AI agents are designed to act autonomously, solving problems and executing tasks in dynamic environments. A key feature in Autogen, enabling their adaptability is AutoGen’s code executors. This feature along with LLMs enables AI agents to generate, evaluate, and execute code in real-time. This capability bridges the gap between static AI models and actionable intelligence. By automating workflows, performing data analysis, and debugging complex systems, it transforms agents from mere thinkers into effective doers. In this article, we will learn more about code executors in AutoGen and how to implement them.
Table of Contents
- Types of Code Executors in AutoGen
- How to Build AI Agents with Code Executors in AutoGen?
- Pre-requisites
- Building an AI Agent Using Command Line Executor
- Building an ML Model Using Jupyter Code Executor
- Building an AI Agent Using Custom Executor
- Conclusion
- Frequently Asked Questions
Types of Code Executors in AutoGen
AutoGen has three kinds of code executors that can be used for different purposes.
- Command Line Executor: It allows AI agents to run the code in the command line. It will save each code block to a separate file and execute that file. This executor is ideal for automating tasks like file management, script execution, or handling external tools. It provides flexibility and low-level control in a workflow.
- Jupyter Code Executor: It enables agents to execute Python code within a Jupyter-like environment. Here, you can define variables in one code block and reuse them in subsequent blocks. One advantage of this setup is that when an error occurs, only the specific block of code with the error needs to be re-executed, rather than the entire script.
- Custom Code Executor: It gives developers the ability to create specialized code execution logic. For example, the custom code executor can access variables defined in the environment without explicitly providing them to the LLM.
These Code Executors can be run on both the host machine (local) as well as the Docker containers.
Also Read: 4 Steps to Build Multi-Agent Nested Chats with AutoGen
How to Build AI Agents with Code Executors in AutoGen?
Now let’s learn how you can use these different code executors in AutoGen:
Pre-requisites
Before building AI agents, ensure you have the necessary API keys for the required LLMs.
Load the .env file with the API keys needed.
from dotenv import load_dotenv load_dotenv(./env)
Key Libraries Required
autogen-agentchat – 0.2.38
jupyter_kernel_gateway-3.0.1
Building an AI Agent Using Command Line Executor
Let’s build an AI agent to know the offers and discounts available on an e-commerce website using the command line executor. Here are the steps to follow.
1. Import the necessary libraries.
from autogen import ConversableAgent, AssistantAgent, UserProxyAgent from autogen.coding import LocalCommandLineCodeExecutor, DockerCommandLineCodeExecutor
2. Define the agents.
user_proxy = UserProxyAgent( name="User", llm_config=False, is_termination_msg=lambda msg: msg.get("content") is not None and "TERMINATE" in msg["content"], human_input_mode="TERMINATE", code_execution_config=False ) code_writer_agent = ConversableAgent( name="CodeWriter", system_message="""You are a Python developer. You use your coding skill to solve problems. Once the task is done, returns 'TERMINATE'.""", llm_config={"config_list": [{"model": "gpt-4o-mini"}]}, ) local_executor = LocalCommandLineCodeExecutor( timeout=15, work_dir='./code files') local_executor_agent = ConversableAgent( "local_executor_agent", llm_config=False, code_execution_config={"executor": local_executor}, human_input_mode="ALWAYS", )
We are using the ‘local_executor’ in the code_execution_config of the local_executor_agent.
3. Define the messages which are used to initialize the chat.
messages = ["""To check whether there are any offers or discounts available on a given e-commerce website - https://www.flipkart.com/ Follow these steps, 1. download the html page of the given URL 2. we only need html content, so remove any CSS, JavaScript, and Image tags content 3. save the remaining html content. """ , "read the text and list all the offers and discounts available"] # Intialize the chat chat_result = local_executor_agent.initiate_chat( code_writer_agent, message=messages[0], )
It will ask for human input after each message from the codeWriter agent. You just need to press the ‘Enter’ key to execute the code written by the agent. We can also any further instructions if there is any problem with the code.
Here are the questions we have asked and the output at the end.
As we can see, with the mentioned questions, we can get a list of offers and discounts from an e-commerce website.
