使用Python數組比列表更適合處理大量數值數據。 1)數組更節省內存,2)數組對數值運算更快,3)數組強制類型一致性,4)數組與C語言數組兼容,但在靈活性和便捷性上不如列表。
When it comes to choosing between a Python list and an array, understanding the nuances can significantly impact the performance and efficiency of your code. Let's dive into a scenario where using a Python array from the array
module would be more appropriate than using a list.
Imagine you're working on a project that involves processing large amounts of numerical data, such as a financial application that needs to handle stock prices or a scientific computing task dealing with sensor data. In such cases, using a Python array can offer substantial benefits over a list.
Here's a detailed exploration of why and how to use arrays effectively in this context:
In the world of Python, lists are incredibly versatile and easy to use. They can store elements of different types, grow or shrink dynamically, and are generally the go-to choice for many programming tasks. But what if you're dealing with a specific kind of data, like numbers, and performance matters a lot?
Let's say you're developing a financial application that processes millions of stock prices. Each stock price is a floating-point number, and you need to perform calculations on these numbers quickly. Here's where the array
module comes into play.
The array
module provides an array
object that is more memory-efficient and faster for numerical operations compared to a list. Unlike lists, which can contain elements of any type, arrays are typed, meaning they can only store elements of a single type. This restriction allows for more efficient memory usage and faster access times.
Here's a simple example to illustrate the difference:
import array import sys # Using a list to store numbers numbers_list = [1.0, 2.0, 3.0, 4.0, 5.0] print(f"Size of list: {sys.getsizeof(numbers_list)} bytes") # Using an array to store numbers numbers_array = array.array('d', [1.0, 2.0, 3.0, 4.0, 5.0]) print(f"Size of array: {sys.getsizeof(numbers_array)} bytes")
When you run this code, you'll notice that the array takes up less memory than the list. This difference becomes even more significant as the size of the data increases.
Now, let's consider a more practical scenario in our financial application:
import array import time # Simulating a large dataset of stock prices stock_prices_list = [float(i) for i in range(1000000)] stock_prices_array = array.array('d', [float(i) for i in range(1000000)]) # Measuring time to sum up all prices using a list start_time = time.time() total_list = sum(stock_prices_list) list_time = time.time() - start_time # Measuring time to sum up all prices using an array start_time = time.time() total_array = sum(stock_prices_array) array_time = time.time() - start_time print(f"Sum using list: {total_list}, Time: {list_time:.6f} seconds") print(f"Sum using array: {total_array}, Time: {array_time:.6f} seconds")
In this example, you'll likely see that the array performs the summation faster than the list, especially as the size of the dataset grows. This is because arrays are more optimized for numerical operations.
But it's not just about performance. Here are some additional considerations:
- Memory Efficiency : Arrays use less memory than lists for storing numerical data, which is crucial when dealing with large datasets.
- Type Safety : Arrays enforce type consistency, which can prevent bugs that might occur if you accidentally mix data types in a list.
- Interoperability : Arrays can be easily converted to and from C arrays, making them useful when interfacing with C libraries or when you need to optimize certain parts of your code.
However, there are some potential pitfalls to watch out for:
- Limited Flexibility : Since arrays are typed, you can't mix different types of data within the same array. This might limit their use in more general-purpose scenarios.
- Less Convenient : Arrays don't support some of the convenient methods that lists do, like
append
orinsert
. You'll need to useextend
to add elements, which can be less intuitive.
In practice, I've found that the choice between lists and arrays often comes down to the specific needs of your project. For general-purpose programming, lists are usually the better choice due to their flexibility. But when you're dealing with large datasets of numerical data and performance is critical, arrays can be a game-changer.
To wrap up, if you're working on a project that involves processing millions of numbers quickly and efficiently, consider using a Python array from the array
module. It might just be the edge you need to optimize your code and make your application run faster and more smoothly.
以上是舉一個場景的示例,其中使用Python數組比使用列表更合適。的詳細內容。更多資訊請關注PHP中文網其他相關文章!
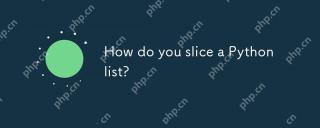
SlicingaPythonlistisdoneusingthesyntaxlist[start:stop:step].Here'showitworks:1)Startistheindexofthefirstelementtoinclude.2)Stopistheindexofthefirstelementtoexclude.3)Stepistheincrementbetweenelements.It'susefulforextractingportionsoflistsandcanuseneg
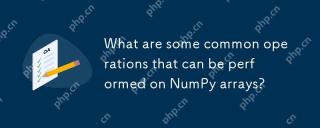
numpyallowsforvariousoperationsonArrays:1)basicarithmeticlikeaddition,減法,乘法和division; 2)evationAperationssuchasmatrixmultiplication; 3)element-wiseOperations wiseOperationswithOutexpliitloops; 4)
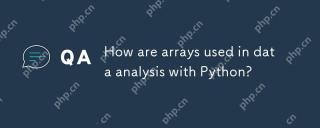
Arresinpython,尤其是Throughnumpyandpandas,weessentialFordataAnalysis,offeringSpeedAndeffied.1)NumpyArseNable efflaysenable efficefliceHandlingAtaSetSetSetSetSetSetSetSetSetSetSetsetSetSetSetSetsopplexoperationslikemovingaverages.2)
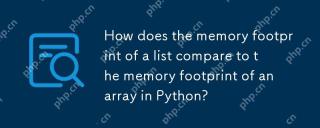
列表sandnumpyArraysInpythonHavedIfferentMemoryfootprints:listSaremoreFlexibleButlessMemory-效率,而alenumpyArraySareSareOptimizedFornumericalData.1)listsStorReereReereReereReereFerenceStoObjects,with withOverHeadeBheadaroundAroundaround64byty64-bitsysysysysysysysysyssyssyssyssysssyssys2)
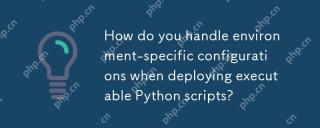
toensurepythonscriptsbehavecorrectlyacrycrosdevelvermations,分期和生產,USETHESTERTATE:1)Environment varriablesForsimplesettings,2)configurationfilesfilesForcomPlexSetups,3)dynamiCofforComplexSetups,dynamiqualloadingForaptaptibality.eachmethodoffersuniquebeneiquebeneqeniquebenefitsandrefitsandrequiresandrequiresandrequiresca
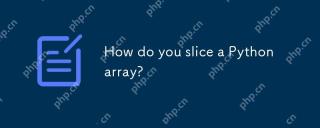
Python列表切片的基本語法是list[start:stop:step]。 1.start是包含的第一個元素索引,2.stop是排除的第一個元素索引,3.step決定元素之間的步長。切片不僅用於提取數據,還可以修改和反轉列表。
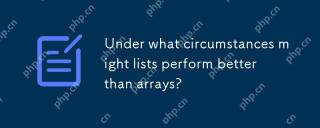
ListSoutPerformarRaysin:1)DynamicsizicsizingandFrequentInsertions/刪除,2)儲存的二聚體和3)MemoryFeliceFiceForceforseforsparsedata,butmayhaveslightperformancecostsinclentoperations。
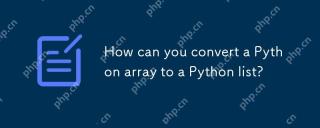
toConvertapythonarraytoalist,usEthelist()constructororageneratorexpression.1)intimpthearraymoduleandcreateanArray.2)USELIST(ARR)或[XFORXINARR] to ConconverTittoalist,請考慮performorefformanceandmemoryfformanceandmemoryfformienceforlargedAtasetset。


