>該教程通過使用Python和Openai構建檢索增強發電(RAG)系統,為您引導您。 RAG通過從您的文檔中檢索相關信息來增強AI的響應,然後再產生答案 - 本質上,讓AI“研究”事先進行。
>您將要學到的內容:
- >從頭開始構建抹布系統。
- >抹布的文檔準備和處理。
- > >使用OpenAi嵌入。
- 創建一個基本的檢索系統。
- > 與OpenAI API集成。
- >
項目結構:
<code>rag-project/ │ ├── src/ │ ├── __init__.py │ ├── document_loader.py │ ├── text_processor.py │ ├── embeddings_manager.py │ ├── retrieval_system.py │ └── rag_system.py │ ├── data/ │ └── documents/ │ ├── requirements.txt ├── test.py ├── README.md └── .env</code>
步驟1:環境設置:
- (在Windows:
- 上)
python -m venv venv
>venvScriptsactivate
激活它: -
source venv/bin/activate
>安裝軟件包: -
pip install openai python-dotenv numpy pandas
創建 : -
requirements.txt
<code>openai==1.12.0 python-dotenv==1.0.0 numpy==1.24.3 pandas==2.1.0</code>configure
- :
-
.env
<code>OPENAI_API_KEY=your_api_key_here</code>步驟2:document loading(
):src/document_loader.py
>
import os from typing import List class DocumentLoader: def __init__(self, documents_path: str): self.documents_path = documents_path def load_documents(self) -> List[str]: documents = [] for filename in os.listdir(self.documents_path): if filename.endswith('.txt'): with open(os.path.join(self.documents_path, filename), 'r') as file: documents.append(file.read()) return documents):
>
src/text_processor.py
步驟4:嵌入式創建(
from typing import List class TextProcessor: def __init__(self, chunk_size: int = 1000): self.chunk_size = chunk_size def split_into_chunks(self, text: str) -> List[str]: words = text.split() chunks = [] current_chunk = [] current_size = 0 for word in words: if current_size + len(word) > self.chunk_size: chunks.append(' '.join(current_chunk)) current_chunk = [word] current_size = len(word) else: current_chunk.append(word) current_size += len(word) + 1 if current_chunk: chunks.append(' '.join(current_chunk)) return chunks
src/embeddings_manager.py
步驟5:檢索系統(
from typing import List import openai import numpy as np class EmbeddingsManager: def __init__(self, api_key: str): openai.api_key = api_key def create_embeddings(self, texts: List[str]) -> List[np.ndarray]: embeddings = [] for text in texts: response = openai.embeddings.create( model="text-embedding-ada-002", input=text ) embeddings.append(np.array(response.data[0].embedding)) return embeddings
>
src/retrieval_system.py
>步驟6:OpenAI Integration(
import numpy as np from typing import List, Tuple class RetrievalSystem: def __init__(self, chunks: List[str], embeddings: List[np.ndarray]): self.chunks = chunks self.embeddings = embeddings def find_similar_chunks(self, query_embedding: np.ndarray, top_k: int = 3) -> List[Tuple[str, float]]: similarities = [] for i, embedding in enumerate(self.embeddings): similarity = np.dot(query_embedding, embedding) / ( np.linalg.norm(query_embedding) * np.linalg.norm(embedding) ) similarities.append((self.chunks[i], similarity)) return sorted(similarities, key=lambda x: x[1], reverse=True)[:top_k]>
>src/rag_system.py
步驟7:系統用法():
import os from dotenv import load_dotenv from typing import List import openai from .document_loader import DocumentLoader from .text_processor import TextProcessor from .embeddings_manager import EmbeddingsManager from .retrieval_system import RetrievalSystem class RAGSystem: def __init__(self): load_dotenv() self.api_key = os.getenv('OPENAI_API_KEY') self.loader = DocumentLoader('data/documents') self.processor = TextProcessor() self.embeddings_manager = EmbeddingsManager(self.api_key) # Initialize system self.initialize_system() def initialize_system(self): # Load and process documents documents = self.loader.load_documents() self.chunks = [] for doc in documents: self.chunks.extend(self.processor.split_into_chunks(doc)) # Create embeddings