動機
在使用Golang 和LLM 進行「假期」測試(之前的帖子...)之後,我一直在尋找一種在Go 中實現LangChain 呼叫的簡單方法,最好使用watsonx.ai。
幸運的是,我找到了以下 Github 儲存庫:https://github.com/tmc/langchaingo(向 Travis Cline 行屈膝禮 https://github.com/tmc)。
在他的儲存庫中,有一個特定的資料夾:https://github.com/tmc/langchaingo/blob/main/examples/watsonx-llm-example/watsonx_example.go 引起了我的注意!
所以像往常一樣,我建立了一個專案並嘗試實現它,並提出了我自己的想法(à ma醬?)。
執行
像往常一樣需要環境變量,我設定了一個 .env 文件,稍後在應用程式中使用。
export WATSONX_API_KEY="your-watsonx-api-key" export WATSONX_PROJECT_ID="your-watsonx-projectid" # I used the US-SOUTH, could be any other region of IBM Cloud export SERVICE_URL="https://us-south.ml.cloud.ibm.com"
在上一篇文章中,我提到嘗試計算法學碩士發送和接收的代幣數量。這項工作仍在進行中,因此我直接在應用程式中使用了「tiktoken-go」庫,並打算對其進行一些更改(在不久的將來?)。無論如何,就我目前的進度而言,它並沒有真正起作用,但它就在那裡。
對於應用程式本身,我幾乎按原樣使用了 Travis 儲存庫中的程式碼,並添加和包裝了以下功能;
- 使用對話框進行提示輸入(?我喜歡對話框?)
- 「嘗試」計算發送給法學碩士和從法學碩士收到的「令牌」數量。 程式碼本身如下;
package main import ( "context" "fmt" "log" "os" "os/exec" "runtime" "fyne.io/fyne/v2" "fyne.io/fyne/v2/app" "fyne.io/fyne/v2/container" "fyne.io/fyne/v2/dialog" "fyne.io/fyne/v2/widget" "github.com/joho/godotenv" "github.com/pkoukk/tiktoken-go" "github.com/tmc/langchaingo/llms" "github.com/tmc/langchaingo/llms/watsonx" ) const ( _tokenApproximation = 4 ) const ( _gpt35TurboContextSize = 4096 _gpt432KContextSize = 32768 _gpt4ContextSize = 8192 _textDavinci3ContextSize = 4097 _textBabbage1ContextSize = 2048 _textAda1ContextSize = 2048 _textCurie1ContextSize = 2048 _codeDavinci2ContextSize = 8000 _codeCushman1ContextSize = 2048 _textBisonContextSize = 2048 _chatBisonContextSize = 2048 _defaultContextSize = 2048 ) // nolint:gochecknoglobals var modelToContextSize = map[string]int{ "gpt-3.5-turbo": _gpt35TurboContextSize, "gpt-4-32k": _gpt432KContextSize, "gpt-4": _gpt4ContextSize, "text-davinci-003": _textDavinci3ContextSize, "text-curie-001": _textCurie1ContextSize, "text-babbage-001": _textBabbage1ContextSize, "text-ada-001": _textAda1ContextSize, "code-davinci-002": _codeDavinci2ContextSize, "code-cushman-001": _codeCushman1ContextSize, } var tokens int func runCmd(name string, arg ...string) { cmd := exec.Command(name, arg...) cmd.Stdout = os.Stdout cmd.Run() } func ClearTerminal() { switch runtime.GOOS { case "darwin": runCmd("clear") case "linux": runCmd("clear") case "windows": runCmd("cmd", "/c", "cls") default: runCmd("clear") } } func promptEntryDialog() string { var promptEntry string // Create a new Fyne application myApp := app.New() myWindow := myApp.NewWindow("Prompt Entry Dialog") // Variable to store user input var userInput string // Button to show the dialog button := widget.NewButton("Click to Enter your prompt's text", func() { entry := widget.NewEntry() dialog.ShowCustomConfirm("Input Dialog", "OK", "Cancel", entry, func(confirm bool) { if confirm { userInput = entry.Text promptEntry = userInput fmt.Println("User Input:", userInput) // Print to the console myWindow.Close() } }, myWindow) }) // Add the button to the window myWindow.SetContent(container.NewVBox( widget.NewLabel("Click the button below to enter text:"), button, )) // Set the window size and run the application myWindow.Resize(fyne.NewSize(400, 200)) myWindow.ShowAndRun() return promptEntry } func CountTokens(model, text string, inorout string) int { var txtLen int e, err := tiktoken.EncodingForModel(model) if err != nil { e, err = tiktoken.GetEncoding("gpt2") if err != nil { log.Printf("[WARN] Failed to calculate number of tokens for model, falling back to approximate count") txtLen = len([]rune(text)) fmt.Println("Guessed tokens for the "+inorout+" text:", txtLen/_tokenApproximation) return txtLen } } return len(e.Encode(text, nil, nil)) } func GetModelContextSize(model string) int { contextSize, ok := modelToContextSize[model] if !ok { return _defaultContextSize } return contextSize } func CalculateMaxTokens(model, text