嗨,
我想測試一個小型 LLM 程序,我決定使用 TensorFlow 來實現。
我的原始碼可以在 https://github.com/victordalet/first_llm
一、要求
您需要安裝tensorflow和numpy
pip install 'numpy <hr> <h2> II - 建立資料集 </h2> <p>您需要建立一個資料字串陣列來計算一個小資料集,例如我建立:</p> <pre class="brush:php;toolbar:false"> data = [ "Salut comment ca va", "Je suis en train de coder", "Le machine learning est une branche de l'intelligence artificielle", "Le deep learning est une branche du machine learning", ]
如果你沒有靈感,可以在kaggle上找到一個資料集。
III - 建立模型並訓練它
為此,我使用各種方法建立了一個小型 LLM 類別。
class LLM: def __init__(self): self.model = None self.max_sequence_length = None self.input_sequences = None self.total_words = None self.tokenizer = None self.tokenize() self.create_input_sequences() self.create_model() self.train() test_sentence = "Pour moi le machine learning est" print(self.test(test_sentence, 10)) def tokenize(self): self.tokenizer = Tokenizer() self.tokenizer.fit_on_texts(data) self.total_words = len(self.tokenizer.word_index) + 1 def create_input_sequences(self): self.input_sequences = [] for line in data: token_list = self.tokenizer.texts_to_sequences([line])[0] for i in range(1, len(token_list)): n_gram_sequence = token_list[:i + 1] self.input_sequences.append(n_gram_sequence) self.max_sequence_length = max([len(x) for x in self.input_sequences]) self.input_sequences = pad_sequences(self.input_sequences, maxlen=self.max_sequence_length, padding='pre') def create_model(self): self.model = Sequential() self.model.add(Embedding(self.total_words, 100, input_length=self.max_sequence_length - 1)) self.model.add(LSTM(150, return_sequences=True)) self.model.add(Dropout(0.2)) self.model.add(LSTM(100)) self.model.add(Dense(self.total_words, activation='softmax')) def train(self): self.model.compile(loss='categorical_crossentropy', optimizer='adam', metrics=['accuracy']) X, y = self.input_sequences[:, :-1], self.input_sequences[:, -1] y = tf.keras.utils.to_categorical(y, num_classes=self.total_words) self.model.fit(X, y, epochs=200, verbose=1)
IV - 測試
最後,我使用類別的建構子中呼叫的測試方法來測試模型。
警告:如果產生的單字與前一個單字相同,我會在此測試函數中阻止生成。
def test(self, sentence: str, nb_word_to_generate: int): last_word = "" for _ in range(nb_word_to_generate): token_list = self.tokenizer.texts_to_sequences([sentence])[0] token_list = pad_sequences([token_list], maxlen=self.max_sequence_length - 1, padding='pre') predicted = np.argmax(self.model.predict(token_list), axis=-1) output_word = "" for word, index in self.tokenizer.word_index.items(): if index == predicted: output_word = word break if last_word == output_word: return sentence sentence += " " + output_word last_word = output_word return sentence
以上是建立 LLM 以在 Python 中使用張量流進行測試的詳細內容。更多資訊請關注PHP中文網其他相關文章!
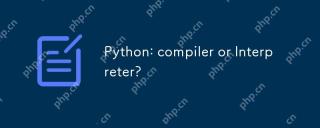
Python是解釋型語言,但也包含編譯過程。 1)Python代碼先編譯成字節碼。 2)字節碼由Python虛擬機解釋執行。 3)這種混合機制使Python既靈活又高效,但執行速度不如完全編譯型語言。
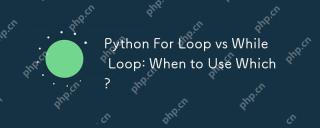
UseeAforloopWheniteratingOveraseQuenceOrforAspecificnumberoftimes; useAwhiLeLoopWhenconTinuingUntilAcIntiment.forloopsareIdealForkNownsences,而WhileLeleLeleLeleLeleLoopSituationSituationsItuationsItuationSuationSituationswithUndEtermentersitations。
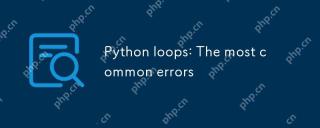
pythonloopscanleadtoerrorslikeinfiniteloops,modifyingListsDuringteritation,逐個偏置,零indexingissues,andnestedloopineflinefficiencies
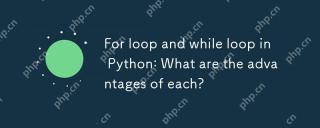
forloopsareadvantageousforknowniterations and sequests,供應模擬性和可讀性;而LileLoopSareIdealFordyNamicConcitionSandunknowniterations,提供ControloperRoverTermination.1)forloopsareperfectForeTectForeTerToratingOrtratingRiteratingOrtratingRitterlistlistslists,callings conspass,calplace,cal,ofstrings ofstrings,orstrings,orstrings,orstrings ofcces
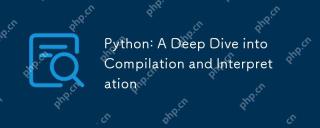
pythonisehybridmodeLofCompilation和interpretation:1)thepythoninterpretercompilesourcecececodeintoplatform- interpententbybytecode.2)thepythonvirtualmachine(pvm)thenexecutecutestestestestestesthisbytecode,ballancingEaseofuseEfuseWithPerformance。
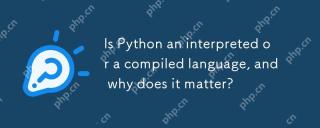
pythonisbothinterpretedAndCompiled.1)它的compiledTobyTecodeForportabilityAcrosplatforms.2)bytecodeisthenInterpreted,允許fordingfordforderynamictynamictymictymictymictyandrapiddefupment,儘管Ititmaybeslowerthananeflowerthanancompiledcompiledlanguages。
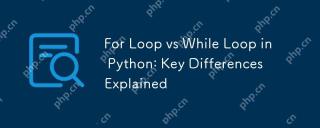
在您的知識之際,而foroopsareideal insinAdvance中,而WhileLoopSareBetterForsituations則youneedtoloopuntilaconditionismet
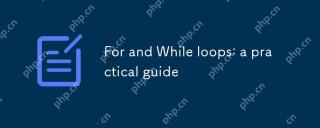
ForboopSareSusedwhenthentheneMberofiterationsiskNownInAdvance,而WhileLoopSareSareDestrationsDepportonAcondition.1)ForloopSareIdealForiteratingOverSequencesLikelistSorarrays.2)whileLeleLooleSuitableApeableableableableableableforscenarioscenarioswhereTheLeTheLeTheLeTeLoopContinusunuesuntilaspecificiccificcificCondond


