以下是代码各部分功能的详细说明:
导入库:
matplotlib.pyplot 用于创建绘图。
yahooquery.Ticker 用于从雅虎财经获取历史股票数据。
datetime 和 timedelta 用于日期操作。
pandas 用于数据处理。
pytz 用于处理时区。
os 用于文件系统操作。
函数plot_stock_last_n_days:
函数参数:
符号:股票代码(例如“NVDA”)。
n_days:显示历史数据的天数。
filename:保存绘图的文件名。
timezone:显示数据的时区。
日期范围:
当前日期和周期开始日期是根据n_days计算的。
获取数据:
yahooquery 用于检索指定时间段内的历史股票数据。
检查数据可用性:
如果没有可用数据,则会打印一条消息,然后函数退出。
数据处理:
数据的索引转换为日期时间格式并设置时区。
周末(周六和周日)被过滤掉。
计算收盘价的百分比变化。
创建和配置绘图:
主要情节是根据收盘价创建的。
绘图中添加了注释,显示收盘价和百分比变化。
配置 X 轴和 Y 轴、设置日期格式并添加网格线。
添加了额外的交易量图,用不同的颜色表示收盘价的正向和负向变化。
添加水印:
水印添加到图的左下角和右上角。
保存并显示绘图:
绘图将保存为具有指定文件名的图像文件并显示。
用法示例:
使用代码“NVDA”(NVIDIA) 调用该函数,显示过去 14 天的数据,将绘图保存为“output.png”,并使用 GMT 时区。
总之,代码生成历史股票数据的可视化表示,包括收盘价和交易量,以及百分比变化和时区注意事项的注释。
import matplotlib.pyplot as plt from yahooquery import Ticker from datetime import datetime, timedelta import matplotlib.dates as mdates import os import pandas as pd import pytz def plot_stock_last_n_days(symbol, n_days=30, filename='stock_plot.png', timezone='UTC'): # Define the date range end_date = datetime.now(pytz.timezone(timezone)) start_date = end_date - timedelta(days=n_days) # Convert dates to the format expected by Yahoo Finance start_date_str = start_date.strftime('%Y-%m-%d') end_date_str = end_date.strftime('%Y-%m-%d') # Fetch historical data for the last n days ticker = Ticker(symbol) historical_data = ticker.history(start=start_date_str, end=end_date_str, interval='1d') # Check if the data is available if historical_data.empty: print("No data available.") return # Ensure the index is datetime for proper plotting and localize to the specified timezone historical_data.index = pd.to_datetime(historical_data.index.get_level_values('date')).tz_localize('UTC').tz_convert(timezone) # Filter out weekends historical_data = historical_data[historical_data.index.weekday 0 else 'red' ax1.text(date, close, f'{close:.2f}\n({pct_change:.2f}%)', fontsize=9, ha='right', color=color) # Set up daily gridlines and print date for every day ax1.xaxis.set_major_locator(mdates.DayLocator(interval=1)) ax1.xaxis.set_major_formatter(mdates.DateFormatter('%Y-%m-%d')) ax1.set_xlabel('Date') ax1.set_ylabel('Price (USD)') ax1.set_title(f'{symbol} Stock Price - Last {n_days} Days') ax1.legend(loc='upper left') ax1.grid(True) ax1.tick_params(axis='x', rotation=80) fig.tight_layout() # Adding the trading volume plot ax2 = ax1.twinx() calm_green = (0.6, 1, 0.6) # Calm green color calm_red = (1, 0.6, 0.6) # Calm red color colors = [calm_green if historical_data['close'].iloc[i] > historical_data['open'].iloc[i] else calm_red for i in range(len(historical_data))] ax2.bar(historical_data.index, historical_data['volume'], color=colors, alpha=0.5, width=0.8) ax2.set_ylabel('Volume') ax2.tick_params(axis='y') # Format y-axis for volume in millions def millions(x, pos): 'The two args are the value and tick position' return '%1.0fM' % (x * 1e-6) ax2.yaxis.set_major_formatter(plt.FuncFormatter(millions)) # Adjust the visibility and spacing of the volume axis fig.subplots_adjust(right=0.85) ax2.spines['right'].set_position(('outward', 60)) ax2.yaxis.set_label_position('right') ax2.yaxis.set_ticks_position('right') # Add watermarks plt.text(0.01, 0.01, 'medium.com/@dmitry.romanoff', fontsize=12, color='grey', ha='left', va='bottom', alpha=0.5, transform=plt.gca().transAxes) plt.text(0.99, 0.99, 'medium.com/@dmitry.romanoff', fontsize=12, color='grey', ha='right', va='top', alpha=0.5, transform=plt.gca().transAxes) # Save the plot as an image file plt.savefig(filename) plt.show() # Example usage plot_stock_last_n_days('NVDA', n_days=14, filename='output.png', timezone='GMT')
以上是生成最近 n 天的股票价格图表的 Python 代码。的详细内容。更多信息请关注PHP中文网其他相关文章!
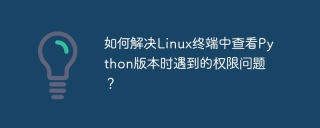
Linux终端中查看Python版本时遇到权限问题的解决方法当你在Linux终端中尝试查看Python的版本时,输入python...
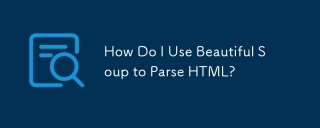
本文解释了如何使用美丽的汤库来解析html。 它详细介绍了常见方法,例如find(),find_all(),select()和get_text(),以用于数据提取,处理不同的HTML结构和错误以及替代方案(SEL)
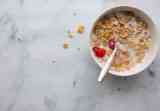
Python 对象的序列化和反序列化是任何非平凡程序的关键方面。如果您将某些内容保存到 Python 文件中,如果您读取配置文件,或者如果您响应 HTTP 请求,您都会进行对象序列化和反序列化。 从某种意义上说,序列化和反序列化是世界上最无聊的事情。谁会在乎所有这些格式和协议?您想持久化或流式传输一些 Python 对象,并在以后完整地取回它们。 这是一种在概念层面上看待世界的好方法。但是,在实际层面上,您选择的序列化方案、格式或协议可能会决定程序运行的速度、安全性、维护状态的自由度以及与其他系
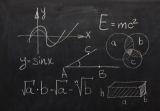
Python的statistics模块提供强大的数据统计分析功能,帮助我们快速理解数据整体特征,例如生物统计学和商业分析等领域。无需逐个查看数据点,只需查看均值或方差等统计量,即可发现原始数据中可能被忽略的趋势和特征,并更轻松、有效地比较大型数据集。 本教程将介绍如何计算平均值和衡量数据集的离散程度。除非另有说明,本模块中的所有函数都支持使用mean()函数计算平均值,而非简单的求和平均。 也可使用浮点数。 import random import statistics from fracti
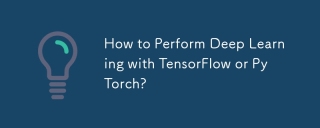
本文比较了Tensorflow和Pytorch的深度学习。 它详细介绍了所涉及的步骤:数据准备,模型构建,培训,评估和部署。 框架之间的关键差异,特别是关于计算刻度的
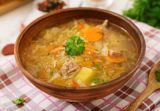
该教程建立在先前对美丽汤的介绍基础上,重点是简单的树导航之外的DOM操纵。 我们将探索有效的搜索方法和技术,以修改HTML结构。 一种常见的DOM搜索方法是EX
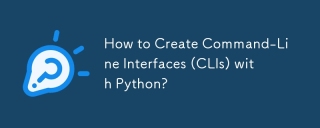
本文指导Python开发人员构建命令行界面(CLIS)。 它使用Typer,Click和ArgParse等库详细介绍,强调输入/输出处理,并促进用户友好的设计模式,以提高CLI可用性。
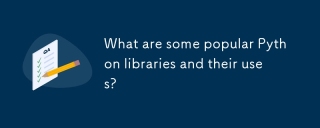
本文讨论了诸如Numpy,Pandas,Matplotlib,Scikit-Learn,Tensorflow,Tensorflow,Django,Blask和请求等流行的Python库,并详细介绍了它们在科学计算,数据分析,可视化,机器学习,网络开发和H中的用途


