本教學示範使用 LLMOps 最佳實務建構可用於生產的 AI 拉取請求審核器。 最終應用程式可在此處訪問,接受公共 PR URL 並返回人工智慧生成的評論。
應用概述
本教學涵蓋:
- 程式碼開發:從 GitHub 檢索 PR 差異並利用 LiteLLM 進行 LLM 互動。
- 可觀察性: 實現 Agenta 以進行應用程式監控和調試。
- 提示工程:使用 Agenta 的遊樂場迭代提示和模型選擇。
- 法學碩士評估:聘請法學碩士作為法官進行及時和模型評估。
- 部署:將應用程式部署為 API 並使用 v0.dev 建立簡單的 UI。
核心邏輯
AI 助理的工作流程很簡單:給定 PR URL,它從 GitHub 檢索差異並將其提交給 LLM 進行審核。
GitHub 差異可透過以下方式存取:
<code>https://patch-diff.githubusercontent.com/raw/{owner}/{repo}/pull/{pr_number}.diff</code>
這個 Python 函數取得差異:
def get_pr_diff(pr_url): # ... (Code remains the same) return response.text
LiteLLM 促進了 LLM 交互,在不同提供者之間提供一致的介面。
prompt_system = """ You are an expert Python developer performing a file-by-file review of a pull request. You have access to the full diff of the file to understand the overall context and structure. However, focus on reviewing only the specific hunk provided. """ prompt_user = """ Here is the diff for the file: {diff} Please provide a critique of the changes made in this file. """ def generate_critique(pr_url: str): diff = get_pr_diff(pr_url) response = litellm.completion( model=config.model, messages=[ {"content": config.system_prompt, "role": "system"}, {"content": config.user_prompt.format(diff=diff), "role": "user"}, ], ) return response.choices[0].message.content
使用 Agenta 實現可觀察性
Agenta 增強了可觀察性,追蹤輸入、輸出和資料流,以便於偵錯。
初始化 Agenta 並配置 LiteLLM 回呼:
import agenta as ag ag.init() litellm.callbacks = [ag.callbacks.litellm_handler()]
帶有 Agenta 裝飾器的儀器函數:
@ag.instrument() def generate_critique(pr_url: str): # ... (Code remains the same) return response.choices[0].message.content
設定AGENTA_API_KEY
環境變數(從Agenta取得)和可選的AGENTA_HOST
用於自架。
創建法學碩士遊樂場
Agenta 的自訂工作流程功能為迭代開發提供了類似 IDE 的遊樂場。 以下程式碼片段演示了與 Agenta 的配置和整合:
from pydantic import BaseModel, Field from typing import Annotated import agenta as ag import litellm from agenta.sdk.assets import supported_llm_models # ... (previous code) class Config(BaseModel): system_prompt: str = prompt_system user_prompt: str = prompt_user model: Annotated[str, ag.MultipleChoice(choices=supported_llm_models)] = Field(default="gpt-3.5-turbo") @ag.route("/", config_schema=Config) @ag.instrument() def generate_critique(pr_url:str): diff = get_pr_diff(pr_url) config = ag.ConfigManager.get_from_route(schema=Config) response = litellm.completion( model=config.model, messages=[ {"content": config.system_prompt, "role": "system"}, {"content": config.user_prompt.format(diff=diff), "role": "user"}, ], ) return response.choices[0].message.content
使用 Agenta 進行服務和評估
- 執行
agenta init
並指定應用程式名稱和 API 金鑰。 - 運行
agenta variant serve app.py
。
這使得應用程式可以透過 Agenta 的遊樂場進行存取以進行端到端測試。 LLM-as-a-judge用於評估。 評估者提示為:
<code>You are an evaluator grading the quality of a PR review. CRITERIA: ... (criteria remain the same) ANSWER ONLY THE SCORE. DO NOT USE MARKDOWN. DO NOT PROVIDE ANYTHING OTHER THAN THE NUMBER</code>
評估器的使用者提示:
<code>https://patch-diff.githubusercontent.com/raw/{owner}/{repo}/pull/{pr_number}.diff</code>
部署與前端
部署是透過 Agenta 的 UI 完成的:
- 導航到概述頁面。
- 點選所選變體旁的三個點。
- 選擇「部署到生產」。
v0.dev 前端用於快速 UI 建立。
後續步驟與結論
未來的改進包括及時細化、合併完整的程式碼上下文以及處理大的差異。 本教學成功示範了使用 Agenta 和 LiteLLM 建置、評估和部署生產就緒的 AI 拉取請求審閱器。
以上是使用 vev、litellm 和 Agenta 建立 AI 程式碼審查助手的詳細內容。更多資訊請關注PHP中文網其他相關文章!
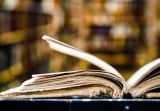
本教程演示如何使用Python處理Zipf定律這一統計概念,並展示Python在處理該定律時讀取和排序大型文本文件的效率。 您可能想知道Zipf分佈這個術語是什麼意思。要理解這個術語,我們首先需要定義Zipf定律。別擔心,我會盡量簡化說明。 Zipf定律 Zipf定律簡單來說就是:在一個大型自然語言語料庫中,最頻繁出現的詞的出現頻率大約是第二頻繁詞的兩倍,是第三頻繁詞的三倍,是第四頻繁詞的四倍,以此類推。 讓我們來看一個例子。如果您查看美國英語的Brown語料庫,您會注意到最頻繁出現的詞是“th
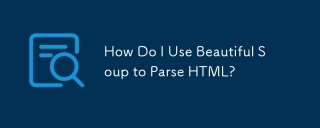
本文解釋瞭如何使用美麗的湯庫來解析html。 它詳細介紹了常見方法,例如find(),find_all(),select()和get_text(),以用於數據提取,處理不同的HTML結構和錯誤以及替代方案(SEL)
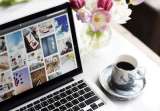
處理嘈雜的圖像是一個常見的問題,尤其是手機或低分辨率攝像頭照片。 本教程使用OpenCV探索Python中的圖像過濾技術來解決此問題。 圖像過濾:功能強大的工具圖像過濾器
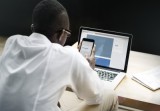
Python 提供多種從互聯網下載文件的方法,可以使用 urllib 包或 requests 庫通過 HTTP 進行下載。本教程將介紹如何使用這些庫通過 Python 從 URL 下載文件。 requests 庫 requests 是 Python 中最流行的庫之一。它允許發送 HTTP/1.1 請求,無需手動將查詢字符串添加到 URL 或對 POST 數據進行表單編碼。 requests 庫可以執行許多功能,包括: 添加表單數據 添加多部分文件 訪問 Python 的響應數據 發出請求 首
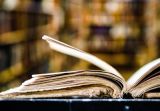
PDF 文件因其跨平台兼容性而廣受歡迎,內容和佈局在不同操作系統、閱讀設備和軟件上保持一致。然而,與 Python 處理純文本文件不同,PDF 文件是二進製文件,結構更複雜,包含字體、顏色和圖像等元素。 幸運的是,借助 Python 的外部模塊,處理 PDF 文件並非難事。本文將使用 PyPDF2 模塊演示如何打開 PDF 文件、打印頁面和提取文本。關於 PDF 文件的創建和編輯,請參考我的另一篇教程。 準備工作 核心在於使用外部模塊 PyPDF2。首先,使用 pip 安裝它: pip 是 P
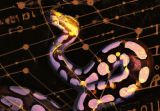
本教程演示瞭如何利用Redis緩存以提高Python應用程序的性能,特別是在Django框架內。 我們將介紹REDIS安裝,Django配置和性能比較,以突出顯示BENE
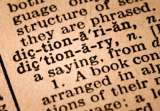
自然語言處理(NLP)是人類語言的自動或半自動處理。 NLP與語言學密切相關,並與認知科學,心理學,生理學和數學的研究有聯繫。在計算機科學
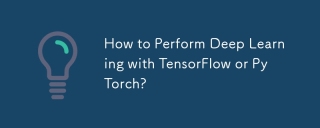
本文比較了Tensorflow和Pytorch的深度學習。 它詳細介紹了所涉及的步驟:數據準備,模型構建,培訓,評估和部署。 框架之間的關鍵差異,特別是關於計算刻度的


