我們在我工作的公司廣泛使用 Plotly 圖表。它們可以輕鬆創建看起來不錯的互動式圖形。透過 Plotly Express 函式庫獲得的 Python 體驗非常棒,而且入門門檻很低。
Plotly 圖表有兩個主要用例:
- 使用 Plotly Dash 的互動式儀表板。將 Plotly 圖表整合到 Dash 中顯然很棒。
- 對於我們的 PDF 報告,我們在渲染 PDF 之前將圖表轉換為圖像。
對於典型的 PDF 報告,我們使用 5-20 個數字來顯示特定指標隨時間的演變、某些值在多個類別上的分佈,或不同類別之間的比較。
為了建立 PDF 報告,我們結合了 Weasyprint、Jinja 和 Plotly 圖表。要將報告呈現為 PDF,我們首先必須將所有圖表呈現為圖像。
使用 Kaleido 渲染圖表
為此,我們使用了很棒的 Kaleido 包。它使用 Chrome 瀏覽器渲染圖形並將其儲存為圖像。該 API 易於使用。
from kaleido.scopes.plotly import PlotlyScope scope = PlotlyScope() img_bytes = scope.transform( figure=figure, format="png", width=1000, height=1000, scale=4, )
這會將圖中的圖形渲染為高度和寬度為 1000 像素、渲染比例為 4 的圖像(即圖像實際尺寸為 4000 像素 x 4000 像素)。比例越高,最終影像的 DPI 越高,看起來越好,最終的 PDF 也越大。
渲染大量圖表
渲染圖表需要一點時間,如果您渲染大量圖表(10-20),它將佔用程式運行時間的很大一部分。為了加快 PDF 渲染管道的速度,我們部署了以下解決方案。
在內部,Kaleido 只是將圖形渲染為圖像的問題外包給附帶的 Chrome 網路瀏覽器。這意味著,對於Python本身來說,渲染這個影像基本上是在等待I/O。
為了加速這個特定的過程,並且由於我們只是等待 I/O,所以我們可以使用多執行緒。
創建隨機圖
讓我們先建立一個隨機圖形,如下所示:
import pandas as pd import numpy as np import plotly.graph_objects as go def get_random_figure() -> go.Figure: n_bars = 50 dates = pd.date_range(start="2021-01-01", end="2021-12-31", freq="M") figure = go.Figure() for i in range(n_bars): values = np.random.rand(len(dates)) figure.add_trace(go.Bar(x=dates, y=values, name=f"Label {i+1}")) figure.update_layout( dict( barmode="group", legend=dict(orientation="h", yanchor="top", xanchor="left"), ) ) figure.update_layout(yaxis=dict(tickformat=".0%"), xaxis=dict(showgrid=False)) return figure
現在,可以使用上面的程式碼將圖形轉換為圖像:
from kaleido.scopes.plotly import PlotlyScope import plotly.graph_objects as go def figure_to_bytes(figure: go.Figure) -> bytes: scope = PlotlyScope() return scope.transform(figure=figure, format="png", width=1000, height=1000, scale=4)
最後我們也為以後定義:
def transform_random_figure() -> bytes: return figure_to_bytes(get_random_figure())
在線程中運行圖像轉換
你可能知道,也可能不知道,由於Python中的GIL(全域解釋器鎖定),只有一個執行緒可以同時執行Python程式碼。由於圖到影像的轉換不是Python程式碼,因此我們可以利用執行緒同時啟動大量圖的轉換,然後收集結果。
為此,我們定義了一個輔助類別:
from kaleido.scopes.plotly import PlotlyScope scope = PlotlyScope() img_bytes = scope.transform( figure=figure, format="png", width=1000, height=1000, scale=4, )
這個類別將幫助我們檢索轉換的結果(即影像的位元組)。
接下來我們要做的就是遵循在 Python 中使用執行緒的標準模式:
- 使用start()方法啟動你想要啟動的執行緒。
- 使用join()方法等待執行緒傳回結果。
我們的執行緒應該每個呼叫transform_random_figure(),然後返回位元組。在本例中我們啟動 10 個執行緒。
import pandas as pd import numpy as np import plotly.graph_objects as go def get_random_figure() -> go.Figure: n_bars = 50 dates = pd.date_range(start="2021-01-01", end="2021-12-31", freq="M") figure = go.Figure() for i in range(n_bars): values = np.random.rand(len(dates)) figure.add_trace(go.Bar(x=dates, y=values, name=f"Label {i+1}")) figure.update_layout( dict( barmode="group", legend=dict(orientation="h", yanchor="top", xanchor="left"), ) ) figure.update_layout(yaxis=dict(tickformat=".0%"), xaxis=dict(showgrid=False)) return figure
start()方法也會呼叫啟動實際邏輯的執行緒的run()方法(即執行給定的函數,在我們的例子中意味著transform_random_figure())。
為了收集結果,我們使用執行緒的 join() 方法並將結果寫入檔案。
from kaleido.scopes.plotly import PlotlyScope import plotly.graph_objects as go def figure_to_bytes(figure: go.Figure) -> bytes: scope = PlotlyScope() return scope.transform(figure=figure, format="png", width=1000, height=1000, scale=4)
