请我喝杯咖啡☕
*备忘录:
- 我的帖子解释了 add()。
- 我的帖子解释了 mul()。
- 我的帖子解释了 div()。
- 我的帖子解释了余数()。
- 我的帖子解释了 fmod()。
sub() 可以与零个或多个元素或标量的 0D 或多个 D 张量中的两个或零个或多个元素的 0D 或多个 D 张量与一个标量进行减法,得到为零的 0D 或多个 D 张量或更多元素,如下所示:
*备忘录:
- sub() 可以与 torch 或张量一起使用。
- 第一个参数(输入)与 torch(类型:int、float 或 complex 的张量或标量)或使用张量(类型:int、float 或 complex 的张量)(必需)。
- 带有 torch 的第二个参数或带有张量的第一个参数是其他(必需类型:张量或 int、float 或complex 标量)。
- 带有 torch 的第三个参数或带有张量的第二个参数是 alpha(可选-默认:1-类型:张量或整数、浮点或复数标量)。 *other 乘以 alpha(输入或张量 -(otherxalpha))。
- torch 存在 out 参数(可选-默认:无-类型:张量):
*备注:
- 必须使用 out=。
- 我的帖子解释了论点。
- minus() 是 sub() 的别名。
import torch tensor1 = torch.tensor([9, 7, 6]) tensor2 = torch.tensor([[4, -4, 3], [-2, 5, -5]]) torch.sub(input=tensor1, other=tensor2) tensor1.sub(other=tensor2) torch.sub(input=tensor1, other=tensor2, alpha=1) torch.sub(input=tensor1, other=tensor2, alpha=torch.tensor(1)) # tensor([[5, 11, 3], [11, 2, 11]]) torch.sub(input=tensor1, other=tensor2, alpha=0) torch.sub(input=tensor1, other=tensor2, alpha=torch.tensor(0)) # tensor([[9, 7, 6], [9, 7, 6]]) torch.sub(input=tensor1, other=tensor2, alpha=2) torch.sub(input=tensor1, other=tensor2, alpha=torch.tensor(2)) # tensor([[1, 15, 0], [13, -3, 16]]) torch.sub(input=tensor1, other=tensor2, alpha=-1) torch.sub(input=tensor1, other=tensor2, alpha=torch.tensor(-1)) # tensor([[13, 3, 9], [7, 12, 1]]) torch.sub(input=tensor1, other=tensor2, alpha=-2) torch.sub(input=tensor1, other=tensor2, alpha=torch.tensor(-2)) # tensor([[17, -1, 12], [5, 17, -4]]) torch.sub(input=9, other=tensor2) torch.sub(input=9, other=tensor2, alpha=1) torch.sub(input=9, other=tensor2, alpha=torch.tensor(1)) # tensor([[5, 13, 6], [11, 4, 14]]) torch.sub(input=tensor1, other=4) torch.sub(input=tensor1, other=4, alpha=1) torch.sub(input=tensor1, other=4, alpha=torch.tensor(1)) # tensor([5, 3, 2]) torch.sub(input=9, other=4) torch.sub(input=9, other=4, alpha=1) torch.sub(input=9, other=4, alpha=torch.tensor(1)) # tensor(5) tensor1 = torch.tensor([9., 7., 6.]) tensor2 = torch.tensor([[4., -4., 3.], [-2., 5., -5.]]) torch.sub(input=tensor1, other=tensor2) torch.sub(input=tensor1, other=tensor2, alpha=1.) torch.sub(input=tensor1, other=tensor2, alpha=torch.tensor(1.)) # tensor([[5., 11., 3.], [11., 2., 11.]]) torch.sub(input=9., other=tensor2) torch.sub(input=9., other=tensor2, alpha=1.) torch.sub(input=9., other=tensor2, alpha=torch.tensor(1.)) # tensor([[5., 13., 6.], [11., 4., 14.]]) torch.sub(input=tensor1, other=4) torch.sub(input=tensor1, other=4, alpha=1.) torch.sub(input=tensor1, other=4, alpha=torch.tensor(1.)) # tensor([5., 3., 2.]) torch.sub(input=9., other=4) torch.sub(input=9., other=4, alpha=1.) torch.sub(input=9., other=4, alpha=torch.tensor(1.)) # tensor(5.) tensor1 = torch.tensor([9.+0.j, 7.+0.j, 6.+0.j]) tensor2 = torch.tensor([[4.+0.j, -4.+0.j, 3.