請我喝杯咖啡☕
*我的貼文解釋了 MS COCO。
CocoDetection() 可以使用 MS COCO 資料集,如下所示。 *這適用於帶有captions_train2017.json、instances_train2017.json和person_keypoints_train2017.json的train2017,帶有captions_v al2017.json、instances_val2017.json和person_keypoints_val2017.json的val2017以及帶有image_info_test2017.json和的test2017 image_info_test-dev2017.json:
from torchvision.datasets import CocoDetection cap_train2017_data = CocoDetection( root="data/coco/imgs/train2017", annFile="data/coco/anns/trainval2017/captions_train2017.json" ) ins_train2017_data = CocoDetection( root="data/coco/imgs/train2017", annFile="data/coco/anns/trainval2017/instances_train2017.json" ) pk_train2017_data = CocoDetection( root="data/coco/imgs/train2017", annFile="data/coco/anns/trainval2017/person_keypoints_train2017.json" ) len(cap_train2017_data), len(ins_train2017_data), len(pk_train2017_data) # (118287, 118287, 118287) cap_val2017_data = CocoDetection( root="data/coco/imgs/val2017", annFile="data/coco/anns/trainval2017/captions_val2017.json" ) ins_val2017_data = CocoDetection( root="data/coco/imgs/val2017", annFile="data/coco/anns/trainval2017/instances_val2017.json" ) pk_val2017_data = CocoDetection( root="data/coco/imgs/val2017", annFile="data/coco/anns/trainval2017/person_keypoints_val2017.json" ) len(cap_val2017_data), len(ins_val2017_data), len(pk_val2017_data) # (5000, 5000, 5000) test2017_data = CocoDetection( root="data/coco/imgs/test2017", annFile="data/coco/anns/test2017/image_info_test2017.json" ) testdev2017_data = CocoDetection( root="data/coco/imgs/test2017", annFile="data/coco/anns/test2017/image_info_test-dev2017.json" ) len(test2017_data), len(testdev2017_data) # (40670, 20288) cap_train2017_data[2] # (<pil.image.image image mode="RGB" size="640x428">, # [{'image_id': 30, 'id': 695774, # 'caption': 'A flower vase is sitting on a porch stand.'}, # {'image_id': 30, 'id': 696557, # 'caption': 'White vase with different colored flowers sitting inside of it. '}, # {'image_id': 30, 'id': 699041, # 'caption': 'a white vase with many flowers on a stage'}, # {'image_id': 30, 'id': 701216, # 'caption': 'A white vase filled with different colored flowers.'}, # {'image_id': 30, 'id': 702428, # 'caption': 'A vase with red and white flowers outside on a sunny day.'}]) cap_train2017_data[47] # (<pil.image.image image mode="RGB" size="640x427">, # [{'image_id': 294, 'id': 549895, # 'caption': 'A man standing in front of a microwave next to pots and pans.'}, # {'image_id': 294, 'id': 556411, # 'caption': 'A man displaying pots and utensils on a wall.'}, # {'image_id': 294, 'id': 556507, # 'caption': 'A man stands in a kitchen and motions towards pots and pans. '}, # {'image_id': 294, 'id': 556993, # 'caption': 'a man poses in front of some pots and pans '}, # {'image_id': 294, 'id': 560728, # 'caption': 'A man pointing to pots hanging from a pegboard on a gray wall.'}]) cap_train2017_data[64] # (<pil.image.image image mode="RGB" size="480x640">, # [{'image_id': 370, 'id': 468271, # 'caption': 'A little girl holding wet broccoli in her hand. '}, # {'image_id': 370, 'id': 471646, # 'caption': 'The young child is happily holding a fresh vegetable. '}, # {'image_id': 370, 'id': 475471, # 'caption': 'A little girl holds a hand full of wet broccoli. '}, # {'image_id': 370, 'id': 475663, # 'caption': 'A little girl holds a piece of broccoli towards the camera.'