Also Read: Hands-on Guide to Building Multi-Agent Chatbots with AutoGen
Building an ML Model Using Jupyter Code Executor
By using this, we can access the variables defined in one code block from another code block, unlike the command line executor.
Now, let’s try to build an ML model using this.
1. Import the additional methods.
from autogen.coding.jupyter import LocalJupyterServer, DockerJupyterServer, JupyterCodeExecutor from pathlib import Path
2. Initialize the jupyter server and output directory.
server = LocalJupyterServer() output_dir = Path("coding") output_dir.mkdir()
Note that LocalJupyterServer may not function on Windows due to a bug. In this case, you can use the DockerJupyterServer instead or use the EmbeddedIPythonCodeExecutor.
3. Define the executor agent and writer agent with a custom system message.
jupyter_executor_agent = ConversableAgent( name="jupyter_executor_agent", llm_config=False, code_execution_config={ "executor": JupyterCodeExecutor(server, output_dir=output_dir), }, human_input_mode="ALWAYS", ) code_writer_system_message = """ You have been given coding capability to solve tasks using Python code in a stateful IPython kernel. You are responsible for writing the code, and the user is responsible for executing the code. When you write Python code, put the code in a markdown code block with the language set to Python. For example: ```python x = 3 ``` You can use the variable `x` in subsequent code blocks. ```python print(x) ``` Always use print statements for the output of the code. Write code incrementally and leverage the statefulness of the kernel to avoid repeating code. Import libraries in a separate code block. Define a function or a class in a separate code block. Run code that produces output in a separate code block. Run code that involves expensive operations like download, upload, and call external APIs in a separate code block. When your code produces an output, the output will be returned to you. Because you have limited conversation memory, if your code creates an image, the output will be a path to the image instead of the image itself.""" code_writer_agent = ConversableAgent( "code_writer", system_message=code_writer_system_message, llm_config={"config_list": [{"model": "gpt-4o"}]}, human_input_mode="TERMINATE", )
4. Define the initial message and initialize the chat
message = "read the datasets/user_behavior_dataset.csv and print what the data is about" chat_result = jupyter_executor_agent.initiate_chat( code_writer_agent, message=message, ) # Once the chat is completed we can stop the server. server.stop()
5. Once the chat is completed we can stop the server.
We can print the messages as follows
for chat in chat_result.chat_history[:]: if chat['name'] == 'code_writer' and 'TERMINATE' not in chat['content']: print("--------agent-----------") print(chat['content']) if chat['name'] == 'jupyter_executor_agent' and 'exitcode' not in chat['content']: print("--------user------------") print(chat['content'])
Here’s the sample
As we can see, we can get the code generated by the agent and also the results after executing the code.
Also Read: Building Agentic Chatbots Using AutoGen
Building an AI Agent Using Custom Executor
Now, let’s try to create a custom executor that can run the code in the same jupyter notebook where we are creating this executor. So, we can read a CSV file, and then ask an agent to build an ML model on the already imported file.
Here’s how we’ll do it.
1. Import the necessary libraries.
import pandas as pd from typing import List from IPython import get_ipython from autogen.coding import CodeBlock, CodeExecutor, CodeExtractor, CodeResult, MarkdownCodeExtractor
2. Define the executor that can extract and run the code from jupyter cells.
class NotebookExecutor(CodeExecutor): @property def code_extractor(self) -> CodeExtractor: # Extact code from markdown blocks. return MarkdownCodeExtractor() def __init__(self) -> None: # Get the current IPython instance running in this notebook. self._ipython = get_ipython() def execute_code_blocks(self, code_blocks: List[CodeBlock]) -> CodeResult: log = "" for code_block in code_blocks: result = self._ipython.run_cell("%%capture --no-display cap\n" + code_block.code) log += self._ipython.ev("cap.stdout") log += self._ipython.ev("cap.stderr") if result.result is not None: log += str(result.result) exitcode = 0 if result.success else 1 if result.error_before_exec is not None: log += f"\n{result.error_before_exec}" exitcode = 1 if result.error_in_exec is not None: log += f"\n{result.error_in_exec}" exitcode = 1 if exitcode != 0: break return CodeResult(exit_code=exitcode, output=log)
