1. 簡介和目標
歡迎來到我們關於實施複雜訂單處理系統系列的第四部分!在先前的文章中,我們為我們的專案奠定了基礎,探索了高階時態工作流程,並深入研究了高階資料庫操作。今天,我們專注於任何生產就緒系統的一個同樣重要的方面:監控和警報。
回顧以前的帖子
- 在第 1 部分中,我們設定了專案結構並實作了基本的 CRUD API。
- 在第 2 部分中,我們擴展了 Temporal 的使用,實施複雜的工作流程並探索高階概念。
- 在第 3 部分中,我們將重點放在高階資料庫操作,包括最佳化、分片以及確保分散式系統的一致性。
微服務架構中監控和警報的重要性
在微服務架構中,尤其是處理訂單管理等複雜流程的架構中,有效的監控和警報至關重要。它們使我們能夠:
- 即時了解我們系統的行為與效能
- 在問題影響使用者之前快速識別和診斷問題
- 制定數據驅動的擴展和最佳化決策
- 確保我們服務的可靠性和可用性
Prometheus 及其生態系概述
Prometheus 是一個開源系統監控和警報工具包。由於其強大的功能和廣泛的生態系統,它已成為雲端原生世界的標準。關鍵組件包括:
- Prometheus Server:抓取並儲存時間序列資料
- 客戶端庫:允許輕鬆偵測應用程式程式碼
- Alertmanager :處理來自 Prometheus 伺服器的警報
- Pushgateway:允許臨時和批次作業公開指標
- Exporters:允許第三方系統向 Prometheus 公開指標
我們也將使用 Grafana(一個流行的監控和可觀察性開源平台)來建立儀表板並視覺化我們的 Prometheus 資料。
本系列這一部分的目標
讀完本文,您將能夠:
- 設定 Prometheus 來監控我們的訂單處理系統
- 在我們的 Go 服務中實作自訂指標
- 使用 Grafana 建立資訊豐富的儀表板
- 設定警報規則以通知我們潛在問題
- 有效監控資料庫效能與時態工作流程
讓我們開始吧!
2 理論背景與概念
在開始實施之前,讓我們回顧一些對於我們的監控和警報設定至關重要的關鍵概念。
分散式系統中的可觀察性
可觀察性是指透過檢查系統的輸出來了解系統內部狀態的能力。在像我們的訂單處理系統這樣的分散式系統中,可觀察性通常包含三個主要支柱:
- 指標:在一段時間內測量的數據的數字表示
- 日誌:系統內離散事件的詳細記錄
- 痕跡:跨組件事件因果鏈的表示
在這篇文章中,我們將主要關注指標,儘管我們將討論如何將這些指標與日誌和追蹤整合。
普羅米修斯架構
Prometheus 遵循基於拉動的架構:
- 資料收集:Prometheus 透過 HTTP 從偵測作業抓取指標
- 資料儲存:指標儲存在本機儲存上的時間序列資料庫
- 查詢:PromQL 允許靈活查詢此資料
- 警報:Prometheus 可以根據查詢結果觸發警報
- 可視化:雖然 Prometheus 具有基本的 UI,但它通常與 Grafana 配合使用以實現更豐富的可視化
Prometheus 中的指標類型
Prometheus 提供四種核心指標類型:
- Counter : A cumulative metric that only goes up (e.g., number of requests processed)
- Gauge : A metric that can go up and down (e.g., current memory usage)
- Histogram : Samples observations and counts them in configurable buckets (e.g., request durations)
- Summary : Similar to histogram, but calculates configurable quantiles over a sliding time window
Introduction to PromQL
PromQL (Prometheus Query Language) is a powerful functional language for querying Prometheus data. It allows you to select and aggregate time series data in real time. Key features include:
- Instant vector selectors
- Range vector selectors
- Offset modifier
- Aggregation operators
- Binary operators
We’ll see examples of PromQL queries as we build our dashboards and alerts.
Overview of Grafana
Grafana is a multi-platform open source analytics and interactive visualization web application. It provides charts, graphs, and alerts for the web when connected to supported data sources, of which Prometheus is one. Key features include:
- Flexible dashboard creation
- Wide range of visualization options
- Alerting capabilities
- User authentication and authorization
- Plugin system for extensibility
Now that we’ve covered these concepts, let’s start implementing our monitoring and alerting system.
3. Setting Up Prometheus for Our Order Processing System
Let’s begin by setting up Prometheus to monitor our order processing system.
