Python 資料驗證對於建立健全的應用程式至關重要。我發現實施徹底的驗證技術可以顯著減少錯誤並提高整體程式碼品質。讓我們探討一下我在專案中經常使用的五種強大方法。
Pydantic 已成為我進行資料建模和驗證的首選函式庫。它的簡單性和強大功能使其成為許多場景的絕佳選擇。以下是我通常的使用方式:
from pydantic import BaseModel, EmailStr, validator from typing import List class User(BaseModel): username: str email: EmailStr age: int tags: List[str] = [] @validator('age') def check_age(cls, v): if v <p>在此範例中,Pydantic 自動驗證電子郵件格式並確保所有欄位都具有正確的類型。年齡的自訂驗證器增加了額外的驗證層。 </p> <p>Cerberus 是我經常使用的另一個優秀函式庫,特別是當我需要對驗證過程進行更多控制時。它基於模式的方法非常靈活:<br> </p> <pre class="brush:php;toolbar:false">from cerberus import Validator schema = { 'name': {'type': 'string', 'required': True, 'minlength': 2}, 'age': {'type': 'integer', 'min': 18, 'max': 99}, 'email': {'type': 'string', 'regex': '^[a-zA-Z0-9_.+-]+@[a-zA-Z0-9-]+\.[a-zA-Z0-9-.]+$'}, 'interests': {'type': 'list', 'schema': {'type': 'string'}} } v = Validator(schema) document = {'name': 'John Doe', 'age': 30, 'email': 'john@example.com', 'interests': ['python', 'data science']} if v.validate(document): print("Document is valid") else: print(v.errors)
Cerberus 允許我定義複雜的模式,甚至自訂驗證規則,使其成為具有特定資料要求的專案的理想選擇。
當我使用 Web 框架或 ORM 函式庫時,Marshmallow 特別有用。它的序列化和反序列化能力是一流的:
from marshmallow import Schema, fields, validate, ValidationError class UserSchema(Schema): id = fields.Int(dump_only=True) username = fields.Str(required=True, validate=validate.Length(min=3)) email = fields.Email(required=True) created_at = fields.DateTime(dump_only=True) user_data = {'username': 'john', 'email': 'john@example.com'} schema = UserSchema() try: result = schema.load(user_data) print(result) except ValidationError as err: print(err.messages)
當我需要驗證來自或去往資料庫或 API 的資料時,這種方法特別有效。
Python 的內建類型提示與 mypy 等靜態類型檢查器結合,徹底改變了我編寫和驗證程式碼的方式:
from typing import List, Dict, Optional def process_user_data(name: str, age: int, emails: List[str], metadata: Optional[Dict[str, str]] = None) -> bool: if not 0 <p>當我在此程式碼上執行 mypy 時,它會在運行前捕獲與類型相關的錯誤,從而顯著提高程式碼品質並減少錯誤。 </p> <p>對於 JSON 資料驗證,尤其是 API 開發中,我經常求助於 jsonschema:<br> </p> <pre class="brush:php;toolbar:false">import jsonschema schema = { "type": "object", "properties": { "name": {"type": "string"}, "age": {"type": "number", "minimum": 0}, "pets": { "type": "array", "items": {"type": "string"}, "minItems": 1 } }, "required": ["name", "age"] } data = { "name": "John Doe", "age": 30, "pets": ["dog", "cat"] } try: jsonschema.validate(instance=data, schema=schema) print("Data is valid") except jsonschema.exceptions.ValidationError as err: print(f"Invalid data: {err}")
當我處理複雜的 JSON 結構或需要驗證設定檔時,這種方法特別有用。
在實際應用中,我常常結合這些技巧。例如,我可能會使用 Pydantic 在 FastAPI 應用程式中進行輸入驗證,使用 Marshmallow 進行 ORM 集成,並在整個程式碼庫中使用類型提示進行靜態分析。
以下是我如何使用多種驗證技術建立 Flask 應用程式的範例:
from flask import Flask, request, jsonify from marshmallow import Schema, fields, validate, ValidationError from pydantic import BaseModel, EmailStr from typing import List, Optional import jsonschema app = Flask(__name__) # Pydantic model for request validation class UserCreate(BaseModel): username: str email: EmailStr age: int tags: Optional[List[str]] = [] # Marshmallow schema for database serialization class UserSchema(Schema): id = fields.Int(dump_only=True) username = fields.Str(required=True, validate=validate.Length(min=3)) email = fields.Email(required=True) age = fields.Int(required=True, validate=validate.Range(min=18)) tags = fields.List(fields.Str()) # JSON schema for API response validation response_schema = { "type": "object", "properties": { "id": {"type": "number"}, "username": {"type": "string"}, "email": {"type": "string", "format": "email"}, "age": {"type": "number", "minimum": 18}, "tags": { "type": "array", "items": {"type": "string"} } }, "required": ["id", "username", "email", "age"] } @app.route('/users', methods=['POST']) def create_user(): try: # Validate request data with Pydantic user_data = UserCreate(**request.json) # Simulate database operation user_dict = user_data.dict() user_dict['id'] = 1 # Assume this is set by the database # Serialize with Marshmallow user_schema = UserSchema() result = user_schema.dump(user_dict) # Validate response with jsonschema jsonschema.validate(instance=result, schema=response_schema) return jsonify(result), 201 except ValidationError as err: return jsonify(err.messages), 400 except jsonschema.exceptions.ValidationError as err: return jsonify({"error": str(err)}), 500 if __name__ == '__main__': app.run(debug=True)