+0.j], [-2.+0.j, 5.+0.j, -5.+0.j]]) torch.sub(input=tensor1, other=tensor2) torch.sub(input=tensor1, other=tensor2, alpha=1.+0.j) torch.sub(input=tensor1, other=tensor2, alpha=torch.tensor(1.+0.j)) # tensor([[5.+0.j, 11.+0.j, 3.+0.j], # [11.+0.j, 2.+0.j, 11.+0.j]]) torch.sub(input=9.+0.j, other=tensor2) torch.sub(input=9.+0.j, other=tensor2, alpha=1.+0.j) torch.sub(input=9.+0.j, other=tensor2, alpha=torch.tensor(1.+0.j)) # tensor([[5.+0.j, 13.+0.j, 6.+0.j], # [11.+0.j, 4.+0.j, 14.+0.j]]) torch.sub(input=tensor1, other=4.+0.j) torch.sub(input=tensor1, other=4.+0.j, alpha=1.+0.j) torch.sub(input=tensor1, other=4.+0.j, alpha=torch.tensor(1.+0.j)) # tensor([5.+0.j, 3.+0.j, 2.+0.j]) torch.sub(input=9.+0.j, other=4.+0.j) torch.sub(input=9.+0.j, other=4.+0.j, alpha=1.+0.j) torch.sub(input=9.+0.j, other=4.+0.j, alpha=torch.tensor(1.+0.j)) # tensor(5.+0.j)
以上是PyTorch 中的子项目的详细内容。更多信息请关注PHP中文网其他相关文章!
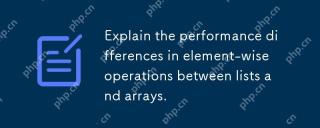
ArraySareBetterForlement-WiseOperationsDuetofasterAccessCessCessCessCessCessAndOptimizedImplementations.1)ArrayshaveContiguucuulmemoryfordirectAccesscess.2)列出sareflexible butslible dueTopotentEnallymideNamicizing.3)forlarargedAtaTasetsetsetsetsetsetsetsetsetsetsetlib
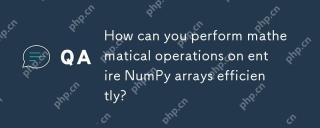
在NumPy中进行整个数组的数学运算可以通过向量化操作高效实现。 1)使用简单运算符如加法(arr 2)可对数组进行运算。 2)NumPy使用C语言底层库,提升了运算速度。 3)可以进行乘法、除法、指数等复杂运算。 4)需注意广播操作,确保数组形状兼容。 5)使用NumPy函数如np.sum()能显着提高性能。
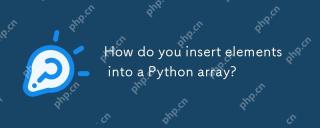
在Python中,向列表插入元素有两种主要方法:1)使用insert(index,value)方法,可以在指定索引处插入元素,但在大列表开头插入效率低;2)使用append(value)方法,在列表末尾添加元素,效率高。对于大列表,建议使用append()或考虑使用deque或NumPy数组来优化性能。
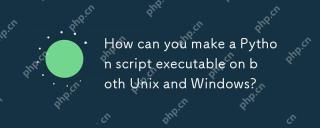
tomakeapythonscriptexecutableonbothunixandwindows:1)Addashebangline(#!/usr/usr/bin/envpython3)Andusechmod Xtomakeitexecutableonix.2)onWindows,确保pytythonisinsinstalledandassociatedwithedandassociatedwith.pyuunwith.pyun.pyfiles,oruseabatchfile(runuseabatchfile(rugitter)。
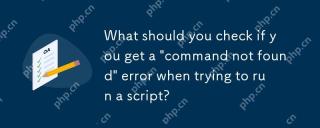
当遇到“commandnotfound”错误时,应检查以下几点:1.确认脚本存在且路径正确;2.检查文件权限,必要时使用chmod添加执行权限;3.确保脚本解释器已安装并在PATH中;4.验证脚本开头的shebang行是否正确。这样做可以有效解决脚本运行问题,确保编码过程顺利进行。
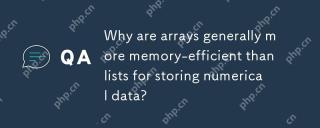
ArraySareAryallyMoremory-Moremory-forigationDataDatueTotheIrfixed-SizenatureAntatureAntatureAndirectMemoryAccess.1)arraysStorelelementsInAcontiguxufulock,ReducingOveringOverheadHeadefromenterSormetormetAdata.2)列表,通常
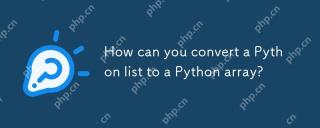
ToconvertaPythonlisttoanarray,usethearraymodule:1)Importthearraymodule,2)Createalist,3)Usearray(typecode,list)toconvertit,specifyingthetypecodelike'i'forintegers.Thisconversionoptimizesmemoryusageforhomogeneousdata,enhancingperformanceinnumericalcomp
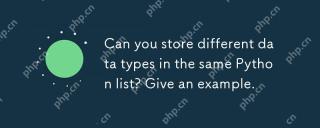
Python列表可以存储不同类型的数据。示例列表包含整数、字符串、浮点数、布尔值、嵌套列表和字典。列表的灵活性在数据处理和原型设计中很有价值,但需谨慎使用以确保代码的可读性和可维护性。


