電腦科學中良好的問題解決很大程度上依賴高效的演算法,例如貪婪最佳優先搜尋(GBFS)。 GBFS 已經確立了作為尋路或優化問題的最佳解決方法的可信度。因此,我們在本文中深入討論 GBFS,同時探索其使用 C 的實作方法。
文法
void greedyBestFirstSearch(Graph graph, Node startNode, Node goalNode);
演算法
貪心最佳優先搜尋演算法旨在找到圖中從給定起始節點到目標節點的路徑。以下是該演算法的一般步驟 -
初始化一個空的優先權佇列。
將起始節點放入優先權佇列。
建立一個空集合來追蹤造訪過的節點。
當優先權佇列不為空時 -
將優先權最高的節點從佇列中出列。
如果出隊的節點是目標節點,則演算法終止,並找到路徑。
否則,將出隊節點標記為已存取。
將出隊節點的所有未存取的鄰居節點放入優先權佇列中。
如果優先權佇列在到達目標節點之前變空,則不存在路徑。
方法一:基於歐氏距離的啟發式函數
範例
#include <iostream> #include <queue> #include <cmath> #include <vector> #include <unordered_set> using namespace std; // Structure to represent a node in the graph struct Node { int x, y; // Coordinates of the node int cost; // Cost to reach this node }; // Euclidean distance heuristic function double euclideanDistance(int x1, int y1, int x2, int y2) { return sqrt(pow((x1 - x2), 2) + pow((y1 - y2), 2)); } // Custom comparison function for nodes in the priority queue struct NodeCompare { bool operator()(const Node& node1, const Node& node2) const { return node1.cost > node2.cost; } }; // Greedy Best-First Search function void greedyBestFirstSearch(vector<vector<int>>& graph, Node start, Node goal) { int rows = graph.size(); int cols = graph[0].size(); // Priority queue for nodes to be explored priority_queue<Node, vector<Node>, NodeCompare> pq; // Visited nodes set unordered_set<int> visited; // Add the start node to the priority queue pq.push(start); while (!pq.empty()) { // Get the node with the lowest cost Node current = pq.top(); pq.pop(); // Check if the current node is the goal node if (current.x == goal.x && current.y == goal.y) { cout << "Goal node reached!" << endl; return; } // Mark the current node as visited int nodeId = current.x * cols + current.y; visited.insert(nodeId); // Explore the neighboring nodes int dx[] = {-1, 1, 0, 0}; // Possible x-direction movements int dy[] = {0, 0, -1, 1}; // Possible y-direction movements for (int i = 0; i < 4; i++) { int newX = current.x + dx[i]; int newY = current.y + dy[i]; // Check if the neighboring node is within the graph boundaries if (newX >= 0 && newX < rows && newY >= 0 && newY < cols) { // Calculate the heuristic value for the neighboring node double heuristicValue = euclideanDistance(newX, newY, goal.x, goal.y); // Check if the neighboring node has not been visited if (visited.find(newX * cols + newY) == visited.end()) { // Create a new node for the neighboring position Node neighbor; neighbor.x = newX; neighbor.y = newY; neighbor.cost = current.cost + graph[newX][newY]; // Add the neighboring node to the priority queue pq.push(neighbor); } } } } cout << "Goal node not reachable!" << endl; } int main() { // Example graph represented as a 2D vector vector<vector<int>> graph = { {3, 5, 1, 2}, {1, 3, 2, 4}, {5, 2, 6, 7}, {4, 3, 1, 2} }; Node start; start.x = 0; // Starting x-coordinate start.y = 0; // Starting y-coordinate start.cost = 0; // Cost to reach the starting node Node goal; goal.x = 3; // Goal x-coordinate goal.y = 3; // Goal y-coordinate // Run Greedy Best-First Search algorithm greedyBestFirstSearch(graph, start, goal); return 0; }
輸出
Goal node reached!
說明
這段程式碼包含兩個關鍵元素。首先,它包含 Graph 類別的定義,該類別表示使用鄰接表的圖結構。
其次,它引入了 CompareEuclideanDistance - 一個自訂比較器,用於透過使用歐幾里德距離公式估計節點與目標節點的距離來評估節點。
greedyBestFirstSearch 函數實作貪婪最佳優先搜尋演算法。它使用優先權隊列根據節點的啟發值來儲存節點。
此演算法首先將起始節點放入優先權佇列中。
在每次迭代中,它將最高優先順序的節點出隊並檢查它是否是目標節點。
如果找到目標節點,則會顯示「路徑已找到!」訊息被列印。否則,演算法將出隊的節點標記為已訪問,並將其未訪問的相鄰節點放入佇列。
如果優先權佇列變空而沒有找到目標節點,則會顯示「不存在路徑!」訊息已列印。