3. Define the agents.
code_writer_agent = ConversableAgent( name="CodeWriter", system_message="You are a helpful AI assistant.\n" "You use your coding skill to solve problems.\n" "You have access to a IPython kernel to execute Python code.\n" "You can suggest Python code in Markdown blocks, each block is a cell.\n" "The code blocks will be executed in the IPython kernel in the order you suggest them.\n" "All necessary libraries have already been installed.\n" "Add return or print statements to the code to get the output\n" "Once the task is done, returns 'TERMINATE'.", llm_config={"config_list": [{"model": "gpt-4o-mini"}]}, ) code_executor_agent = ConversableAgent( name="CodeExecutor", llm_config=False, code_execution_config={"executor": NotebookExecutor()}, is_termination_msg=lambda msg: "TERMINATE" in msg.get("content", "").strip().upper(), human_input_mode="ALWAYS" )
4. Read the file and initiate the chat with the file.
df = pd.read_csv('datasets/mountains_vs_beaches_preferences.csv') chat_result = code_executor_agent.initiate_chat( code_writer_agent, message="What are the column names in the dataframe defined above as df?", )
5. We can print the chat history as follows:
for chat in chat_result.chat_history[:]: if chat['name'] == 'CodeWriter' and 'TERMINATE' not in chat['content']: print("--------agent-----------") print(chat['content']) if chat['name'] == 'CodeExecutor' and 'exitcode' not in chat['content']: print("--------user------------") print(chat['content'])
As we can see again, we can get the code generated by the agent and also the results after executing the code.
Conclusion
AutoGen’s code executors provide flexibility and functionality for AI agents to perform real-world tasks. The command line executor enables script execution, while the Jupyter code executor supports iterative development. Custom executors, on the other hand, allow developers to create tailored workflows.
These tools empower AI agents to transition from problem solvers to solution implementers. Developers can use these features to build intelligent systems that deliver actionable insights and automate complex processes.
Frequently Asked Questions
Q1. What is the primary purpose of Code Executors in AutoGen?A. Code Executors in AutoGen allow AI agents to generate, execute, and evaluate code in real time. This enables agents to automate tasks, perform data analysis, debug systems, and implement dynamic workflows.
Q2. What are the differences between Command Line and Jupyter Code Executors?A. The Command Line Executor saves and executes code as separate files, ideal for tasks like file management and script execution. The Jupyter Code Executor operates in a stateful environment, allowing reuse of variables and selective re-execution of code blocks, making it more suitable for iterative coding tasks like building ML models.
Q3. Can Code Executors be used with Docker containers?A. Yes, both the Command Line Executor and Jupyter Code Executor can be configured to run on Docker containers, providing a flexible environment for execution.
Q4. What is the advantage of using a Custom Code Executor?A. Custom Code Executors allow developers to define specialized execution logic, such as running code within the same Jupyter notebook. This is useful for tasks requiring a high level of integration or customization.
Q5. What are the prerequisites for using Code Executors in AutoGen?A. Before using Code Executors, ensure you have the necessary API keys for your preferred LLMs. You should also have the required libraries, such as `autogen-agentchat` and `jupyter_kernel_gateway`, installed in your environment.
以上是使用Autogen中的代碼執行人完成複雜的任務的詳細內容。更多資訊請關注PHP中文網其他相關文章!
