花崗岩3.0
Granite 3.0 是一個開源、輕量級的生成語言模型系列,專為一系列企業級任務而設計。它原生支援多語言功能、編碼、推理和工具使用,適合企業環境。
我測試了運行這個模型,看看它可以處理哪些任務。
環境設定
我在 Google Colab 中設定了 Granite 3.0 環境,並使用以下指令安裝了必要的函式庫:
!pip install torch torchvision torchaudio !pip install accelerate !pip install -U transformers
執行
我測試了Granite 3.0的2B和8B型號的性能。
2B型號
我運行了 2B 模型。這是 2B 模型的程式碼範例:
import torch from transformers import AutoModelForCausalLM, AutoTokenizer device = "auto" model_path = "ibm-granite/granite-3.0-2b-instruct" tokenizer = AutoTokenizer.from_pretrained(model_path) model = AutoModelForCausalLM.from_pretrained(model_path, device_map=device) model.eval() chat = [ { "role": "user", "content": "Please list one IBM Research laboratory located in the United States. You should only output its name and location." }, ] chat = tokenizer.apply_chat_template(chat, tokenize=False, add_generation_prompt=True) input_tokens = tokenizer(chat, return_tensors="pt").to("cuda") output = model.generate(**input_tokens, max_new_tokens=100) output = tokenizer.batch_decode(output) print(output[0])
輸出
userPlease list one IBM Research laboratory located in the United States. You should only output its name and location. assistant1. IBM Research - Austin, Texas
8B型號
將2b替換為8b即可使用8B模型。以下是 8B 模型的沒有角色和使用者輸入欄位的程式碼範例:
import torch from transformers import AutoModelForCausalLM, AutoTokenizer device = "auto" model_path = "ibm-granite/granite-3.0-8b-instruct" tokenizer = AutoTokenizer.from_pretrained(model_path) model = AutoModelForCausalLM.from_pretrained(model_path, device_map=device) model.eval() chat = [ { "content": "Please list one IBM Research laboratory located in the United States. You should only output its name and location." }, ] chat = tokenizer.apply_chat_template(chat, tokenize=False, add_generation_prompt=True) input_tokens = tokenizer(chat, add_special_tokens=False, return_tensors="pt").to("cuda") output = model.generate(**input_tokens, max_new_tokens=100) generated_text = tokenizer.decode(output[0][input_tokens["input_ids"].shape[1]:], skip_special_tokens=True) print(generated_text)
輸出
1. IBM Almaden Research Center - San Jose, California
函數呼叫
我探索了函數呼叫功能,並使用虛擬函數對其進行了測試。這裡,get_current_weather 被定義為傳回模擬天氣資料。
虛擬函數
import json def get_current_weather(location: str) -> dict: """ Retrieves current weather information for the specified location (default: San Francisco). Args: location (str): Name of the city to retrieve weather data for. Returns: dict: Dictionary containing weather information (temperature, description, humidity). """ print(f"Getting current weather for {location}") try: weather_description = "sample" temperature = "20.0" humidity = "80.0" return { "description": weather_description, "temperature": temperature, "humidity": humidity } except Exception as e: print(f"Error fetching weather data: {e}") return {"weather": "NA"}
即時創作
我建立了一個呼叫函數的提示:
functions = [ { "name": "get_current_weather", "description": "Get the current weather", "parameters": { "type": "object", "properties": { "location": { "type": "string", "description": "The city and country code, e.g. San Francisco, US", } }, "required": ["location"], }, }, ] query = "What's the weather like in Boston?" payload = { "functions_str": [json.dumps(x) for x in functions] } chat = [ {"role":"system","content": f"You are a helpful assistant with access to the following function calls. Your task is to produce a sequence of function calls necessary to generate response to the user utterance. Use the following function calls as required.{payload}"}, {"role": "user", "content": query } ]
響應生成
使用以下程式碼,我產生了一個回應:
instruction_1 = tokenizer.apply_chat_template(chat, tokenize=False, add_generation_prompt=True) input_tokens = tokenizer(instruction_1, return_tensors="pt").to("cuda") output = model.generate(**input_tokens, max_new_tokens=1024) generated_text = tokenizer.decode(output[0][input_tokens["input_ids"].shape[1]:], skip_special_tokens=True) print(generated_text)
輸出
{'name': 'get_current_weather', 'arguments': {'location': 'Boston'}}
這證實了模型能夠根據指定城市產生正確的函數呼叫。
增強互動流程的格式規範
Granite 3.0 允許格式規格以促進結構化格式的回應。本節說明如何使用 [UTTERANCE] 進行回應,並使用 [THINK] 進行內心想法。
另一方面,由於函數呼叫以純文字形式輸出,因此可能需要實作單獨的機制來區分函數呼叫和常規文字回應。
指定輸出格式
以下是指導 AI 輸出的範例提示:
prompt = """You are a conversational AI assistant that deepens interactions by alternating between responses and inner thoughts. <constraints> * Record spoken responses after the [UTTERANCE] tag and inner thoughts after the [THINK] tag. * Use [UTTERANCE] as a start marker to begin outputting an utterance. * After [THINK], describe your internal reasoning or strategy for the next response. This may include insights on the user's reaction, adjustments to improve interaction, or further goals to deepen the conversation. * Important: **Use [UTTERANCE] and [THINK] as a start signal without needing a closing tag.** </constraints> Follow these instructions, alternating between [UTTERANCE] and [THINK] formats for responses. <output example> example1: [UTTERANCE]Hello! How can I assist you today?[THINK]I’ll start with a neutral tone to understand their needs. Preparing to offer specific suggestions based on their response.[UTTERANCE]Thank you! In that case, I have a few methods I can suggest![THINK]Since I now know what they’re looking for, I'll move on to specific suggestions, maintaining a friendly and approachable tone. ... </output>example> Please respond to the following user_input. <user_input> Hello! What can you do? </user_input> """
執行程式碼範例
產生回應的程式碼:
chat = [ { "role": "user", "content": prompt }, ] chat = tokenizer.apply_chat_template(chat, tokenize=False, add_generation_prompt=True) input_tokens = tokenizer(chat, return_tensors="pt").to("cuda") output = model.generate(**input_tokens, max_new_tokens=1024) generated_text = tokenizer.decode(output[0][input_tokens["input_ids"].shape[1]:], skip_special_tokens=True) print(generated_text)
範例輸出
輸出如下:
[UTTERANCE]Hello! I'm here to provide information, answer questions, and assist with various tasks. I can help with a wide range of topics, from general knowledge to specific queries. How can I assist you today? [THINK]I've introduced my capabilities and offered assistance, setting the stage for the user to share their needs or ask questions.