main函數透過建立圖表、定義起始節點和目標節點以及呼叫greedyBestFirstSearch函數來演示了演算法的用法。
方法2:基於曼哈頓距離的啟發式函數
我們解決此問題的策略需要使用依賴曼哈頓距離概念的啟發式函數。這種距離度量有時稱為出租車距離,涉及將節點之間的水平和垂直距離相加。
範例
#include <iostream> #include <queue> #include <cmath> #include <vector> #include <unordered_set> using namespace std; // Structure to represent a node in the graph struct Node { int x, y; // Coordinates of the node int cost; // Cost to reach this node }; // Manhattan distance heuristic function int manhattanDistance(int x1, int y1, int x2, int y2) { return abs(x1 - x2) + abs(y1 - y2); } // Custom comparison function for nodes in the priority queue struct NodeCompare { bool operator()(const Node& node1, const Node& node2) const { return node1.cost > node2.cost; } }; // Greedy Best-First Search function void greedyBestFirstSearch(vector<vector<int>>& graph, Node start, Node goal) { int rows = graph.size(); int cols = graph[0].size(); // Priority queue for nodes to be explored priority_queue<Node, vector<Node>, NodeCompare> pq; // Visited nodes set unordered_set<int> visited; // Add the start node to the priority queue pq.push(start); while (!pq.empty()) { // Get the node with the lowest cost Node current = pq.top(); pq.pop(); // Check if the current node is the goal node if (current.x == goal.x && current.y == goal.y) { cout << "Goal node reached!" << endl; return; } // Mark the current node as visited int nodeId = current.x * cols + current.y; visited.insert(nodeId); // Explore the neighboring nodes int dx[] = {-1, 1, 0, 0}; // Possible x-direction movements int dy[] = {0, 0, -1, 1}; // Possible y-direction movements for (int i = 0; i < 4; i++) { int newX = current.x + dx[i]; int newY = current.y + dy[i]; // Check if the neighboring node is within the graph boundaries if (newX >= 0 && newX < rows && newY >= 0 && newY < cols) { // Calculate the heuristic value for the neighboring node int heuristicValue = manhattanDistance(newX, newY, goal.x, goal.y); // Check if the neighboring node has not been visited if (visited.find(newX * cols + newY) == visited.end()) { // Create a new node for the neighboring position Node neighbor; neighbor.x = newX; neighbor.y = newY; neighbor.cost = current.cost + graph[newX][newY]; // Add the neighboring node to the priority queue pq.push(neighbor); } } } } cout << "Goal node not reachable!" << endl; } int main() { // Example graph represented as a 2D vector vector<vector<int>> graph = { {3, 5, 1, 2}, {1, 3, 2, 4}, {5, 2, 6, 7}, {4, 3, 1, 2} }; Node start; start.x = 0; // Starting x-coordinate start.y = 0; // Starting y-coordinate start.cost = 0; // Cost to reach the starting node Node goal; goal.x = 3; // Goal x-coordinate goal.y = 3; // Goal y-coordinate // Run Greedy Best-First Search algorithm greedyBestFirstSearch(graph, start, goal); return 0; }
輸出
Goal node reached!
說明
程式碼遵循與方法 1 類似的結構,但使用自訂比較器 CompareManhattanDistance,該比較器使用曼哈頓距離公式根據到目標節點的估計距離來比較節點。
greedyBestFirstSearch 函數使用曼哈頓距離啟發式實現貪婪最佳優先搜尋演算法。
main函數示範了演算法的使用,建立一個圖,定義起始節點和目標節點,並呼叫greedyBestFirstSearch函數。
結論
在本文中,我們探討了貪婪最佳優先搜尋演算法及其在 C 中的實作。透過採用這些方法,程式設計師可以有效地找到圖中的路徑並解決最佳化問題。啟發式函數的選擇,例如歐氏距離或曼哈頓距離,可以顯著影響演算法在不同場景下的表現。
以上是貪婪最佳優先搜尋演算法(Greedy Best-First Search Algorithm)在C++中的實現的詳細內容。更多資訊請關注PHP中文網其他相關文章!
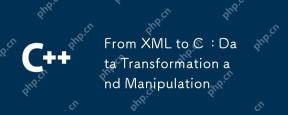
從XML轉換到C 並進行數據操作可以通過以下步驟實現:1)使用tinyxml2庫解析XML文件,2)將數據映射到C 的數據結構中,3)使用C 標準庫如std::vector進行數據操作。通過這些步驟,可以高效地處理和操作從XML轉換過來的數據。
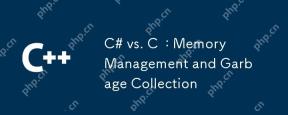
C#使用自動垃圾回收機制,而C 採用手動內存管理。 1.C#的垃圾回收器自動管理內存,減少內存洩漏風險,但可能導致性能下降。 2.C 提供靈活的內存控制,適合需要精細管理的應用,但需謹慎處理以避免內存洩漏。

