Iris,一個多元花卉資料集,是最有用的 Pyhton scikit-learn 資料集之一。它分為 3 類,每類 50 個實例,包含三種鳶尾花(山鳶尾花、維吉尼亞鳶尾和雜色鳶尾)的萼片和花瓣部分的測量值。除此之外,Iris 資料集包含這三個物種中每個物種的 50 個實例,並由四個特徵組成,即 sepal_length (cm)、sepal_width (cm)、petal_length (cm)、petal_width (cm)。
我們可以使用主成分分析(PCA)將 IRIS 資料集轉換為具有 2 個特徵的新特徵空間。
步驟
我們可以按照下面給出的步驟,使用 Python 中的 PCA 將 IRIS 資料集轉換為 2 特徵資料集 -
第 1 步 - 首先,從 scikit-learn 導入必要的套件。我們需要導入資料集和分解包。
步驟 2 - 載入 IRIS 資料集。
步驟 3 - 列印資料集的詳細資訊。
步驟 4 - 初始化主成分分析 (PCA) 並應用 fit() 函數來擬合資料。 p>
步驟 5 - 將資料集轉換為新維度,即 2 個特徵資料集。
範例
在下面的範例中,我們將使用上述步驟透過 PCA 將 scikit-learn IRIS 植物資料集轉換為 2 個特徵。
# Importing the necessary packages from sklearn import datasets from sklearn import decomposition # Load iris plant dataset iris = datasets.load_iris() # Print details about the dataset print('Features names : '+str(iris.feature_names)) print('\n') print('Features size : '+str(iris.data.shape)) print('\n') print('Target names : '+str(iris.target_names)) print('\n') X_iris, Y_iris = iris.data, iris.target # Initialize PCA and fit the data pca_2 = decomposition.PCA(n_components=2) pca_2.fit(X_iris) # Transforming iris data to new dimensions(with 2 features) X_iris_pca2 = pca_2.transform(X_iris) # Printing new dataset print('New Dataset size after transformations: ', X_iris_pca2.shape)
輸出
它將產生以下輸出 -
Features names : ['sepal length (cm)', 'sepal width (cm)', 'petal length (cm)', 'petal width (cm)'] Features size : (150, 4) Target names : ['setosa' 'versicolor' 'virginica'] New Dataset size after transformations: (150, 2)
如何將 Iris 資料集轉換為 3 特徵資料集?
我們可以使用稱為主成分分析(PCA)的統計方法將 Iris 資料集轉換為具有 3 個特徵的新特徵空間。 PCA透過分析原始資料集的特徵,基本上將資料線性投影到新的特徵空間。
PCA 背後的主要概念是選擇資料的「主要」特徵並基於它們建立特徵。它將為我們提供新的資料集,該資料集的大小較小,但具有與原始資料集相同的資訊。
範例
在下面的範例中,我們將使用 PCA 轉換 scikit-learn Iris 植物資料集(以 3 個元件初始化)。
# Importing the necessary packages from sklearn import datasets from sklearn import decomposition # Load iris plant dataset iris = datasets.load_iris() # Print details about the dataset print('Features names : '+str(iris.feature_names)) print('\n') print('Features size : '+str(iris.data.shape)) print('\n') print('Target names : '+str(iris.target_names)) print('\n') print('Target size : '+str(iris.target.shape)) X_iris, Y_iris = iris.data, iris.target # Initialize PCA and fit the data pca_3 = decomposition.PCA(n_components=3) pca_3.fit(X_iris) # Transforming iris data to new dimensions(with 2 features) X_iris_pca3 = pca_3.transform(X_iris) # Printing new dataset print('New Dataset size after transformations : ', X_iris_pca3.shape) print('\n') # Getting the direction of maximum variance in data print("Components : ", pca_3.components_) print('\n') # Getting the amount of variance explained by each component print("Explained Variance:",pca_3.explained_variance_) print('\n') # Getting the percentage of variance explained by each component print("Explained Variance Ratio:",pca_3.explained_variance_ratio_) print('\n') # Getting the singular values for each component print("Singular Values :",pca_3.singular_values_) print('\n') # Getting estimated noise covariance print("Noise Variance :",pca_3.noise_variance_)
輸出
