请我喝杯咖啡☕
*我的帖子解释了加州理工学院 101。
Caltech101()可以使用Caltech 101数据集,如下所示:
*备忘录:
- 第一个参数是 root(必需类型:str 或 pathlib.Path)。 *绝对或相对路径都是可能的。
- 第二个参数是 target_type(可选-默认:“category”-类型:str 或元组或 str 列表)。 *可以为其设置“类别”和/或“注释”。
- 第三个参数是transform(Optional-Default:None-Type:callable)。
- 第四个参数是 target_transform(Optional-Default:None-Type:callable)。
- 第五个参数是 download(可选-默认:False-类型:bool):
*备注:
- 如果为 True,则从互联网下载数据集并解压(解压)到根目录。
- 如果为 True 并且数据集已下载,则将其提取。
- 如果为 True 并且数据集已下载并提取,则不会发生任何事情。
- 如果数据集已经下载并提取,则应该为 False,因为它速度更快。
- 您可以从此处手动下载并提取数据集(101_ObjectCategories.tar.gz 和 Annotations.tar)到 data/caltech101/。
- 关于图像索引的类别,Faces(0) 为 0~434,Faces_easy(1) 为 435~869,豹子(2 )为870~1069, 摩托车(3)是1070~1867,手风琴(4)是1868~1922,飞机(5)是1923~2722,锚(6) 是2723~2764,蚂蚁(7)为2765~2806,桶(8)为2807~2853,低音(9)为2854~2907等。
from torchvision.datasets import Caltech101 category_data = Caltech101( root="data" ) category_data = Caltech101( root="data", target_type="category", transform=None, target_transform=None, download=False ) annotation_data = Caltech101( root="data", target_type="annotation" ) all_data = Caltech101( root="data", target_type=["category", "annotation"] ) len(category_data), len(annotation_data), len(all_data) # (8677, 8677, 8677) category_data # Dataset Caltech101 # Number of datapoints: 8677 # Root location: data\caltech101 # Target type: ['category'] category_data.root # 'data/caltech101' category_data.target_type # ['category'] print(category_data.transform) # None print(category_data.target_transform) # None category_data.download # <bound method caltech101.download of dataset caltech101 number datapoints: root location: data target type:> len(category_data.categories) # 101 category_data.categories # ['Faces', 'Faces_easy', 'Leopards', 'Motorbikes', 'accordion', # 'airplanes', 'anchor', 'ant', 'barrel', 'bass', 'beaver', # 'binocular', 'bonsai', 'brain', 'brontosaurus', 'buddha', # 'butterfly', 'camera', 'cannon', 'car_side', 'ceiling_fan', # 'cellphone', 'chair', 'chandelier', 'cougar_body', 'cougar_face', ...] len(category_data.annotation_categories) # 101 category_data.annotation_categories # ['Faces_2', 'Faces_3', 'Leopards', 'Motorbikes_16', 'accordion', # 'Airplanes_Side_2', 'anchor', 'ant', 'barrel', 'bass', # 'beaver', 'binocular', 'bonsai', 'brain', 'brontosaurus', # 'buddha', 'butterfly', 'camera', 'cannon', 'car_side', # 'ceiling_fan', 'cellphone', 'chair', 'chandelier', 'cougar_body', ...] category_data[0] # (<pil.jpegimageplugin.jpegimagefile image mode="RGB" size="510x337">, 0) category_data[1] # (<pil.jpegimageplugin.jpegimagefile image mode="RGB" size="519x343">, 0) category_data[2] # (<pil.jpegimageplugin.jpegimagefile image mode="RGB" size="492x325">, 0) category_data[435] # (<pil.jpegimageplugin.jpegimagefile image mode="RGB" size="290x334">, 1) category_data[870] # (<pil.jpegimageplugin.jpegimagefile image mode="RGB" size="192x128">, 2) annotation_data[0] # (<pil.jpegimageplugin.jpegimagefile image mode="RGB" size="510x337">, # array([[10.00958466, 8.18210863, 8.18210863, 10.92332268, ...], # [132.30670927, 120.42811502, 103.52396166, 90.73162939, ...]])) annotation_data[1] # (<pil.jpegimageplugin.jpegimagefile image mode="RGB" size="519x343">, # array([[15.19298246, 13.71929825, 15.19298246, 19.61403509, ...], # [121.5877193, 103.90350877, 80.81578947, 64.11403509, ...]])) annotation_data[2] # (<pil.jpegimageplugin.jpegimagefile image mode="RGB" size="492x325">, # array([[10.40789474, 7.17807018, 5.79385965, 9.02368421, ...], # [131.30789474, 120.69561404, 102.23947368, 86.09035088, ...]])) annotation_data[435] # (<pil.jpegimageplugin.jpegimagefile image mode="RGB" size="290x334">, # array([[64.52631579, 95.31578947, 123.26315789, 149.31578947, ...], # [15.42105263, 8.31578947, 10.21052632, 28.21052632, ...]])) annotation_data[870] # (<pil.jpegimageplugin.jpegimagefile image mode="RGB" size="192x128">, # array([[2.96536524, 7.55604534, 19.45780856, 33.73992443, ...], # [23.63413098, 32.13539043, 33.83564232, 8.84193955, ...]])) all_data[0] # (<pil.jpegimageplugin.jpegimagefile