string) int { return GetModelContextSize(model) - CountTokens(model, text, text) } func main() { var prompt, model string // read the '.env' file err := godotenv.Load() if err != nil { log.Fatal("Error loading .env file") } ApiKey := os.Getenv("WATSONX_API_KEY") if ApiKey == "" { log.Fatal("WATSONX_API_KEY environment variable is not set") } ServiceURL := os.Getenv("SERVICE_URL") if ServiceURL == "" { log.Fatal("SERVICE_URL environment variable is not set") } ProjectID := os.Getenv("WATSONX_PROJECT_ID") if ProjectID == "" { log.Fatal("WATSONX_PROJECT_ID environment variable is not set") } // LLM from watsonx.ai model = "ibm/granite-13b-instruct-v2" // model = "meta-llama/llama-3-70b-instruct" llm, err := watsonx.New( model, //// Optional parameters: to be implemented if needed - Not used at this stage but all ready // wx.WithWatsonxAPIKey(ApiKey), // wx.WithWatsonxProjectID("YOUR WATSONX PROJECT ID"), ) if err != nil { log.Fatal(err) } ctx := context.Background() prompt = promptEntryDialog() // for the output visibility on the consol - getting rid of system messages ClearTerminal() // Use the entry variable here fmt.Println("Calling the llm with the user's prompt:", prompt) tokens = CountTokens(model, prompt, "input") completion, err := llms.GenerateFromSinglePrompt( ctx, llm, prompt, llms.WithTopK(10), llms.WithTopP(0.95), llms.WithSeed(25), ) // Check for errors if err != nil { log.Fatal(err) } fmt.Println(completion) tokens = CountTokens(model, completion, "output") }
效果很好,輸出如下圖。
Calling the llm with the user's prompt: What is the distance in Kilmometers from Earth to Moon? 2024/12/31 11:08:04 [WARN] Failed to calculate number of tokens for model, falling back to approximate count Guessed tokens for the input text: 13 The distance from Earth to the Moon is about 384,400 kilometers. 2024/12/31 11:08:04 [WARN] Failed to calculate number of tokens for model, falling back to approximate count Guessed tokens for the output text: 16 ##### Calling the llm with the user's prompt: What is the name of the capital city of France? 2024/12/31 11:39:28 [WARN] Failed to calculate number of tokens for model, falling back to approximate count Guessed tokens for the input text: 11 Paris 2024/12/31 11:39:28 [WARN] Failed to calculate number of tokens for model, falling back to approximate count Guessed tokens for the output text: 1
瞧!
後續步驟
我將為版本 0.2 實現以下功能;
- 提出使用者想要使用的模型,
- 確定令牌數量的更準確方法,
- 一些真正的LangChain實作。
結論
這是我從 Go 應用程式呼叫 LangChain 的工作的一個非常簡單的反映。
敬請期待更多精彩內容?
以上是Go 調用 LangChain(一)的詳細內容。更多資訊請關注PHP中文網其他相關文章!
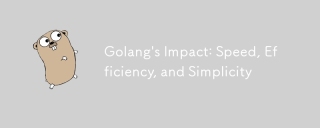
goimpactsdevelopmentpositationality throughspeed,效率和模擬性。 1)速度:gocompilesquicklyandrunseff,IdealforlargeProjects.2)效率:效率:ITScomprehenSevestAndardArdardArdArdArdArdArdArdArdArdArdArdArdArdArdArdArdArdArdArdArdArdArdArdArdArdArdArdArdArdArdArdArdArdArdArdArdArdEcceSteral Depentencies,增強的Depleflovelmentimency.3)簡單性。
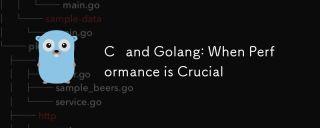
C 更適合需要直接控制硬件資源和高性能優化的場景,而Golang更適合需要快速開發和高並發處理的場景。 1.C 的優勢在於其接近硬件的特性和高度的優化能力,適合遊戲開發等高性能需求。 2.Golang的優勢在於其簡潔的語法和天然的並發支持,適合高並發服務開發。
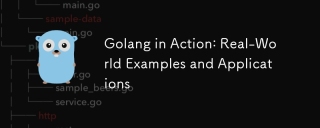
Golang在实际应用中表现出色,以简洁、高效和并发性著称。1)通过Goroutines和Channels实现并发编程,2)利用接口和多态编写灵活代码,3)使用net/http包简化网络编程,4)构建高效并发爬虫,5)通过工具和最佳实践进行调试和优化。
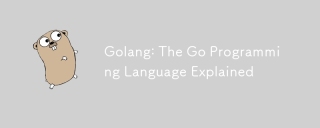
Go語言的核心特性包括垃圾回收、靜態鏈接和並發支持。 1.Go語言的並發模型通過goroutine和channel實現高效並發編程。 2.接口和多態性通過實現接口方法,使得不同類型可以統一處理。 3.基本用法展示了函數定義和調用的高效性。 4.高級用法中,切片提供了動態調整大小的強大功能。 5.常見錯誤如競態條件可以通過gotest-race檢測並解決。 6.性能優化通過sync.Pool重用對象,減少垃圾回收壓力。
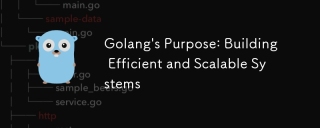
Go語言在構建高效且可擴展的系統中表現出色,其優勢包括:1.高性能:編譯成機器碼,運行速度快;2.並發編程:通過goroutines和channels簡化多任務處理;3.簡潔性:語法簡潔,降低學習和維護成本;4.跨平台:支持跨平台編譯,方便部署。
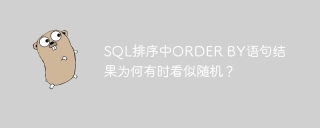
關於SQL查詢結果排序的疑惑學習SQL的過程中,常常會遇到一些令人困惑的問題。最近,筆者在閱讀《MICK-SQL基礎�...
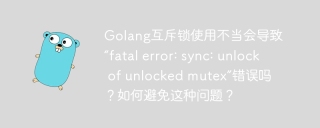
golang ...


