AI agents are designed to act autonomously, solving problems and executing tasks in dynamic environments. A key feature in Autogen, enabling their adaptability is AutoGen’s code executors. This feature along with LLMs enables AI agents to generate, evaluate, and execute code in real-time. This capability bridges the gap between static AI models and actionable intelligence. By automating workflows, performing data analysis, and debugging complex systems, it transforms agents from mere thinkers into effective doers. In this article, we will learn more about code executors in AutoGen and how to implement them.
Table of Contents
- Types of Code Executors in AutoGen
- How to Build AI Agents with Code Executors in AutoGen?
- Pre-requisites
- Building an AI Agent Using Command Line Executor
- Building an ML Model Using Jupyter Code Executor
- Building an AI Agent Using Custom Executor
- Conclusion
- Frequently Asked Questions
Types of Code Executors in AutoGen
AutoGen has three kinds of code executors that can be used for different purposes.
- Command Line Executor: It allows AI agents to run the code in the command line. It will save each code block to a separate file and execute that file. This executor is ideal for automating tasks like file management, script execution, or handling external tools. It provides flexibility and low-level control in a workflow.
- Jupyter Code Executor: It enables agents to execute Python code within a Jupyter-like environment. Here, you can define variables in one code block and reuse them in subsequent blocks. One advantage of this setup is that when an error occurs, only the specific block of code with the error needs to be re-executed, rather than the entire script.
- Custom Code Executor: It gives developers the ability to create specialized code execution logic. For example, the custom code executor can access variables defined in the environment without explicitly providing them to the LLM.
These Code Executors can be run on both the host machine (local) as well as the Docker containers.
Also Read: 4 Steps to Build Multi-Agent Nested Chats with AutoGen
How to Build AI Agents with Code Executors in AutoGen?
Now let’s learn how you can use these different code executors in AutoGen:
Pre-requisites
Before building AI agents, ensure you have the necessary API keys for the required LLMs.
Load the .env file with the API keys needed.
from dotenv import load_dotenv load_dotenv(./env)
Key Libraries Required
autogen-agentchat – 0.2.38
jupyter_kernel_gateway-3.0.1
Building an AI Agent Using Command Line Executor
Let’s build an AI agent to know the offers and discounts available on an e-commerce website using the command line executor. Here are the steps to follow.
1. Import the necessary libraries.
from autogen import ConversableAgent, AssistantAgent, UserProxyAgent from autogen.coding import LocalCommandLineCodeExecutor, DockerCommandLineCodeExecutor
2. Define the agents.
user_proxy = UserProxyAgent( name="User", llm_config=False, is_termination_msg=lambda msg: msg.get("content") is not None and "TERMINATE" in msg["content"], human_input_mode="TERMINATE", code_execution_config=False ) code_writer_agent = ConversableAgent( name="CodeWriter", system_message="""You are a Python developer. You use your coding skill to solve problems. Once the task is done, returns 'TERMINATE'.""", llm_config={"config_list": [{"model": "gpt-4o-mini"}]}, ) local_executor = LocalCommandLineCodeExecutor( timeout=15, work_dir='./code files') local_executor_agent = ConversableAgent( "local_executor_agent", llm_config=False, code_execution_config={"executor": local_executor}, human_input_mode="ALWAYS", )
We are using the ‘local_executor’ in the code_execution_config of the local_executor_agent.
3. Define the messages which are used to initialize the chat.
messages = ["""To check whether there are any offers or discounts available on a given e-commerce website - https://www.flipkart.com/ Follow these steps, 1. download the html page of the given URL 2. we only need html content, so remove any CSS, JavaScript, and Image tags content 3. save the remaining html content. """ , "read the text and list all the offers and discounts available"] # Intialize the chat chat_result = local_executor_agent.initiate_chat( code_writer_agent, message=messages[0], )
It will ask for human input after each message from the codeWriter agent. You just need to press the ‘Enter’ key to execute the code written by the agent. We can also any further instructions if there is any problem with the code.
