如何使用C 進行高效率的文字探勘與文字分析?
概述:
文本探勘和文字分析是現代資料分析和機器學習領域中的重要任務。在本文中,我們將介紹如何使用C 語言來進行高效率的文本探勘和文本分析。我們將著重討論文字預處理、特徵提取和文字分類等方面的技術,並配以程式碼範例。
文字預處理:
在進行文字探勘和文字分析之前,通常需要對原始文字進行預處理。預處理包括去除標點符號、停用詞和特殊字符,轉換為小寫字母,並進行詞幹化等操作。以下是使用C 進行文字預處理的範例程式碼:
#include <iostream> #include <string> #include <algorithm> #include <cctype> std::string preprocessText(const std::string& text) { std::string processedText = text; // 去掉标点符号和特殊字符 processedText.erase(std::remove_if(processedText.begin(), processedText.end(), [](char c) { return !std::isalnum(c) && !std::isspace(c); }), processedText.end()); // 转换为小写 std::transform(processedText.begin(), processedText.end(), processedText.begin(), [](unsigned char c) { return std::tolower(c); }); // 进行词干化等其他操作 return processedText; } int main() { std::string text = "Hello, World! This is a sample text."; std::string processedText = preprocessText(text); std::cout << processedText << std::endl; return 0; }
特徵提取:
在進行文字分析任務時,需要將文字轉換為數值特徵向量,以便機器學習演算法能夠處理。常用的特徵提取方法包括詞袋模型和TF-IDF。以下是一個使用C 進行詞袋模型和TF-IDF特徵提取的範例程式碼:
#include <iostream> #include <string> #include <vector> #include <map> #include <algorithm> std::vector<std::string> extractWords(const std::string& text) { std::vector<std::string> words; // 通过空格分割字符串 std::stringstream ss(text); std::string word; while (ss >> word) { words.push_back(word); } return words; } std::map<std::string, int> createWordCount(const std::vector<std::string>& words) { std::map<std::string, int> wordCount; for (const std::string& word : words) { wordCount[word]++; } return wordCount; } std::map<std::string, double> calculateTFIDF(const std::vector<std::map<std::string, int>>& documentWordCounts, const std::map<std::string, int>& wordCount) { std::map<std::string, double> tfidf; int numDocuments = documentWordCounts.size(); for (const auto& wordEntry : wordCount) { const std::string& word = wordEntry.first; int wordDocumentCount = 0; // 统计包含该词的文档数 for (const auto& documentWordCount : documentWordCounts) { if (documentWordCount.count(word) > 0) { wordDocumentCount++; } } // 计算TF-IDF值 double tf = static_cast<double>(wordEntry.second) / wordCount.size(); double idf = std::log(static_cast<double>(numDocuments) / (wordDocumentCount + 1)); double tfidfValue = tf * idf; tfidf[word] = tfidfValue; } return tfidf; } int main() { std::string text1 = "Hello, World! This is a sample text."; std::string text2 = "Another sample text."; std::vector<std::string> words1 = extractWords(text1); std::vector<std::string> words2 = extractWords(text2); std::map<std::string, int> wordCount1 = createWordCount(words1); std::map<std::string, int> wordCount2 = createWordCount(words2); std::vector<std::map<std::string, int>> documentWordCounts = {wordCount1, wordCount2}; std::map<std::string, double> tfidf1 = calculateTFIDF(documentWordCounts, wordCount1); std::map<std::string, double> tfidf2 = calculateTFIDF(documentWordCounts, wordCount2); // 打印TF-IDF特征向量 for (const auto& tfidfEntry : tfidf1) { std::cout << tfidfEntry.first << ": " << tfidfEntry.second << std::endl; } return 0; }
文字分類:
文字分類是一項常見的文本探勘任務,它將文本分為不同的類別。常用的文字分類演算法包括樸素貝葉斯分類器和支援向量機(SVM)。以下是一個使用C 進行文字分類的範例程式碼:
#include <iostream> #include <string> #include <vector> #include <map> #include <cmath> std::map<std::string, double> trainNaiveBayes(const std::vector<std::map<std::string, int>>& documentWordCounts, const std::vector<int>& labels) { std::map<std::string, double> classPriors; std::map<std::string, std::map<std::string, double>> featureProbabilities; int numDocuments = documentWordCounts.size(); int numFeatures = documentWordCounts[0].size(); std::vector<int> classCounts(numFeatures, 0); // 统计每个类别的先验概率和特征的条件概率 for (int i = 0; i < numDocuments; i++) { std::string label = std::to_string(labels[i]); classCounts[labels[i]]++; for (const auto& wordCount : documentWordCounts[i]) { const std::string& word = wordCount.first; featureProbabilities[label][word] += wordCount.second; } } // 计算每个类别的先验概率 for (int i = 0; i < numFeatures; i++) { double classPrior = static_cast<double>(classCounts[i]) / numDocuments; classPriors[std::to_string(i)] = classPrior; } // 计算每个特征的条件概率 for (auto& classEntry : featureProbabilities) { std::string