人脸识别技术在从安全系统到社交媒体的各种应用中变得越来越普遍。对于此任务最有效的模型之一是 FaceNet,这是一种专为人脸验证、识别和聚类而设计的深度学习模型。
在本教程中,我将向您展示如何使用 FaceNet 在 Python 中构建人脸识别系统。我们将涵盖从加载模型到比较面部的所有内容。读完本指南后,您将为在自己的项目中实现人脸识别奠定坚实的基础。
什么是FaceNet?
FaceNet 是 Google 开发的深度学习模型,可将人脸映射到 128 维欧几里德空间。这些嵌入代表了面部的基本特征,可以轻松地以高精度比较和识别面部。与传统的人脸识别方法不同,FaceNet 专注于嵌入学习,这使得它非常有效且可扩展。
先决条件
在深入研究代码之前,请确保您已安装以下软件:
- Python 3.x
- TensorFlow 或 Keras(用于深度学习模型)
- NumPy(用于数值运算)
- OpenCV(用于图像处理)
- Scikit-learn(用于应用最近邻搜索)
您可以使用 pip 安装这些依赖项:
pip install tensorflow numpy opencv-python scikit-learn
第 1 步:加载预训练的 FaceNet 模型
首先,我们将加载预先训练的 FaceNet 模型。您可以从可信来源下载模型,也可以使用 keras-facenet 库提供的模型。
from keras.models import load_model # Load the pre-trained FaceNet model model = load_model('facenet_keras.h5') print("Model Loaded Successfully")
加载模型是设置我们的人脸识别系统的第一步。该模型将用于生成图像的嵌入,这是面部的数字表示。
第 2 步:为 FaceNet 预处理图像
FaceNet 期望输入图像为 RGB 格式的 160x160 像素。此外,像素值在输入模型之前需要进行标准化。
import cv2 import numpy as np def preprocess_image(image_path): # Load the image using OpenCV img = cv2.imread(image_path) # Convert the image to RGB (FaceNet expects RGB images) img = cv2.cvtColor(img, cv2.COLOR_BGR2RGB) # Resize the image to 160x160 pixels img = cv2.resize(img, (160, 160)) # Normalize the pixel values img = img.astype('float32') / 255.0 # Expand dimensions to match the input shape of FaceNet (1, 160, 160, 3) img = np.expand_dims(img, axis=0) return img
该函数处理 FaceNet 所需的图像预处理。它将图像转换为适当的格式和大小,确保模型接收到可以有效使用的输入。
第 3 步:生成人脸嵌入
接下来,我们将使用 FaceNet 模型从预处理图像生成嵌入。这些嵌入将作为面部的独特数字表示。
def get_face_embedding(model, image_path): # Preprocess the image img = preprocess_image(image_path) # Generate the embedding embedding = model.predict(img) return embedding
get_face_embedding 函数接收模型和图像路径,处理图像并返回嵌入。我们将使用此嵌入来进行面部比较。
第 4 步:使用嵌入比较人脸
为了确定两个面孔是否匹配,我们通过计算它们之间的欧几里得距离来比较它们的嵌入。如果距离低于某个阈值,则认为面孔匹配。
from numpy import linalg as LA def compare_faces(embedding1, embedding2, threshold=0.5): # Compute the Euclidean distance between the embeddings distance = LA.norm(embedding1 - embedding2) # Compare the distance to the threshold if distance <p>compare_faces 函数计算两个嵌入之间的距离。如果该距离小于指定阈值(默认为 0.5),该函数将打印“Face Matched”。否则,它会打印“面孔不同。”</p> <h2> 第5步:测试人脸识别系统 </h2> <p>最后,让我们用两张图像来测试我们的人脸识别系统,看看它是否正确地将它们识别为同一个人。<br> </p> <pre class="brush:php;toolbar:false"># Load the FaceNet model model = load_model('facenet_keras.h5') # Get embeddings for two images embedding1 = get_face_embedding(model, 'face1.jpg') embedding2 = get_face_embedding(model, 'face2.jpg') # Compare the two faces distance = compare_faces(embedding1, embedding2) print(f"Euclidean Distance: {distance}")
输出
- 如果面孔匹配,您将看到:面孔匹配。
- 如果它们不匹配,您会看到:面孔不同。
此外,还将打印两个嵌入之间的欧几里德距离。
结论
您刚刚使用 Python 中的 FaceNet 构建了一个简单但功能强大的人脸识别系统。该系统可以轻松扩展以包含更多面孔、处理实时识别或集成到更大的项目中。 FaceNet 的高精度和高效率使其成为人脸识别任务的绝佳选择。
随意尝试阈值,或尝试在实时应用程序中使用该系统,例如基于网络摄像头的人脸识别工具。
如果您有任何疑问或需要进一步帮助,请在下面发表评论。快乐编码!
以上是如何在 Python 中使用 FaceNet 构建人脸识别系统的详细内容。更多信息请关注PHP中文网其他相关文章!
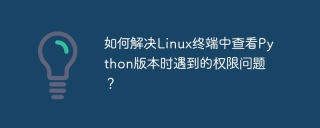
Linux终端中查看Python版本时遇到权限问题的解决方法当你在Linux终端中尝试查看Python的版本时,输入python...
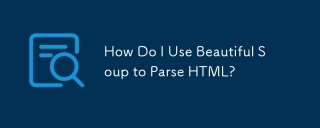
本文解释了如何使用美丽的汤库来解析html。 它详细介绍了常见方法,例如find(),find_all(),select()和get_text(),以用于数据提取,处理不同的HTML结构和错误以及替代方案(SEL)
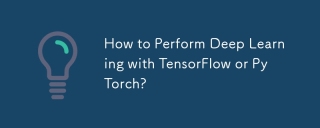
本文比较了Tensorflow和Pytorch的深度学习。 它详细介绍了所涉及的步骤:数据准备,模型构建,培训,评估和部署。 框架之间的关键差异,特别是关于计算刻度的
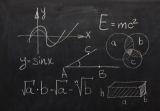
Python的statistics模块提供强大的数据统计分析功能,帮助我们快速理解数据整体特征,例如生物统计学和商业分析等领域。无需逐个查看数据点,只需查看均值或方差等统计量,即可发现原始数据中可能被忽略的趋势和特征,并更轻松、有效地比较大型数据集。 本教程将介绍如何计算平均值和衡量数据集的离散程度。除非另有说明,本模块中的所有函数都支持使用mean()函数计算平均值,而非简单的求和平均。 也可使用浮点数。 import random import statistics from fracti
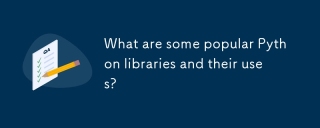
本文讨论了诸如Numpy,Pandas,Matplotlib,Scikit-Learn,Tensorflow,Tensorflow,Django,Blask和请求等流行的Python库,并详细介绍了它们在科学计算,数据分析,可视化,机器学习,网络开发和H中的用途
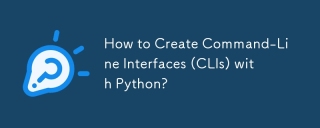
本文指导Python开发人员构建命令行界面(CLIS)。 它使用Typer,Click和ArgParse等库详细介绍,强调输入/输出处理,并促进用户友好的设计模式,以提高CLI可用性。
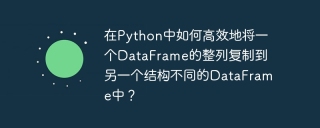
在使用Python的pandas库时,如何在两个结构不同的DataFrame之间进行整列复制是一个常见的问题。假设我们有两个Dat...
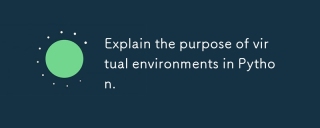
文章讨论了虚拟环境在Python中的作用,重点是管理项目依赖性并避免冲突。它详细介绍了他们在改善项目管理和减少依赖问题方面的创建,激活和利益。