Here are the questions we have asked and the output at the end.
As we can see, with the mentioned questions, we can get a list of offers and discounts from an e-commerce website.
Also Read: Hands-on Guide to Building Multi-Agent Chatbots with AutoGen
Building an ML Model Using Jupyter Code Executor
By using this, we can access the variables defined in one code block from another code block, unlike the command line executor.
Now, let’s try to build an ML model using this.
1. Import the additional methods.
from autogen.coding.jupyter import LocalJupyterServer, DockerJupyterServer, JupyterCodeExecutor from pathlib import Path
2. Initialize the jupyter server and output directory.
server = LocalJupyterServer() output_dir = Path("coding") output_dir.mkdir()
Note that LocalJupyterServer may not function on Windows due to a bug. In this case, you can use the DockerJupyterServer instead or use the EmbeddedIPythonCodeExecutor.
3. Define the executor agent and writer agent with a custom system message.
jupyter_executor_agent = ConversableAgent( name="jupyter_executor_agent", llm_config=False, code_execution_config={ "executor": JupyterCodeExecutor(server, output_dir=output_dir), }, human_input_mode="ALWAYS", ) code_writer_system_message = """ You have been given coding capability to solve tasks using Python code in a stateful IPython kernel. You are responsible for writing the code, and the user is responsible for executing the code. When you write Python code, put the code in a markdown code block with the language set to Python. For example: ```python x = 3 ``` You can use the variable `x` in subsequent code blocks. ```python print(x) ``` Always use print statements for the output of the code. Write code incrementally and leverage the statefulness of the kernel to avoid repeating code. Import libraries in a separate code block. Define a function or a class in a separate code block. Run code that produces output in a separate code block. Run code that involves expensive operations like download, upload, and call external APIs in a separate code block. When your code produces an output, the output will be returned to you. Because you have limited conversation memory, if your code creates an image, the output will be a path to the image instead of the image itself.""" code_writer_agent = ConversableAgent( "code_writer", system_message=code_writer_system_message, llm_config={"config_list": [{"model": "gpt-4o"}]}, human_input_mode="TERMINATE", )
4. Define the initial message and initialize the chat
message = "read the datasets/user_behavior_dataset.csv and print what the data is about" chat_result = jupyter_executor_agent.initiate_chat( code_writer_agent, message=message, ) # Once the chat is completed we can stop the server. server.stop()
5. Once the chat is completed we can stop the server.
We can print the messages as follows
for chat in chat_result.chat_history[:]: if chat['name'] == 'code_writer' and 'TERMINATE' not in chat['content']: print("--------agent-----------") print(chat['content']) if chat['name'] == 'jupyter_executor_agent' and 'exitcode' not in chat['content']: print("--------user------------") print(chat['content'])
Here’s the sample
As we can see, we can get the code generated by the agent and also the results after executing the code.
Also Read: Building Agentic Chatbots Using AutoGen
Building an AI Agent Using Custom Executor
Now, let’s try to create a custom executor that can run the code in the same jupyter notebook where we are creating this executor. So, we can read a CSV file, and then ask an agent to build an ML model on the already imported file.
Here’s how we’ll do it.
1. Import the necessary libraries.
import pandas as pd from typing import List from IPython import get_ipython from autogen.coding import CodeBlock, CodeExecutor, CodeExtractor, CodeResult, MarkdownCodeExtractor
2. Define the executor that can extract and run the code from jupyter cells.
class NotebookExecutor(CodeExecutor): @property def code_extractor(self) -> CodeExtractor: # Extact code from markdown blocks. return MarkdownCodeExtractor() def __init__(self) -> None: # Get the current IPython instance running in this notebook. self._ipython = get_ipython() def execute_code_blocks(self, code_blocks: List[CodeBlock]) -> CodeResult: log = "" for code_block in code_blocks: result = self._ipython.run_cell("%%capture --no-display cap\n" + code_block.code) log += self._ipython.ev("cap.stdout") log += self._ipython.ev("cap.stderr") if result.result is not None: log += str(result.result) exitcode = 0 if result.success else 1 if result.error_before_exec is not None: log += f"\n{result.error_before_exec}" exitcode = 1 if result.error_in_exec is not None: log += f"\n{result.error_in_exec}" exitcode = 1 if exitcode != 0: break return CodeResult(exit_code=exitcode, output=log)
