Python 的記憶體管理是一個引人入勝的話題,但常常被許多開發人員忽略。但了解它的工作原理可以極大地提高你的編碼水平。讓我們仔細看看一些高級概念,特別是weakref和循環垃圾收集。
首先,我們來談談弱引用。這些是非常酷的工具,允許您引用物件而不增加其引用計數。當您試圖避免記憶體洩漏或循環引用時,這非常有用。
這是一個如何使用弱引用的簡單範例:
import weakref class MyClass: def __init__(self, name): self.name = name obj = MyClass("example") weak_ref = weakref.ref(obj) print(weak_ref()) # Output: <__main__.myclass object at ...> del obj print(weak_ref()) # Output: None </__main__.myclass>
在此範例中,我們建立了對物件的弱引用。當我們刪除原來的物件時,弱引用自動變成None。這在快取場景或實現觀察者模式時非常有用。
現在,讓我們深入了解循環垃圾收集。 Python 使用引用計數作為垃圾收集的主要方法,但它也有一個循環垃圾收集器來處理引用循環。當物件相互引用時,就會發生這些循環,從而創建一個循環,防止引用計數達到零。
循環垃圾收集器的工作原理是定期檢查這些循環並打破它們。您實際上可以使用 gc 模組控制何時發生這種情況:
import gc # Disable automatic garbage collection gc.disable() # Do some memory-intensive work here # Manually run garbage collection gc.collect()
這種程度的控制在程式碼的效能關鍵部分非常有用。您可以將垃圾收集推遲到更方便的時間,這可能會加快您的程序。
但是檢測記憶體洩漏又如何呢?這可能很棘手,但 Python 提供了一些工具來提供幫助。 Python 3.4 中引入的tracemalloc 模組特別有用:
import tracemalloc tracemalloc.start() # Your code here snapshot = tracemalloc.take_snapshot() top_stats = snapshot.statistics('lineno') print("[ Top 10 ]") for stat in top_stats[:10]: print(stat)
此程式碼將向您顯示分配最多記憶體的前 10 行程式碼。這是識別潛在記憶體問題的一個很好的起點。
在最佳化大型應用程式中的記憶體使用時,您可以採用多種策略。最有效的方法之一是物件池。您可以維護一個可重複使用物件池,而不是頻繁地建立和銷毀物件:
class ObjectPool: def __init__(self, create_func): self.create_func = create_func self.pool = [] def get(self): if self.pool: return self.pool.pop() return self.create_func() def release(self, obj): self.pool.append(obj) # Usage def create_expensive_object(): # Imagine this is a resource-intensive operation return [0] * 1000000 pool = ObjectPool(create_expensive_object) obj = pool.get() # Use obj... pool.release(obj)
此技術可以顯著減少物件建立和銷毀的開銷,特別是對於資源密集型物件。
記憶體管理的另一個重要方面是了解不同的資料結構如何使用記憶體。例如,Python 中的列表是動態數組,它會過度分配以分攤調整大小的成本。這意味著它們通常使用比您預期更多的記憶體:
import weakref class MyClass: def __init__(self, name): self.name = name obj = MyClass("example") weak_ref = weakref.ref(obj) print(weak_ref()) # Output: <__main__.myclass object at ...> del obj print(weak_ref()) # Output: None </__main__.myclass>
如您所見,清單的記憶體使用量成塊增長,而不是與元素數量呈線性增長。如果記憶體使用很關鍵,您可能需要考慮使用元組(它是不可變的,因此不能過度分配)或數組模組中的數組(它根據元素數量使用固定量的記憶體)。
處理大型資料集時,您可能會發現記憶體不足。在這些情況下,您可以使用生成器來處理區塊中的資料:
import gc # Disable automatic garbage collection gc.disable() # Do some memory-intensive work here # Manually run garbage collection gc.collect()
此方法可讓您處理大於可用 RAM 的檔案。
現在,我們來談談一些不太為人所知的記憶體最佳化技術。您是否知道可以使用 slots 來減少類別的記憶體佔用?當您定義 slots 時,Python 會為該類別的實例使用更節省記憶體的儲存方法:
import tracemalloc tracemalloc.start() # Your code here snapshot = tracemalloc.take_snapshot() top_stats = snapshot.statistics('lineno') print("[ Top 10 ]") for stat in top_stats[:10]: print(stat)
開槽類別每個實例使用的記憶體顯著減少。這可以在創建多個類別實例的程式中節省大量成本。
另一種有趣的技術是使用元類別來實現單例模式,它可以透過確保類別只存在一個實例來幫助控制記憶體使用:
class ObjectPool: def __init__(self, create_func): self.create_func = create_func self.pool = [] def get(self): if self.pool: return self.pool.pop() return self.create_func() def release(self, obj): self.pool.append(obj) # Usage def create_expensive_object(): # Imagine this is a resource-intensive operation return [0] * 1000000 pool = ObjectPool(create_expensive_object) obj = pool.get() # Use obj... pool.release(obj)
這確保了無論您嘗試創建 MyClass 實例多少次,您總是會獲得相同的對象,從而可能節省記憶體。
說到緩存,functools.lru_cache 裝飾器是一個強大的工具。它可以透過快取昂貴的函數呼叫的結果來顯著加速您的程式碼:
import sys l = [] print(sys.getsizeof(l)) # Output: 56 l.append(1) print(sys.getsizeof(l)) # Output: 88 l.extend(range(2, 5)) print(sys.getsizeof(l)) # Output: 120