热AI工具

Undresser.AI Undress
人工智能驱动的应用程序,用于创建逼真的裸体照片

AI Clothes Remover
用于从照片中去除衣服的在线人工智能工具。

Undress AI Tool
免费脱衣服图片

Clothoff.io
AI脱衣机

AI Hentai Generator
免费生成ai无尽的。

热门文章

热工具
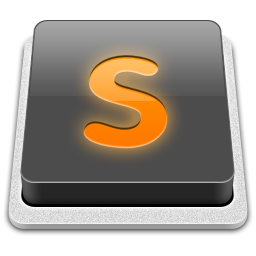
SublimeText3 Mac版
神级代码编辑软件(SublimeText3)

适用于 Eclipse 的 SAP NetWeaver 服务器适配器
将Eclipse与SAP NetWeaver应用服务器集成。

MinGW - 适用于 Windows 的极简 GNU
这个项目正在迁移到osdn.net/projects/mingw的过程中,你可以继续在那里关注我们。MinGW:GNU编译器集合(GCC)的本地Windows移植版本,可自由分发的导入库和用于构建本地Windows应用程序的头文件;包括对MSVC运行时的扩展,以支持C99功能。MinGW的所有软件都可以在64位Windows平台上运行。
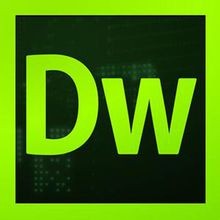
Dreamweaver CS6
视觉化网页开发工具
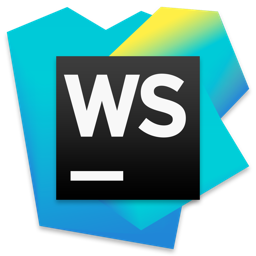
WebStorm Mac版
好用的JavaScript开发工具