3. Define the agents.
code_writer_agent = ConversableAgent( name="CodeWriter", system_message="You are a helpful AI assistant.\n" "You use your coding skill to solve problems.\n" "You have access to a IPython kernel to execute Python code.\n" "You can suggest Python code in Markdown blocks, each block is a cell.\n" "The code blocks will be executed in the IPython kernel in the order you suggest them.\n" "All necessary libraries have already been installed.\n" "Add return or print statements to the code to get the output\n" "Once the task is done, returns 'TERMINATE'.", llm_config={"config_list": [{"model": "gpt-4o-mini"}]}, ) code_executor_agent = ConversableAgent( name="CodeExecutor", llm_config=False, code_execution_config={"executor": NotebookExecutor()}, is_termination_msg=lambda msg: "TERMINATE" in msg.get("content", "").strip().upper(), human_input_mode="ALWAYS" )
4. Read the file and initiate the chat with the file.
df = pd.read_csv('datasets/mountains_vs_beaches_preferences.csv') chat_result = code_executor_agent.initiate_chat( code_writer_agent, message="What are the column names in the dataframe defined above as df?", )
5. We can print the chat history as follows:
for chat in chat_result.chat_history[:]: if chat['name'] == 'CodeWriter' and 'TERMINATE' not in chat['content']: print("--------agent-----------") print(chat['content']) if chat['name'] == 'CodeExecutor' and 'exitcode' not in chat['content']: print("--------user------------") print(chat['content'])
As we can see again, we can get the code generated by the agent and also the results after executing the code.
Conclusion
AutoGen’s code executors provide flexibility and functionality for AI agents to perform real-world tasks. The command line executor enables script execution, while the Jupyter code executor supports iterative development. Custom executors, on the other hand, allow developers to create tailored workflows.
These tools empower AI agents to transition from problem solvers to solution implementers. Developers can use these features to build intelligent systems that deliver actionable insights and automate complex processes.
Frequently Asked Questions
Q1. What is the primary purpose of Code Executors in AutoGen?A. Code Executors in AutoGen allow AI agents to generate, execute, and evaluate code in real time. This enables agents to automate tasks, perform data analysis, debug systems, and implement dynamic workflows.
Q2. What are the differences between Command Line and Jupyter Code Executors?A. The Command Line Executor saves and executes code as separate files, ideal for tasks like file management and script execution. The Jupyter Code Executor operates in a stateful environment, allowing reuse of variables and selective re-execution of code blocks, making it more suitable for iterative coding tasks like building ML models.
Q3. Can Code Executors be used with Docker containers?A. Yes, both the Command Line Executor and Jupyter Code Executor can be configured to run on Docker containers, providing a flexible environment for execution.
Q4. What is the advantage of using a Custom Code Executor?A. Custom Code Executors allow developers to define specialized execution logic, such as running code within the same Jupyter notebook. This is useful for tasks requiring a high level of integration or customization.
Q5. What are the prerequisites for using Code Executors in AutoGen?A. Before using Code Executors, ensure you have the necessary API keys for your preferred LLMs. You should also have the required libraries, such as `autogen-agentchat` and `jupyter_kernel_gateway`, installed in your environment.
Atas ialah kandungan terperinci Menyelesaikan tugas kompleks menggunakan pelaksana kod dalam autogen. Untuk maklumat lanjut, sila ikut artikel berkaitan lain di laman web China PHP!