在此範例中,我使用 Pydantic 驗證傳入的請求數據,使用 Marshmallow 序列化資料庫操作的數據,並使用 jsonschema 確保 API 回應符合定義的架構。這種多層方法在資料處理的不同階段提供了強大的驗證。
在實現資料驗證時,我總是考慮專案的具體需求。對於簡單的腳本或小型應用程序,使用內建的 Python 功能(例如類型提示和斷言)可能就足夠了。對於較大的項目或具有複雜資料結構的項目,結合 Pydantic、Marshmallow 或 Cerberus 等函式庫可以提供更全面的驗證。
考慮效能影響也很重要。雖然徹底的驗證對於資料完整性至關重要,但過於複雜的驗證可能會減慢應用程式的速度。我經常分析我的程式碼,以確保驗證不會成為瓶頸,尤其是在高流量應用程式中。
錯誤處理是資料驗證的另一個關鍵面向。我確保提供清晰、可操作的錯誤訊息,幫助使用者或其他開發人員理解和修正無效資料。這可能涉及自訂錯誤類別或詳細的錯誤報告機制。
from pydantic import BaseModel, EmailStr, validator from typing import List class User(BaseModel): username: str email: EmailStr age: int tags: List[str] = [] @validator('age') def check_age(cls, v): if v <p>這種方法允許更精細的錯誤處理和報告,這在 API 開發或面向使用者的應用程式中特別有用。 </p> <p>安全性是資料驗證的另一個重要考慮因素。正確的驗證可以防止許多常見的安全漏洞,例如 SQL 注入或跨站點腳本 (XSS) 攻擊。處理使用者輸入時,我總是在將資料用於資料庫查詢或以 HTML 形式呈現之前對其進行清理和驗證。 <br> </p> <pre class="brush:php;toolbar:false">from cerberus import Validator schema = { 'name': {'type': 'string', 'required': True, 'minlength': 2}, 'age': {'type': 'integer', 'min': 18, 'max': 99}, 'email': {'type': 'string', 'regex': '^[a-zA-Z0-9_.+-]+@[a-zA-Z0-9-]+\.[a-zA-Z0-9-.]+$'}, 'interests': {'type': 'list', 'schema': {'type': 'string'}} } v = Validator(schema) document = {'name': 'John Doe', 'age': 30, 'email': 'john@example.com', 'interests': ['python', 'data science']} if v.validate(document): print("Document is valid") else: print(v.errors)
這個簡單的範例示範如何清理使用者輸入以防止 XSS 攻擊。在現實應用程式中,我經常使用更全面的程式庫或框架來提供針對常見安全威脅的內建保護。
測試是實現穩健資料驗證的一個組成部分。我編寫了大量的單元測試,以確保我的驗證邏輯對於有效和無效輸入都能正確運作。這包括測試邊緣情況和邊界條件。
from marshmallow import Schema, fields, validate, ValidationError class UserSchema(Schema): id = fields.Int(dump_only=True) username = fields.Str(required=True, validate=validate.Length(min=3)) email = fields.Email(required=True) created_at = fields.DateTime(dump_only=True) user_data = {'username': 'john', 'email': 'john@example.com'} schema = UserSchema() try: result = schema.load(user_data) print(result) except ValidationError as err: print(err.messages)
這些測試確保使用者模型正確驗證有效和無效輸入,包括類型檢查和必填欄位驗證。
總之,有效的資料驗證是建立健全的 Python 應用程式的關鍵組成部分。透過利用內建 Python 功能和第三方函式庫的組合,我們可以創建全面的驗證系統,以確保資料完整性、提高應用程式可靠性並增強安全性。關鍵是為每個特定用例選擇正確的工具和技術,平衡徹底性與效能和可維護性。透過正確的實施和測試,資料驗證成為創建高品質、可靠的 Python 應用程式的寶貴資產。
我們的創作
一定要看看我們的創作:
投資者中心 | 投資者中央西班牙語 | 投資者中德意志 | 智能生活 | 時代與迴響 | 令人費解的謎團 | 印度教 | 菁英發展 | JS學校
我們在媒體上
科技無尾熊洞察 | 時代與迴響世界 | 投資人中央媒體 | 令人費解的謎團 | | 令人費解的謎團 | >科學與時代媒介 |
現代印度教以上是用於強大應用程式的強大 Python 資料驗證技術的詳細內容。更多資訊請關注PHP中文網其他相關文章!
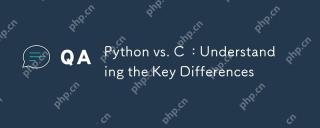
Python和C 各有優勢,選擇應基於項目需求。 1)Python適合快速開發和數據處理,因其簡潔語法和動態類型。 2)C 適用於高性能和系統編程,因其靜態類型和手動內存管理。
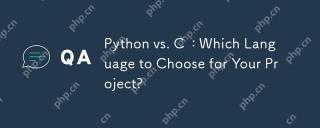
選擇Python還是C 取決於項目需求:1)如果需要快速開發、數據處理和原型設計,選擇Python;2)如果需要高性能、低延遲和接近硬件的控制,選擇C 。
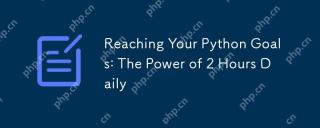
通過每天投入2小時的Python學習,可以有效提升編程技能。 1.學習新知識:閱讀文檔或觀看教程。 2.實踐:編寫代碼和完成練習。 3.複習:鞏固所學內容。 4.項目實踐:應用所學於實際項目中。這樣的結構化學習計劃能幫助你係統掌握Python並實現職業目標。
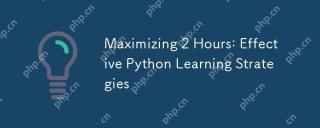
在兩小時內高效學習Python的方法包括:1.回顧基礎知識,確保熟悉Python的安裝和基本語法;2.理解Python的核心概念,如變量、列表、函數等;3.通過使用示例掌握基本和高級用法;4.學習常見錯誤與調試技巧;5.應用性能優化與最佳實踐,如使用列表推導式和遵循PEP8風格指南。
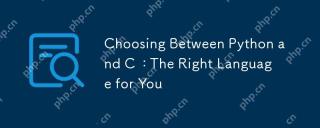
Python適合初學者和數據科學,C 適用於系統編程和遊戲開發。 1.Python簡潔易用,適用於數據科學和Web開發。 2.C 提供高性能和控制力,適用於遊戲開發和系統編程。選擇應基於項目需求和個人興趣。
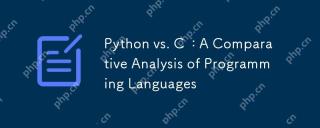
Python更適合數據科學和快速開發,C 更適合高性能和系統編程。 1.Python語法簡潔,易於學習,適用於數據處理和科學計算。 2.C 語法複雜,但性能優越,常用於遊戲開發和系統編程。
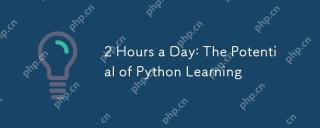
每天投入兩小時學習Python是可行的。 1.學習新知識:用一小時學習新概念,如列表和字典。 2.實踐和練習:用一小時進行編程練習,如編寫小程序。通過合理規劃和堅持不懈,你可以在短時間內掌握Python的核心概念。
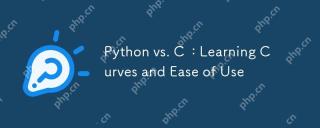
Python更易學且易用,C 則更強大但複雜。 1.Python語法簡潔,適合初學者,動態類型和自動內存管理使其易用,但可能導致運行時錯誤。 2.C 提供低級控制和高級特性,適合高性能應用,但學習門檻高,需手動管理內存和類型安全。