self.embeddings = self.embeddings_manager.create_embeddings(self.chunks) # Initialize retrieval system self.retrieval_system = RetrievalSystem(self.chunks, self.embeddings) def answer_question(self, question: str) -> str: # Get question embedding question_embedding = self.embeddings_manager.create_embeddings([question])[0] # Get relevant chunks relevant_chunks = self.retrieval_system.find_similar_chunks(question_embedding) # Prepare context context = "\n".join([chunk[0] for chunk in relevant_chunks]) # Create prompt prompt = f"""Context: {context}\n\nQuestion: {question}\n\nAnswer:""" # Get response from OpenAI response = openai.chat.completions.create( model="gpt-4-turbo-preview", messages=[ {"role": "system", "content": "You are a helpful assistant. Use the provided context to answer the question."}, {"role": "user", "content": prompt} ] ) return response.choices[0].message.content>
>將樣本文檔放在test.py
>中。 然後,運行:
.txt
data/documents
結論:test.py
# test.py from src.rag_system import RAGSystem # Initialize the RAG system rag = RAGSystem() # Ask a question question = "What was the answer to the guardian’s riddle, and how did it help Kai?" #Replace with your question based on your documents answer = rag.answer_question(question) print(answer)>
以上是使用Python和Openai構建您的第一個抹布系統的詳細內容。更多資訊請關注PHP中文網其他相關文章!
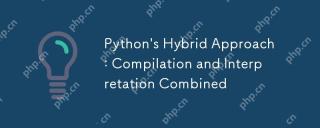
pythonuseshybridapprace,ComminingCompilationTobyTecoDeAndInterpretation.1)codeiscompiledtoplatform-Indepententbybytecode.2)bytecodeisisterpretedbybythepbybythepythonvirtualmachine,增強效率和通用性。
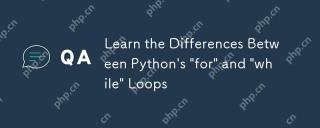
theKeyDifferencesBetnewpython's“ for”和“ for”和“ loopsare:1)” for“ loopsareIdealForiteringSequenceSquencesSorkNowniterations,而2)”,而“ loopsareBetterforConterContinuingUntilacTientInditionIntionismetismetistismetistwithOutpredefinedInedIterations.un
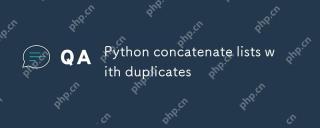
在Python中,可以通過多種方法連接列表並管理重複元素:1)使用 運算符或extend()方法可以保留所有重複元素;2)轉換為集合再轉回列表可以去除所有重複元素,但會丟失原有順序;3)使用循環或列表推導式結合集合可以去除重複元素並保持原有順序。
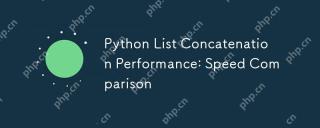
fasteStmethodMethodMethodConcatenationInpythondependersonListsize:1)forsmalllists,operatorseffited.2)forlargerlists,list.extend.extend()orlistComprechensionfaster,withextendEffaster,withExtendEffers,withextend()withextend()是extextend()asmoremory-ememory-emmoremory-emmoremory-emmodifyinginglistsin-place-place-place。
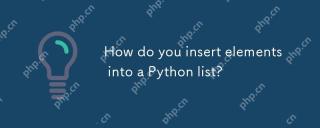
toInSerteLementIntoApythonList,useAppend()toaddtotheend,insert()foreSpificPosition,andextend()formultiplelements.1)useappend()foraddingsingleitemstotheend.2)useAddingsingLeitemStotheend.2)useeapecificindex,toadapecificindex,toadaSpecificIndex,toadaSpecificIndex,blyit'ssssssslorist.3 toaddextext.3
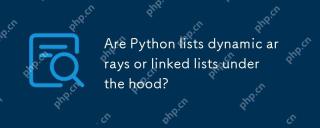
pythonlistsareimplementedasdynamicarrays,notlinkedlists.1)他們areStoredIncoNtiguulMemoryBlocks,mayrequireRealLealLocationWhenAppendingItems,EmpactingPerformance.2)LinkesedlistSwoldOfferefeRefeRefeRefeRefficeInsertions/DeletionsButslowerIndexeDexedAccess,Lestpypytypypytypypytypy
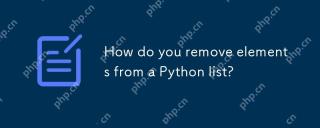
pythonoffersFourmainMethodStoreMoveElement Fromalist:1)刪除(值)emovesthefirstoccurrenceofavalue,2)pop(index)emovesanderturnsanelementataSpecifiedIndex,3)delstatementremoveselemsbybybyselementbybyindexorslicebybyindexorslice,and 4)
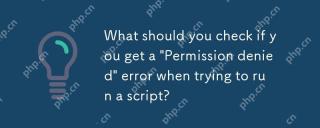
toresolvea“ dermissionded”錯誤Whenrunningascript,跟隨台詞:1)CheckAndAdjustTheScript'Spermissions ofchmod xmyscript.shtomakeitexecutable.2)nesureThEseRethEserethescriptistriptocriptibationalocatiforecationAdirectorywherewhereyOuhaveWritePerMissionsyOuhaveWritePermissionsyYouHaveWritePermissions,susteSyAsyOURHomeRecretectory。