image mode="RGB" size="510x337">, # (0, array([[10.00958466, 8.18210863, 8.18210863, 10.92332268, ...], # [132.30670927, 120.42811502, 103.52396166, 90.73162939, ...]])) all_data[1] # (<pil.jpegimageplugin.jpegimagefile image mode="RGB" size="519x343">, # (0, array([[15.19298246, 13.71929825, 15.19298246, 19.61403509, ...], # [121.5877193, 103.90350877, 80.81578947, 64.11403509, ...]])) all_data[2] # (<pil.jpegimageplugin.jpegimagefile image mode="RGB" size="492x325">, # (0, array([[10.40789474, 7.17807018, 5.79385965, 9.02368421, ...], # [131.30789474, 120.69561404, 102.23947368, 86.09035088, ...]])) all_data[3] # (<pil.jpegimageplugin.jpegimagefile image mode="RGB" size="538x355">, # (0, array([[19.54035088, 18.57894737, 26.27017544, 38.2877193, ...], # [131.49122807, 100.24561404, 74.2877193, 49.29122807, ...]])) all_data[4] # (<pil.jpegimageplugin.jpegimagefile image mode="RGB" size="528x349">, # (0, array([[11.87982456, 11.87982456, 13.86578947, 15.35526316, ...], # [128.34649123, 105.50789474, 91.60614035, 76.71140351, ...]])) import matplotlib.pyplot as plt def show_images(data, main_title=None): plt.figure(figsize=(10, 5)) plt.suptitle(t=main_title, y=1.0, fontsize=14) ims = (0, 1, 2, 435, 870, 1070, 1868, 1923, 2723, 2765, 2807, 2854) for i, j in enumerate(ims, start=1): plt.subplot(2, 5, i) if len(data.target_type) == 1: if data.target_type[0] == "category": im, lab = data[j] plt.title(label=lab) elif data.target_type[0] == "annotation": im, (px, py) = data[j] plt.scatter(x=px, y=py) plt.imshow(X=im) elif len(data.target_type) == 2: if data.target_type[0] == "category": im, (lab, (px, py)) = data[j] elif data.target_type[0] == "annotation": im, ((px, py), lab) = data[j] plt.title(label=lab) plt.imshow(X=im) plt.scatter(x=px, y=py) if i == 10: break plt.tight_layout() plt.show() show_images(data=category_data, main_title="category_data") show_images(data=annotation_data, main_title="annotation_data") show_images(data=all_data, main_title="all_data") </pil.jpegimageplugin.jpegimagefile></pil.jpegimageplugin.jpegimagefile></pil.jpegimageplugin.jpegimagefile></pil.jpegimageplugin.jpegimagefile></pil.jpegimageplugin.jpegimagefile></pil.jpegimageplugin.jpegimagefile></pil.jpegimageplugin.jpegimagefile></pil.jpegimageplugin.jpegimagefile></pil.jpegimageplugin.jpegimagefile></pil.jpegimageplugin.jpegimagefile></pil.jpegimageplugin.jpegimagefile></pil.jpegimageplugin.jpegimagefile></pil.jpegimageplugin.jpegimagefile></pil.jpegimageplugin.jpegimagefile></pil.jpegimageplugin.jpegimagefile></bound>
以上是PyTorch 中的加州理工学院的详细内容。更多信息请关注PHP中文网其他相关文章!
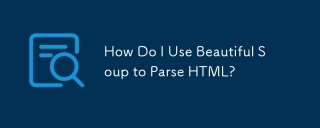
本文解释了如何使用美丽的汤库来解析html。 它详细介绍了常见方法,例如find(),find_all(),select()和get_text(),以用于数据提取,处理不同的HTML结构和错误以及替代方案(SEL)
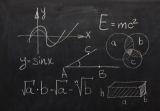
Python的statistics模块提供强大的数据统计分析功能,帮助我们快速理解数据整体特征,例如生物统计学和商业分析等领域。无需逐个查看数据点,只需查看均值或方差等统计量,即可发现原始数据中可能被忽略的趋势和特征,并更轻松、有效地比较大型数据集。 本教程将介绍如何计算平均值和衡量数据集的离散程度。除非另有说明,本模块中的所有函数都支持使用mean()函数计算平均值,而非简单的求和平均。 也可使用浮点数。 import random import statistics from fracti
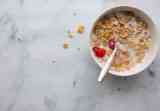
Python 对象的序列化和反序列化是任何非平凡程序的关键方面。如果您将某些内容保存到 Python 文件中,如果您读取配置文件,或者如果您响应 HTTP 请求,您都会进行对象序列化和反序列化。 从某种意义上说,序列化和反序列化是世界上最无聊的事情。谁会在乎所有这些格式和协议?您想持久化或流式传输一些 Python 对象,并在以后完整地取回它们。 这是一种在概念层面上看待世界的好方法。但是,在实际层面上,您选择的序列化方案、格式或协议可能会决定程序运行的速度、安全性、维护状态的自由度以及与其他系
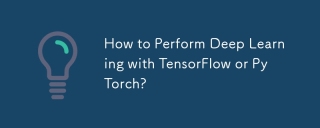
本文比较了Tensorflow和Pytorch的深度学习。 它详细介绍了所涉及的步骤:数据准备,模型构建,培训,评估和部署。 框架之间的关键差异,特别是关于计算刻度的
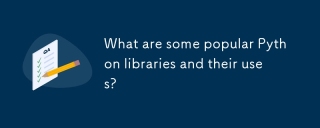
本文讨论了诸如Numpy,Pandas,Matplotlib,Scikit-Learn,Tensorflow,Tensorflow,Django,Blask和请求等流行的Python库,并详细介绍了它们在科学计算,数据分析,可视化,机器学习,网络开发和H中的用途
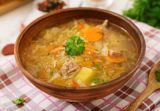
该教程建立在先前对美丽汤的介绍基础上,重点是简单的树导航之外的DOM操纵。 我们将探索有效的搜索方法和技术,以修改HTML结构。 一种常见的DOM搜索方法是EX
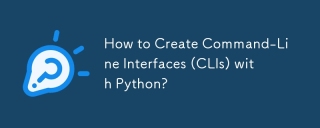
本文指导Python开发人员构建命令行界面(CLIS)。 它使用Typer,Click和ArgParse等库详细介绍,强调输入/输出处理,并促进用户友好的设计模式,以提高CLI可用性。
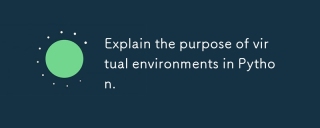
文章讨论了虚拟环境在Python中的作用,重点是管理项目依赖性并避免冲突。它详细介绍了他们在改善项目管理和减少依赖问题方面的创建,激活和利益。