它是如何運作的
這裡的主要思想是,每當我們想要將圖形轉換為圖像時,我們都會啟動一個線程,並且該線程將在後台等待圖形完成。
將整個報告放在一起後,我們在所有執行緒上呼叫 join() 並檢索所有圖形的圖像,然後將它們放入報告中。
這樣,我們就可以產生沒有圖表的整個報告,並且無需等待每個圖表本身都被轉換,從而節省時間。
概括
綜上所述,如果您想將多個 Plotly 圖表轉換為映像,請使用 Python 標準庫中的多執行緒模組來加快轉換過程。
您可以非常輕鬆地做到這一點,只需將 transform() 呼叫移到一個執行緒中,然後等待所有執行緒完成即可。
附錄:守則
def transform_random_figure() -> bytes: return figure_to_bytes(get_random_figure())
以上是將 Plotly 圖表並行轉換為影像的詳細內容。更多資訊請關注PHP中文網其他相關文章!
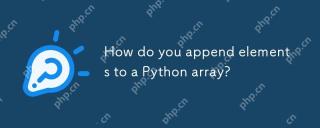
Inpython,YouAppendElementStoAlistusingTheAppend()方法。 1)useappend()forsingleelements:my_list.append(4).2)useextend()orextend()或= formultiplelements:my_list.extend.extend(emote_list)ormy_list = [4,5,6] .3)useInsert()forspefificpositions:my_list.insert(1,5).beaware
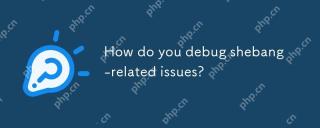
調試shebang問題的方法包括:1.檢查shebang行確保是腳本首行且無前置空格;2.驗證解釋器路徑是否正確;3.直接調用解釋器運行腳本以隔離shebang問題;4.使用strace或truss跟踪系統調用;5.檢查環境變量對shebang的影響。
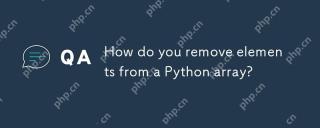
pythonlistscanbemanipulationusseveralmethodstoremovelements:1)theremove()MethodRemovestHefirStocCurrenceOfAstePecificiedValue.2)thepop()thepop()methodRemovesandReturnturnturnturnsanaNelementAgivenIndex.3)
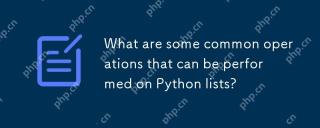
pythristssupportnumeroferations:1)addingElementSwithAppend(),Extend(),andInsert()。 2)emovingItemSusingRemove(),pop(),andclear(),and clear()。 3)訪問andModifyingandmodifyingwithIndexingandSlicing.4)
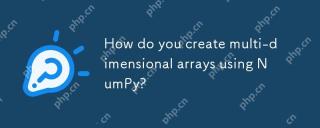
使用NumPy創建多維數組可以通過以下步驟實現:1)使用numpy.array()函數創建數組,例如np.array([[1,2,3],[4,5,6]])創建2D數組;2)使用np.zeros(),np.ones(),np.random.random()等函數創建特定值填充的數組;3)理解數組的shape和size屬性,確保子數組長度一致,避免錯誤;4)使用np.reshape()函數改變數組形狀;5)注意內存使用,確保代碼清晰高效。
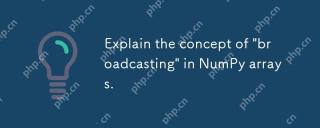
播放innumpyisamethodtoperformoperationsonArraySofDifferentsHapesbyAutapityallate AligningThem.itSimplifififiesCode,增強可讀性,和Boostsperformance.Shere'shore'showitworks:1)較小的ArraySaraySaraysAraySaraySaraySaraySarePaddedDedWiteWithOnestOmatchDimentions.2)
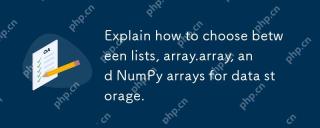
forpythondataTastorage,choselistsforflexibilityWithMixedDatatypes,array.ArrayFormeMory-effficityHomogeneousnumericalData,andnumpyArraysForAdvancedNumericalComputing.listsareversareversareversareversArversatilebutlessEbutlesseftlesseftlesseftlessforefforefforefforefforefforefforefforefforefforlargenumerdataSets; arrayoffray.array.array.array.array.array.ersersamiddreddregro


熱AI工具

Undresser.AI Undress
人工智慧驅動的應用程序,用於創建逼真的裸體照片

AI Clothes Remover
用於從照片中去除衣服的線上人工智慧工具。

Undress AI Tool
免費脫衣圖片

Clothoff.io
AI脫衣器

Video Face Swap
使用我們完全免費的人工智慧換臉工具,輕鬆在任何影片中換臉!

熱門文章

熱工具

SAP NetWeaver Server Adapter for Eclipse
將Eclipse與SAP NetWeaver應用伺服器整合。

MinGW - Minimalist GNU for Windows
這個專案正在遷移到osdn.net/projects/mingw的過程中,你可以繼續在那裡關注我們。 MinGW:GNU編譯器集合(GCC)的本機Windows移植版本,可自由分發的導入函式庫和用於建置本機Windows應用程式的頭檔;包括對MSVC執行時間的擴展,以支援C99功能。 MinGW的所有軟體都可以在64位元Windows平台上運作。
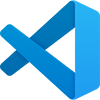
VSCode Windows 64位元 下載
微軟推出的免費、功能強大的一款IDE編輯器

禪工作室 13.0.1
強大的PHP整合開發環境

SublimeText3 英文版
推薦:為Win版本,支援程式碼提示!