熱AI工具

Undresser.AI Undress
人工智慧驅動的應用程序,用於創建逼真的裸體照片

AI Clothes Remover
用於從照片中去除衣服的線上人工智慧工具。

Undress AI Tool
免費脫衣圖片

Clothoff.io
AI脫衣器

Video Face Swap
使用我們完全免費的人工智慧換臉工具,輕鬆在任何影片中換臉!

熱門文章

熱工具

Safe Exam Browser
Safe Exam Browser是一個安全的瀏覽器環境,安全地進行線上考試。該軟體將任何電腦變成一個安全的工作站。它控制對任何實用工具的訪問,並防止學生使用未經授權的資源。
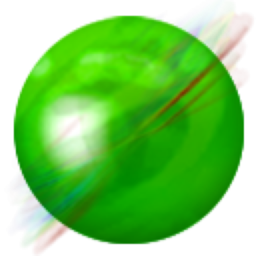
ZendStudio 13.5.1 Mac
強大的PHP整合開發環境

記事本++7.3.1
好用且免費的程式碼編輯器
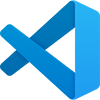
VSCode Windows 64位元 下載
微軟推出的免費、功能強大的一款IDE編輯器

MinGW - Minimalist GNU for Windows
這個專案正在遷移到osdn.net/projects/mingw的過程中,你可以繼續在那裡關注我們。 MinGW:GNU編譯器集合(GCC)的本機Windows移植版本,可自由分發的導入函式庫和用於建置本機Windows應用程式的頭檔;包括對MSVC執行時間的擴展,以支援C99功能。 MinGW的所有軟體都可以在64位元Windows平台上運作。