熱AI工具

Undresser.AI Undress
人工智慧驅動的應用程序,用於創建逼真的裸體照片

AI Clothes Remover
用於從照片中去除衣服的線上人工智慧工具。

Undress AI Tool
免費脫衣圖片

Clothoff.io
AI脫衣器

AI Hentai Generator
免費產生 AI 無盡。

熱門文章

熱工具
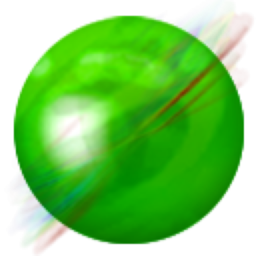
ZendStudio 13.5.1 Mac
強大的PHP整合開發環境

SAP NetWeaver Server Adapter for Eclipse
將Eclipse與SAP NetWeaver應用伺服器整合。
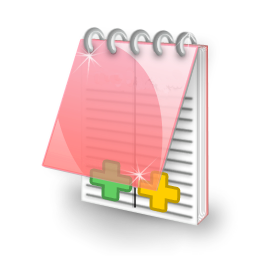
EditPlus 中文破解版
體積小,語法高亮,不支援程式碼提示功能

DVWA
Damn Vulnerable Web App (DVWA) 是一個PHP/MySQL的Web應用程序,非常容易受到攻擊。它的主要目標是成為安全專業人員在合法環境中測試自己的技能和工具的輔助工具,幫助Web開發人員更好地理解保護網路應用程式的過程,並幫助教師/學生在課堂環境中教授/學習Web應用程式安全性。 DVWA的目標是透過簡單直接的介面練習一些最常見的Web漏洞,難度各不相同。請注意,該軟體中

Atom編輯器mac版下載
最受歡迎的的開源編輯器