[UTTERANCE] 和 [THINK] 標籤已成功使用,允許有效的回應格式。
根據提示的不同,輸出中有時可能會出現結束標籤(例如[/UTTERANCE]或[/THINK]),但總的來說,一般都可以成功指定輸出格式。
串流程式碼範例
讓我們看看如何輸出流響應。
以下程式碼使用 asyncio 和線程庫來非同步傳輸來自 Granite 3.0 的回應。
!pip install torch torchvision torchaudio !pip install accelerate !pip install -U transformers
範例輸出
執行上述程式碼將產生以下格式的非同步回應:
import torch from transformers import AutoModelForCausalLM, AutoTokenizer device = "auto" model_path = "ibm-granite/granite-3.0-2b-instruct" tokenizer = AutoTokenizer.from_pretrained(model_path) model = AutoModelForCausalLM.from_pretrained(model_path, device_map=device) model.eval() chat = [ { "role": "user", "content": "Please list one IBM Research laboratory located in the United States. You should only output its name and location." }, ] chat = tokenizer.apply_chat_template(chat, tokenize=False, add_generation_prompt=True) input_tokens = tokenizer(chat, return_tensors="pt").to("cuda") output = model.generate(**input_tokens, max_new_tokens=100) output = tokenizer.batch_decode(output) print(output[0])
此範例示範了成功的串流。每個token都是非同步生成並順序顯示,讓使用者可以即時查看生成過程。
概括
Granite 3.0 即使使用 8B 機型也能提供相當強的反應。函數呼叫和格式規範功能也運作良好,顯示其具有廣泛的應用潛力。
以上是我嘗試過花崗岩。的詳細內容。更多資訊請關注PHP中文網其他相關文章!
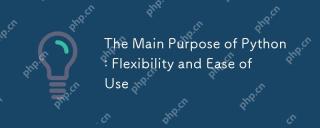
Python的靈活性體現在多範式支持和動態類型系統,易用性則源於語法簡潔和豐富的標準庫。 1.靈活性:支持面向對象、函數式和過程式編程,動態類型系統提高開發效率。 2.易用性:語法接近自然語言,標準庫涵蓋廣泛功能,簡化開發過程。
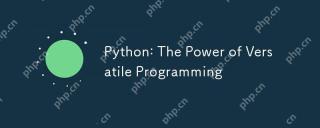
Python因其簡潔與強大而備受青睞,適用於從初學者到高級開發者的各種需求。其多功能性體現在:1)易學易用,語法簡單;2)豐富的庫和框架,如NumPy、Pandas等;3)跨平台支持,可在多種操作系統上運行;4)適合腳本和自動化任務,提升工作效率。
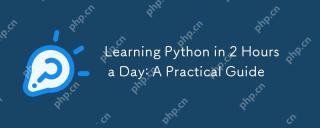
可以,在每天花費兩個小時的時間內學會Python。 1.制定合理的學習計劃,2.選擇合適的學習資源,3.通過實踐鞏固所學知識,這些步驟能幫助你在短時間內掌握Python。
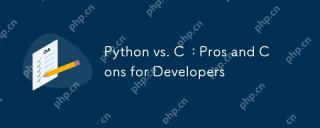
Python適合快速開發和數據處理,而C 適合高性能和底層控制。 1)Python易用,語法簡潔,適用於數據科學和Web開發。 2)C 性能高,控制精確,常用於遊戲和系統編程。
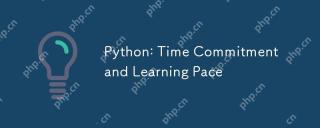
學習Python所需時間因人而異,主要受之前的編程經驗、學習動機、學習資源和方法及學習節奏的影響。設定現實的學習目標並通過實踐項目學習效果最佳。
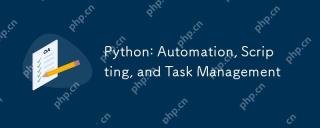
Python在自動化、腳本編寫和任務管理中表現出色。 1)自動化:通過標準庫如os、shutil實現文件備份。 2)腳本編寫:使用psutil庫監控系統資源。 3)任務管理:利用schedule庫調度任務。 Python的易用性和豐富庫支持使其在這些領域中成為首選工具。
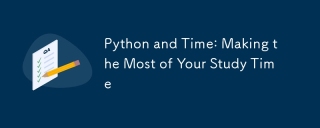
要在有限的時間內最大化學習Python的效率,可以使用Python的datetime、time和schedule模塊。 1.datetime模塊用於記錄和規劃學習時間。 2.time模塊幫助設置學習和休息時間。 3.schedule模塊自動化安排每週學習任務。
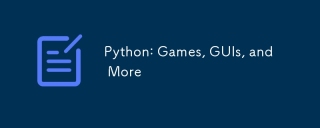
Python在遊戲和GUI開發中表現出色。 1)遊戲開發使用Pygame,提供繪圖、音頻等功能,適合創建2D遊戲。 2)GUI開發可選擇Tkinter或PyQt,Tkinter簡單易用,PyQt功能豐富,適合專業開發。


熱AI工具

Undresser.AI Undress
人工智慧驅動的應用程序,用於創建逼真的裸體照片

AI Clothes Remover
用於從照片中去除衣服的線上人工智慧工具。

Undress AI Tool
免費脫衣圖片

Clothoff.io
AI脫衣器

AI Hentai Generator
免費產生 AI 無盡。

熱門文章

熱工具

記事本++7.3.1
好用且免費的程式碼編輯器

Atom編輯器mac版下載
最受歡迎的的開源編輯器

SAP NetWeaver Server Adapter for Eclipse
將Eclipse與SAP NetWeaver應用伺服器整合。

SecLists
SecLists是最終安全測試人員的伙伴。它是一個包含各種類型清單的集合,這些清單在安全評估過程中經常使用,而且都在一個地方。 SecLists透過方便地提供安全測試人員可能需要的所有列表,幫助提高安全測試的效率和生產力。清單類型包括使用者名稱、密碼、URL、模糊測試有效載荷、敏感資料模式、Web shell等等。測試人員只需將此儲存庫拉到新的測試機上,他就可以存取所需的每種類型的清單。
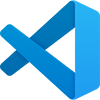
VSCode Windows 64位元 下載
微軟推出的免費、功能強大的一款IDE編輯器