![無法使用chatgpt!解釋可以立即測試的原因和解決方案[最新2025]](https://img.php.cn/upload/article/001/242/473/174717025174979.jpg?x-oss-process=image/resize,p_40)
ChatGPT無法訪問?本文提供多種實用解決方案!許多用戶在日常使用ChatGPT時,可能會遇到無法訪問或響應緩慢等問題。本文將根據不同情況,逐步指導您解決這些問題。 ChatGPT無法訪問的原因及初步排查 首先,我們需要確定問題是出在OpenAI服務器端,還是用戶自身網絡或設備問題。 請按照以下步驟進行排查: 步驟1:檢查OpenAI官方狀態 訪問OpenAI Status頁面 (status.openai.com),查看ChatGPT服務是否正常運行。如果顯示紅色或黃色警報,則表示Open
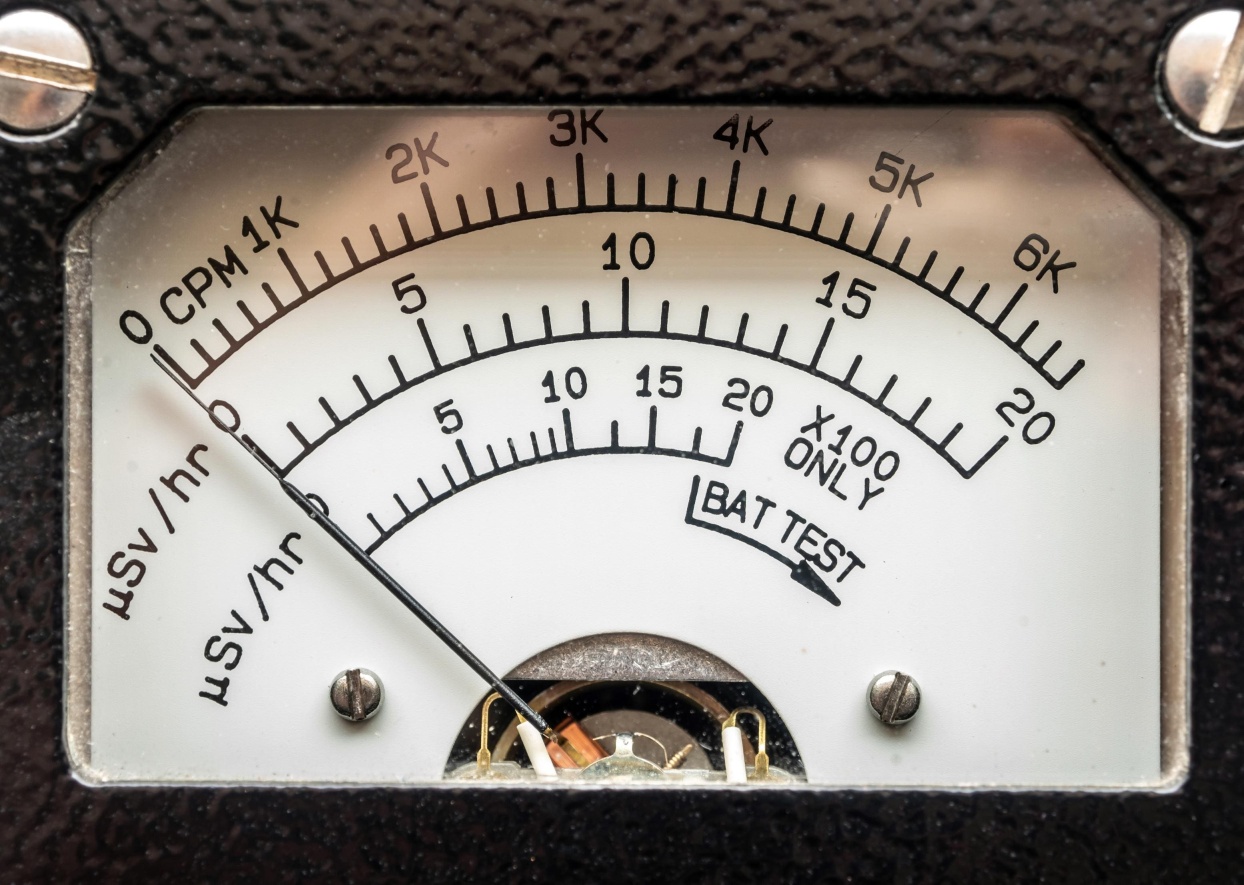
2025年5月10日,麻省理工學院物理學家Max Tegmark告訴《衛報》,AI實驗室應在釋放人工超級智能之前模仿Oppenheimer的三位一體測試演算。 “我的評估是'康普頓常數',這是一場比賽的可能性
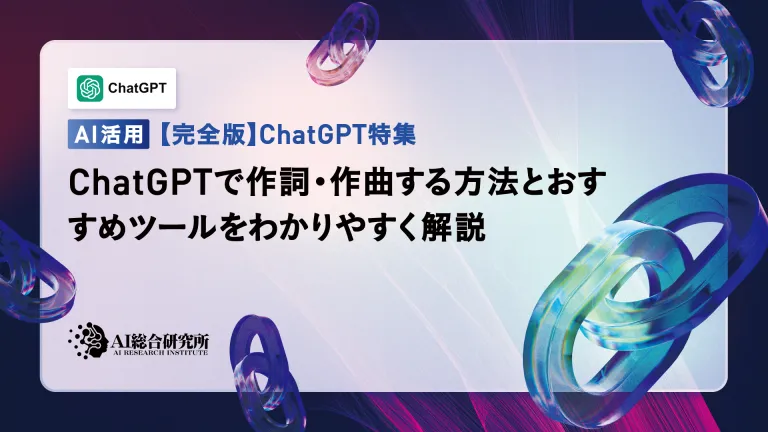
AI音樂創作技術日新月異,本文將以ChatGPT等AI模型為例,詳細講解如何利用AI輔助音樂創作,並輔以實際案例進行說明。我們將分別介紹如何通過SunoAI、Hugging Face上的AI jukebox以及Python的Music21庫進行音樂創作。 