熱AI工具

Undresser.AI Undress
人工智慧驅動的應用程序,用於創建逼真的裸體照片

AI Clothes Remover
用於從照片中去除衣服的線上人工智慧工具。

Undress AI Tool
免費脫衣圖片

Clothoff.io
AI脫衣器

Video Face Swap
使用我們完全免費的人工智慧換臉工具,輕鬆在任何影片中換臉!

熱門文章

熱工具

SecLists
SecLists是最終安全測試人員的伙伴。它是一個包含各種類型清單的集合,這些清單在安全評估過程中經常使用,而且都在一個地方。 SecLists透過方便地提供安全測試人員可能需要的所有列表,幫助提高安全測試的效率和生產力。清單類型包括使用者名稱、密碼、URL、模糊測試有效載荷、敏感資料模式、Web shell等等。測試人員只需將此儲存庫拉到新的測試機上,他就可以存取所需的每種類型的清單。

記事本++7.3.1
好用且免費的程式碼編輯器

DVWA
Damn Vulnerable Web App (DVWA) 是一個PHP/MySQL的Web應用程序,非常容易受到攻擊。它的主要目標是成為安全專業人員在合法環境中測試自己的技能和工具的輔助工具,幫助Web開發人員更好地理解保護網路應用程式的過程,並幫助教師/學生在課堂環境中教授/學習Web應用程式安全性。 DVWA的目標是透過簡單直接的介面練習一些最常見的Web漏洞,難度各不相同。請注意,該軟體中
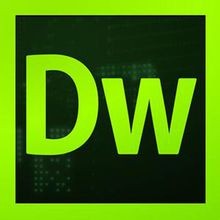
Dreamweaver CS6
視覺化網頁開發工具

MinGW - Minimalist GNU for Windows
這個專案正在遷移到osdn.net/projects/mingw的過程中,你可以繼續在那裡關注我們。 MinGW:GNU編譯器集合(GCC)的本機Windows移植版本,可自由分發的導入函式庫和用於建置本機Windows應用程式的頭檔;包括對MSVC執行時間的擴展,以支援C99功能。 MinGW的所有軟體都可以在64位元Windows平台上運作。