Python 数据验证对于构建健壮的应用程序至关重要。我发现实施彻底的验证技术可以显着减少错误并提高整体代码质量。让我们探讨一下我在项目中经常使用的五种强大方法。
Pydantic 已成为我进行数据建模和验证的首选库。它的简单性和强大功能使其成为许多场景的绝佳选择。以下是我通常的使用方式:
from pydantic import BaseModel, EmailStr, validator from typing import List class User(BaseModel): username: str email: EmailStr age: int tags: List[str] = [] @validator('age') def check_age(cls, v): if v <p>在此示例中,Pydantic 自动验证电子邮件格式并确保所有字段都具有正确的类型。年龄的自定义验证器添加了额外的验证层。</p> <p>Cerberus 是我经常使用的另一个优秀库,特别是当我需要对验证过程进行更多控制时。它基于模式的方法非常灵活:<br> </p> <pre class="brush:php;toolbar:false">from cerberus import Validator schema = { 'name': {'type': 'string', 'required': True, 'minlength': 2}, 'age': {'type': 'integer', 'min': 18, 'max': 99}, 'email': {'type': 'string', 'regex': '^[a-zA-Z0-9_.+-]+@[a-zA-Z0-9-]+\.[a-zA-Z0-9-.]+$'}, 'interests': {'type': 'list', 'schema': {'type': 'string'}} } v = Validator(schema) document = {'name': 'John Doe', 'age': 30, 'email': 'john@example.com', 'interests': ['python', 'data science']} if v.validate(document): print("Document is valid") else: print(v.errors)
Cerberus 允许我定义复杂的模式,甚至自定义验证规则,使其成为具有特定数据要求的项目的理想选择。
当我使用 Web 框架或 ORM 库时,Marshmallow 特别有用。它的序列化和反序列化能力是一流的:
from marshmallow import Schema, fields, validate, ValidationError class UserSchema(Schema): id = fields.Int(dump_only=True) username = fields.Str(required=True, validate=validate.Length(min=3)) email = fields.Email(required=True) created_at = fields.DateTime(dump_only=True) user_data = {'username': 'john', 'email': 'john@example.com'} schema = UserSchema() try: result = schema.load(user_data) print(result) except ValidationError as err: print(err.messages)
当我需要验证来自或去往数据库或 API 的数据时,这种方法特别有效。
Python 的内置类型提示与 mypy 等静态类型检查器相结合,彻底改变了我编写和验证代码的方式:
from typing import List, Dict, Optional def process_user_data(name: str, age: int, emails: List[str], metadata: Optional[Dict[str, str]] = None) -> bool: if not 0 <p>当我在此代码上运行 mypy 时,它会在运行前捕获与类型相关的错误,从而显着提高代码质量并减少错误。</p> <p>对于 JSON 数据验证,尤其是 API 开发中,我经常求助于 jsonschema:<br> </p> <pre class="brush:php;toolbar:false">import jsonschema schema = { "type": "object", "properties": { "name": {"type": "string"}, "age": {"type": "number", "minimum": 0}, "pets": { "type": "array", "items": {"type": "string"}, "minItems": 1 } }, "required": ["name", "age"] } data = { "name": "John Doe", "age": 30, "pets": ["dog", "cat"] } try: jsonschema.validate(instance=data, schema=schema) print("Data is valid") except jsonschema.exceptions.ValidationError as err: print(f"Invalid data: {err}")
当我处理复杂的 JSON 结构或需要验证配置文件时,这种方法特别有用。
在实际应用中,我经常结合这些技术。例如,我可能会使用 Pydantic 在 FastAPI 应用程序中进行输入验证,使用 Marshmallow 进行 ORM 集成,并在整个代码库中使用类型提示进行静态分析。
以下是我如何使用多种验证技术构建 Flask 应用程序的示例:
from flask import Flask, request, jsonify from marshmallow import Schema, fields, validate, ValidationError from pydantic import BaseModel, EmailStr from typing import List, Optional import jsonschema app = Flask(__name__) # Pydantic model for request validation class UserCreate(BaseModel): username: str email: EmailStr age: int tags: Optional[List[str]] = [] # Marshmallow schema for database serialization class UserSchema(Schema): id = fields.Int(dump_only=True) username = fields.Str(required=True, validate=validate.Length(min=3)) email = fields.Email(required=True) age = fields.Int(required=True, validate=validate.Range(min=18)) tags = fields.List(fields.Str()) # JSON schema for API response validation response_schema = { "type": "object", "properties": { "id": {"type": "number"}, "username": {"type": "string"}, "email": {"type": "string", "format": "email"}, "age": {"type": "number", "minimum": 18}, "tags": { "type": "array", "items": {"type": "string"} } }, "required": ["id", "username", "email", "age"] } @app.route('/users', methods=['POST']) def create_user(): try: # Validate request data with Pydantic user_data = UserCreate(**request.json) # Simulate database operation user_dict = user_data.dict() user_dict['id'] = 1 # Assume this is set by the database # Serialize with Marshmallow user_schema = UserSchema() result = user_schema.dump(user_dict) # Validate response with jsonschema jsonschema.validate(instance=result, schema=response_schema) return jsonify(result), 201 except ValidationError as err: return jsonify(err.messages), 400 except jsonschema.exceptions.ValidationError as err: return jsonify({"error": str(err)}), 500 if __name__ == '__main__': app.run(debug=True)