![Tidak boleh menggunakan chatgpt! Menjelaskan sebab dan penyelesaian yang boleh diuji dengan segera [terbaru 2025]](https://img.php.cn/upload/article/001/242/473/174717025174979.jpg?x-oss-process=image/resize,p_40)
Chatgpt tidak boleh diakses? Artikel ini menyediakan pelbagai penyelesaian praktikal! Ramai pengguna mungkin menghadapi masalah seperti tidak dapat diakses atau tindak balas yang perlahan apabila menggunakan chatgpt setiap hari. Artikel ini akan membimbing anda untuk menyelesaikan masalah ini langkah demi langkah berdasarkan situasi yang berbeza. Punca ketidakmampuan dan penyelesaian masalah awal Chatgpt Pertama, kita perlu menentukan sama ada masalah itu berada di sisi pelayan Openai, atau masalah rangkaian atau peranti pengguna sendiri. Sila ikuti langkah di bawah untuk menyelesaikan masalah: Langkah 1: Periksa status rasmi Openai Lawati halaman Status Openai (status.openai.com) untuk melihat sama ada perkhidmatan ChATGPT berjalan secara normal. Sekiranya penggera merah atau kuning dipaparkan, ini bermakna terbuka
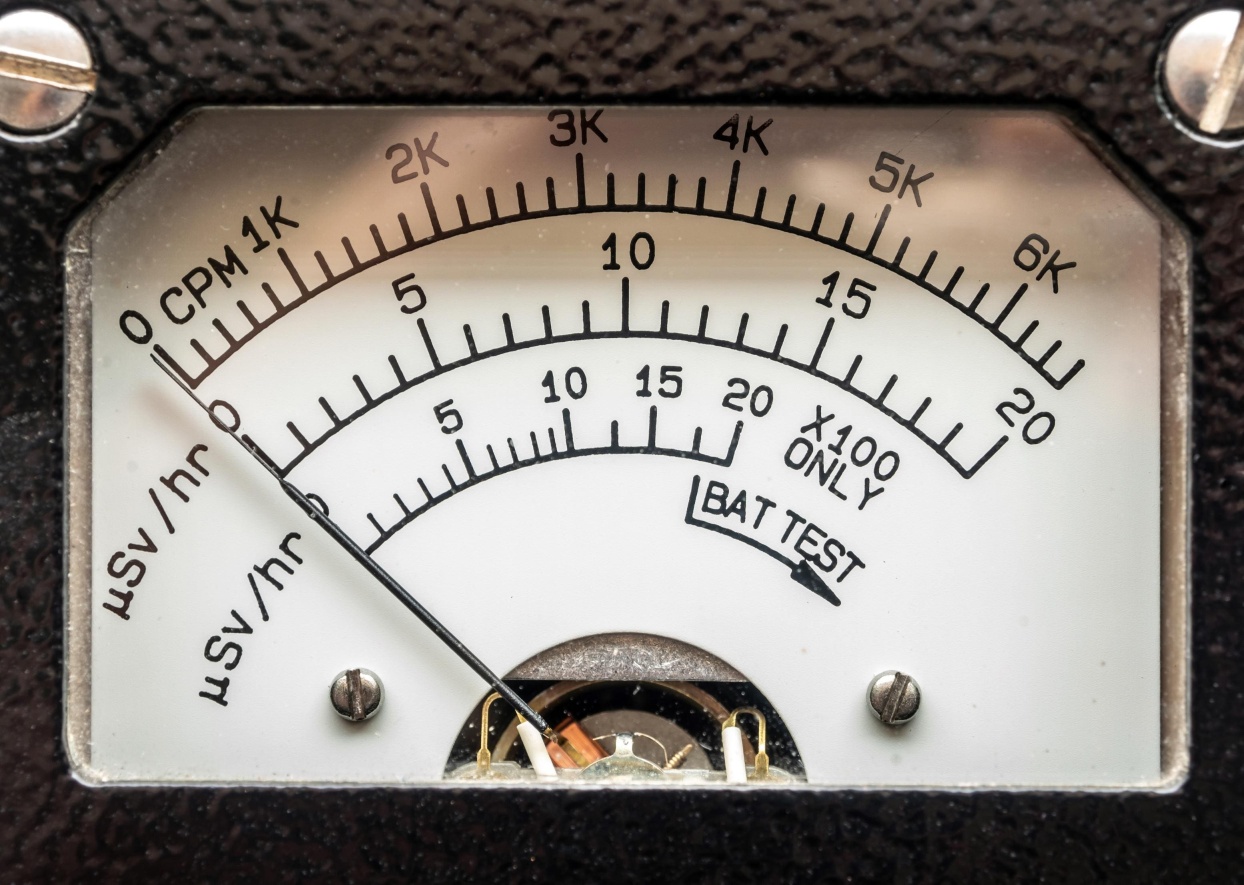
Pada 10 Mei 2025, ahli fizik MIT Max Tegmark memberitahu The Guardian bahawa AI Labs harus mencontohi kalkulus ujian triniti Oppenheimer sebelum melepaskan kecerdasan super buatan. "Penilaian saya ialah 'Compton Constant', kebarangkalian perlumbaan
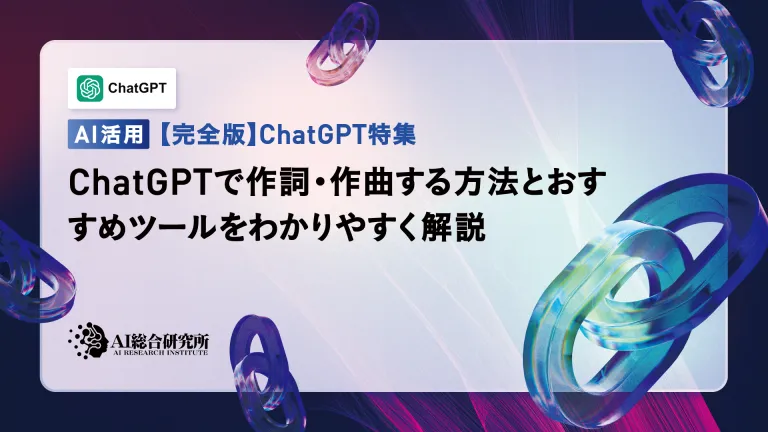
