Climate data plays a crucial role in several sectors, assisting in studies and forecasts that impact areas such as agriculture, urban planning, and natural resource management.
The National Institute of Meteorology (INMET) offers the Meteorological Database (BDMEP) on its website monthly. This database contains a historical series of climate information collected by hundreds of measuring stations distributed throughout Brazil. In BDMEP, you will find detailed data on rainfall, temperature, air humidity and wind speed.
With hourly updates, this data is quite voluminous, providing a rich basis for detailed analysis and informed decision making.
In this post I will show how to collect and process climate data from INMET-BDMEP. We will collect the raw data files available on the INMET website and then process this data to facilitate analysis.
1. Required Python Packages
To achieve the aforementioned objectives, you will only need to have installed three packages:
- httpx to make HTTP requests
- Pandas for reading and processing data
- tqdm to show a friendly progress bar in the terminal while the program downloads or reads files
To install the necessary packages, run the following command in the terminal:
pip install httpx pandas tqdm
If you are using a virtual environment (venv) with poetry, for example, use the following command:
poetry add httpx pandas tqdm
2. Collection of files
2.1 File URL pattern
The address of BDMEP data files follows a very simple pattern. The pattern is as follows:
https://portal.inmet.gov.br/uploads/dadoshistoricos/{year}.zip
The only part that changes is the file name, which is simply the reference year of the data. Monthly, the file for the most recent (current) year is replaced with updated data.
This makes it easy to create code to automatically collect data files from all available years.
In fact, the historical series available begins in the year 2000.
2.2 Collection strategy
To collect data files from INMET-BDMEP we will use the httpx library to make HTTP requests and the tqdm library to show a friendly progress bar in the terminal.
First, let's import the necessary packages:
import datetime as dt from pathlib import Path import httpx from tqdm import tqdm
We have already identified the URL pattern of the INMET-BDMEP data files. Now let's create a function that accepts a year as an argument and returns the URL of the file for that year.
def build_url(year): return f"https://portal.inmet.gov.br/uploads/dadoshistoricos/{year}.zip"
To check whether the URL file has been updated, we can use the information present in the header returned by an HTTP request. On well-configured servers, we can request just this header with the HEAD method. In this case, the server was well configured and we can use this method.
The response to the HEAD request will have the following format:
Mon, 01 Sep 2024 00:01:00 GMT
To parse this date/time I created the following function in Python, which accepts a string and returns a datetime object:
def parse_last_modified(last_modified: str) -> dt.datetime: return dt.datetime.strptime( last_modified, "%a, %d %b %Y %H:%M:%S %Z" )
So, we can use the date/time of the last modification to include it in the name of the file we are going to download, using string interpolation (f-strings):
def build_local_filename(year: int, last_modified: dt.datetime) -> str: return f"inmet-bdmep_{year}_{last_modified:%Y%m%d}.zip"
This way, you can easily check whether the file with the most recent data already exists on our local file system. If the file already exists, the program can be terminated; otherwise, we must proceed with collecting the file, making the request to the server.
The download_year function below downloads the file for a specific year. If the file already exists in the destination directory, the function simply returns without doing anything.
Note how we use tqdm to show a friendly progress bar in the terminal while the file is downloaded.