}, # {'image_id': 370, 'id': 822588, # 'caption': 'a small kid holds on to some vegetables '}]) ins_train2017_data[2] # (<pil.image.image image mode="RGB" size="640x428">, # [{'segmentation': [[267.38, 330.14, 281.81, ..., 269.3, 329.18]], # 'area': 47675.66289999999, 'iscrowd': 0, 'image_id': 30, # 'bbox': [204.86, 31.02, 254.88, 324.12], 'category_id': 64, # 'id': 291613}, # {'segmentation': ..., 'category_id': 86, 'id': 1155486}]) ins_train2017_data[47] # (<pil.image.image image mode="RGB" size="640x427">, # [{'segmentation': [[27.7, 423.27, 27.7, ..., 28.66, 427.0]], # 'area': 64624.86664999999, 'iscrowd': 0, 'image_id': 294, # 'bbox': [27.7, 69.83, 364.91, 357.17], 'category_id': 1, # 'id': 470246}, # {'segmentation': ..., 'category_id': 50, 'id': 708187}, # ... # {'segmentation': ..., 'category_id': 50, 'id': 2217190}]) ins_train2017_data[67] # (<pil.image.image image mode="RGB" size="480x640">, # [{'segmentation': [[90.81, 155.68, 90.81, ..., 98.02, 207.57]], # 'area': 137679.34520000007, 'iscrowd': 0, 'image_id': 370, # 'bbox': [90.81, 24.5, 389.19, 615.5], 'category_id': 1, # 'id': 436109}, # {'segmentation': [[257.51, 446.79, 242.45, ..., 262.02, 460.34]], # 'area': 43818.18095, 'iscrowd': 0, 'image_id': 370, # 'bbox': [242.45, 257.05, 237.55, 243.95], 'category_id': 56, # 'id': 1060727}]) pk_train2017_data[2] # (<pil.image.image image mode="RGB" size="640x428">, []) pk_train2017_data[47] # (<pil.image.image image mode="RGB" size="640x427">, # [{'segmentation': [[27.7, 423.27, 27.7, ..., 28.66, 427]], # 'num_keypoints': 11, 'area': 64624.86665, 'iscrowd': 0, # 'keypoints': [149, 133, 2, 159, ..., 0, 0], 'image_id': 294, # 'bbox': [27.7, 69.83, 364.91, 357.17], 'category_id': 1, # 'id': 470246}]) pk_train2017_data[64] # (<pil.image.image image mode="RGB" size="480x640">, # [{'segmentation': [[90.81, 155.68, 90.81, ..., 98.02, 207.57]], # 'num_keypoints': 12, 'area': 137679.3452, 'iscrowd': 0, # 'keypoints': [229, 171, 2, 263, ..., 0, 0], 'image_id': 370, # 'bbox': [90.81, 24.5, 389.19, 615.5], 'category_id': 1, # 'id': 436109}]) cap_val2017_data[2] # (<pil.image.image image mode="RGB" size="640x483">, # [{'image_id': 632, 'id': 301804, # 'caption': 'Bedroom scene with a bookcase, blue comforter and window.'}, # {'image_id': 632, 'id': 302791, # 'caption': 'A bedroom with a bookshelf full of books.'}, # {'image_id': 632, 'id': 305425, # 'caption': 'This room has a bed with blue sheets and a large bookcase'}, # {'image_id': 632, 'id': 305953, # 'caption': 'A bed and a mirror in a small room.'}, # {'image_id': 632, 'id': 306511, # 'caption': 'a bed room with a neatly made bed a window and a book shelf'}]) cap_val2017_data[47] # (<pil.image.image image mode="RGB" size="640x480">, # [{'image_id': 5001, 'id': 542124, # 'caption': 'A group of people cutting a ribbon on a street.'}, # {'image_id': 5001, 'id': 545685, # 'caption': 'A man uses a pair of big scissors to cut a pink ribbon.'}, # {'image_id': 5001, 'id': 549285, # 'caption': 'A man cutting a ribbon at a ceremony '}, # {'image_id': 5001, 'id': 549666, # 'caption': 'A group of people on the sidewalk watching two young children.'}, # {'image_id': 5001, 'id': 549696, # 'caption': 'A group of people holding a large pair of scissors to a ribbon.'}]) cap_val2017_data[64] # (<pil.image.image image mode="RGB" size="375x500">, # [{'image_id': 6763, 'id': 708378, # 'caption': 'A man and a women posing next to one another in front of a table.'