Teknologi penciptaan muzik AI berubah dengan setiap hari berlalu. Artikel ini akan menggunakan model AI seperti CHATGPT sebagai contoh untuk menerangkan secara terperinci bagaimana menggunakan AI untuk membantu penciptaan muzik, dan menerangkannya dengan kes -kes sebenar. Kami akan memperkenalkan bagaimana untuk membuat muzik melalui Sunoai, AI Jukebox pada muka yang memeluk, dan perpustakaan Python Music21. Dengan teknologi ini, semua orang boleh membuat muzik asli dengan mudah. Walau bagaimanapun, perlu diperhatikan bahawa isu hak cipta kandungan AI yang dihasilkan tidak boleh diabaikan, dan anda mesti berhati-hati apabila menggunakannya. Mari kita meneroka kemungkinan AI yang tidak terhingga dalam bidang muzik bersama -sama! Ejen AI terbaru Terbuka "Openai Deep Research" memperkenalkan: [Chatgpt] Ope
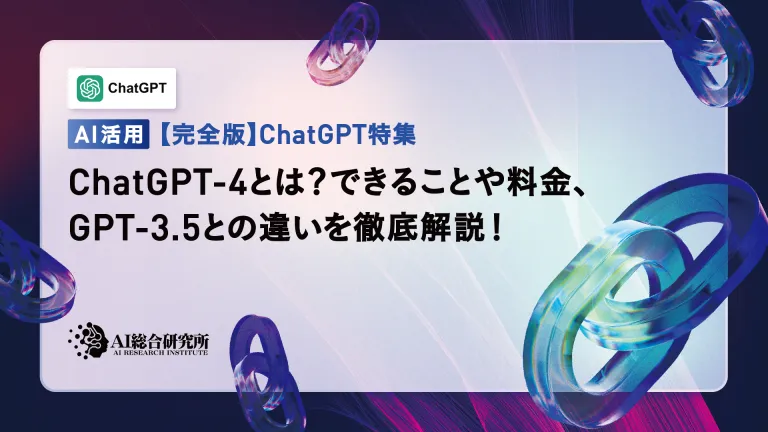
Kemunculan CHATGPT-4 telah memperluaskan kemungkinan aplikasi AI. Berbanding dengan GPT-3.5, CHATGPT-4 telah meningkat dengan ketara. Ia mempunyai keupayaan pemahaman konteks yang kuat dan juga dapat mengenali dan menghasilkan imej. Ia adalah pembantu AI sejagat. Ia telah menunjukkan potensi yang besar dalam banyak bidang seperti meningkatkan kecekapan perniagaan dan membantu penciptaan. Walau bagaimanapun, pada masa yang sama, kita juga harus memberi perhatian kepada langkah berjaga -jaga dalam penggunaannya. Artikel ini akan menerangkan ciri-ciri CHATGPT-4 secara terperinci dan memperkenalkan kaedah penggunaan yang berkesan untuk senario yang berbeza. Artikel ini mengandungi kemahiran untuk memanfaatkan sepenuhnya teknologi AI terkini, sila rujuknya. Ejen AI Terbuka Terbuka, sila klik pautan di bawah untuk butiran "Penyelidikan Deep Openai"