def download_year( year: int, destdirpath: Path, blocksize: int = 2048, ) -> None: if not destdirpath.exists(): destdirpath.mkdir(parents=True) url = build_url(year) headers = httpx.head(url).headers last_modified = parse_last_modified(headers["Last-Modified"]) file_size = int(headers.get("Content-Length", 0)) destfilename = build_local_filename(year, last_modified) destfilepath = destdirpath / destfilename if destfilepath.exists(): return with httpx.stream("GET", url) as r: pb = tqdm( desc=f"{year}", dynamic_ncols=True, leave=True, total=file_size, unit="iB", unit_scale=True, ) with open(destfilepath, "wb") as f: for data in r.iter_bytes(blocksize): f.write(data) pb.update(len(data)) pb.close()
2.3 File collection
Now that we have all the necessary functions, we can collect the INMET-BDMEP data files.
Using a for loop we can download the files for all available years. The following code does exactly that. Starting from the year 2000 to the current year.
destdirpath = Path("data") for year in range(2000, dt.datetime.now().year + 1): download_year(year, destdirpath)
3. Reading and processing of data
With the INMET-BDMEP raw data files downloaded, we can now read and process the data.
Let's import the necessary packages:
import csv import datetime as dt import io import re import zipfile from pathlib import Path import numpy as np import pandas as pd from tqdm import tqdm
3.1 File structure
Inside the ZIP file provided by INMET we find several CSV files, one for each weather station.
Porém, nas primeiras linhas desses arquivos CSV encontramos informações sobre a estação, como a região, a unidade federativa, o nome da estação, o código WMO, as coordenadas geográficas (latitude e longitude), a altitude e a data de fundação. Vamos extrair essas informações para usar como metadados.
3.2 Leitura dos dados com pandas
A leitura dos arquivos será feita em duas partes: primeiro, será feita a leitura dos metadados das estações meteorológicas; depois, será feita a leitura dos dados históricos propriamente ditos.
3.2.1 Metadados
Para extrair os metadados nas primeiras 8 linhas do arquivo CSV vamos usar o pacote embutido csv do Python.
Para entender a função a seguir é necessário ter um conhecimento um pouco mais avançado de como funciona handlers de arquivos (open), iteradores (next) e expressões regulares (re.match).
def read_metadata(filepath: Path | zipfile.ZipExtFile) -> dict[str, str]: if isinstance(filepath, zipfile.ZipExtFile): f = io.TextIOWrapper(filepath, encoding="latin-1") else: f = open(filepath, "r", encoding="latin-1") reader = csv.reader(f, delimiter=";") _, regiao = next(reader) _, uf = next(reader) _, estacao = next(reader) _, codigo_wmo = next(reader) _, latitude = next(reader) try: latitude = float(latitude.replace(",", ".")) except: latitude = np.nan _, longitude = next(reader) try: longitude = float(longitude.replace(",", ".")) except: longitude = np.nan _, altitude = next(reader) try: altitude = float(altitude.replace(",", ".")) except: altitude = np.nan _, data_fundacao = next(reader) if re.match("[0-9]{4}-[0-9]{2}-[0-9]{2}", data_fundacao): data_fundacao = dt.datetime.strptime( data_fundacao, "%Y-%m-%d", ) elif re.match("[0-9]{2}/[0-9]{2}/[0-9]{2}", data_fundacao): data_fundacao = dt.datetime.strptime( data_fundacao, "%d/%m/%y", ) f.close() return { "regiao": regiao, "uf": uf, "estacao": estacao, "codigo_wmo": codigo_wmo, "latitude": latitude, "longitude": longitude, "altitude": altitude, "data_fundacao": data_fundacao, }
Em resumo, a função read_metadata definida acima lê as primeiras oito linhas do arquivo, processa os dados e retorna um dicionário com as informações extraídas.
3.2.2 Dados históricos
Aqui, finalmente, veremos como fazer a leitura do arquivo CSV. Na verdade é bastante simples. Basta usar a função read_csv do Pandas com os argumentos certos.
A seguir está exposto a chamada da função com os argumentos que eu determinei para a correta leitura do arquivo.
pd.read_csv( "arquivo.csv", sep=";", decimal=",", na_values="-9999", encoding="latin-1", skiprows=8, usecols=range(19), )
Primeiro é preciso dizer que o caractere separador das colunas é o ponto-e-vírgula (;), o separador de número decimal é a vírgula (,) e o encoding é latin-1, muito comum no Brasil.
Também é preciso dizer para pular as 8 primeiras linhas do arquivo (skiprows=8), que contém os metadados da estação), e usar apenas as 19 primeiras colunas (usecols=range(19)).