}, # {'image_id': 6763, 'id': 709983, # 'caption': 'A man and woman hugging in a restaurant'}, # {'image_id': 6763, 'id': 711438, # 'caption': 'A man and woman standing next to a table.'}, # {'image_id': 6763, 'id': 711723, # 'caption': 'A happy man and woman pose for a picture.'}, # {'image_id': 6763, 'id': 714720, # 'caption': 'A man and woman posing for a picture in a sports bar.'}]) ins_val2017_data[2] # (<pil.image.image image mode="RGB" size="640x483">, # [{'segmentation': [[5.45, 269.03, 25.08, ..., 3.27, 266.85]], # 'area': 64019.87940000001, 'iscrowd': 0, 'image_id': 632, # 'bbox': [3.27, 266.85, 401.23, 208.25], 'category_id': 65, # 'id': 315724}, # {'segmentation': ..., 'category_id': 64, 'id': 1610466}, # ... # {'segmentation': {'counts': [201255, 6, 328, 6, 142, ..., 4, 34074], # 'size': [483, 640]}, 'area': 20933, 'iscrowd': 1, 'image_id': 632, # 'bbox': [416, 43, 153, 303], 'category_id': 84, # 'id': 908400000632}]) ins_val2017_data[47] # (<pil.image.image image mode="RGB" size="640x480">, # [{'segmentation': [[210.34, 204.76, 227.6, ..., 195.24, 211.24]], # 'area': 5645.972500000001, 'iscrowd': 0, 'image_id': 5001, # 'bbox': [173.66, 204.76, 107.87, 238.39], 'category_id': 87, # 'id': 1158531}, # {'segmentation': ..., 'category_id': 1, 'id': 1201627}, # ... # {'segmentation': {'counts': [251128, 24, 451, 32, 446, ..., 43, 353], # 'size': [480, 640]}, 'area': 10841, 'iscrowd': 1, 'image_id': 5001, # 'bbox': [523, 26, 116, 288], 'category_id': 1, 'id': 900100005001}]) ins_val2017_data[64] # (<pil.image.image image mode="RGB" size="375x500">, # [{'segmentation': [[232.06, 92.6, 369.96, ..., 223.09, 93.72]], # 'area': 11265.648799999995, 'iscrowd': 0, 'image_id': 6763 # 'bbox': [219.73, 64.57, 151.35, 126.69], 'category_id': 72, # 'id': 30601}, # {'segmentation': ..., 'category_id': 1, 'id': 197649}, # ... # {'segmentation': ..., 'category_id': 1, 'id': 1228674}]) pk_val2017_data[2] # (<pil.image.image image mode="RGB" size="640x483">, []) pk_val2017_data[47] # (<pil.image.image image mode="RGB" size="640x480">, # [{'segmentation': [[42.07, 190.11, 45.3, ..., 48.54, 201.98]], # 'num_keypoints': 8, 'area': 5156.63, 'iscrowd': 0, # 'keypoints': [58, 56, 2, 61, ..., 0, 0], 'image_id': 5001, # 'bbox': [10.79, 32.63, 58.24, 169.35], 'category_id': 1, # 'id': 1201627}, # {'segmentation': ..., 'category_id': 1, 'id': 1220394}, # ... # {'segmentation': {'counts': [251128, 24, 451, 32, 446, ..., 43, 353], # 'size': [480, 640]}, 'num_keypoints': 0, 'area': 10841, # 'iscrowd': 1, 'keypoints': [0, 0, 0, 0, ..., 0, 0], # 'image_id': 5001, 'bbox': [523, 26, 116, 288], # 'category_id': 1, 'id': 900100005001}]) pk_val2017_data[64] # (<pil.image.image image mode="RGB" size="375x500">, # [{'segmentation': [[94.38, 462.92, 141.57, ..., 100.27, 459.94]], # 'num_keypoints': 10, 'area': 36153.48825, 'iscrowd': 0, # 'keypoints': [228, 202, 2, 252, ..., 0, 0], 'image_id': 6763, # 'bbox': [79.48, 131.87, 254.23, 331.05], 'category_id': 1, # 'id': 197649}, # {'segmentation': ..., 'category_id': 1, 'id': 212640}, # ... # {'segmentation': ..., 'category_id': 1, 'id': 1228674}]) test2017_data[2] # (<pil.image.image image mode="RGB" size="640x427">, []) test2017_data[47] # (<pil.image.image image mode="RGB" size="640x406">, []) test2017_data[64] # (<pil.image.image image mode="RGB" size="640x427">, []) testdev2017_data[2] # (<pil.image.image image mode="RGB" size="640x427">, []) testdev2017_data[47] # (<pil.image.image image mode="RGB" size="480x640">, []) testdev2017_data[64] # (<pil.image.image