Installing and Configuring Prometheus
First, let’s add Prometheus to our docker-compose.yml file:
services: # ... other services ... prometheus: image: prom/prometheus:v2.30.3 volumes: - ./prometheus:/etc/prometheus - prometheus_data:/prometheus command: - '--config.file=/etc/prometheus/prometheus.yml' - '--storage.tsdb.path=/prometheus' - '--web.console.libraries=/usr/share/prometheus/console_libraries' - '--web.console.templates=/usr/share/prometheus/consoles' ports: - 9090:9090 volumes: # ... other volumes ... prometheus_data: {}
Next, create a prometheus.yml file in the ./prometheus directory:
global: scrape_interval: 15s evaluation_interval: 15s scrape_configs: - job_name: 'prometheus' static_configs: - targets: ['localhost:9090'] - job_name: 'order_processing_api' static_configs: - targets: ['order_processing_api:8080'] - job_name: 'postgres' static_configs: - targets: ['postgres_exporter:9187']
This configuration tells Prometheus to scrape metrics from itself, our order processing API, and a Postgres exporter (which we’ll set up later).
Implementing Prometheus Exporters for Our Go Services
To expose metrics from our Go services, we’ll use the Prometheus client library. First, add it to your go.mod:
go get github.com/prometheus/client_golang
Now, let’s modify our main Go file to expose metrics:
package main import ( "net/http" "github.com/gin-gonic/gin" "github.com/prometheus/client_golang/prometheus" "github.com/prometheus/client_golang/prometheus/promhttp" ) var ( httpRequestsTotal = prometheus.NewCounterVec( prometheus.CounterOpts{ Name: "http_requests_total", Help: "Total number of HTTP requests", }, []string{"method", "endpoint", "status"}, ) httpRequestDuration = prometheus.NewHistogramVec( prometheus.HistogramOpts{ Name: "http_request_duration_seconds", Help: "Duration of HTTP requests in seconds", Buckets: prometheus.DefBuckets, }, []string{"method", "endpoint"}, ) ) func init() { prometheus.MustRegister(httpRequestsTotal) prometheus.MustRegister(httpRequestDuration) } func main() { r := gin.Default() // Middleware to record metrics r.Use(func(c *gin.Context) { timer := prometheus.NewTimer(httpRequestDuration.WithLabelValues(c.Request.Method, c.FullPath())) c.Next() timer.ObserveDuration() httpRequestsTotal.WithLabelValues(c.Request.Method, c.FullPath(), string(c.Writer.Status())).Inc() }) // Expose metrics endpoint r.GET("/metrics", gin.WrapH(promhttp.Handler())) // ... rest of your routes ... r.Run(":8080") }
This code sets up two metrics:
- http_requests_total: A counter that tracks the total number of HTTP requests
- http_request_duration_seconds: A histogram that tracks the duration of HTTP requests
Setting Up Service Discovery for Dynamic Environments
For more dynamic environments, Prometheus supports various service discovery mechanisms. For example, if you’re running on Kubernetes, you might use the Kubernetes SD configuration:
scrape_configs: - job_name: 'kubernetes-pods' kubernetes_sd_configs: - role: pod relabel_configs: - source_labels: [__meta_kubernetes_pod_annotation_prometheus_io_scrape] action: keep regex: true - source_labels: [__meta_kubernetes_pod_annotation_prometheus_io_path] action: replace target_label: __metrics_path__ regex: (.+)
This configuration will automatically discover and scrape metrics from pods with the appropriate annotations.
Configuring Retention and Storage for Prometheus Data
Prometheus stores data in a time-series database on the local filesystem. You can configure retention time and storage size in the Prometheus configuration:
global: scrape_interval: 15s evaluation_interval: 15s storage: tsdb: retention.time: 15d retention.size: 50GB # ... rest of the configuration ...
This configuration sets a retention period of 15 days and a maximum storage size of 50GB.
In the next section, we’ll dive into defining and implementing custom metrics for our order processing system.
4. Defining and Implementing Custom Metrics
Now that we have Prometheus set up and basic HTTP metrics implemented, let’s define and implement custom metrics specific to our order processing system.