通過這些技術,每個人都能輕鬆創作原創音樂。但需注意,AI生成內容的版權問題不容忽視,使用時務必謹慎。 讓我們一起探索AI在音樂領域的無限可能! OpenAI最新AI代理“OpenAI Deep Research”介紹: [ChatGPT]Ope
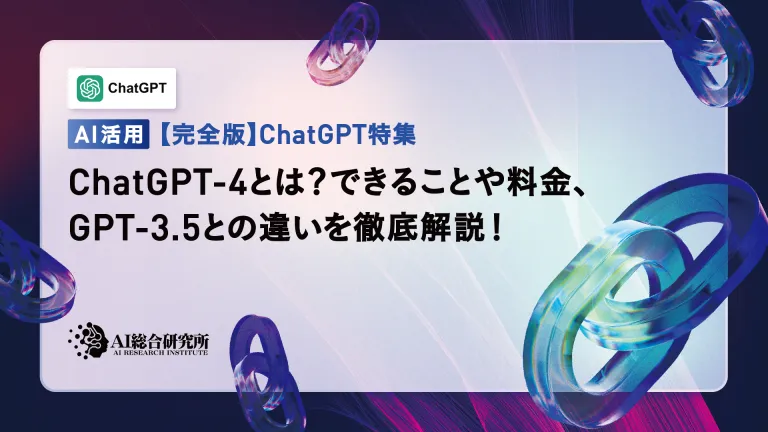
ChatGPT-4的出现,极大地拓展了AI应用的可能性。相较于GPT-3.5,ChatGPT-4有了显著提升,它具备强大的语境理解能力,还能识别和生成图像,堪称万能的AI助手。在提高商业效率、辅助创作等诸多领域,它都展现出巨大的潜力。然而,与此同时,我们也必须注意其使用上的注意事项。 本文将详细解读ChatGPT-4的特性,并介绍针对不同场景的有效使用方法。文中包含充分利用最新AI技术的技巧,敬请参考。 OpenAI发布的最新AI代理,“OpenAI Deep Research”详情请点击下方链
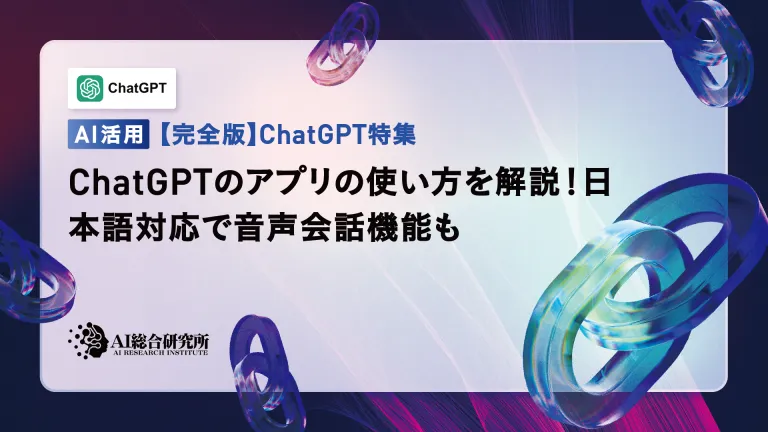
CHATGPT應用程序:與AI助手釋放您的創造力!初學者指南 ChatGpt應用程序是一位創新的AI助手,可處理各種任務,包括寫作,翻譯和答案。它是一種具有無限可能性的工具,可用於創意活動和信息收集。 在本文中,我們將以一種易於理解的方式解釋初學者,從如何安裝chatgpt智能手機應用程序到語音輸入功能和插件等應用程序所獨有的功能,以及在使用該應用時要牢記的要點。我們還將仔細研究插件限制和設備對設備配置同步
