请我喝杯咖啡☕
*我的帖子解释了加州理工学院 101。
Caltech101()可以使用Caltech 101数据集,如下所示:
*备忘录:
- 第一个参数是 root(必需类型:str 或 pathlib.Path)。 *绝对或相对路径都是可能的。
- 第二个参数是 target_type(可选-默认:“category”-类型:str 或元组或 str 列表):
*备注:
- 可以为其设置“类别”和/或“注释”。
- 返回带有 101 个类别(类)标签和/或带有注释的 8.677 张图像。
- 第三个参数是transform(Optional-Default:None-Type:callable)。
- 第四个参数是 target_transform(Optional-Default:None-Type:callable)。
- 第五个参数是 download(Optional-Default:False-Type:bool):
*备注:
- 如果为 True,则从互联网下载数据集并解压(解压)到根目录。
- 如果为 True 并且数据集已下载,则将其提取。
- 如果为 True 并且数据集已下载并提取,则不会发生任何事情。
- 如果数据集已经下载并提取,则应该为 False,因为它速度更快。
- 下载数据集需要 gdown。
- 您可以从此处手动下载并提取数据集(101_ObjectCategories.tar.gz 和 Annotations.tar)到 data/caltech101/。
- 关于图像索引的类别(标签),Faces(0) 为 0~434,Faces_easy(1) 为 435~869,豹子(2)为870~1069, 摩托车(3)是1070~1867,手风琴(4)是1868~1922,飞机(5)是1923~2722,锚(6) 是2723~2764,蚂蚁(7)为2765~2806,桶(8)为2807~2853,低音(9)为2854~2907等。
from torchvision.datasets import Caltech101 category_data = Caltech101( root="data" ) category_data = Caltech101( root="data", target_type="category", transform=None, target_transform=None, download=False ) annotation_data = Caltech101( root="data", target_type="annotation" ) all_data = Caltech101( root="data", target_type=["category", "annotation"] ) len(category_data), len(annotation_data), len(all_data) # (8677, 8677, 8677) category_data # Dataset Caltech101 # Number of datapoints: 8677 # Root location: data\caltech101 # Target type: ['category'] category_data.root # 'data/caltech101' category_data.target_type # ['category'] print(category_data.transform) # None print(category_data.target_transform) # None category_data.download # <bound method caltech101.download of dataset caltech101 number datapoints: root location: data target type:> len(category_data.categories) # 101 category_data.categories # ['Faces', 'Faces_easy', 'Leopards', 'Motorbikes', 'accordion', # 'airplanes', 'anchor', 'ant', 'barrel', 'bass', 'beaver', # 'binocular', 'bonsai', 'brain', 'brontosaurus', 'buddha', # 'butterfly', 'camera', 'cannon', 'car_side', 'ceiling_fan', # 'cellphone', 'chair', 'chandelier', 'cougar_body', 'cougar_face', ...] len(category_data.annotation_categories) # 101 category_data.annotation_categories # ['Faces_2', 'Faces_3', 'Leopards', 'Motorbikes_16', 'accordion', # 'Airplanes_Side_2', 'anchor', 'ant', 'barrel', 'bass', # 'beaver', 'binocular', 'bonsai', 'brain', 'brontosaurus', # 'buddha', 'butterfly', 'camera', 'cannon', 'car_side', # 'ceiling_fan', 'cellphone', 'chair', 'chandelier', 'cougar_body', ...] category_data[0] # (<pil.jpegimageplugin.jpegimagefile image mode="RGB" size="510x337">, 0) category_data[1] # (<pil.jpegimageplugin.jpegimagefile image mode="RGB" size="519x343">, 0) category_data[2] # (<pil.jpegimageplugin.jpegimagefile image mode="RGB" size="492x325">, 0) category_data[435] # (<pil.jpegimageplugin.jpegimagefile image mode="RGB" size="290x334">, 1) category_data[870] # (<pil.jpegimageplugin.jpegimagefile image mode="RGB" size="192x128">, 2) annotation_data[0] # (<pil.jpegimageplugin.jpegimagefile image mode="RGB" size="510x337">, # array([[10.00958466, 8.18210863, 8.18210863, 10.92332268, ...], # [132.30670927, 120.42811502, 103.52396166, 90.73162939, ...]])) annotation_data[1] # (<pil.jpegimageplugin.jpegimagefile image mode="RGB" size="519x343">, # array([[15.19298246, 13.71929825, 15.19298246, 19.61403509, ...], # [121.5877193, 103.90350877, 80.81578947, 64.11403509, ...]])) annotation_data[2] # (<pil.jpegimageplugin.jpegimagefile image mode="RGB" size="492x325">, # array([[10.40789474, 7.17807018, 5.79385965, 9.02368421, ...], # [131.30789474, 120.69561404, 102.23947368, 86.09035088, ...]])) annotation_data[435] # (<pil.jpegimageplugin.jpegimagefile image mode="RGB" size="290x334">, # array([[64.52631579, 95.31578947, 123.26315789, 149.31578947, ...], # [15.42105263, 8.31578947, 10.21052632, 28.21052632, ...]])) annotation_data[870] # (<pil.jpegimageplugin.jpegimagefile image mode="RGB" size="192x128">, # array([[2.96536524, 7.55604534, 19.45780856, 33.73992443, ...], # [23.63413098, 32.13539043, 33.83564232, 8.84193955, ...]])) all_data[0] # (<pil.jpegimageplugin.jpegimagefile image mode="RGB" size="510x337">, # (0, array([[10.00958466, 8.18210863, 8.18210863, 10.92332268, ...], # [132.30670927, 120.42811502, 103.52396166, 90.73162939, ...]])) all_data[1] # (<pil.jpegimageplugin.jpegimagefile image mode="RGB" size="519x343">, # (0, array([[15.19298246, 13.71929825, 15.19298246, 19.61403509, ...], # [121.5877193, 103.90350877, 80.81578947, 64.11403509, ...]])) all_data[2] # (<pil.jpegimageplugin.jpegimagefile