Por fim, vamos considerar o valor -9999 como sendo nulo (na_values="-9999").
3.3 Tratamento dos dados
Os nomes das colunas dos arquivos CSV do INMET-BDMEP são bem descritivos, mas um pouco longos. E os nomes não são consistentes entre os arquivos e ao longo do tempo. Vamos renomear as colunas para padronizar os nomes e facilitar a manipulação dos dados.
A seguinte função será usada para renomear as colunas usando expressões regulares (RegEx):
def columns_renamer(name: str) -> str: name = name.lower() if re.match(r"data", name): return "data" if re.match(r"hora", name): return "hora" if re.match(r"precipita[çc][ãa]o", name): return "precipitacao" if re.match(r"press[ãa]o atmosf[ée]rica ao n[íi]vel", name): return "pressao_atmosferica" if re.match(r"press[ãa]o atmosf[ée]rica m[áa]x", name): return "pressao_atmosferica_maxima" if re.match(r"press[ãa]o atmosf[ée]rica m[íi]n", name): return "pressao_atmosferica_minima" if re.match(r"radia[çc][ãa]o", name): return "radiacao" if re.match(r"temperatura do ar", name): return "temperatura_ar" if re.match(r"temperatura do ponto de orvalho", name): return "temperatura_orvalho" if re.match(r"temperatura m[áa]x", name): return "temperatura_maxima" if re.match(r"temperatura m[íi]n", name): return "temperatura_minima" if re.match(r"temperatura orvalho m[áa]x", name): return "temperatura_orvalho_maxima" if re.match(r"temperatura orvalho m[íi]n", name): return "temperatura_orvalho_minima" if re.match(r"umidade rel\. m[áa]x", name): return "umidade_relativa_maxima" if re.match(r"umidade rel\. m[íi]n", name): return "umidade_relativa_minima" if re.match(r"umidade relativa do ar", name): return "umidade_relativa" if re.match(r"vento, dire[çc][ãa]o", name): return "vento_direcao" if re.match(r"vento, rajada", name): return "vento_rajada" if re.match(r"vento, velocidade", name): return "vento_velocidade"
Agora que temos os nomes das colunas padronizados, vamos tratar a data/hora. Os arquivos CSV do INMET-BDMEP têm duas colunas separadas para data e hora. Isso é inconveniente, pois é mais prático ter uma única coluna de data/hora. Além disso existem inconsistências nos horários, que às vezes têm minutos e às vezes não.
As três funções a seguir serão usadas para criar uma única coluna de data/hora:
def convert_dates(dates: pd.Series) -> pd.DataFrame: dates = dates.str.replace("/", "-") return dates def convert_hours(hours: pd.Series) -> pd.DataFrame: def fix_hour_string(hour: str) -> str: if re.match(r"^\d{2}\:\d{2}$", hour): return hour else: return hour[:2] + ":00" hours = hours.apply(fix_hour_string) return hours def fix_data_hora(d: pd.DataFrame) -> pd.DataFrame: d = d.assign( data_hora=pd.to_datetime( convert_dates(d["data"]) + " " + convert_hours(d["hora"]), format="%Y-%m-%d %H:%M", ), ) d = d.drop(columns=["data", "hora"]) return d
Existe um problema com os dados do INMET-BDMEP que é a presença de linhas vazias. Vamos remover essas linhas vazias para evitar problemas futuros. O código a seguir faz isso:
# Remove empty rows empty_columns = [ "precipitacao", "pressao_atmosferica", "pressao_atmosferica_maxima", "pressao_atmosferica_minima", "radiacao", "temperatura_ar", "temperatura_orvalho", "temperatura_maxima", "temperatura_minima", "temperatura_orvalho_maxima", "temperatura_orvalho_minima", "umidade_relativa_maxima", "umidade_relativa_minima", "umidade_relativa", "vento_direcao", "vento_rajada", "vento_velocidade", ] empty_rows = data[empty_columns].isnull().all(axis=1) data = data.loc[~empty_rows]
Problema resolvido! (•̀ᴗ•́)و ̑̑
3.4 Encapsulando em funções
Para finalizar esta seção vamos encapsular o código de leitura e tratamento em funções.
Primeiro uma função para a leitura do arquivo CSV contino no arquivo comprimido.
def read_data(filepath: Path) -> pd.DataFrame: d = pd.read_csv( filepath, sep=";", decimal=",", na_values="-9999", encoding="latin-1", skiprows=8, usecols=range(19), ) d = d.rename(columns=columns_renamer) # Remove empty rows empty_columns = [ "precipitacao", "pressao_atmosferica", "pressao_atmosferica_maxima", "pressao_atmosferica_minima", "radiacao", "temperatura_ar", "temperatura_orvalho", "temperatura_maxima", "temperatura_minima", "temperatura_orvalho_maxima", "temperatura_orvalho_minima", "umidade_relativa_maxima", "umidade_relativa_minima", "umidade_relativa", "vento_direcao", "vento_rajada", "vento_velocidade", ] empty_rows = d[empty_columns].isnull().all(axis=1) d = d.loc[~empty_rows] d = fix_data_hora(d) return d