在此示例中,我使用 Pydantic 验证传入的请求数据,使用 Marshmallow 序列化数据库操作的数据,并使用 jsonschema 确保 API 响应符合定义的架构。这种多层方法在数据处理的不同阶段提供了强大的验证。
在实现数据验证时,我总是考虑项目的具体需求。对于简单的脚本或小型应用程序,使用内置的 Python 功能(例如类型提示和断言)可能就足够了。对于较大的项目或具有复杂数据结构的项目,结合 Pydantic、Marshmallow 或 Cerberus 等库可以提供更全面的验证。
考虑性能影响也很重要。虽然彻底的验证对于数据完整性至关重要,但过于复杂的验证可能会减慢应用程序的速度。我经常分析我的代码,以确保验证不会成为瓶颈,尤其是在高流量应用程序中。
错误处理是数据验证的另一个关键方面。我确保提供清晰、可操作的错误消息,帮助用户或其他开发人员理解和纠正无效数据。这可能涉及自定义错误类或详细的错误报告机制。
from pydantic import BaseModel, EmailStr, validator from typing import List class User(BaseModel): username: str email: EmailStr age: int tags: List[str] = [] @validator('age') def check_age(cls, v): if v <p>这种方法允许更精细的错误处理和报告,这在 API 开发或面向用户的应用程序中特别有用。</p> <p>安全性是数据验证中的另一个重要考虑因素。正确的验证可以防止许多常见的安全漏洞,例如 SQL 注入或跨站点脚本 (XSS) 攻击。处理用户输入时,我总是在将数据用于数据库查询或以 HTML 形式呈现之前对其进行清理和验证。<br> </p> <pre class="brush:php;toolbar:false">from cerberus import Validator schema = { 'name': {'type': 'string', 'required': True, 'minlength': 2}, 'age': {'type': 'integer', 'min': 18, 'max': 99}, 'email': {'type': 'string', 'regex': '^[a-zA-Z0-9_.+-]+@[a-zA-Z0-9-]+\.[a-zA-Z0-9-.]+$'}, 'interests': {'type': 'list', 'schema': {'type': 'string'}} } v = Validator(schema) document = {'name': 'John Doe', 'age': 30, 'email': 'john@example.com', 'interests': ['python', 'data science']} if v.validate(document): print("Document is valid") else: print(v.errors)
这个简单的示例演示了如何清理用户输入以防止 XSS 攻击。在现实应用程序中,我经常使用更全面的库或框架来提供针对常见安全威胁的内置保护。
测试是实现稳健数据验证的一个组成部分。我编写了大量的单元测试,以确保我的验证逻辑对于有效和无效输入都能正确工作。这包括测试边缘情况和边界条件。
from marshmallow import Schema, fields, validate, ValidationError class UserSchema(Schema): id = fields.Int(dump_only=True) username = fields.Str(required=True, validate=validate.Length(min=3)) email = fields.Email(required=True) created_at = fields.DateTime(dump_only=True) user_data = {'username': 'john', 'email': 'john@example.com'} schema = UserSchema() try: result = schema.load(user_data) print(result) except ValidationError as err: print(err.messages)
这些测试确保用户模型正确验证有效和无效输入,包括类型检查和必填字段验证。
总之,有效的数据验证是构建健壮的 Python 应用程序的关键组成部分。通过利用内置 Python 功能和第三方库的组合,我们可以创建全面的验证系统,以确保数据完整性、提高应用程序可靠性并增强安全性。关键是为每个特定用例选择正确的工具和技术,平衡彻底性与性能和可维护性。通过正确的实施和测试,数据验证成为创建高质量、可靠的 Python 应用程序的宝贵资产。
我们的创作
一定要看看我们的创作:
投资者中心 | 投资者中央西班牙语 | 投资者中德意志 | 智能生活 | 时代与回响 | 令人费解的谜团 | 印度教 | 精英开发 | JS学校
我们在媒体上
科技考拉洞察 | 时代与回响世界 | 投资者中央媒体 | 令人费解的谜团 | 科学与时代媒介 | 现代印度教
以上是用于强大应用程序的强大 Python 数据验证技术的详细内容。更多信息请关注PHP中文网其他相关文章!
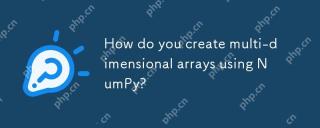
使用NumPy创建多维数组可以通过以下步骤实现:1)使用numpy.array()函数创建数组,例如np.array([[1,2,3],[4,5,6]])创建2D数组;2)使用np.zeros(),np.ones(),np.random.random()等函数创建特定值填充的数组;3)理解数组的shape和size属性,确保子数组长度一致,避免错误;4)使用np.reshape()函数改变数组形状;5)注意内存使用,确保代码清晰高效。
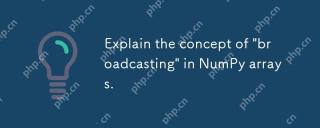
播放innumpyisamethodtoperformoperationsonArraySofDifferentsHapesbyAutapityallate AligningThem.itSimplifififiesCode,增强可读性,和Boostsperformance.Shere'shore'showitworks:1)较小的ArraySaraySaraysAraySaraySaraySaraySarePaddedDedWiteWithOnestOmatchDimentions.2)
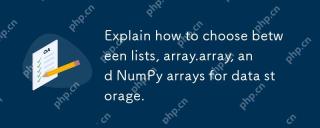
forpythondataTastorage,choselistsforflexibilityWithMixedDatatypes,array.ArrayFormeMory-effficityHomogeneousnumericalData,andnumpyArraysForAdvancedNumericalComputing.listsareversareversareversareversArversatilebutlessEbutlesseftlesseftlesseftlessforefforefforefforefforefforefforefforefforefforlargenumerdataSets; arrayoffray.array.array.array.array.array.ersersamiddreddregro
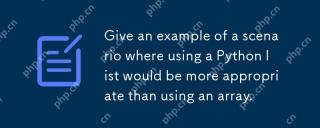
Pythonlistsarebetterthanarraysformanagingdiversedatatypes.1)Listscanholdelementsofdifferenttypes,2)theyaredynamic,allowingeasyadditionsandremovals,3)theyofferintuitiveoperationslikeslicing,but4)theyarelessmemory-efficientandslowerforlargedatasets.
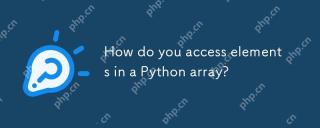
toAccesselementsInapyThonArray,useIndIndexing:my_array [2] accessEsthethEthErlement,returning.3.pythonosezero opitedEndexing.1)usepositiveandnegativeIndexing:my_list [0] fortefirstElment,fortefirstelement,my_list,my_list [-1] fornelast.2] forselast.2)
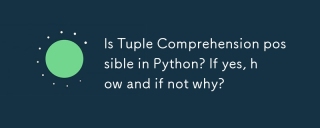
文章讨论了由于语法歧义而导致的Python中元组理解的不可能。建议使用tuple()与发电机表达式使用tuple()有效地创建元组。(159个字符)
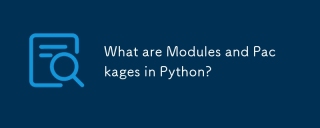
本文解释了Python中的模块和包装,它们的差异和用法。模块是单个文件,而软件包是带有__init__.py文件的目录,在层次上组织相关模块。
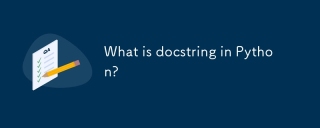
文章讨论了Python中的Docstrings,其用法和收益。主要问题:Docstrings对于代码文档和可访问性的重要性。