image mode="RGB" size="492x325">, # (0, array([[10.40789474, 7.17807018, 5.79385965, 9.02368421, ...], # [131.30789474, 120.69561404, 102.23947368, 86.09035088, ...]])) all_data[3] # (<pil.jpegimageplugin.jpegimagefile image mode="RGB" size="538x355">, # (0, array([[19.54035088, 18.57894737, 26.27017544, 38.2877193, ...], # [131.49122807, 100.24561404, 74.2877193, 49.29122807, ...]])) all_data[4] # (<pil.jpegimageplugin.jpegimagefile image mode="RGB" size="528x349">, # (0, array([[11.87982456, 11.87982456, 13.86578947, 15.35526316, ...], # [128.34649123, 105.50789474, 91.60614035, 76.71140351, ...]])) import matplotlib.pyplot as plt def show_images(data, main_title=None): plt.figure(figsize=(10, 5)) plt.suptitle(t=main_title, y=1.0, fontsize=14) ims = (0, 1, 2, 435, 870, 1070, 1868, 1923, 2723, 2765, 2807, 2854) for i, j in enumerate(ims, start=1): plt.subplot(2, 5, i) if len(data.target_type) == 1: if data.target_type[0] == "category": im, lab = data[j] plt.title(label=lab) elif data.target_type[0] == "annotation": im, (px, py) = data[j] plt.scatter(x=px, y=py) plt.imshow(X=im) elif len(data.target_type) == 2: if data.target_type[0] == "category": im, (lab, (px, py)) = data[j] elif data.target_type[0] == "annotation": im, ((px, py), lab) = data[j] plt.title(label=lab) plt.imshow(X=im) plt.scatter(x=px, y=py) if i == 10: break plt.tight_layout() plt.show() show_images(data=category_data, main_title="category_data") show_images(data=annotation_data, main_title="annotation_data") show_images(data=all_data, main_title="all_data") </pil.jpegimageplugin.jpegimagefile></pil.jpegimageplugin.jpegimagefile></pil.jpegimageplugin.jpegimagefile></pil.jpegimageplugin.jpegimagefile></pil.jpegimageplugin.jpegimagefile></pil.jpegimageplugin.jpegimagefile></pil.jpegimageplugin.jpegimagefile></pil.jpegimageplugin.jpegimagefile></pil.jpegimageplugin.jpegimagefile></pil.jpegimageplugin.jpegimagefile></pil.jpegimageplugin.jpegimagefile></pil.jpegimageplugin.jpegimagefile></pil.jpegimageplugin.jpegimagefile></pil.jpegimageplugin.jpegimagefile></pil.jpegimageplugin.jpegimagefile></bound>
以上是PyTorch 中的加州理工学院的详细内容。更多信息请关注PHP中文网其他相关文章!
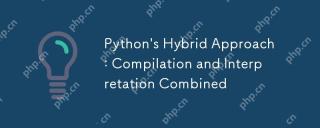
pythonuseshybridapprace,ComminingCompilationTobyTecoDeAndInterpretation.1)codeiscompiledtoplatform-Indepententbybytecode.2)bytecodeisisterpretedbybythepbybythepythonvirtualmachine,增强效率和通用性。
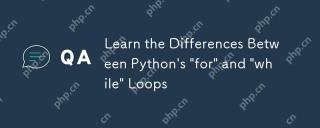
theKeyDifferencesBetnewpython's“ for”和“ for”和“ loopsare:1)” for“ loopsareIdealForiteringSequenceSquencesSorkNowniterations,而2)”,而“ loopsareBetterforConterContinuingUntilacTientInditionIntionismetismetistismetistwithOutpredefinedInedIterations.un
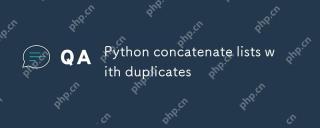
在Python中,可以通过多种方法连接列表并管理重复元素:1)使用 运算符或extend()方法可以保留所有重复元素;2)转换为集合再转回列表可以去除所有重复元素,但会丢失原有顺序;3)使用循环或列表推导式结合集合可以去除重复元素并保持原有顺序。
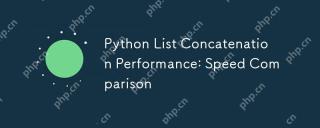
fasteStmethodMethodMethodConcatenationInpythondependersonListsize:1)forsmalllists,operatorseffited.2)forlargerlists,list.extend.extend()orlistComprechensionfaster,withextendEffaster,withExtendEffers,withextend()withextend()是extextend()asmoremory-ememory-emmoremory-emmoremory-emmodifyinginglistsin-place-place-place。
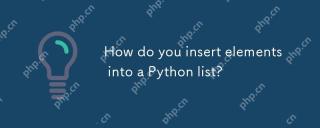
toInSerteLementIntoApythonList,useAppend()toaddtotheend,insert()foreSpificPosition,andextend()formultiplelements.1)useappend()foraddingsingleitemstotheend.2)useAddingsingLeitemStotheend.2)useeapecificindex,toadapecificindex,toadaSpecificIndex,toadaSpecificIndex,blyit'ssssssslorist.3 toaddextext.3
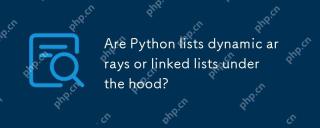
pythonlistsareimplementedasdynamicarrays,notlinkedlists.1)他们areStoredIncoNtiguulMemoryBlocks,mayrequireRealLealLocationWhenAppendingItems,EmpactingPerformance.2)LinkesedlistSwoldOfferefeRefeRefeRefeRefficeInsertions/DeletionsButslowerIndexeDexedAccess,Lestpypytypypytypypytypy
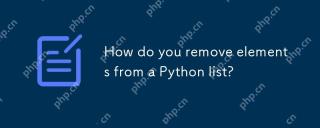
pythonoffersFourmainMethodStoreMoveElement Fromalist:1)删除(值)emovesthefirstoccurrenceofavalue,2)pop(index)emovesanderturnsanelementataSpecifiedIndex,3)delstatementremoveselemsbybybyselementbybyindexorslicebybyindexorslice,and 4)
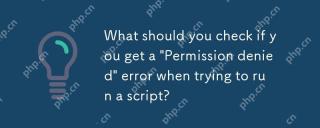
toresolvea“ dermissionded”错误Whenrunningascript,跟随台词:1)CheckAndAdjustTheScript'Spermissions ofchmod xmyscript.shtomakeitexecutable.2)nesureThEseRethEserethescriptistriptocriptibationalocatiforecationAdirectorywherewhereyOuhaveWritePerMissionsyOuhaveWritePermissionsyYouHaveWritePermissions,susteSyAsyOURHomeRecretectory。