Tem um problema com a função acima. Ela não lida com arquivos ZIP.
Criamos, então, a função read_zipfile para a leitura de todos os arquivos contidos no arquivo ZIP. Essa função itera sobre todos os arquivos CSV no arquivo zipado, faz a leitura usando a função read_data e os metadados usando a função read_metadata, e depois junta os dados e os metadados em um único DataFrame.
def read_zipfile(filepath: Path) -> pd.DataFrame: data = pd.DataFrame() with zipfile.ZipFile(filepath) as z: files = [zf for zf in z.infolist() if not zf.is_dir()] for zf in tqdm(files): d = read_data(z.open(zf.filename)) meta = read_metadata(z.open(zf.filename)) d = d.assign(**meta) data = pd.concat((data, d), ignore_index=True) return data
No final, basta usar essa última função definida (read_zipfile) para fazer a leitura dos arquivos ZIP baixados do site do INMET. (. ❛ ᴗ ❛.)
df = reader.read_zipfile("inmet-bdmep_2023_20240102.zip") # 100%|████████████████████████████████████████████████████████████████████████████████| 567/567 [01:46 <h2> 4. Gráfico de exemplo </h2> <p>Para finalizar, nada mais satisfatório do que fazer gráficos com os dados que coletamos e tratamos. ヾ(≧▽≦*)o</p> <p>Nessa parte uso o R com o pacote tidyverse para fazer um gráfico combinando a temperatura horária e a média diária em São Paulo.<br> </p> <pre class="brush:php;toolbar:false">library(tidyverse) dados filter(uf == "SP") # Temperatura horária em São Paulo dados_sp_h group_by(data_hora) |> summarise( temperatura_ar = mean(temperatura_ar, na.rm = TRUE), ) # Temperatura média diária em São Paulo dados_sp_d group_by(data = floor_date(data_hora, "day")) |> summarise( temperatura_ar = mean(temperatura_ar, na.rm = TRUE), ) # Gráfico combinando temperatura horária e média diária em São Paulo dados_sp_h |> ggplot(aes(x = data_hora, y = temperatura_ar)) + geom_line( alpha = 0.5, aes( color = "Temperatura horária" ) ) + geom_line( data = dados_sp_d, aes( x = data, y = temperatura_ar, color = "Temperatura média diária" ), linewidth = 1 ) + labs( x = "Data", y = "Temperatura (°C)", title = "Temperatura horária e média diária em São Paulo", color = "Variável" ) + theme_minimal() + theme(legend.position = "top") ggsave("temperatura_sp.png", width = 16, height = 8, dpi = 300)
5. Conclusão
Neste texto mostrei como coletar e tratar os dados climáticos do INMET-BDMEP. Os dados coletados são muito úteis para estudos e previsões nas mais variadas áreas. Com os dados tratados, é possível fazer análises e gráficos como o que mostrei no final.
Espero que tenha gostado do texto e que tenha sido útil para você.
我使用本文中展示的函數創建了一個 Python 套件。該包可在我的 Git 存儲庫中找到。如果需要,您可以下載軟體包並在自己的程式碼中使用該函數。
Git 儲存庫:https://github.com/dankkom/inmet-bdmep-data
(~ ̄▽ ̄)~
以上是收集和處理 INMET-BDMEP 氣候數據的詳細內容。更多資訊請關注PHP中文網其他相關文章!
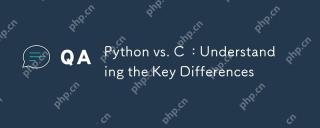
Python和C 各有優勢,選擇應基於項目需求。 1)Python適合快速開發和數據處理,因其簡潔語法和動態類型。 2)C 適用於高性能和系統編程,因其靜態類型和手動內存管理。
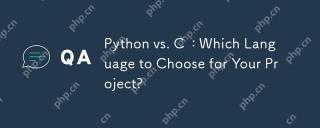
選擇Python還是C 取決於項目需求:1)如果需要快速開發、數據處理和原型設計,選擇Python;2)如果需要高性能、低延遲和接近硬件的控制,選擇C 。
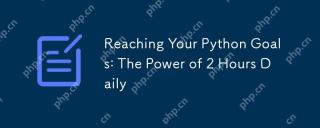
通過每天投入2小時的Python學習,可以有效提升編程技能。 1.學習新知識:閱讀文檔或觀看教程。 2.實踐:編寫代碼和完成練習。 3.複習:鞏固所學內容。 4.項目實踐:應用所學於實際項目中。這樣的結構化學習計劃能幫助你係統掌握Python並實現職業目標。
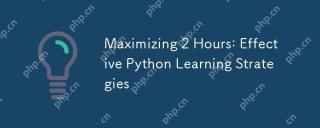
在兩小時內高效學習Python的方法包括:1.回顧基礎知識,確保熟悉Python的安裝和基本語法;2.理解Python的核心概念,如變量、列表、函數等;3.通過使用示例掌握基本和高級用法;4.學習常見錯誤與調試技巧;5.應用性能優化與最佳實踐,如使用列表推導式和遵循PEP8風格指南。
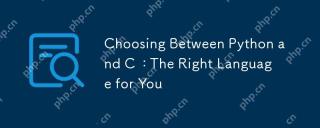
Python適合初學者和數據科學,C 適用於系統編程和遊戲開發。 1.Python簡潔易用,適用於數據科學和Web開發。 2.C 提供高性能和控制力,適用於遊戲開發和系統編程。選擇應基於項目需求和個人興趣。
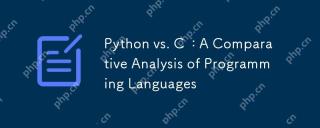
Python更適合數據科學和快速開發,C 更適合高性能和系統編程。 1.Python語法簡潔,易於學習,適用於數據處理和科學計算。 2.C 語法複雜,但性能優越,常用於遊戲開發和系統編程。
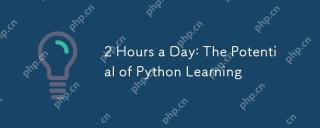
每天投入兩小時學習Python是可行的。 1.學習新知識:用一小時學習新概念,如列表和字典。 2.實踐和練習:用一小時進行編程練習,如編寫小程序。通過合理規劃和堅持不懈,你可以在短時間內掌握Python的核心概念。
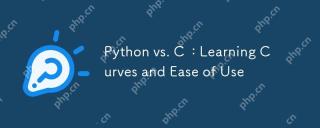
Python更易學且易用,C 則更強大但複雜。 1.Python語法簡潔,適合初學者,動態類型和自動內存管理使其易用,但可能導致運行時錯誤。 2.C 提供低級控制和高級特性,適合高性能應用,但學習門檻高,需手動管理內存和類型安全。