Designing a Metrics Schema for Our Order Processing System
When designing metrics, it’s important to think about what insights we want to gain from our system. For our order processing system, we might want to track:
- Order creation rate
- Order processing time
- Order status distribution
- Payment processing success/failure rate
- Inventory update operations
- Shipping arrangement time
Let’s implement these metrics:
package metrics import ( "github.com/prometheus/client_golang/prometheus" "github.com/prometheus/client_golang/prometheus/promauto" ) var ( OrdersCreated = promauto.NewCounter(prometheus.CounterOpts{ Name: "orders_created_total", Help: "The total number of created orders", }) OrderProcessingTime = promauto.NewHistogram(prometheus.HistogramOpts{ Name: "order_processing_seconds", Help: "Time taken to process an order", Buckets: prometheus.LinearBuckets(0, 30, 10), // 0-300 seconds, 30-second buckets }) OrderStatusGauge = promauto.NewGaugeVec(prometheus.GaugeOpts{ Name: "orders_by_status", Help: "Number of orders by status", }, []string{"status"}) PaymentProcessed = promauto.NewCounterVec(prometheus.CounterOpts{ Name: "payments_processed_total", Help: "The total number of processed payments", }, []string{"status"}) InventoryUpdates = promauto.NewCounter(prometheus.CounterOpts{ Name: "inventory_updates_total", Help: "The total number of inventory updates", }) ShippingArrangementTime = promauto.NewHistogram(prometheus.HistogramOpts{ Name: "shipping_arrangement_seconds", Help: "Time taken to arrange shipping", Buckets: prometheus.LinearBuckets(0, 60, 5), // 0-300 seconds, 60-second buckets }) )
Implementing Application-Specific Metrics in Our Go Services
Now that we’ve defined our metrics, let’s implement them in our service:
package main import ( "time" "github.com/yourusername/order-processing-system/metrics" ) func createOrder(order Order) error { startTime := time.Now() // Order creation logic... metrics.OrdersCreated.Inc() metrics.OrderProcessingTime.Observe(time.Since(startTime).Seconds()) metrics.OrderStatusGauge.WithLabelValues("pending").Inc() return nil } func processPayment(payment Payment) error { // Payment processing logic... if paymentSuccessful { metrics.PaymentProcessed.WithLabelValues("success").Inc() } else { metrics.PaymentProcessed.WithLabelValues("failure").Inc() } return nil } func updateInventory(item Item) error { // Inventory update logic... metrics.InventoryUpdates.Inc() return nil } func arrangeShipping(order Order) error { startTime := time.Now() // Shipping arrangement logic... metrics.ShippingArrangementTime.Observe(time.Since(startTime).Seconds()) return nil }
Best Practices for Naming and Labeling Metrics
When naming and labeling metrics, consider these best practices:
- Use a consistent naming scheme (e.g.,
_ _ ) - Use clear, descriptive names
- Include units in the metric name (e.g., _seconds, _bytes)
- Use labels to differentiate instances of a metric, but be cautious of high cardinality
- Keep the number of labels manageable
Instrumenting Key Components: API Endpoints, Database Operations, Temporal Workflows
For API endpoints, we’ve already implemented basic instrumentation. For database operations, we can add metrics like this:
func (s *Store) GetOrder(ctx context.Context, id int64) (Order, error) { startTime := time.Now() defer func() { metrics.DBOperationDuration.WithLabelValues("GetOrder").Observe(time.Since(startTime).Seconds()) }() // Existing GetOrder logic... }
For Temporal workflows, we can add metrics in our activity implementations:
func ProcessOrderActivity(ctx context.Context, order Order) error { startTime := time.Now() defer func() { metrics.WorkflowActivityDuration.WithLabelValues("ProcessOrder").Observe(time.Since(startTime).Seconds()) }() // Existing ProcessOrder logic... }
5. Creating Dashboards with Grafana
Now that we have our metrics set up, let’s visualize them using Grafana.
Installing and Configuring Grafana
First, let’s add Grafana to our docker-compose.yml:
services: # ... other services ... grafana: image: grafana/grafana:8.2.2 ports: - 3000:3000 volumes: - grafana_data:/var/lib/grafana volumes: # ... other volumes ... grafana_data: {}
Connecting Grafana to Our Prometheus Data Source
- Access Grafana at http://localhost:3000 (default credentials are admin/admin)
- Go to Configuration > Data Sources
- Click “Add data source” and select Prometheus
- Set the URL to http://prometheus:9090 (this is the Docker service name)
- Click “Save & Test”
Designing Effective Dashboards for Our Order Processing System
Let’s create a dashboard for our order processing system:
- Click “Create” > “Dashboard”
- Add a new panel
For our first panel, let’s create a graph of order creation rate:
- In the query editor, enter: rate(orders_created_total[5m])
- Set the panel title to “Order Creation Rate”
- Under Settings, set the unit to “orders/second”
Let’s add another panel for order processing time:
- Add a new panel
- Query: histogram_quantile(0.95, rate(order_processing_seconds_bucket[5m]))
- Title: “95th Percentile Order Processing Time”
- Unit: “seconds”
For order status distribution:
- Add a new panel
- Query: orders_by_status
- Visualization: Pie Chart
- Title: “Order Status Distribution”
Continue adding panels for other metrics we’ve defined.