熱AI工具

Undresser.AI Undress
人工智慧驅動的應用程序,用於創建逼真的裸體照片

AI Clothes Remover
用於從照片中去除衣服的線上人工智慧工具。

Undress AI Tool
免費脫衣圖片

Clothoff.io
AI脫衣器

AI Hentai Generator
免費產生 AI 無盡。

熱門文章

熱工具
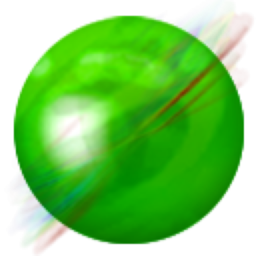
ZendStudio 13.5.1 Mac
強大的PHP整合開發環境
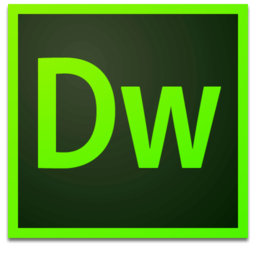
Dreamweaver Mac版
視覺化網頁開發工具

SecLists
SecLists是最終安全測試人員的伙伴。它是一個包含各種類型清單的集合,這些清單在安全評估過程中經常使用,而且都在一個地方。 SecLists透過方便地提供安全測試人員可能需要的所有列表,幫助提高安全測試的效率和生產力。清單類型包括使用者名稱、密碼、URL、模糊測試有效載荷、敏感資料模式、Web shell等等。測試人員只需將此儲存庫拉到新的測試機上,他就可以存取所需的每種類型的清單。
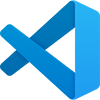
VSCode Windows 64位元 下載
微軟推出的免費、功能強大的一款IDE編輯器
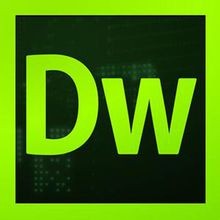
Dreamweaver CS6
視覺化網頁開發工具