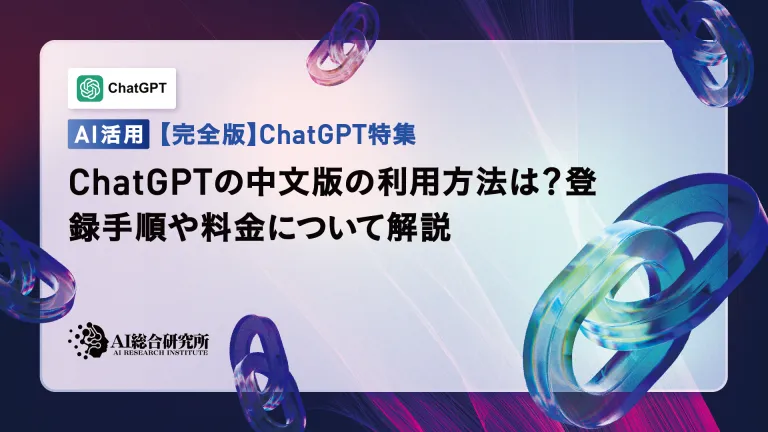
ChatGPT中文版:解鎖中文AI對話新體驗 ChatGPT風靡全球,您知道它也提供中文版本嗎?這款強大的AI工具不僅支持日常對話,還能處理專業內容,並兼容簡體中文和繁體中文。無論是中國地區的使用者,還是正在學習中文的朋友,都能從中受益。 本文將詳細介紹ChatGPT中文版的使用方法,包括賬戶設置、中文提示詞輸入、過濾器的使用、以及不同套餐的選擇,並分析潛在風險及應對策略。此外,我們還將對比ChatGPT中文版和其他中文AI工具,幫助您更好地了解其優勢和應用場景。 OpenAI最新發布的AI智能
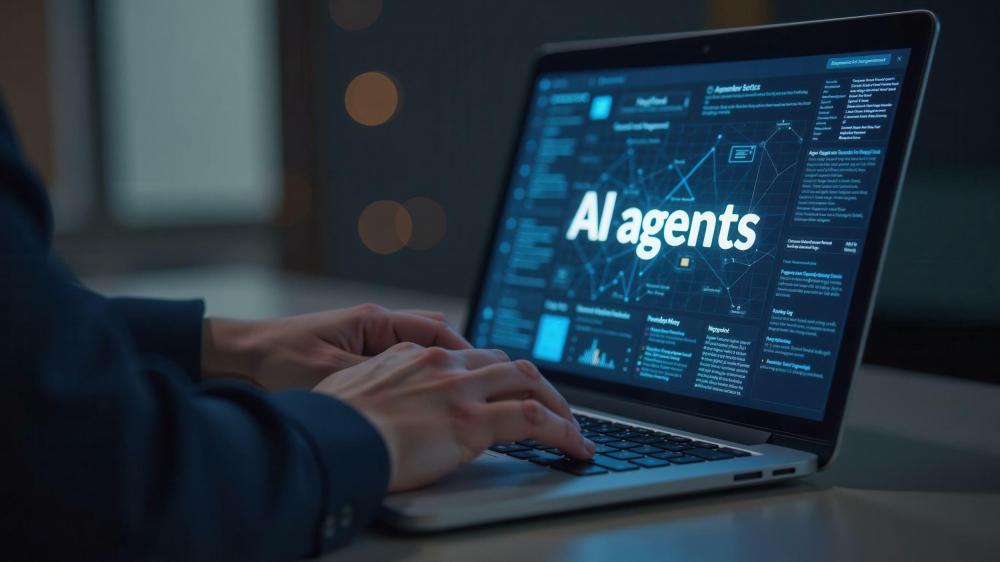
這些可以將其視為生成AI領域的下一個飛躍,這為我們提供了Chatgpt和其他大型語言模型聊天機器人。他們可以代表我們採取行動,而不是簡單地回答問題或產生信息
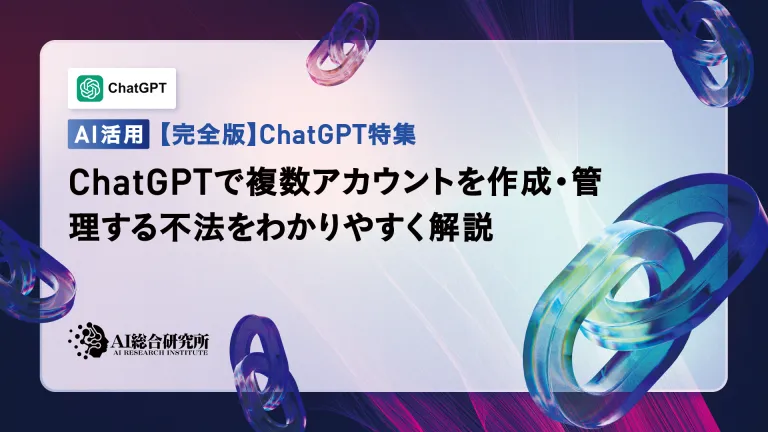
使用chatgpt有效的多個帳戶管理技術|關於如何使用商業和私人生活的詳盡解釋! Chatgpt在各種情況下都使用,但是有些人可能擔心管理多個帳戶。本文將詳細解釋如何為ChatGpt創建多個帳戶,使用時該怎麼做以及如何安全有效地操作它。我們還介紹了重要的一點,例如業務和私人使用差異,並遵守OpenAI的使用條款,並提供指南,以幫助您安全地利用多個帳戶。 Openai


熱AI工具

Undresser.AI Undress
人工智慧驅動的應用程序,用於創建逼真的裸體照片