Implementing Variable Templating for Flexible Dashboards
Grafana allows us to create variables that can be used across the dashboard. Let’s create a variable for time range:
- Go to Dashboard Settings > Variables
- Click “Add variable”
- Name: time_range
- Type: Interval
- Values: 5m,15m,30m,1h,6h,12h,24h,7d
Now we can use this in our queries like this: rate(orders_created_total[$time_range])
Best Practices for Dashboard Design and Organization
- Group related panels together
- Use consistent color schemes
- Include a description for each panel
- Use appropriate visualizations for each metric type
- Consider creating separate dashboards for different aspects of the system (e.g., Orders, Inventory, Shipping)
In the next section, we’ll set up alerting rules to notify us of potential issues in our system.
6. Implementing Alerting Rules
Now that we have our metrics and dashboards set up, let’s implement alerting to proactively notify us of potential issues in our system.
Designing an Alerting Strategy for Our System
When designing alerts, consider the following principles:
- Alert on symptoms, not causes
- Ensure alerts are actionable
- Avoid alert fatigue by only alerting on critical issues
- Use different severity levels for different types of issues
For our order processing system, we might want to alert on:
- High error rate in order processing
- Slow order processing time
- Unusual spike or drop in order creation rate
- Low inventory levels
- High rate of payment failures
Implementing Prometheus Alerting Rules
Let’s create an alerts.yml file in our Prometheus configuration directory:
groups: - name: order_processing_alerts rules: - alert: HighOrderProcessingErrorRate expr: rate(order_processing_errors_total[5m]) / rate(orders_created_total[5m]) > 0.05 for: 5m labels: severity: critical annotations: summary: High order processing error rate description: "Error rate is over the last 5 minutes" - alert: SlowOrderProcessing expr: histogram_quantile(0.95, rate(order_processing_seconds_bucket[5m])) > 300 for: 10m labels: severity: warning annotations: summary: Slow order processing description: "95th percentile of order processing time is over the last 5 minutes" - alert: UnusualOrderRate expr: abs(rate(orders_created_total[1h]) - rate(orders_created_total[1h] offset 1d)) > (rate(orders_created_total[1h] offset 1d) * 0.3) for: 30m labels: severity: warning annotations: summary: Unusual order creation rate description: "Order creation rate has changed by more than 30% compared to the same time yesterday" - alert: LowInventory expr: inventory_level 0.1 for: 15m labels: severity: critical annotations: summary: High payment failure rate description: "Payment failure rate is over the last 15 minutes"
Update your prometheus.yml to include this alerts file:
rule_files: - "alerts.yml"
Setting Up Alertmanager for Alert Routing and Grouping
Now, let’s set up Alertmanager to handle our alerts. Add Alertmanager to your docker-compose.yml:
services: # ... other services ... alertmanager: image: prom/alertmanager:v0.23.0 ports: - 9093:9093 volumes: - ./alertmanager:/etc/alertmanager command: - '--config.file=/etc/alertmanager/alertmanager.yml'
Create an alertmanager.yml in the ./alertmanager directory:
route: group_by: ['alertname'] group_wait: 30s group_interval: 5m repeat_interval: 1h receiver: 'email-notifications' receivers: - name: 'email-notifications' email_configs: - to: 'team@example.com' from: 'alertmanager@example.com' smarthost: 'smtp.example.com:587' auth_username: 'alertmanager@example.com' auth_identity: 'alertmanager@example.com' auth_password: 'password'
Update your prometheus.yml to point to Alertmanager:
alerting: alertmanagers: - static_configs: - targets: - alertmanager:9093
Configuring Notification Channels
In the Alertmanager configuration above, we’ve set up email notifications. You can also configure other channels like Slack, PagerDuty, or custom webhooks.
Implementing Alert Severity Levels and Escalation Policies
In our alerts, we’ve used severity labels. We can use these in Alertmanager to implement different routing or notification strategies based on severity:
route: group_by: ['alertname'] group_wait: 30s group_interval: 5m repeat_interval: 1h receiver: 'email-notifications' routes: - match: severity: critical receiver: 'pagerduty-critical' - match: severity: warning receiver: 'slack-warnings' receivers: - name: 'email-notifications' email_configs: - to: 'team@example.com' - name: 'pagerduty-critical' pagerduty_configs: - service_key: '<your-pagerduty-service-key>' - name: 'slack-warnings' slack_configs: - api_url: '<your-slack-webhook-url>' channel: '#alerts' </your-slack-webhook-url></your-pagerduty-service-key>
7. Monitoring Database Performance
Monitoring database performance is crucial for maintaining a responsive and reliable system. Let’s set up monitoring for our PostgreSQL database.
