份验证逻辑添加到 @cl.password_auth_callback
。 这会添加一个登录表单。
创建一个脚本来生成哈希密码。将用户添加到数据库(使用散列密码)。部署身份验证和测试登录。
结论
本教程演示了如何在 Upsun 上部署 Chainlit 应用程序,并使用两个 RAG 实现和身份验证。 灵活的架构允许各种适应和集成。
以上是在 Upsun 上使用 RAG 试验 Chainlit AI 界面的详细内容。更多信息请关注PHP中文网其他相关文章!
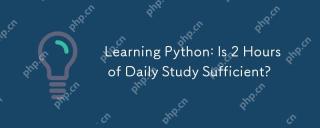
每天学习Python两个小时是否足够?这取决于你的目标和学习方法。1)制定清晰的学习计划,2)选择合适的学习资源和方法,3)动手实践和复习巩固,可以在这段时间内逐步掌握Python的基本知识和高级功能。
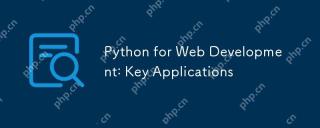
Python在Web开发中的关键应用包括使用Django和Flask框架、API开发、数据分析与可视化、机器学习与AI、以及性能优化。1.Django和Flask框架:Django适合快速开发复杂应用,Flask适用于小型或高度自定义项目。2.API开发:使用Flask或DjangoRESTFramework构建RESTfulAPI。3.数据分析与可视化:利用Python处理数据并通过Web界面展示。4.机器学习与AI:Python用于构建智能Web应用。5.性能优化:通过异步编程、缓存和代码优
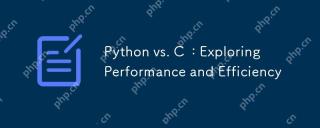
Python在开发效率上优于C ,但C 在执行性能上更高。1.Python的简洁语法和丰富库提高开发效率。2.C 的编译型特性和硬件控制提升执行性能。选择时需根据项目需求权衡开发速度与执行效率。
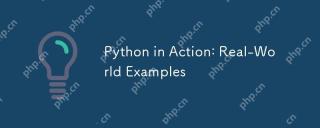
Python在现实世界中的应用包括数据分析、Web开发、人工智能和自动化。1)在数据分析中,Python使用Pandas和Matplotlib处理和可视化数据。2)Web开发中,Django和Flask框架简化了Web应用的创建。3)人工智能领域,TensorFlow和PyTorch用于构建和训练模型。4)自动化方面,Python脚本可用于复制文件等任务。
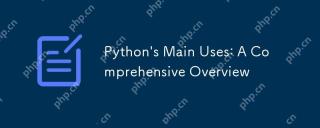
Python在数据科学、Web开发和自动化脚本领域广泛应用。1)在数据科学中,Python通过NumPy、Pandas等库简化数据处理和分析。2)在Web开发中,Django和Flask框架使开发者能快速构建应用。3)在自动化脚本中,Python的简洁性和标准库使其成为理想选择。
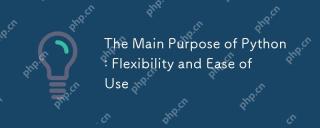
Python的灵活性体现在多范式支持和动态类型系统,易用性则源于语法简洁和丰富的标准库。1.灵活性:支持面向对象、函数式和过程式编程,动态类型系统提高开发效率。2.易用性:语法接近自然语言,标准库涵盖广泛功能,简化开发过程。
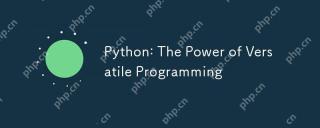
Python因其简洁与强大而备受青睐,适用于从初学者到高级开发者的各种需求。其多功能性体现在:1)易学易用,语法简单;2)丰富的库和框架,如NumPy、Pandas等;3)跨平台支持,可在多种操作系统上运行;4)适合脚本和自动化任务,提升工作效率。
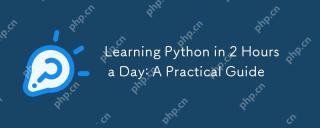
可以,在每天花费两个小时的时间内学会Python。1.制定合理的学习计划,2.选择合适的学习资源,3.通过实践巩固所学知识,这些步骤能帮助你在短时间内掌握Python。


热AI工具

Undresser.AI Undress
人工智能驱动的应用程序,用于创建逼真的裸体照片

AI Clothes Remover
用于从照片中去除衣服的在线人工智能工具。

Undress AI Tool
免费脱衣服图片

Clothoff.io
AI脱衣机

AI Hentai Generator
免费生成ai无尽的。

热门文章

热工具

DVWA
Damn Vulnerable Web App (DVWA) 是一个PHP/MySQL的Web应用程序,非常容易受到攻击。它的主要目标是成为安全专业人员在合法环境中测试自己的技能和工具的辅助工具,帮助Web开发人员更好地理解保护Web应用程序的过程,并帮助教师/学生在课堂环境中教授/学习Web应用程序安全。DVWA的目标是通过简单直接的界面练习一些最常见的Web漏洞,难度各不相同。请注意,该软件中

PhpStorm Mac 版本
最新(2018.2.1 )专业的PHP集成开发工具

SublimeText3 英文版
推荐:为Win版本,支持代码提示!

SecLists
SecLists是最终安全测试人员的伙伴。它是一个包含各种类型列表的集合,这些列表在安全评估过程中经常使用,都在一个地方。SecLists通过方便地提供安全测试人员可能需要的所有列表,帮助提高安全测试的效率和生产力。列表类型包括用户名、密码、URL、模糊测试有效载荷、敏感数据模式、Web shell等等。测试人员只需将此存储库拉到新的测试机上,他就可以访问到所需的每种类型的列表。
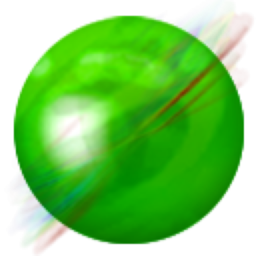
ZendStudio 13.5.1 Mac
功能强大的PHP集成开发环境