热AI工具

Undresser.AI Undress
人工智能驱动的应用程序,用于创建逼真的裸体照片

AI Clothes Remover
用于从照片中去除衣服的在线人工智能工具。

Undress AI Tool
免费脱衣服图片

Clothoff.io
AI脱衣机

AI Hentai Generator
免费生成ai无尽的。

热门文章

热工具
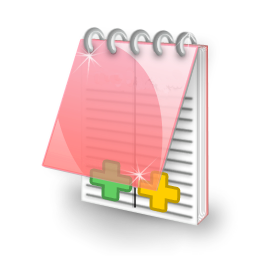
EditPlus 中文破解版
体积小,语法高亮,不支持代码提示功能

SecLists
SecLists是最终安全测试人员的伙伴。它是一个包含各种类型列表的集合,这些列表在安全评估过程中经常使用,都在一个地方。SecLists通过方便地提供安全测试人员可能需要的所有列表,帮助提高安全测试的效率和生产力。列表类型包括用户名、密码、URL、模糊测试有效载荷、敏感数据模式、Web shell等等。测试人员只需将此存储库拉到新的测试机上,他就可以访问到所需的每种类型的列表。

禅工作室 13.0.1
功能强大的PHP集成开发环境

Atom编辑器mac版下载
最流行的的开源编辑器

SublimeText3汉化版
中文版,非常好用