Implementing the Postgres Exporter for Prometheus
First, add the Postgres exporter to your docker-compose.yml:
services: # ... other services ... postgres_exporter: image: wrouesnel/postgres_exporter:latest environment: DATA_SOURCE_NAME: "postgresql://user:password@postgres:5432/dbname?sslmode=disable" ports: - 9187:9187
Make sure to replace user, password, and dbname with your actual PostgreSQL credentials.
Key Metrics to Monitor for Postgres Performance
Some important PostgreSQL metrics to monitor include:
- Number of active connections
- Database size
- Query execution time
- Cache hit ratio
- Replication lag (if using replication)
- Transaction rate
- Tuple operations (inserts, updates, deletes)
Creating a Database Performance Dashboard in Grafana
Let’s create a new dashboard for database performance:
- Create a new dashboard in Grafana
- Add a panel for active connections:
- Query: pg_stat_activity_count{datname="your_database_name"}
- Title: “Active Connections”
- Add a panel for database size:
- Query: pg_database_size_bytes{datname="your_database_name"}
- Title: “Database Size”
- Unit: bytes(IEC)
- Add a panel for query execution time:
- Query: rate(pg_stat_database_xact_commit{datname="your_database_name"}[5m]) + rate(pg_stat_database_xact_rollback{datname="your_database_name"}[5m])
- Title: “Transactions per Second”
- Add a panel for cache hit ratio:
- Query: pg_stat_database_blks_hit{datname="your_database_name"} / (pg_stat_database_blks_hit{datname="your_database_name"} + pg_stat_database_blks_read{datname="your_database_name"})
- Title: “Cache Hit Ratio”
Setting Up Alerts for Database Issues
Let’s add some database-specific alerts to our alerts.yml:
- alert: HighDatabaseConnections expr: pg_stat_activity_count > 100 for: 5m labels: severity: warning annotations: summary: High number of database connections description: "There are active database connections" - alert: LowCacheHitRatio expr: pg_stat_database_blks_hit / (pg_stat_database_blks_hit + pg_stat_database_blks_read) <h2> 8. Monitoring Temporal Workflows </h2> <p>Monitoring Temporal workflows is essential for ensuring the reliability and performance of our order processing system.</p> <h3> Implementing Temporal Metrics in Our Go Services </h3> <p>Temporal provides a metrics client that we can use to expose metrics to Prometheus. Let’s update our Temporal worker to include metrics:<br> </p> <pre class="brush:php;toolbar:false">import ( "go.temporal.io/sdk/client" "go.temporal.io/sdk/worker" "go.temporal.io/sdk/contrib/prometheus" ) func main() { // ... other setup ... // Create Prometheus metrics handler metricsHandler := prometheus.NewPrometheusMetricsHandler() // Create Temporal client with metrics c, err := client.NewClient(client.Options{ MetricsHandler: metricsHandler, }) if err != nil { log.Fatalln("Unable to create Temporal client", err) } defer c.Close() // Create worker with metrics w := worker.New(c, "order-processing-task-queue", worker.Options{ MetricsHandler: metricsHandler, }) // ... register workflows and activities ... // Run the worker err = w.Run(worker.InterruptCh()) if err != nil { log.Fatalln("Unable to start worker", err) } }
Key Metrics to Monitor for Temporal Workflows
Important Temporal metrics to monitor include:
- Workflow start rate
- Workflow completion rate
- Workflow execution time
- Activity success/failure rate
- Activity execution time
- Task queue latency
Creating a Temporal Workflow Dashboard in Grafana
Let’s create a dashboard for Temporal workflows:
- Create a new dashboard in Grafana
- Add a panel for workflow start rate:
- Query: rate(temporal_workflow_start_total[5m])
- Title: “Workflow Start Rate”
- Add a panel for workflow completion rate:
- Query: rate(temporal_workflow_completed_total[5m])
- Title: “Workflow Completion Rate”
- Add a panel for workflow execution time:
- Query: histogram_quantile(0.95, rate(temporal_workflow_execution_time_bucket[5m]))
- Title: “95th Percentile Workflow Execution Time”
- Unit: seconds
- Add a panel for activity success rate:
- Query: rate(temporal_activity_success_total[5m]) / (rate(temporal_activity_success_total[5m]) + rate(temporal_activity_fail_total[5m]))
- Title: “Activity Success Rate”
Setting Up Alerts for Workflow Issues
Let’s add some Temporal-specific alerts to our alerts.yml:
- alert: HighWorkflowFailureRate expr: rate(temporal_workflow_failed_total[15m]) / rate(temporal_workflow_completed_total[15m]) > 0.05 for: 15m labels: severity: critical annotations: summary: High workflow failure rate description: "Workflow failure rate is over the last 15 minutes" - alert: LongRunningWorkflow expr: histogram_quantile(0.95, rate(temporal_workflow_execution_time_bucket[1h])) > 3600 for: 30m labels: severity: warning annotations: summary: Long-running workflows detected description: "95th percentile of workflow execution time is over 1 hour"
These alerts will help you detect issues with your Temporal workflows, such as high failure rates or unexpectedly long-running workflows.