热AI工具

Undresser.AI Undress
人工智能驱动的应用程序,用于创建逼真的裸体照片

AI Clothes Remover
用于从照片中去除衣服的在线人工智能工具。

Undress AI Tool
免费脱衣服图片

Clothoff.io
AI脱衣机

Video Face Swap
使用我们完全免费的人工智能换脸工具轻松在任何视频中换脸!

热门文章

热工具

PhpStorm Mac 版本
最新(2018.2.1 )专业的PHP集成开发工具
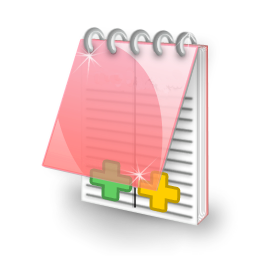
EditPlus 中文破解版
体积小,语法高亮,不支持代码提示功能
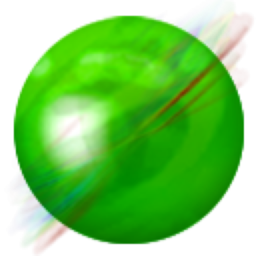
ZendStudio 13.5.1 Mac
功能强大的PHP集成开发环境
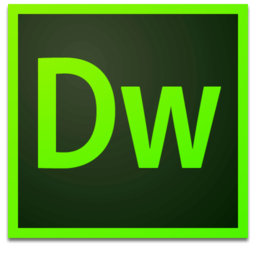
Dreamweaver Mac版
视觉化网页开发工具

MinGW - 适用于 Windows 的极简 GNU
这个项目正在迁移到osdn.net/projects/mingw的过程中,你可以继续在那里关注我们。MinGW:GNU编译器集合(GCC)的本地Windows移植版本,可自由分发的导入库和用于构建本地Windows应用程序的头文件;包括对MSVC运行时的扩展,以支持C99功能。MinGW的所有软件都可以在64位Windows平台上运行。