image mode="RGB" size="640x480">, []) import matplotlib.pyplot as plt from matplotlib.patches import Polygon, Rectangle import numpy as np from pycocotools import mask # `show_images1()` doesn't work very well for the images with # segmentations and keypoints so for them, use `show_images2()` which # more uses the original coco functions. def show_images1(data, ims, main_title=None): file = data.root.split('/')[-1] fig, axes = plt.subplots(nrows=1, ncols=3, figsize=(14, 8)) fig.suptitle(t=main_title, y=0.9, fontsize=14) x_crd = 0.02 for i, axis in zip(ims, axes.ravel()): if data[i][1] and "caption" in data[i][1][0]: im, anns = data[i] axis.imshow(X=im) axis.set_title(label=anns[0]["image_id"]) y_crd = 0.0 for ann in anns: text_list = ann["caption"].split() if len(text_list) > 9: text = " ".join(text_list[0:10]) + " ..." else: text = " ".join(text_list) plt.figtext(x=x_crd, y=y_crd, fontsize=10, s=f'{ann["id"]}:\n{text}') y_crd -= 0.06 x_crd += 0.325 if i == 2 and file == "val2017": x_crd += 0.06 if data[i][1] and "segmentation" in data[i][1][0]: im, anns = data[i] axis.imshow(X=im) axis.set_title(label=anns[0]["image_id"]) for ann in anns: if "counts" in ann['segmentation']: seg = ann['segmentation'] # rle is Run Length Encoding. uncompressed_rle = [seg['counts']] height, width = seg['size'] compressed_rle = mask.frPyObjects(pyobj=uncompressed_rle, h=height, w=width) # rld is Run Length Decoding. compressed_rld = mask.decode(rleObjs=compressed_rle) y_plts, x_plts = np.nonzero(a=np.squeeze(a=compressed_rld)) axis.plot(x_plts, y_plts, color='yellow') else: for seg in ann['segmentation']: seg_arrs = np.split(ary=np.array(seg), indices_or_sections=len(seg)/2) poly = Polygon(xy=seg_arrs, facecolor="lightgreen", alpha=0.7) axis.add_patch(p=poly) x_plts = [seg_arr[0] for seg_arr in seg_arrs] y_plts = [seg_arr[1] for seg_arr in seg_arrs] axis.plot(x_plts, y_plts, color='yellow') x, y, w, h = ann['bbox'] rect = Rectangle(xy=(x, y), width=w, height=h, linewidth=3, edgecolor='r', facecolor='none', zorder=2) axis.add_patch(p=rect) if data[i][1] and 'keypoints' in data[i][1][0]: kps = ann['keypoints'] kps_arrs = np.split(ary=np.array(kps), indices_or_sections=len(kps)/3) x_plts = [kps_arr[0] for kps_arr in kps_arrs] y_plts = [kps_arr[1] for kps_arr in kps_arrs] nonzeros_x_plts = [] nonzeros_y_plts = [] for x_plt, y_plt in zip(x_plts, y_plts): if x_plt == 0 and y_plt == 0: continue nonzeros_x_plts.append(x_plt) nonzeros_y_plts.append(y_plt) axis.scatter(x=nonzeros_x_plts, y=nonzeros_y_plts, color='yellow') # ↓ ↓ ↓ ↓ ↓ ↓ ↓ ↓ Bad result ↓ ↓ ↓ ↓ ↓ ↓ ↓ ↓ # axis.plot(nonzeros_x_plts, nonzeros_y_plts) if not data[i][1]: im, _ = data[i] axis.imshow(X=im) fig.tight_layout() plt.show() ims = (2, 47, 64) show_images1(data=cap_train2017_data, ims=ims, main_title="cap_train2017_data") show_images1(data=ins_train2017_data, ims=ims, main_title="ins_train2017_data") show_images1(data=pk_train2017_data, ims=ims, main_title="pk_train2017_data") print() show_images1(data=cap_val2017_data, ims=ims, main_title="cap_val2017_data") show_images1(data=ins_val2017_data, ims=ims, main_title="ins_val2017_data") show_images1(data=pk_val2017_data, ims=ims, main_title="pk_val2017_data") print() show_images(data=test2017_data, ims=ims, main_title="test2017_data") show_images(data=testdev2017_data, ims=ims, main_title="testdev2017_data") # `show_images2()` works very well for the images with segmentations and # keypoints. def