Speeding Up API Development: Handling High-Load Requests Efficiently with Python
API development is a cornerstone of modern software applications, from mobile apps to web platforms and microservices. However, as user demands grow, so do the challenges of handling high-load requests efficiently. Python, a versatile and powerful language, often comes under scrutiny for its performance limitations in high-load scenarios. But with the right techniques, Python can handle large-scale API requests smoothly.
In this article, we’ll explore best practices and techniques for optimizing Python APIs to efficiently process millions of requests per second, minimizing latency and improving overall performance.
Python’s Role in API Development
Python is widely used for API development due to its simplicity, rich ecosystem, and ability to rapidly prototype and deploy applications. Frameworks like Flask and FastAPI have made it easy to develop APIs, but Python is often criticized for not being as fast as languages like Go or Rust. However, there are several strategies you can employ to get the most out of Python’s performance when building APIs.
1. Asynchronous Programming with AsyncIO
One of the key challenges in handling a large number of API requests is managing I/O-bound tasks, such as reading from a database or external services. Traditional Python programs execute tasks sequentially, which can slow down performance. Enter asynchronous programming.
Using asyncio and other asynchronous libraries allows Python to handle multiple tasks concurrently, without blocking the execution of other operations. This is particularly useful for APIs that need to make frequent external calls (e.g., to databases or third-party APIs).
import asyncio async def fetch_data(session, url): async with session.get(url) as response: return await response.json() async def main(): async with aiohttp.ClientSession() as session: tasks = [fetch_data(session, f'http://example.com/{i}') for i in range(100)] results = await asyncio.gather(*tasks) print(results) asyncio.run(main())
2. Leveraging FastAPI for Performance
If you’re looking to boost your Python API’s performance, FastAPI is an excellent choice. FastAPI is designed to be modern, fast, and easy to use. It's built on Starlette for the web parts and Pydantic for data validation, enabling it to serve APIs at speeds comparable to Node.js and Go.
FastAPI supports asynchronous programming natively, and its performance benefits are noticeable right out of the box:
Auto-generated documentation: FastAPI automatically creates OpenAPI and JSON Schema for your API endpoints, which saves time and effort.
High-speed performance: It uses the same async patterns as other high-performance frameworks but is more Pythonic and developer-friendly.
from fastapi import FastAPI app = FastAPI() @app.get("/items/{item_id}") async def read_item(item_id: int): return {"item_id": item_id}
FastAPI can serve tens of thousands of requests per second, depending on your infrastructure, and is highly optimized for asynchronous I/O.
3. Optimizing Database Queries
APIs that rely heavily on database interactions can face significant slowdowns if queries are not optimized. Here are a few strategies to improve database performance:
Batch queries: Rather than querying the database for each individual request, batch multiple queries into a single one to reduce the number of round trips to the database.
Use connection pooling: Database connection setup can be a performance bottleneck. Using a connection pool ensures that connections are reused and not constantly created and destroyed.
Optimize query design: Ensure your SQL queries are using appropriate indexes and avoid fetching unnecessary data.
In Python, using an ORM like SQLAlchemy can help manage database interactions, but for performance-critical tasks, it’s often better to write raw SQL queries.
from sqlalchemy import create_engine engine = create_engine('sqlite:///example.db') def get_data(): with engine.connect() as connection: result = connection.execute("SELECT * FROM data LIMIT 1000") return result.fetchall()
4. Caching for High-Load Scenarios
When dealing with high loads, one of the most effective ways to reduce the strain on your API is by implementing caching. Frequently requested data can be cached either in-memory (using tools like Redis) or via HTTP headers to minimize redundant processing.
In-memory caching: Use a tool like Redis to store frequently accessed data and reduce the number of database calls.
Response caching: Set appropriate HTTP cache headers to instruct clients and intermediate proxies to cache responses.
import redis r = redis.Redis() # Example: caching API response def get_user_profile(user_id): cache_key = f"user_profile:{user_id}" cached_profile = r.get(cache_key) if cached_profile: return cached_profile # Simulate a database call profile = {"id": user_id, "name": "John Doe"} # Cache for future requests r.set(cache_key, profile, ex=3600) # Cache for 1 hour return profile
5. Horizontal Scaling with Load Balancing
For truly high-load applications, even the most optimized Python code can hit bottlenecks. At this point, horizontal scaling becomes necessary. This involves adding more servers or instances of your API, and using a load balancer to distribute incoming requests across all available resources.
Tools like NGINX or HAProxy can be used as load balancers to evenly distribute traffic across multiple API instances, ensuring that no single server is overwhelmed.
以上是Speeding Up API Development: Handling High-Load Requests Efficiently with Python的详细内容。更多信息请关注PHP中文网其他相关文章!
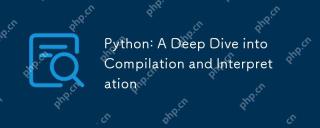
pythonisehybridmodelofcompilationand interpretation:1)thepythoninterspretercompilesourcececodeintoplatform- interpententbybytecode.2)thepytythonvirtualmachine(pvm)thenexecuteCutestestestesteSteSteSteSteSteSthisByTecode,BelancingEaseofuseWithPerformance。
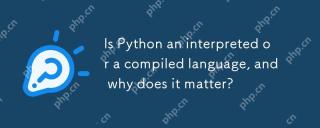
pythonisbothinterpretedAndCompiled.1)它的compiledTobyTecodeForportabilityAcrosplatforms.2)bytecodeisthenInterpreted,允许fordingfordforderynamictynamictymictymictymictyandrapiddefupment,尽管Ititmaybeslowerthananeflowerthanancompiledcompiledlanguages。
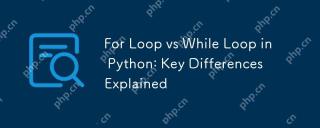
在您的知识之际,而foroopsareideal insinAdvance中,而WhileLoopSareBetterForsituations则youneedtoloopuntilaconditionismet
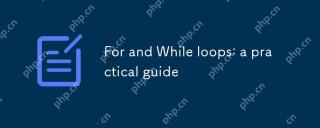
ForboopSareSusedwhenthentheneMberofiterationsiskNownInAdvance,而WhileLoopSareSareDestrationsDepportonAcondition.1)ForloopSareIdealForiteratingOverSequencesLikelistSorarrays.2)whileLeleLooleSuitableApeableableableableableableforscenarioscenarioswhereTheLeTheLeTheLeTeLoopContinusunuesuntilaspecificiccificcificCondond
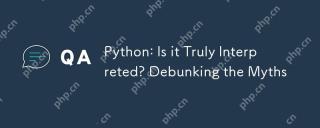
pythonisnotpuroly interpred; itosisehybridablectofbytecodecompilationandruntimeinterpretation.1)PythonCompiLessourceceCeceDintobyTecode,whitsthenexecececected bytybytybythepythepythepythonvirtirtualmachine(pvm).2)
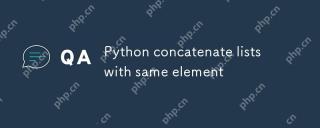
concateNateListsinpythonwithTheSamelements,使用:1)operatototakeepduplicates,2)asettoremavelemavphicates,or3)listCompreanspearensionforcontroloverduplicates,每个methodhasdhasdifferentperferentperferentperforentperforentperforentperfortenceandordormplications。
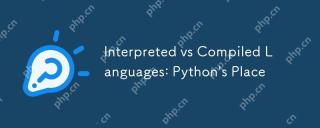
pythonisanterpretedlanguage,offeringosofuseandflexibilitybutfacingperformancelanceLimitationsInCricapplications.1)drightingedlanguageslikeLikeLikeLikeLikeLikeLikeLikeThonexecuteline-by-line,允许ImmediaMediaMediaMediaMediaMediateFeedBackAndBackAndRapidPrototypiD.2)compiledLanguagesLanguagesLagagesLikagesLikec/c thresst
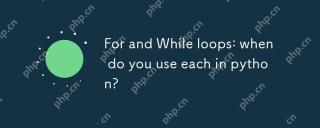
Useforloopswhenthenumberofiterationsisknowninadvance,andwhileloopswheniterationsdependonacondition.1)Forloopsareidealforsequenceslikelistsorranges.2)Whileloopssuitscenarioswheretheloopcontinuesuntilaspecificconditionismet,usefulforuserinputsoralgorit