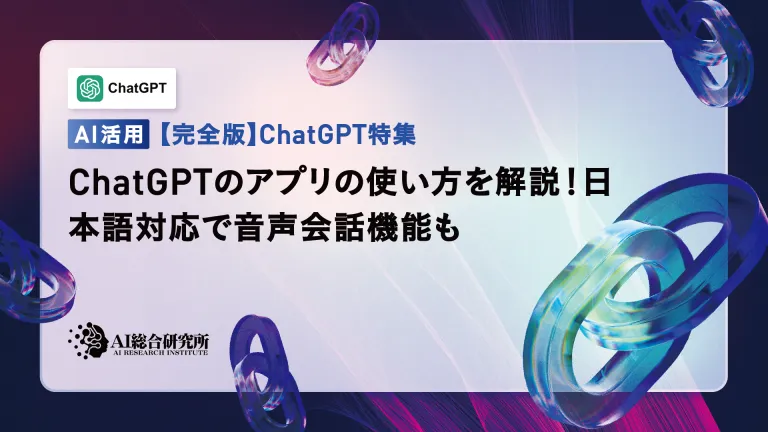
App ChatGPT: Melepaskan kreativiti anda dengan pembantu AI! Panduan pemula Aplikasi CHATGPT adalah pembantu AI yang inovatif yang mengendalikan pelbagai tugas, termasuk menulis, terjemahan, dan menjawab soalan. Ia adalah alat dengan kemungkinan tidak berkesudahan yang berguna untuk aktiviti kreatif dan pengumpulan maklumat. Dalam artikel ini, kami akan menerangkan dengan cara yang mudah difahami untuk pemula, dari cara memasang aplikasi telefon pintar ChATGPT, kepada ciri-ciri yang unik untuk aplikasi seperti fungsi input suara dan plugin, serta mata yang perlu diingat apabila menggunakan aplikasi. Kami juga akan melihat dengan lebih dekat sekatan plugin dan penyegerakan konfigurasi peranti-ke-peranti
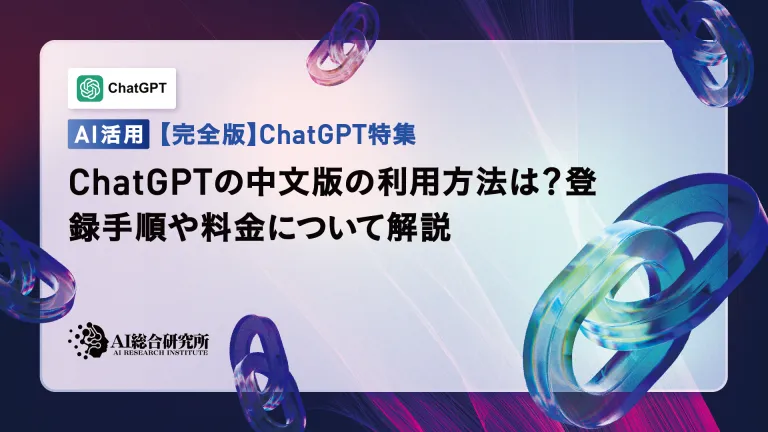
Chatgpt Versi Cina: Buka kunci pengalaman baru dialog Cina AI Chatgpt popular di seluruh dunia, adakah anda tahu ia juga menawarkan versi Cina? Alat AI yang kuat ini bukan sahaja menyokong perbualan harian, tetapi juga mengendalikan kandungan profesional dan serasi dengan Cina yang mudah dan tradisional. Sama ada pengguna di China atau rakan yang belajar bahasa Cina, anda boleh mendapat manfaat daripadanya. Artikel ini akan memperkenalkan secara terperinci bagaimana menggunakan versi CHATGPT Cina, termasuk tetapan akaun, input perkataan Cina, penggunaan penapis, dan pemilihan pakej yang berbeza, dan menganalisis