label = classEntry.first; std::map<std::string, double>& wordProbabilities = classEntry.second; double totalWords = 0.0; for (auto& wordEntry : wordProbabilities) { totalWords += wordEntry.second; } for (auto& wordEntry : wordProbabilities) { std::string& word = wordEntry.first; double& wordCount = wordEntry.second; wordCount = (wordCount + 1) / (totalWords + numFeatures); // 拉普拉斯平滑 } } return classPriors; } int predictNaiveBayes(const std::string& text, const std::map<std::string, double>& classPriors, const std::map<std::string, std::map<std::string, double>>& featureProbabilities) { std::vector<std::string> words = extractWords(text); std::map<std::string, int> wordCount = createWordCount(words); std::map<std::string, double> logProbabilities; // 计算每个类别的对数概率 for (const auto& classEntry : classPriors) { std::string label = classEntry.first; double classPrior = classEntry.second; double logProbability = std::log(classPrior); for (const auto& wordEntry : wordCount) { const std::string& word = wordEntry.first; int wordCount = wordEntry.second; if (featureProbabilities.count(label) > 0 && featureProbabilities.at(label).count(word) > 0) { const std::map<std::string, double>& wordProbabilities = featureProbabilities.at(label); logProbability += std::log(wordProbabilities.at(word)) * wordCount; } } logProbabilities[label] = logProbability; } // 返回概率最大的类别作为预测结果 int predictedLabel = 0; double maxLogProbability = -std::numeric_limits<double>::infinity(); for (const auto& logProbabilityEntry : logProbabilities) { std::string label = logProbabilityEntry.first; double logProbability = logProbabilityEntry.second; if (logProbability > maxLogProbability) { maxLogProbability = logProbability; predictedLabel = std::stoi(label); } } return predictedLabel; } int main() { std::vector<std::string> documents = { "This is a positive document.", "This is a negative document." }; std::vector<int> labels = { 1, 0 }; std::vector<std::map<std::string, int>> documentWordCounts; for (const std::string& document : documents) { std::vector<std::string> words = extractWords(document); std::map<std::string, int> wordCount = createWordCount(words); documentWordCounts.push_back(wordCount); } std::map<std::string, double> classPriors = trainNaiveBayes(documentWordCounts, labels); int predictedLabel = predictNaiveBayes("This is a positive test document.", classPriors, featureProbabilities); std::cout << "Predicted Label: " << predictedLabel << std::endl; return 0; }
總結:
本文介紹如何使用C 進行高效率的文字探勘和文字分析,包括文字預處理、特徵提取和文字分類。我們透過程式碼範例展示如何實現這些功能,希望對你在實際應用中有所幫助。透過這些技術和工具,你可以更有效率地處理和分析大量的文字資料。
以上是如何使用C++進行高效率的文本探勘與文字分析?的詳細內容。更多資訊請關注PHP中文網其他相關文章!
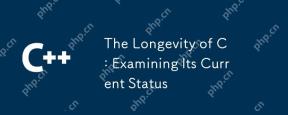
C 在現代編程中依然重要,因其高效、靈活和強大的特性。 1)C 支持面向對象編程,適用於系統編程、遊戲開發和嵌入式系統。 2)多態性是C 的亮點,允許通過基類指針或引用調用派生類方法,增強代碼的靈活性和可擴展性。
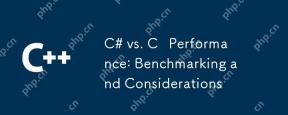
C#和C 在性能上的差異主要體現在執行速度和資源管理上:1)C 在數值計算和字符串操作上通常表現更好,因為它更接近硬件,沒有垃圾回收等額外開銷;2)C#在多線程編程上更為簡潔,但性能略遜於C ;3)選擇哪種語言應根據項目需求和團隊技術棧決定。
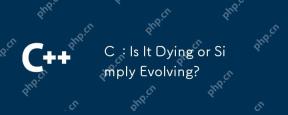
1)c relevantduetoItsAverity and效率和效果臨界。 2)theLanguageIsconTinuellyUped,withc 20introducingFeaturesFeaturesLikeTuresLikeSlikeModeLeslikeMeSandIntIneStoImproutiMimproutimprouteverusabilityandperformance.3)
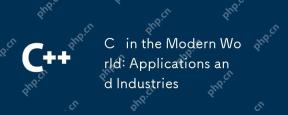
C 在現代世界中的應用廣泛且重要。 1)在遊戲開發中,C 因其高性能和多態性被廣泛使用,如UnrealEngine和Unity。 2)在金融交易系統中,C 的低延遲和高吞吐量使其成為首選,適用於高頻交易和實時數據分析。
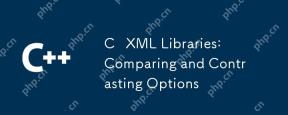
C 中有四種常用的XML庫:TinyXML-2、PugiXML、Xerces-C 和RapidXML。 1.TinyXML-2適合資源有限的環境,輕量但功能有限。 2.PugiXML快速且支持XPath查詢,適用於復雜XML結構。 3.Xerces-C 功能強大,支持DOM和SAX解析,適用於復雜處理。 4.RapidXML專注於性能,解析速度極快,但不支持XPath查詢。
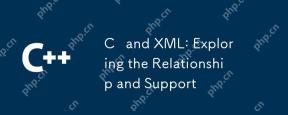
C 通過第三方庫(如TinyXML、Pugixml、Xerces-C )與XML交互。 1)使用庫解析XML文件,將其轉換為C 可處理的數據結構。 2)生成XML時,將C 數據結構轉換為XML格式。 3)在實際應用中,XML常用於配置文件和數據交換,提升開發效率。
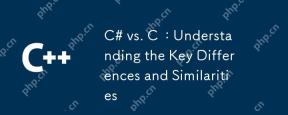
C#和C 的主要區別在於語法、性能和應用場景。 1)C#語法更簡潔,支持垃圾回收,適用於.NET框架開發。 2)C 性能更高,需手動管理內存,常用於系統編程和遊戲開發。
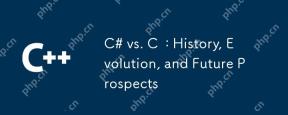
C#和C 的歷史與演變各有特色,未來前景也不同。 1.C 由BjarneStroustrup在1983年發明,旨在將面向對象編程引入C語言,其演變歷程包括多次標準化,如C 11引入auto關鍵字和lambda表達式,C 20引入概念和協程,未來將專注於性能和系統級編程。 2.C#由微軟在2000年發布,結合C 和Java的優點,其演變注重簡潔性和生產力,如C#2.0引入泛型,C#5.0引入異步編程,未來將專注於開發者的生產力和雲計算。