热AI工具

Undresser.AI Undress
人工智能驱动的应用程序,用于创建逼真的裸体照片

AI Clothes Remover
用于从照片中去除衣服的在线人工智能工具。

Undress AI Tool
免费脱衣服图片

Clothoff.io
AI脱衣机

Video Face Swap
使用我们完全免费的人工智能换脸工具轻松在任何视频中换脸!

热门文章

热工具

禅工作室 13.0.1
功能强大的PHP集成开发环境
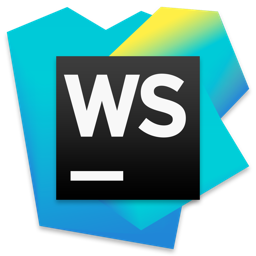
WebStorm Mac版
好用的JavaScript开发工具

适用于 Eclipse 的 SAP NetWeaver 服务器适配器
将Eclipse与SAP NetWeaver应用服务器集成。

安全考试浏览器
Safe Exam Browser是一个安全的浏览器环境,用于安全地进行在线考试。该软件将任何计算机变成一个安全的工作站。它控制对任何实用工具的访问,并防止学生使用未经授权的资源。

mPDF
mPDF是一个PHP库,可以从UTF-8编码的HTML生成PDF文件。原作者Ian Back编写mPDF以从他的网站上“即时”输出PDF文件,并处理不同的语言。与原始脚本如HTML2FPDF相比,它的速度较慢,并且在使用Unicode字体时生成的文件较大,但支持CSS样式等,并进行了大量增强。支持几乎所有语言,包括RTL(阿拉伯语和希伯来语)和CJK(中日韩)。支持嵌套的块级元素(如P、DIV),