熱AI工具

Undresser.AI Undress
人工智慧驅動的應用程序,用於創建逼真的裸體照片

AI Clothes Remover
用於從照片中去除衣服的線上人工智慧工具。

Undress AI Tool
免費脫衣圖片

Clothoff.io
AI脫衣器

Video Face Swap
使用我們完全免費的人工智慧換臉工具,輕鬆在任何影片中換臉!

熱門文章

熱工具
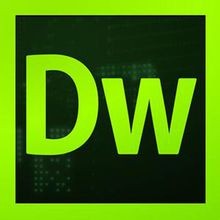
Dreamweaver CS6
視覺化網頁開發工具

DVWA
Damn Vulnerable Web App (DVWA) 是一個PHP/MySQL的Web應用程序,非常容易受到攻擊。它的主要目標是成為安全專業人員在合法環境中測試自己的技能和工具的輔助工具,幫助Web開發人員更好地理解保護網路應用程式的過程,並幫助教師/學生在課堂環境中教授/學習Web應用程式安全性。 DVWA的目標是透過簡單直接的介面練習一些最常見的Web漏洞,難度各不相同。請注意,該軟體中

SublimeText3 英文版
推薦:為Win版本,支援程式碼提示!

mPDF
mPDF是一個PHP庫,可以從UTF-8編碼的HTML產生PDF檔案。原作者Ian Back編寫mPDF以從他的網站上「即時」輸出PDF文件,並處理不同的語言。與原始腳本如HTML2FPDF相比,它的速度較慢,並且在使用Unicode字體時產生的檔案較大,但支援CSS樣式等,並進行了大量增強。支援幾乎所有語言,包括RTL(阿拉伯語和希伯來語)和CJK(中日韓)。支援嵌套的區塊級元素(如P、DIV),
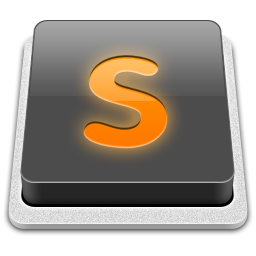
SublimeText3 Mac版
神級程式碼編輯軟體(SublimeText3)