熱AI工具

Undresser.AI Undress
人工智慧驅動的應用程序,用於創建逼真的裸體照片

AI Clothes Remover
用於從照片中去除衣服的線上人工智慧工具。

Undress AI Tool
免費脫衣圖片

Clothoff.io
AI脫衣器

Video Face Swap
使用我們完全免費的人工智慧換臉工具,輕鬆在任何影片中換臉!

熱門文章

熱工具

DVWA
Damn Vulnerable Web App (DVWA) 是一個PHP/MySQL的Web應用程序,非常容易受到攻擊。它的主要目標是成為安全專業人員在合法環境中測試自己的技能和工具的輔助工具,幫助Web開發人員更好地理解保護網路應用程式的過程,並幫助教師/學生在課堂環境中教授/學習Web應用程式安全性。 DVWA的目標是透過簡單直接的介面練習一些最常見的Web漏洞,難度各不相同。請注意,該軟體中
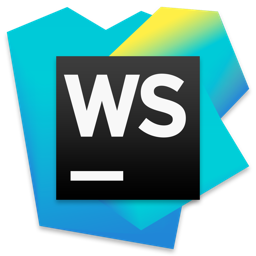
WebStorm Mac版
好用的JavaScript開發工具

Atom編輯器mac版下載
最受歡迎的的開源編輯器
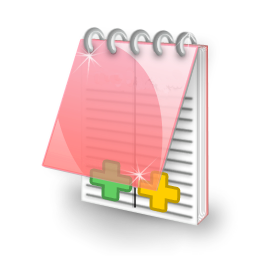
EditPlus 中文破解版
體積小,語法高亮,不支援程式碼提示功能

MinGW - Minimalist GNU for Windows
這個專案正在遷移到osdn.net/projects/mingw的過程中,你可以繼續在那裡關注我們。 MinGW:GNU編譯器集合(GCC)的本機Windows移植版本,可自由分發的導入函式庫和用於建置本機Windows應用程式的頭檔;包括對MSVC執行時間的擴展,以支援C99功能。 MinGW的所有軟體都可以在64位元Windows平台上運作。