show_images2(data, index, main_title=None): img_set = data[index] img, img_anns = img_set if img_anns and "segmentation" in img_anns[0]: img_id = img_anns[0]['image_id'] coco = data.coco def show_image(imgIds, areaRng=[], iscrowd=None, draw_bbox=False): plt.figure(figsize=(11, 8)) plt.imshow(X=img) plt.suptitle(t=main_title, y=1, fontsize=14) plt.title(label=img_id, fontsize=14) anns_ids = coco.getAnnIds(imgIds=img_id, areaRng=areaRng, iscrowd=iscrowd) anns = coco.loadAnns(ids=anns_ids) coco.showAnns(anns=anns, draw_bbox=draw_bbox) plt.show() show_image(imgIds=img_id, draw_bbox=True) show_image(imgIds=img_id, draw_bbox=False) show_image(imgIds=img_id, iscrowd=False, draw_bbox=True) show_image(imgIds=img_id, areaRng=[0, 5000], draw_bbox=True) elif img_anns and not "segmentation" in img_anns[0]: plt.figure(figsize=(11, 8)) img_id = img_anns[0]['image_id'] plt.imshow(X=img) plt.suptitle(t=main_title, y=1, fontsize=14) plt.title(label=img_id, fontsize=14) plt.show() elif not img_anns: plt.figure(figsize=(11, 8)) plt.imshow(X=img) plt.suptitle(t=main_title, y=1, fontsize=14) plt.show() show_images2(data=ins_val2017_data, index=2, main_title="ins_val2017_data") print() show_images2(data=pk_val2017_data, index=2, main_title="pk_val2017_data") print() show_images2(data=ins_val2017_data, index=47, main_title="ins_val2017_data") print() show_images2(data=pk_val2017_data, index=47, main_title="pk_val2017_data") </pil.image.image></pil.image.image></pil.image.image></pil.image.image></pil.image.image></pil.image.image></pil.image.image></pil.image.image></pil.image.image></pil.image.image></pil.image.image></pil.image.image></pil.image.image></pil.image.image></pil.image.image></pil.image.image></pil.image.image></pil.image.image></pil.image.image></pil.image.image></pil.image.image></pil.image.image></pil.image.image></pil.image.image>
以上是PyTorch 中的 CocoDetection(2)的詳細內容。更多資訊請關注PHP中文網其他相關文章!
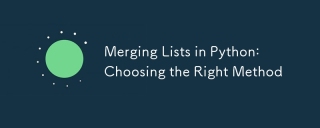
Tomergelistsinpython,YouCanusethe操作員,estextMethod,ListComprehension,Oritertools
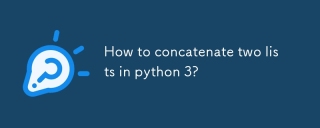
在Python3中,可以通過多種方法連接兩個列表:1)使用 運算符,適用於小列表,但對大列表效率低;2)使用extend方法,適用於大列表,內存效率高,但會修改原列表;3)使用*運算符,適用於合併多個列表,不修改原列表;4)使用itertools.chain,適用於大數據集,內存效率高。
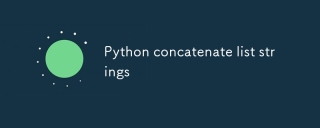
使用join()方法是Python中從列表連接字符串最有效的方法。 1)使用join()方法高效且易讀。 2)循環使用 運算符對大列表效率低。 3)列表推導式與join()結合適用於需要轉換的場景。 4)reduce()方法適用於其他類型歸約,但對字符串連接效率低。完整句子結束。
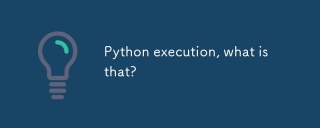
pythonexecutionistheprocessoftransformingpypythoncodeintoExecutablestructions.1)InternterPreterReadSthecode,ConvertingTingitIntObyTecode,whepythonvirtualmachine(pvm)theglobalinterpreterpreterpreterpreterlock(gil)the thepythonvirtualmachine(pvm)
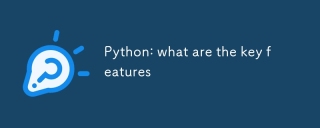
Python的關鍵特性包括:1.語法簡潔易懂,適合初學者;2.動態類型系統,提高開發速度;3.豐富的標準庫,支持多種任務;4.強大的社區和生態系統,提供廣泛支持;5.解釋性,適合腳本和快速原型開發;6.多範式支持,適用於各種編程風格。
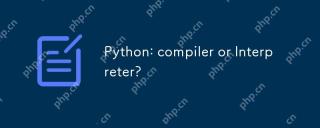
Python是解釋型語言,但也包含編譯過程。 1)Python代碼先編譯成字節碼。 2)字節碼由Python虛擬機解釋執行。 3)這種混合機制使Python既靈活又高效,但執行速度不如完全編譯型語言。
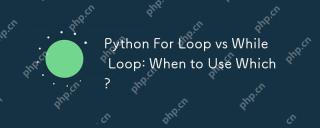
UseeAforloopWheniteratingOveraseQuenceOrforAspecificnumberoftimes; useAwhiLeLoopWhenconTinuingUntilAcIntiment.forloopsareIdealForkNownsences,而WhileLeleLeleLeleLeleLoopSituationSituationsItuationsItuationSuationSituationswithUndEtermentersitations。
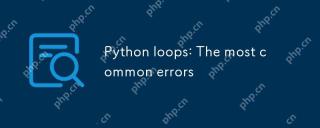
pythonloopscanleadtoerrorslikeinfiniteloops,modifyingListsDuringteritation,逐個偏置,零indexingissues,andnestedloopineflinefficiencies