热AI工具

Undresser.AI Undress
人工智能驱动的应用程序,用于创建逼真的裸体照片

AI Clothes Remover
用于从照片中去除衣服的在线人工智能工具。

Undress AI Tool
免费脱衣服图片

Clothoff.io
AI脱衣机

Video Face Swap
使用我们完全免费的人工智能换脸工具轻松在任何视频中换脸!

热门文章

热工具

mPDF
mPDF是一个PHP库,可以从UTF-8编码的HTML生成PDF文件。原作者Ian Back编写mPDF以从他的网站上“即时”输出PDF文件,并处理不同的语言。与原始脚本如HTML2FPDF相比,它的速度较慢,并且在使用Unicode字体时生成的文件较大,但支持CSS样式等,并进行了大量增强。支持几乎所有语言,包括RTL(阿拉伯语和希伯来语)和CJK(中日韩)。支持嵌套的块级元素(如P、DIV),
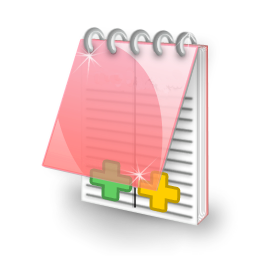
EditPlus 中文破解版
体积小,语法高亮,不支持代码提示功能

SecLists
SecLists是最终安全测试人员的伙伴。它是一个包含各种类型列表的集合,这些列表在安全评估过程中经常使用,都在一个地方。SecLists通过方便地提供安全测试人员可能需要的所有列表,帮助提高安全测试的效率和生产力。列表类型包括用户名、密码、URL、模糊测试有效载荷、敏感数据模式、Web shell等等。测试人员只需将此存储库拉到新的测试机上,他就可以访问到所需的每种类型的列表。

SublimeText3 英文版
推荐:为Win版本,支持代码提示!

PhpStorm Mac 版本
最新(2018.2.1 )专业的PHP集成开发工具