熱AI工具

Undresser.AI Undress
人工智慧驅動的應用程序,用於創建逼真的裸體照片

AI Clothes Remover
用於從照片中去除衣服的線上人工智慧工具。

Undress AI Tool
免費脫衣圖片

Clothoff.io
AI脫衣器

Video Face Swap
使用我們完全免費的人工智慧換臉工具,輕鬆在任何影片中換臉!

熱門文章

熱工具

SublimeText3 Linux新版
SublimeText3 Linux最新版
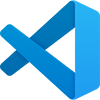
VSCode Windows 64位元 下載
微軟推出的免費、功能強大的一款IDE編輯器

MinGW - Minimalist GNU for Windows
這個專案正在遷移到osdn.net/projects/mingw的過程中,你可以繼續在那裡關注我們。 MinGW:GNU編譯器集合(GCC)的本機Windows移植版本,可自由分發的導入函式庫和用於建置本機Windows應用程式的頭檔;包括對MSVC執行時間的擴展,以支援C99功能。 MinGW的所有軟體都可以在64位元Windows平台上運作。
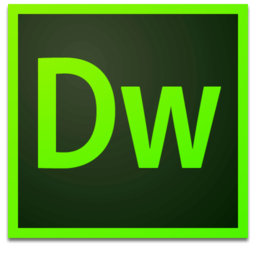
Dreamweaver Mac版
視覺化網頁開發工具

DVWA
Damn Vulnerable Web App (DVWA) 是一個PHP/MySQL的Web應用程序,非常容易受到攻擊。它的主要目標是成為安全專業人員在合法環境中測試自己的技能和工具的輔助工具,幫助Web開發人員更好地理解保護網路應用程式的過程,並幫助教師/學生在課堂環境中教授/學習Web應用程式安全性。 DVWA的目標是透過簡單直接的介面練習一些最常見的Web漏洞,難度各不相同。請注意,該軟體中