lru_cache 裝飾器實現了最近最少使用 (LRU) 緩存,這對於許多應用程式來說是一種很好的記憶體高效緩存策略。
讓我們深入研究一些更進階的記憶體分析技術。雖然tracemalloc 很棒,但有時您需要更詳細的資訊。 memory_profiler 套件可以提供程式碼記憶體使用量的逐行分析:
def process_large_file(filename): with open(filename, 'r') as f: for line in f: # Process line yield line for processed_line in process_large_file('huge_file.txt'): # Do something with processed_line
使用 mprof run script.py 執行此命令,然後使用 mprofplot 查看一段時間內記憶體使用情況的圖表。這對於識別記憶體洩漏和理解程式的記憶體行為非常有價值。
說到記憶體洩漏,在 Web 伺服器等長時間運行的應用程式中,它們可能特別棘手。一個常見的原因是忘記正確關閉資源。 contextlib 模組提供了一些工具來幫助解決這個問題:
class RegularClass: def __init__(self, x, y): self.x = x self.y = y class SlottedClass: __slots__ = ['x', 'y'] def __init__(self, x, y): self.x = x self.y = y regular = RegularClass(1, 2) slotted = SlottedClass(1, 2) print(sys.getsizeof(regular)) # Output: 48 print(sys.getsizeof(slotted)) # Output: 16
此模式可確保資源始終正確釋放,即使發生異常也是如此。
當處理非常大的資料集時,有時甚至連生成器都不夠。在這些情況下,記憶體映射檔可以成為救星:
class Singleton(type): _instances = {} def __call__(cls, *args, **kwargs): if cls not in cls._instances: cls._instances[cls] = super(Singleton, cls).__call__(*args, **kwargs) return cls._instances[cls] class MyClass(metaclass=Singleton): pass a = MyClass() b = MyClass() print(a is b) # Output: True
這允許您透過僅將需要的部分載入到記憶體中來處理大於可用 RAM 的檔案。
最後,我們來談談一些 Python 特定的記憶體最佳化。您知道 Python 會快取小整數和短字串嗎?這意味著:
import weakref class MyClass: def __init__(self, name): self.name = name obj = MyClass("example") weak_ref = weakref.ref(obj) print(weak_ref()) # Output: <__main__.myclass object at ...> del obj print(weak_ref()) # Output: None </__main__.myclass>
這種實習可以節省內存,但要小心不要依賴它來進行相等比較。始終使用 == 來表示相等,而不是 is。
總之,Python 的記憶體管理是一個深刻而迷人的話題。透過理解弱引用、循環垃圾收集和各種記憶體優化技術等概念,您可以編寫更有效率、更健壯的 Python 程式碼。請記住,過早的最佳化是萬惡之源,因此首先進行分析並在重要的地方進行最佳化。快樂編碼!
我們的創作
一定要看看我們的創作:
投資者中心 | 智能生活 | 時代與迴響 | 令人費解的謎團 | 印度教 | 菁英發展 | JS學校
我們在媒體上
科技無尾熊洞察 | 時代與迴響世界 | 投資人中央媒體 | 令人費解的謎團 | | 令人費解的謎團 | >科學與時代媒介 |
現代印度教以上是Python 記憶體掌握:提升效能並消除記憶體洩漏的詳細內容。更多資訊請關注PHP中文網其他相關文章!
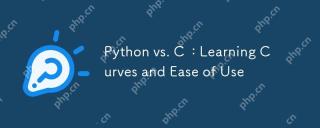
Python更易學且易用,C 則更強大但複雜。 1.Python語法簡潔,適合初學者,動態類型和自動內存管理使其易用,但可能導致運行時錯誤。 2.C 提供低級控制和高級特性,適合高性能應用,但學習門檻高,需手動管理內存和類型安全。
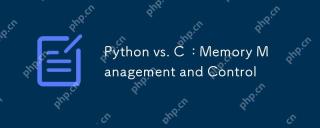
Python和C 在内存管理和控制方面的差异显著。1.Python使用自动内存管理,基于引用计数和垃圾回收,简化了程序员的工作。2.C 则要求手动管理内存,提供更多控制权但增加了复杂性和出错风险。选择哪种语言应基于项目需求和团队技术栈。
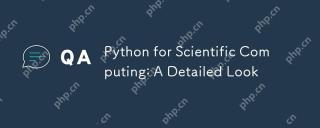
Python在科學計算中的應用包括數據分析、機器學習、數值模擬和可視化。 1.Numpy提供高效的多維數組和數學函數。 2.SciPy擴展Numpy功能,提供優化和線性代數工具。 3.Pandas用於數據處理和分析。 4.Matplotlib用於生成各種圖表和可視化結果。
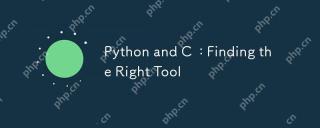
選擇Python還是C 取決於項目需求:1)Python適合快速開發、數據科學和腳本編寫,因其簡潔語法和豐富庫;2)C 適用於需要高性能和底層控制的場景,如係統編程和遊戲開發,因其編譯型和手動內存管理。
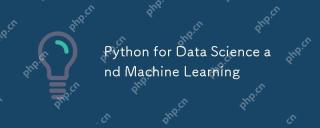
Python在數據科學和機器學習中的應用廣泛,主要依賴於其簡潔性和強大的庫生態系統。 1)Pandas用於數據處理和分析,2)Numpy提供高效的數值計算,3)Scikit-learn用於機器學習模型構建和優化,這些庫讓Python成為數據科學和機器學習的理想工具。
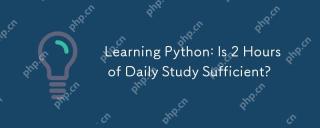
每天學習Python兩個小時是否足夠?這取決於你的目標和學習方法。 1)制定清晰的學習計劃,2)選擇合適的學習資源和方法,3)動手實踐和復習鞏固,可以在這段時間內逐步掌握Python的基本知識和高級功能。
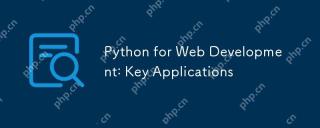
Python在Web開發中的關鍵應用包括使用Django和Flask框架、API開發、數據分析與可視化、機器學習與AI、以及性能優化。 1.Django和Flask框架:Django適合快速開發複雜應用,Flask適用於小型或高度自定義項目。 2.API開發:使用Flask或DjangoRESTFramework構建RESTfulAPI。 3.數據分析與可視化:利用Python處理數據並通過Web界面展示。 4.機器學習與AI:Python用於構建智能Web應用。 5.性能優化:通過異步編程、緩存和代碼優
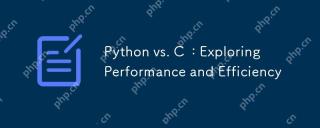
Python在開發效率上優於C ,但C 在執行性能上更高。 1.Python的簡潔語法和豐富庫提高開發效率。 2.C 的編譯型特性和硬件控制提升執行性能。選擇時需根據項目需求權衡開發速度與執行效率。