它將產生以下輸出 -
Features names : ['sepal length (cm)', 'sepal width (cm)', 'petal length (cm)', 'petal width (cm)'] Features size : (150, 4) Target names : ['setosa' 'versicolor' 'virginica'] Target size : (150,) New Dataset size after transformations : (150, 3) Components : [[ 0.36138659 -0.08452251 0.85667061 0.3582892 ] [ 0.65658877 0.73016143 -0.17337266 -0.07548102] [-0.58202985 0.59791083 0.07623608 0.54583143]] Explained Variance: [4.22824171 0.24267075 0.0782095 ] Explained Variance Ratio: [0.92461872 0.05306648 0.01710261] Singular Values : [25.09996044 6.01314738 3.41368064] Noise Variance : 0.02383509297344944
以上是如何在Python中將Scikit-learn的IRIS資料集轉換為只有兩個特徵的資料集?的詳細內容。更多資訊請關注PHP中文網其他相關文章!
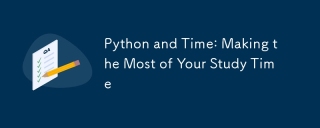
要在有限的時間內最大化學習Python的效率,可以使用Python的datetime、time和schedule模塊。 1.datetime模塊用於記錄和規劃學習時間。 2.time模塊幫助設置學習和休息時間。 3.schedule模塊自動化安排每週學習任務。
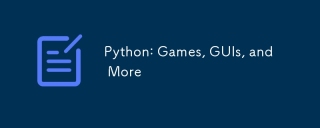
Python在遊戲和GUI開發中表現出色。 1)遊戲開發使用Pygame,提供繪圖、音頻等功能,適合創建2D遊戲。 2)GUI開發可選擇Tkinter或PyQt,Tkinter簡單易用,PyQt功能豐富,適合專業開發。
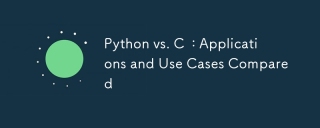
Python适合数据科学、Web开发和自动化任务,而C 适用于系统编程、游戏开发和嵌入式系统。Python以简洁和强大的生态系统著称,C 则以高性能和底层控制能力闻名。
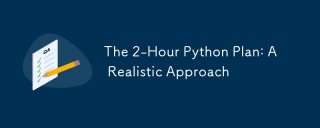
2小時內可以學會Python的基本編程概念和技能。 1.學習變量和數據類型,2.掌握控制流(條件語句和循環),3.理解函數的定義和使用,4.通過簡單示例和代碼片段快速上手Python編程。
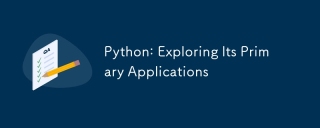
Python在web開發、數據科學、機器學習、自動化和腳本編寫等領域有廣泛應用。 1)在web開發中,Django和Flask框架簡化了開發過程。 2)數據科學和機器學習領域,NumPy、Pandas、Scikit-learn和TensorFlow庫提供了強大支持。 3)自動化和腳本編寫方面,Python適用於自動化測試和系統管理等任務。
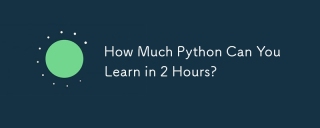
兩小時內可以學到Python的基礎知識。 1.學習變量和數據類型,2.掌握控制結構如if語句和循環,3.了解函數的定義和使用。這些將幫助你開始編寫簡單的Python程序。
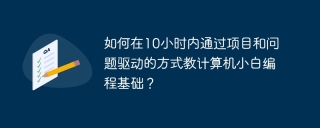
如何在10小時內教計算機小白編程基礎?如果你只有10個小時來教計算機小白一些編程知識,你會選擇教些什麼�...
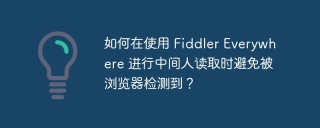
使用FiddlerEverywhere進行中間人讀取時如何避免被檢測到當你使用FiddlerEverywhere...


熱AI工具

Undresser.AI Undress
人工智慧驅動的應用程序,用於創建逼真的裸體照片

AI Clothes Remover
用於從照片中去除衣服的線上人工智慧工具。

Undress AI Tool
免費脫衣圖片

Clothoff.io
AI脫衣器

AI Hentai Generator
免費產生 AI 無盡。

熱門文章

熱工具

SAP NetWeaver Server Adapter for Eclipse
將Eclipse與SAP NetWeaver應用伺服器整合。

Atom編輯器mac版下載
最受歡迎的的開源編輯器
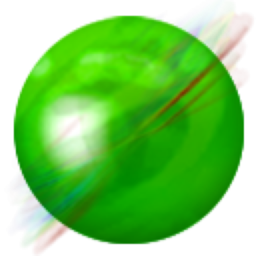
ZendStudio 13.5.1 Mac
強大的PHP整合開發環境
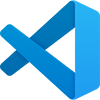
VSCode Windows 64位元 下載
微軟推出的免費、功能強大的一款IDE編輯器

禪工作室 13.0.1
強大的PHP整合開發環境