potensi risiko dan strategi tindak balas. Di samping itu, kami juga akan membandingkan versi CHATGPT Cina dengan alat AI Cina yang lain untuk membantu anda memahami lebih baik kelebihan dan senario aplikasinya. Perisikan AI Terbuka Terbuka
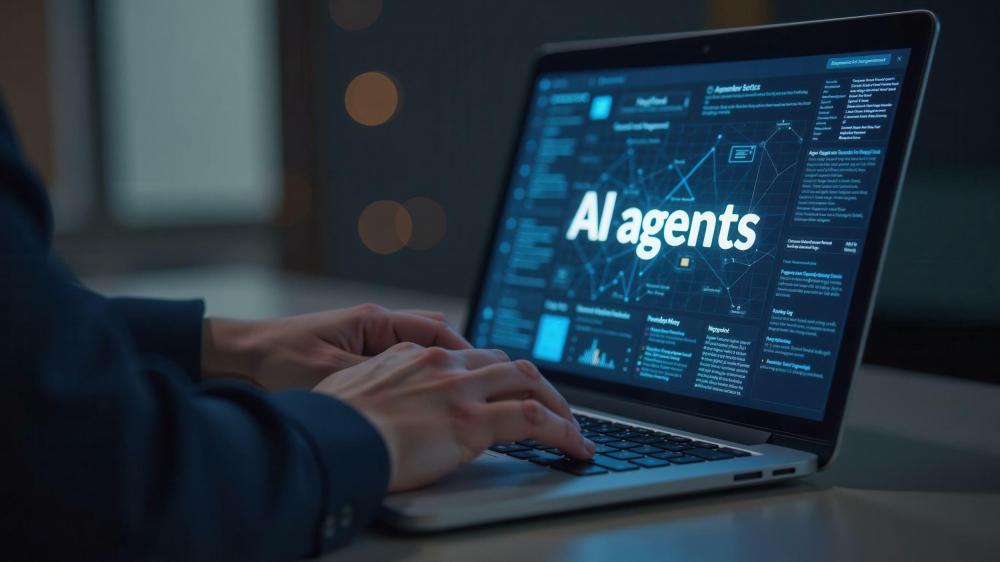
Ini boleh dianggap sebagai lonjakan seterusnya ke hadapan dalam bidang AI generatif, yang memberi kita chatgpt dan chatbots model bahasa besar yang lain. Daripada hanya menjawab soalan atau menghasilkan maklumat, mereka boleh mengambil tindakan bagi pihak kami, Inter
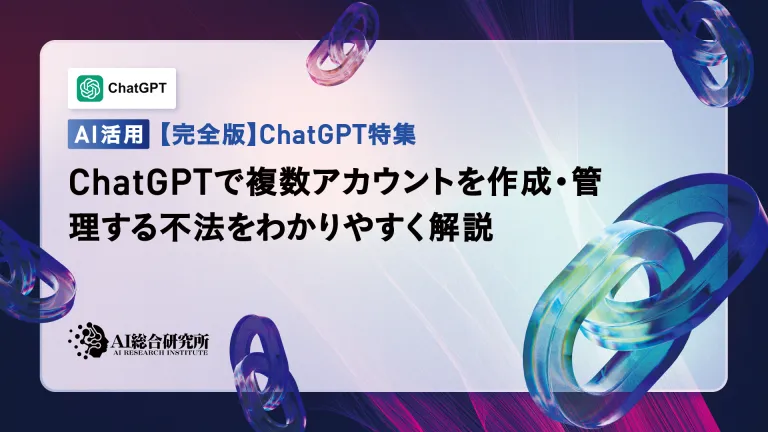
Teknik pengurusan akaun berganda yang cekap menggunakan CHATGPT | Penjelasan menyeluruh tentang cara menggunakan perniagaan dan kehidupan peribadi! ChatGPT digunakan dalam pelbagai situasi, tetapi sesetengah orang mungkin bimbang untuk menguruskan pelbagai akaun. Artikel ini akan menerangkan secara terperinci bagaimana untuk membuat pelbagai akaun untuk chatgpt, apa yang perlu dilakukan apabila menggunakannya, dan bagaimana untuk mengendalikannya dengan selamat dan cekap. Kami juga meliputi perkara penting seperti perbezaan dalam perniagaan dan penggunaan peribadi, dan mematuhi syarat penggunaan OpenAI, dan memberikan panduan untuk membantu anda menggunakan pelbagai akaun. Terbuka


Alat AI Hot

Undresser.AI Undress
Apl berkuasa AI untuk mencipta foto bogel yang realistik

AI Clothes Remover
Alat AI dalam talian untuk mengeluarkan pakaian daripada foto.

Undress AI Tool
Gambar buka pakaian secara percuma