熱AI工具

Undresser.AI Undress
人工智慧驅動的應用程序,用於創建逼真的裸體照片

AI Clothes Remover
用於從照片中去除衣服的線上人工智慧工具。

Undress AI Tool
免費脫衣圖片

Clothoff.io
AI脫衣器

Video Face Swap
使用我們完全免費的人工智慧換臉工具,輕鬆在任何影片中換臉!

熱門文章

熱工具

DVWA
Damn Vulnerable Web App (DVWA) 是一個PHP/MySQL的Web應用程序,非常容易受到攻擊。它的主要目標是成為安全專業人員在合法環境中測試自己的技能和工具的輔助工具,幫助Web開發人員更好地理解保護網路應用程式的過程,並幫助教師/學生在課堂環境中教授/學習Web應用程式安全性。 DVWA的目標是透過簡單直接的介面練習一些最常見的Web漏洞,難度各不相同。請注意,該軟體中

mPDF
mPDF是一個PHP庫,可以從UTF-8編碼的HTML產生PDF檔案。原作者Ian Back編寫mPDF以從他的網站上「即時」輸出PDF文件,並處理不同的語言。與原始腳本如HTML2FPDF相比,它的速度較慢,並且在使用Unicode字體時產生的檔案較大,但支援CSS樣式等,並進行了大量增強。支援幾乎所有語言,包括RTL(阿拉伯語和希伯來語)和CJK(中日韓)。支援嵌套的區塊級元素(如P、DIV),

Atom編輯器mac版下載
最受歡迎的的開源編輯器

MantisBT
Mantis是一個易於部署的基於Web的缺陷追蹤工具,用於幫助產品缺陷追蹤。它需要PHP、MySQL和一個Web伺服器。請查看我們的演示和託管服務。
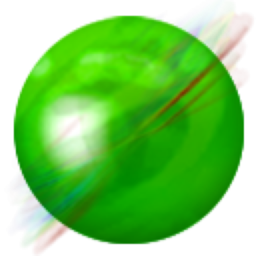
ZendStudio 13.5.1 Mac
強大的PHP整合開發環境