AI Clothes Remover
用於從照片中去除衣服的線上人工智慧工具。

Undress AI Tool
免費脫衣圖片

Clothoff.io
AI脫衣器

Video Face Swap
使用我們完全免費的人工智慧換臉工具,輕鬆在任何影片中換臉!

熱門文章

熱工具

SublimeText3 Linux新版
SublimeText3 Linux最新版

SecLists
SecLists是最終安全測試人員的伙伴。它是一個包含各種類型清單的集合,這些清單在安全評估過程中經常使用,而且都在一個地方。 SecLists透過方便地提供安全測試人員可能需要的所有列表,幫助提高安全測試的效率和生產力。清單類型包括使用者名稱、密碼、URL、模糊測試有效載荷、敏感資料模式、Web shell等等。測試人員只需將此儲存庫拉到新的測試機上,他就可以存取所需的每種類型的清單。
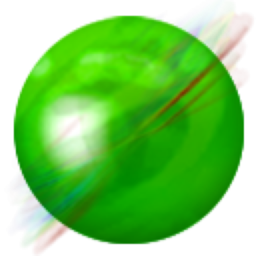
ZendStudio 13.5.1 Mac
強大的PHP整合開發環境

DVWA
Damn Vulnerable Web App (DVWA) 是一個PHP/MySQL的Web應用程序,非常容易受到攻擊。它的主要目標是成為安全專業人員在合法環境中測試自己的技能和工具的輔助工具,幫助Web開發人員更好地理解保護網路應用程式的過程,並幫助教師/學生在課堂環境中教授/學習Web應用程式安全性。 DVWA的目標是透過簡單直接的介面練習一些最常見的Web漏洞,難度各不相同。請注意,該軟體中

記事本++7.3.1
好用且免費的程式碼編輯器