熱AI工具

Undresser.AI Undress
人工智慧驅動的應用程序,用於創建逼真的裸體照片

AI Clothes Remover
用於從照片中去除衣服的線上人工智慧工具。

Undress AI Tool
免費脫衣圖片

Clothoff.io
AI脫衣器

Video Face Swap
使用我們完全免費的人工智慧換臉工具,輕鬆在任何影片中換臉!

熱門文章

熱工具

Atom編輯器mac版下載
最受歡迎的的開源編輯器

SublimeText3 Linux新版
SublimeText3 Linux最新版

mPDF
mPDF是一個PHP庫,可以從UTF-8編碼的HTML產生PDF檔案。原作者Ian Back編寫mPDF以從他的網站上「即時」輸出PDF文件,並處理不同的語言。與原始腳本如HTML2FPDF相比,它的速度較慢,並且在使用Unicode字體時產生的檔案較大,但支援CSS樣式等,並進行了大量增強。支援幾乎所有語言,包括RTL(阿拉伯語和希伯來語)和CJK(中日韓)。支援嵌套的區塊級元素(如P、DIV),

禪工作室 13.0.1
強大的PHP整合開發環境

SecLists
SecLists是最終安全測試人員的伙伴。它是一個包含各種類型清單的集合,這些清單在安全評估過程中經常使用,而且都在一個地方。 SecLists透過方便地提供安全測試人員可能需要的所有列表,幫助提高安全測試的效率和生產力。清單類型包括使用者名稱、密碼、URL、模糊測試有效載荷、敏感資料模式、Web shell等等。測試人員只需將此儲存庫拉到新的測試機上,他就可以存取所需的每種類型的清單。