In the next sections, we’ll cover some advanced Prometheus techniques and discuss testing and validation of our monitoring setup.
9. Advanced Prometheus Techniques
As our monitoring system grows more complex, we can leverage some advanced Prometheus techniques to improve its efficiency and capabilities.
Using Recording Rules for Complex Queries and Aggregations
Recording rules allow you to precompute frequently needed or computationally expensive expressions and save their result as a new set of time series. This can significantly speed up the evaluation of dashboards and alerts.
Let’s add some recording rules to our Prometheus configuration. Create a rules.yml file:
groups: - name: example_recording_rules interval: 5m rules: - record: job:order_processing_rate:5m expr: rate(orders_created_total[5m]) - record: job:order_processing_error_rate:5m expr: rate(order_processing_errors_total[5m]) / rate(orders_created_total[5m]) - record: job:payment_success_rate:5m expr: rate(payments_processed_total{status="success"}[5m]) / rate(payments_processed_total[5m])
Add this file to your Prometheus configuration:
rule_files: - "alerts.yml" - "rules.yml"
Now you can use these precomputed metrics in your dashboards and alerts, which can be especially helpful for complex queries that you use frequently.
Implementing Push Gateway for Batch Jobs and Short-Lived Processes
The Pushgateway allows you to push metrics from jobs that can’t be scraped, such as batch jobs or serverless functions. Let’s add a Pushgateway to our docker-compose.yml:
services: # ... other services ... pushgateway: image: prom/pushgateway ports: - 9091:9091
Now, you can push metrics to the Pushgateway from your batch jobs or short-lived processes. Here’s an example using the Go client:
import ( "github.com/prometheus/client_golang/prometheus" "github.com/prometheus/client_golang/prometheus/push" ) func runBatchJob() { // Define a counter for the batch job batchJobCounter := prometheus.NewCounter(prometheus.CounterOpts{ Name: "batch_job_processed_total", Help: "Total number of items processed by the batch job", }) // Run your batch job and update the counter // ... // Push the metric to the Pushgateway pusher := push.New("http://pushgateway:9091", "batch_job") pusher.Collector(batchJobCounter) if err := pusher.Push(); err != nil { log.Printf("Could not push to Pushgateway: %v", err) } }
Don’t forget to add the Pushgateway as a target in your Prometheus configuration:
scrape_configs: # ... other configs ... - job_name: 'pushgateway' static_configs: - targets: ['pushgateway:9091']
Federated Prometheus Setups for Large-Scale Systems
For large-scale systems, you might need to set up Prometheus federation, where one Prometheus server scrapes data from other Prometheus servers. This allows you to aggregate metrics from multiple Prometheus instances.
Here’s an example configuration for a federated Prometheus setup:
scrape_configs: - job_name: 'federate' scrape_interval: 15s honor_labels: true metrics_path: '/federate' params: 'match[]': - '{job="order_processing_api"}' - '{job="postgres_exporter"}' static_configs: - targets: - 'prometheus-1:9090' - 'prometheus-2:9090'
This configuration allows a higher-level Prometheus server to scrape specific metrics from other Prometheus servers.
Using Exemplars for Tracing Integration
Exemplars allow you to link metrics to trace data, providing a way to drill down from a high-level metric to a specific trace. This is particularly useful when integrating Prometheus with distributed tracing systems like Jaeger or Zipkin.
To use exemplars, you need to enable them in your Prometheus configuration:
global: scrape_interval: 15s evaluation_interval: 15s exemplar_storage: enable: true
Then, when instrumenting your code, you can add exemplars to your metrics:
import ( "github.com/prometheus/client_golang/prometheus" "github.com/prometheus/client_golang/prometheus/promauto" ) var ( orderProcessingDuration = promauto.NewHistogramVec( prometheus.HistogramOpts{ Name: "order_processing_duration_seconds", Help: "Duration of order processing in seconds", Buckets: prometheus.DefBuckets, }, []string{"status"}, ) ) func processOrder(order Order) { start := time.Now() // Process the order... duration := time.Since(start) orderProcessingDuration.WithLabelValues(order.Status).Observe(duration.Seconds(), prometheus.Labels{ "traceID": getCurrentTraceID(), }, ) }
This allows you to link from a spike in order processing duration directly to the trace of a slow order, greatly aiding in debugging and performance analysis.