热AI工具

Undresser.AI Undress
人工智能驱动的应用程序,用于创建逼真的裸体照片

AI Clothes Remover
用于从照片中去除衣服的在线人工智能工具。

Undress AI Tool
免费脱衣服图片

Clothoff.io
AI脱衣机

AI Hentai Generator
免费生成ai无尽的。

热门文章

热工具
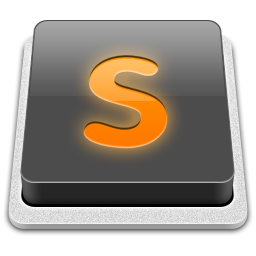
SublimeText3 Mac版
神级代码编辑软件(SublimeText3)

螳螂BT
Mantis是一个易于部署的基于Web的缺陷跟踪工具,用于帮助产品缺陷跟踪。它需要PHP、MySQL和一个Web服务器。请查看我们的演示和托管服务。

MinGW - 适用于 Windows 的极简 GNU
这个项目正在迁移到osdn.net/projects/mingw的过程中,你可以继续在那里关注我们。MinGW:GNU编译器集合(GCC)的本地Windows移植版本,可自由分发的导入库和用于构建本地Windows应用程序的头文件;包括对MSVC运行时的扩展,以支持C99功能。MinGW的所有软件都可以在64位Windows平台上运行。
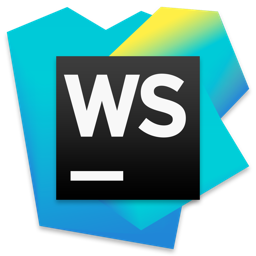
WebStorm Mac版
好用的JavaScript开发工具

安全考试浏览器
Safe Exam Browser是一个安全的浏览器环境,用于安全地进行在线考试。该软件将任何计算机变成一个安全的工作站。它控制对任何实用工具的访问,并防止学生使用未经授权的资源。