熱AI工具

Undresser.AI Undress
人工智慧驅動的應用程序,用於創建逼真的裸體照片

AI Clothes Remover
用於從照片中去除衣服的線上人工智慧工具。

Undress AI Tool
免費脫衣圖片

Clothoff.io
AI脫衣器

AI Hentai Generator
免費產生 AI 無盡。

熱門文章

熱工具

SecLists
SecLists是最終安全測試人員的伙伴。它是一個包含各種類型清單的集合,這些清單在安全評估過程中經常使用,而且都在一個地方。 SecLists透過方便地提供安全測試人員可能需要的所有列表,幫助提高安全測試的效率和生產力。清單類型包括使用者名稱、密碼、URL、模糊測試有效載荷、敏感資料模式、Web shell等等。測試人員只需將此儲存庫拉到新的測試機上,他就可以存取所需的每種類型的清單。
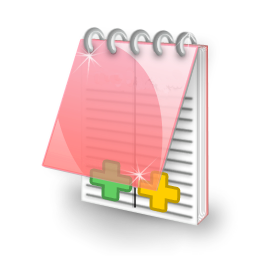
EditPlus 中文破解版
體積小,語法高亮,不支援程式碼提示功能

禪工作室 13.0.1
強大的PHP整合開發環境

SublimeText3 英文版
推薦:為Win版本,支援程式碼提示!

PhpStorm Mac 版本
最新(2018.2.1 )專業的PHP整合開發工具