10. Testing and Validation
Ensuring the reliability of your monitoring system is crucial. Let’s explore some strategies for testing and validating our Prometheus setup.
Unit Testing Metric Instrumentation
When unit testing your Go code, you can use the prometheus/testutil package to verify that your metrics are being updated correctly:
import ( "testing" "github.com/prometheus/client_golang/prometheus/testutil" ) func TestOrderProcessing(t *testing.T) { // Process an order processOrder(Order{ID: 1, Status: "completed"}) // Check if the metric was updated expected := ` # HELP order_processing_duration_seconds Duration of order processing in seconds # TYPE order_processing_duration_seconds histogram order_processing_duration_seconds_bucket{status="completed",le="0.005"} 1 order_processing_duration_seconds_bucket{status="completed",le="0.01"} 1 # ... other buckets ... order_processing_duration_seconds_sum{status="completed"} 0.001 order_processing_duration_seconds_count{status="completed"} 1 ` if err := testutil.CollectAndCompare(orderProcessingDuration, strings.NewReader(expected)); err != nil { t.Errorf("unexpected collecting result:\n%s", err) } }
Integration Testing for Prometheus Scraping
To test that Prometheus is correctly scraping your metrics, you can set up an integration test that starts your application, waits for Prometheus to scrape it, and then queries Prometheus to verify the metrics:
func TestPrometheusIntegration(t *testing.T) { // Start your application go startApp() // Wait for Prometheus to scrape (adjust the sleep time as needed) time.Sleep(30 * time.Second) // Query Prometheus client, err := api.NewClient(api.Config{ Address: "http://localhost:9090", }) if err != nil { t.Fatalf("Error creating client: %v", err) } v1api := v1.NewAPI(client) ctx, cancel := context.WithTimeout(context.Background(), 10*time.Second) defer cancel() result, warnings, err := v1api.Query(ctx, "order_processing_duration_seconds_count", time.Now()) if err != nil { t.Fatalf("Error querying Prometheus: %v", err) } if len(warnings) > 0 { t.Logf("Warnings: %v", warnings) } // Check the result if result.(model.Vector).Len() == 0 { t.Errorf("Expected non-empty result") } }
Load Testing and Observing Metrics Under Stress
It’s important to verify that your monitoring system performs well under load. You can use tools like hey or vegeta to generate load on your system while observing your metrics:
hey -n 10000 -c 100 http://localhost:8080/orders
While the load test is running, observe your Grafana dashboards and check that your metrics are updating as expected and that Prometheus is able to keep up with the increased load.
Validating Alerting Rules and Notification Channels
To test your alerting rules, you can temporarily adjust the thresholds to trigger alerts, or use Prometheus’s API to manually fire alerts:
curl -H "Content-Type: application/json" -d '{ "alerts": [ { "labels": { "alertname": "HighOrderProcessingErrorRate", "severity": "critical" }, "annotations": { "summary": "High order processing error rate" } } ] }' http://localhost:9093/api/v1/alerts
This will send a test alert to your Alertmanager, allowing you to verify that your notification channels are working correctly.
11. Challenges and Considerations
As you implement and scale your monitoring system, keep these challenges and considerations in mind:
Managing Cardinality in High-Dimensional Data
High cardinality can lead to performance issues in Prometheus. Be cautious when adding labels to metrics, especially labels with many possible values (like user IDs or IP addresses). Instead, consider using histogram metrics or reducing the cardinality by grouping similar values.
Scaling Prometheus for Large-Scale Systems
For large-scale systems, consider:
- Using the Pushgateway for batch jobs
- Implementing federation for large-scale setups
- Using remote storage solutions for long-term storage of metrics
Ensuring Monitoring System Reliability and Availability
Your monitoring system is critical infrastructure. Consider:
- Implementing high availability for Prometheus and Alertmanager
- Monitoring your monitoring system (meta-monitoring)
- Regularly backing up your Prometheus data
Security Considerations for Metrics and Alerting
Ensure that:
- Access to Prometheus and Grafana is properly secured
- Sensitive information is not exposed in metrics or alerts
- TLS is used for all communications in your monitoring stack
Dealing with Transient Issues and Flapping Alerts
To reduce alert noise:
- Use appropriate time windows in your alert rules
- Implement alert grouping in Alertmanager
- Consider using alert inhibition for related alerts
12. Next Steps and Preview of Part 5
In this post, we’ve covered comprehensive monitoring and alerting for our order processing system using Prometheus and Grafana. We’ve set up custom metrics, created informative dashboards, implemented alerting, and explored advanced techniques and considerations.
