Introduction
We'll create an AI agent capable of searching Wikipedia and answering questions based on the information it finds. This ReAct (Reason and Act) Agent uses the Google Generative AI API to process queries and generate responses. Our agent will be able to:
- Search Wikipedia for relevant information.
- Extract specific sections from Wikipedia pages.
- Reason about the information gathered and formulate answers.
[2] What is a ReAct Agent?
A ReAct Agent is a specific type of agent that follows a Reflection-Action cycle. It reflects on the current task, based on available information and actions it can perform, and then decides which action to take or whether to conclude the task.
[3] Planning the Agent
3.1 Required Tools
- Node.js
- Axios library for HTTP requests
- Google Generative AI API (gemini-1.5-flash)
- Wikipedia API
3.2 Agent Structure
Our ReAct Agent will have three main states:
- THOUGHT (Reflection)
- ACTION (Execution)
- ANSWER (Response)
[4] Implementing the Agent
Let's build the ReAct Agent step by step, highlighting each state.
4.1 Initial Setup
First, set up the project and install dependencies:
mkdir react-agent-project cd react-agent-project npm init -y npm install axios dotenv @google/generative-ai
Create a .env file at the project's root:
GOOGLE_AI_API_KEY=your_api_key_here
4.2 Creating the Tools.js File
Create Tools.js with the following content:
const axios = require("axios"); class Tools { static async wikipedia(q) { try { const response = await axios.get("https://en.wikipedia.org/w/api.php", { params: { action: "query", list: "search", srsearch: q, srwhat: "text", format: "json", srlimit: 4, }, }); const results = await Promise.all( response.data.query.search.map(async (searchResult) => { const sectionResponse = await axios.get( "https://en.wikipedia.org/w/api.php", { params: { action: "parse", pageid: searchResult.pageid, prop: "sections", format: "json", }, }, ); const sections = Object.values( sectionResponse.data.parse.sections, ).map((section) => `${section.index}, ${section.line}`); return { pageTitle: searchResult.title, snippet: searchResult.snippet, pageId: searchResult.pageid, sections: sections, }; }), ); return results .map( (result) => `Snippet: ${result.snippet}\nPageId: ${result.pageId}\nSections: ${JSON.stringify(result.sections)}`, ) .join("\n\n"); } catch (error) { console.error("Error fetching from Wikipedia:", error); return "Error fetching data from Wikipedia"; } } static async wikipedia_with_pageId(pageId, sectionId) { if (sectionId) { const response = await axios.get("https://en.wikipedia.org/w/api.php", { params: { action: "parse", format: "json", pageid: parseInt(pageId), prop: "wikitext", section: parseInt(sectionId), disabletoc: 1, }, }); return Object.values(response.data.parse?.wikitext ?? {})[0]?.substring( 0, 25000, ); } else { const response = await axios.get("https://en.wikipedia.org/w/api.php", { params: { action: "query", pageids: parseInt(pageId), prop: "extracts", exintro: true, explaintext: true, format: "json", }, }); return Object.values(response.data?.query.pages)[0]?.extract; } } } module.exports = Tools;
4.3 Creating the ReactAgent.js File
Create ReactAgent.js with the following content:
require("dotenv").config(); const { GoogleGenerativeAI } = require("@google/generative-ai"); const Tools = require("./Tools"); const genAI = new GoogleGenerativeAI(process.env.GOOGLE_AI_API_KEY); class ReActAgent { constructor(query, functions) { this.query = query; this.functions = new Set(functions); this.state = "THOUGHT"; this._history = []; this.model = genAI.getGenerativeModel({ model: "gemini-1.5-flash", temperature: 2, }); } get history() { return this._history; } pushHistory(value) { this._history.push(`\n ${value}`); } async run() { this.pushHistory(`**Task: ${this.query} **`); try { return await this.step(); } catch (e) { if (e.message.includes("exhausted")) { return "Sorry, I'm exhausted, I can't process your request anymore. ><"; } return "Unable to process your request, please try again? ><"; } } async step() { const colors = { reset: "\x1b[0m", yellow: "\x1b[33m", red: "\x1b[31m", cyan: "\x1b[36m", }; console.log("===================================="); console.log( `Next Movement: ${ this.state === "THOUGHT" ? colors.yellow : this.state === "ACTION" ? colors.red : this.state === "ANSWER" ? colors.cyan : colors.reset }${this.state}${colors.reset}`, ); console.log(`Last Movement: ${this.history[this.history.length - 1]}`); console.log("===================================="); switch (this.state) { case "THOUGHT": await this.thought(); break; case "ACTION": await this.action(); break; case "ANSWER": await this.answer(); break; } } async promptModel(prompt) { const result = await this.model.generateContent(prompt); const response = await result.response; return response.text(); } async thought() { const availableFunctions = JSON.stringify(Array.from(this.functions)); const historyContext = this.history.join("\n"); const prompt = `Your task to FullFill ${this.query}. Context contains all the reflection you made so far and the ActionResult you collected. AvailableActions are functions you can call whenever you need more data. Context: "${historyContext}" << AvailableActions: "${availableFunctions}" << Task: "${this.query}" << Reflect uppon Your Task using Context, ActionResult and AvailableActions to find your next_step. print your next_step with a Thought or FullFill Your Task `; const thought = await this.promptModel(prompt); this.pushHistory(`\n **${thought.trim()}**`); if ( thought.toLowerCase().includes("fullfill") || thought.toLowerCase().includes("fulfill") ) { this.state = "ANSWER"; return await this.step(); } this.state = "ACTION"; return await this.step(); } async action() { const action = await this.decideAction(); this.pushHistory(`** Action: ${action} **`); const result = await this.executeFunctionCall(action); this.pushHistory(`** ActionResult: ${result} **`); this.state = "THOUGHT"; return await this.step(); } async decideAction() { const availableFunctions = JSON.stringify(Array.from(this.functions)); const historyContext = this.history; const prompt = `Reflect uppon the Thought, Query and AvailableActions ${historyContext[historyContext.length - 2]} Thought <<< ${historyContext[historyContext.length - 1]} Query: "${this.query}" AvailableActions: ${availableFunctions} output only the function,parametervalues separated by a comma. For example: "wikipedia,ronaldinho gaucho, 1450"`; const decision = await this.promptModel(prompt); return `${decision.replace(/`/g, "").trim()}`; } async executeFunctionCall(functionCall) { const [functionName, ...args] = functionCall.split(","); const func = Tools[functionName.trim()]; if (func) { return await func.call(null, ...args); } throw new Error(`Function ${functionName} not found`); } async answer() { const historyContext = this.history; const prompt = `Based on the following context, provide a complete, detailed and descriptive formated answer for the Following Task: ${this.query} . Context: ${historyContext} Task: "${this.query}"`; const finalAnswer = await this.promptModel(prompt); this.history.push(`Answer: ${this.finalAnswer}`); console.log("WE WILL ANSWER >>>>>>>", finalAnswer); return finalAnswer; } } module.exports = ReActAgent;
4.4 Running the agent (index.js)
Create index.js with the following content:
const ReActAgent = require("./ReactAgent.js"); async function main() { const query = "What does England border with?"; const functions = [ [ "wikipedia", "params: query", "Semantic Search Wikipedia API for snippets, pageIds and sectionIds >> \n ex: Date brazil has been colonized? \n Brazil was colonized at 1500, pageId, sections : []", ], [ "wikipedia_with_pageId", "params : pageId, sectionId", "Search Wikipedia API for data using a pageId and a sectionIndex as params. \n ex: 1500, 1234 \n Section information about blablalbal", ], ]; const agent = new ReActAgent(query, functions); try { const result = await agent.run(); console.log("THE AGENT RETURN THE FOLLOWING >>>", result); } catch (e) { console.log("FAILED TO RUN T.T", e); } } main().catch(console.error);
[5] How the Wikipedia Part Works
The interaction with Wikipedia is done in two main steps:
-
Initial search (wikipedia function):
- Makes a request to the Wikipedia search API.
- Returns up to 4 relevant results for the query.
- For each result, it fetches the sections of the page.
-
Detailed search (wikipedia_with_pageId function):
- Uses the page ID and section ID to fetch specific content.
- Returns the text of the requested section.
This process allows the agent to first get an overview of topics related to the query and then dive deeper into specific sections as needed.
[6] Execution Flow Example
- The user asks a question.
- The agent enters the THOUGHT state and reflects on the question.
- It decides to search Wikipedia and enters the ACTION state.
- Executes the wikipedia function and obtains results.
- Returns to the THOUGHT state to reflect on the results.
- May decide to search for more details or a different approach.
- Repeats the THOUGHT and ACTION cycle as necessary.
- When it has sufficient information, it enters the ANSWER state.
- Generates a final answer based on all the information collected.
- Enters infinite loop whenever the wikipedia doesn't have the data to collect. Fix it with a timer =P
[7] Final Considerations
- The modular structure allows for easy addition of new tools or APIs.
- It's important to implement error handling and time/iteration limits to avoid infinite loops or excessive resource use.
- Use Temperature : 99999 lol
以上是Creating a ReAct Agent from the scratch with nodeJS ( wikipedia search )的详细内容。更多信息请关注PHP中文网其他相关文章!
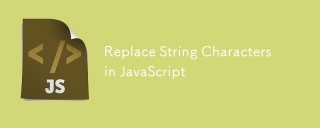
JavaScript字符串替换方法详解及常见问题解答 本文将探讨两种在JavaScript中替换字符串字符的方法:在JavaScript代码内部替换和在网页HTML内部替换。 在JavaScript代码内部替换字符串 最直接的方法是使用replace()方法: str = str.replace("find","replace"); 该方法仅替换第一个匹配项。要替换所有匹配项,需使用正则表达式并添加全局标志g: str = str.replace(/fi
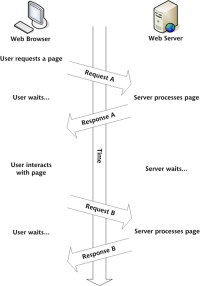
因此,在这里,您准备好了解所有称为Ajax的东西。但是,到底是什么? AJAX一词是指用于创建动态,交互式Web内容的一系列宽松的技术。 Ajax一词,最初由Jesse J创造
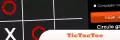
10款趣味横生的jQuery游戏插件,让您的网站更具吸引力,提升用户粘性!虽然Flash仍然是开发休闲网页游戏的最佳软件,但jQuery也能创造出令人惊喜的效果,虽然无法与纯动作Flash游戏媲美,但在某些情况下,您也能在浏览器中获得意想不到的乐趣。 jQuery井字棋游戏 游戏编程的“Hello world”,现在有了jQuery版本。 源码 jQuery疯狂填词游戏 这是一个填空游戏,由于不知道单词的上下文,可能会产生一些古怪的结果。 源码 jQuery扫雷游戏
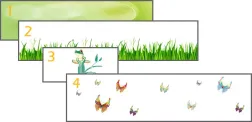
本教程演示了如何使用jQuery创建迷人的视差背景效果。 我们将构建一个带有分层图像的标题横幅,从而创造出令人惊叹的视觉深度。 更新的插件可与JQuery 1.6.4及更高版本一起使用。 下载