Clothoff.io
Penyingkiran pakaian AI

Video Face Swap
Tukar muka dalam mana-mana video dengan mudah menggunakan alat tukar muka AI percuma kami!

Artikel Panas

Alat panas

Hantar Studio 13.0.1
Persekitaran pembangunan bersepadu PHP yang berkuasa

SublimeText3 Linux versi baharu
SublimeText3 Linux versi terkini

Penyesuai Pelayan SAP NetWeaver untuk Eclipse
Integrasikan Eclipse dengan pelayan aplikasi SAP NetWeaver.

MinGW - GNU Minimalis untuk Windows
Projek ini dalam proses untuk dipindahkan ke osdn.net/projects/mingw, anda boleh terus mengikuti kami di sana. MinGW: Port Windows asli bagi GNU Compiler Collection (GCC), perpustakaan import yang boleh diedarkan secara bebas dan fail pengepala untuk membina aplikasi Windows asli termasuk sambungan kepada masa jalan MSVC untuk menyokong fungsi C99. Semua perisian MinGW boleh dijalankan pada platform Windows 64-bit.

DVWA
Damn Vulnerable Web App (DVWA) ialah aplikasi web PHP/MySQL yang sangat terdedah. Matlamat utamanya adalah untuk menjadi bantuan bagi profesional keselamatan untuk menguji kemahiran dan alatan mereka dalam persekitaran undang-undang, untuk membantu pembangun web lebih memahami proses mengamankan aplikasi web, dan untuk membantu guru/pelajar mengajar/belajar dalam persekitaran bilik darjah Aplikasi web keselamatan. Matlamat DVWA adalah untuk mempraktikkan beberapa kelemahan web yang paling biasa melalui antara muka yang mudah dan mudah, dengan pelbagai tahap kesukaran. Sila ambil perhatian bahawa perisian ini