热AI工具

Undresser.AI Undress
人工智能驱动的应用程序,用于创建逼真的裸体照片

AI Clothes Remover
用于从照片中去除衣服的在线人工智能工具。

Undress AI Tool
免费脱衣服图片

Clothoff.io
AI脱衣机

Video Face Swap
使用我们完全免费的人工智能换脸工具轻松在任何视频中换脸!

热门文章

热工具
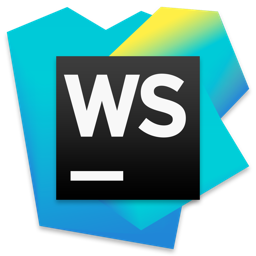
WebStorm Mac版
好用的JavaScript开发工具

SublimeText3汉化版
中文版,非常好用

mPDF
mPDF是一个PHP库,可以从UTF-8编码的HTML生成PDF文件。原作者Ian Back编写mPDF以从他的网站上“即时”输出PDF文件,并处理不同的语言。与原始脚本如HTML2FPDF相比,它的速度较慢,并且在使用Unicode字体时生成的文件较大,但支持CSS样式等,并进行了大量增强。支持几乎所有语言,包括RTL(阿拉伯语和希伯来语)和CJK(中日韩)。支持嵌套的块级元素(如P、DIV),
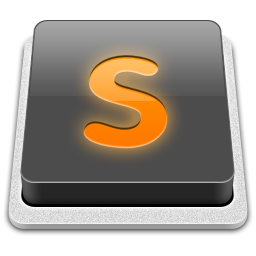
SublimeText3 Mac版
神级代码编辑软件(SublimeText3)

PhpStorm Mac 版本
最新(2018.2.1 )专业的PHP集成开发工具