In the next part of our series, we’ll focus on distributed tracing and logging. We’ll cover:
- Implementing distributed tracing with OpenTelemetry
- Setting up centralized logging with the ELK stack
- Correlating logs, traces, and metrics for effective debugging
- Implementing log aggregation and analysis
- Best practices for logging in a microservices architecture
Stay tuned as we continue to enhance our order processing system, focusing next on gaining deeper insights into our distributed system’s behavior and performance!
Need Help?
Are you facing challenging problems, or need an external perspective on a new idea or project? I can help! Whether you're looking to build a technology proof of concept before making a larger investment, or you need guidance on difficult issues, I'm here to assist.
Services Offered:
- Problem-Solving: Tackling complex issues with innovative solutions.
- Consultation: Providing expert advice and fresh viewpoints on your projects.
- Proof of Concept: Developing preliminary models to test and validate your ideas.
If you're interested in working with me, please reach out via email at hungaikevin@gmail.com.
Let's turn your challenges into opportunities!
以上是實施訂單處理系統:零件監控與警報的詳細內容。更多資訊請關注PHP中文網其他相關文章!
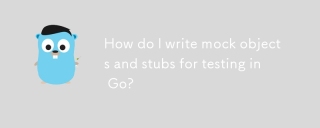
本文演示了創建模擬和存根進行單元測試。 它強調使用接口,提供模擬實現的示例,並討論最佳實踐,例如保持模擬集中並使用斷言庫。 文章
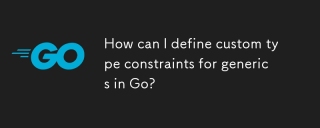
本文探討了GO的仿製藥自定義類型約束。 它詳細介紹了界面如何定義通用功能的最低類型要求,從而改善了類型的安全性和代碼可重複使用性。 本文還討論了局限性和最佳實踐
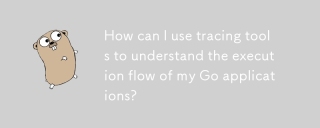
本文使用跟踪工具探討了GO應用程序執行流。 它討論了手冊和自動儀器技術,比較諸如Jaeger,Zipkin和Opentelemetry之類的工具,並突出顯示有效的數據可視化
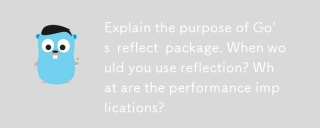
本文討論了GO的反思軟件包,用於運行時操作代碼,對序列化,通用編程等有益。它警告性能成本,例如較慢的執行和更高的內存使用,建議明智的使用和最佳
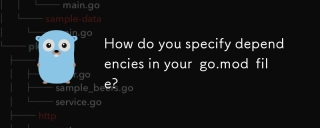
本文討論了通過go.mod,涵蓋規範,更新和衝突解決方案管理GO模塊依賴關係。它強調了最佳實踐,例如語義版本控制和定期更新。
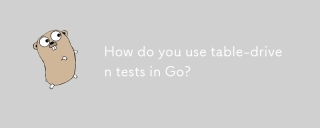
本文討論了GO中使用表驅動的測試,該方法使用測試用例表來測試具有多個輸入和結果的功能。它突出了諸如提高的可讀性,降低重複,可伸縮性,一致性和A


熱AI工具

Undresser.AI Undress
人工智慧驅動的應用程序,用於創建逼真的裸體照片

AI Clothes Remover
用於從照片中去除衣服的線上人工智慧工具。

Undress AI Tool
免費脫衣圖片

Clothoff.io
AI脫衣器

AI Hentai Generator
免費產生 AI 無盡。

熱門文章

熱工具
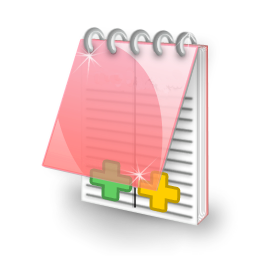
EditPlus 中文破解版
體積小,語法高亮,不支援程式碼提示功能

MantisBT
Mantis是一個易於部署的基於Web的缺陷追蹤工具,用於幫助產品缺陷追蹤。它需要PHP、MySQL和一個Web伺服器。請查看我們的演示和託管服務。

Safe Exam Browser
Safe Exam Browser是一個安全的瀏覽器環境,安全地進行線上考試。該軟體將任何電腦變成一個安全的工作站。它控制對任何實用工具的訪問,並防止學生使用未經授權的資源。
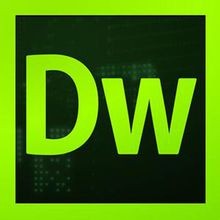
Dreamweaver CS6
視覺化網頁開發工具

PhpStorm Mac 版本
最新(2018.2.1 )專業的PHP整合開發工具