C 在現代編程中仍然具有重要相關性。 1)高性能和硬件直接操作能力使其在遊戲開發、嵌入式系統和高性能計算等領域佔據首選地位。 2)豐富的編程範式和現代特性如智能指針和模板編程增強了其靈活性和效率,儘管學習曲線陡峭,但其強大功能使其在今天的編程生態中依然重要。
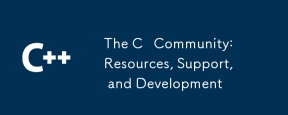
C 學習者和開發者可以從StackOverflow、Reddit的r/cpp社區、Coursera和edX的課程、GitHub上的開源項目、專業諮詢服務以及CppCon等會議中獲得資源和支持。 1.StackOverflow提供技術問題的解答;2.Reddit的r/cpp社區分享最新資訊;3.Coursera和edX提供正式的C 課程;4.GitHub上的開源項目如LLVM和Boost提陞技能;5.專業諮詢服務如JetBrains和Perforce提供技術支持;6.CppCon等會議有助於職業

C#適合需要高開發效率和跨平台支持的項目,而C 適用於需要高性能和底層控制的應用。 1)C#簡化開發,提供垃圾回收和豐富類庫,適合企業級應用。 2)C 允許直接內存操作,適用於遊戲開發和高性能計算。
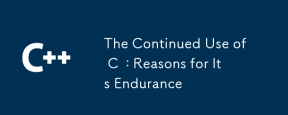
C 持續使用的理由包括其高性能、廣泛應用和不斷演進的特性。 1)高效性能:通過直接操作內存和硬件,C 在系統編程和高性能計算中表現出色。 2)廣泛應用:在遊戲開發、嵌入式系統等領域大放異彩。 3)不斷演進:自1983年發布以來,C 持續增加新特性,保持其競爭力。
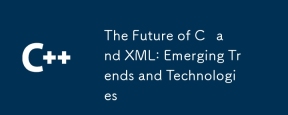
C 和XML的未來發展趨勢分別為:1)C 將通過C 20和C 23標準引入模塊、概念和協程等新特性,提升編程效率和安全性;2)XML將繼續在數據交換和配置文件中佔據重要地位,但會面臨JSON和YAML的挑戰,並朝著更簡潔和易解析的方向發展,如XMLSchema1.1和XPath3.1的改進。
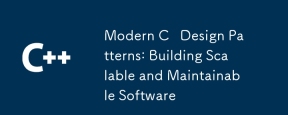
現代C 設計模式利用C 11及以後的新特性實現,幫助構建更靈活、高效的軟件。 1)使用lambda表達式和std::function簡化觀察者模式。 2)通過移動語義和完美轉發優化性能。 3)智能指針確保類型安全和資源管理。


熱AI工具

Undresser.AI Undress
人工智慧驅動的應用程序,用於創建逼真的裸體照片

AI Clothes Remover
用於從照片中去除衣服的線上人工智慧工具。

Undress AI Tool
免費脫衣圖片

Clothoff.io
AI脫衣器

AI Hentai Generator
免費產生 AI 無盡。

熱門文章

熱工具

Atom編輯器mac版下載
最受歡迎的的開源編輯器

Safe Exam Browser
Safe Exam Browser是一個安全的瀏覽器環境,安全地進行線上考試。該軟體將任何電腦變成一個安全的工作站。它控制對任何實用工具的訪問,並防止學生使用未經授權的資源。

禪工作室 13.0.1
強大的PHP整合開發環境

SublimeText3 英文版
推薦:為Win版本,支援程式碼提示!

記事本++7.3.1
好用且免費的程式碼編輯器