本文讨论了在浏览器中优化JavaScript性能的策略,重点是减少执行时间并最大程度地减少对页面负载速度的影响。
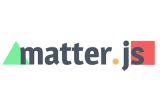
Matter.js是一个用JavaScript编写的2D刚体物理引擎。此库可以帮助您轻松地在浏览器中模拟2D物理。它提供了许多功能,例如创建刚体并为其分配质量、面积或密度等物理属性的能力。您还可以模拟不同类型的碰撞和力,例如重力摩擦力。 Matter.js支持所有主流浏览器。此外,它也适用于移动设备,因为它可以检测触摸并具有响应能力。所有这些功能都使其值得您投入时间学习如何使用该引擎,因为这样您就可以轻松创建基于物理的2D游戏或模拟。在本教程中,我将介绍此库的基础知识,包括其安装和用法,并提供一
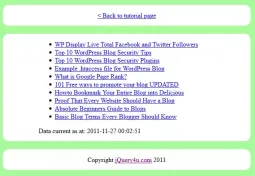
本文演示了如何使用jQuery和ajax自动每5秒自动刷新DIV的内容。 该示例从RSS提要中获取并显示了最新的博客文章以及最后的刷新时间戳。 加载图像是选择


热AI工具

Undresser.AI Undress
人工智能驱动的应用程序,用于创建逼真的裸体照片

AI Clothes Remover
用于从照片中去除衣服的在线人工智能工具。

Undress AI Tool
免费脱衣服图片

Clothoff.io
AI脱衣机

AI Hentai Generator
免费生成ai无尽的。

热门文章

热工具

Atom编辑器mac版下载
最流行的的开源编辑器
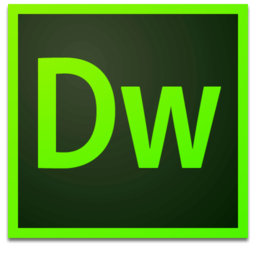
Dreamweaver Mac版
视觉化网页开发工具

安全考试浏览器
Safe Exam Browser是一个安全的浏览器环境,用于安全地进行在线考试。该软件将任何计算机变成一个安全的工作站。它控制对任何实用工具的访问,并防止学生使用未经授权的资源。

DVWA
Damn Vulnerable Web App (DVWA) 是一个PHP/MySQL的Web应用程序,非常容易受到攻击。它的主要目标是成为安全专业人员在合法环境中测试自己的技能和工具的辅助工具,帮助Web开发人员更好地理解保护Web应用程序的过程,并帮助教师/学生在课堂环境中教授/学习Web应用程序安全。DVWA的目标是通过简单直接的界面练习一些最常见的Web漏洞,难度各不相同。请注意,该软件中

mPDF
mPDF是一个PHP库,可以从UTF-8编码的HTML生成PDF文件。原作者Ian Back编写mPDF以从他的网站上“即时”输出PDF文件,并处理不同的语言。与原始脚本如HTML2FPDF相比,它的速度较慢,并且在使用Unicode字体时生成的文件较大,但支持CSS样式等,并进行了大量增强。支持几乎所有语言,包括RTL(阿拉伯语和希伯来语)和CJK(中日韩)。支持嵌套的块级元素(如P、DIV),