


1. Pengenalan dan Matlamat
Selamat datang ke ansuran kelima siri kami untuk melaksanakan sistem pemprosesan pesanan yang canggih! Dalam catatan kami sebelum ini, kami telah merangkumi segala-galanya daripada menyediakan seni bina asas kepada melaksanakan aliran kerja lanjutan dan pemantauan menyeluruh. Hari ini, kami menyelami dunia pengesanan dan pembalakan yang diedarkan, dua komponen penting untuk mengekalkan kebolehmerhatian dalam seni bina perkhidmatan mikro.
Rekap Catatan Sebelumnya
- Dalam Bahagian 1, kami menyediakan struktur projek kami dan melaksanakan API CRUD asas.
- Bahagian 2 memfokuskan pada mengembangkan penggunaan Temporal kami untuk aliran kerja yang kompleks.
- Dalam Bahagian 3, kami menyelidiki operasi pangkalan data lanjutan, termasuk pengoptimuman dan sharding.
- Bahagian 4 merangkumi pemantauan dan amaran komprehensif menggunakan Prometheus dan Grafana.
Kepentingan Pengesanan dan Pengelogan Teragih dalam Seni Bina Perkhidmatan Mikro
Dalam seni bina perkhidmatan mikro, permintaan pengguna tunggal selalunya merangkumi berbilang perkhidmatan. Sifat teragih ini menjadikannya mencabar untuk memahami aliran permintaan dan mendiagnosis isu apabila ia timbul. Pengesanan teragih dan pembalakan berpusat menangani cabaran ini dengan menyediakan:
- Keterlihatan hujung ke hujung aliran permintaan merentas perkhidmatan
- Pandangan terperinci tentang prestasi komponen individu
- Keupayaan untuk mengaitkan acara merentas perkhidmatan yang berbeza
- Pandangan terpusat tentang tingkah laku dan kesihatan sistem
Gambaran keseluruhan OpenTelemetry dan ELK Stack
Untuk melaksanakan pengesanan dan pengelogan yang diedarkan, kami akan menggunakan dua set alat yang berkuasa:
-
OpenTelemetry : Rangka kerja pemerhatian untuk perisian asli awan yang menyediakan satu set API, perpustakaan, ejen dan perkhidmatan pengumpul untuk menangkap jejak dan metrik yang diedarkan daripada aplikasi anda.
ELK Stack : Koleksi tiga produk sumber terbuka - Elasticsearch, Logstash dan Kibana - daripada Elastic, yang bersama-sama menyediakan platform yang teguh untuk pengingesan log, penyimpanan dan visualisasi.
Matlamat untuk Bahagian Siri ini
Menjelang akhir siaran ini, anda akan dapat:
- Laksanakan pengesanan teragih merentas perkhidmatan mikro anda menggunakan OpenTelemetry
- Sediakan pengelogan berpusat menggunakan tindanan ELK
- Kaitkan log, surih dan metrik untuk pandangan bersatu tingkah laku sistem
- Melaksanakan strategi pengagregatan log dan analisis yang berkesan
- Gunakan amalan terbaik untuk log masuk seni bina perkhidmatan mikro
Jom selami!
2. Latar Belakang Teori dan Konsep
Sebelum kita mula melaksanakan, mari semak beberapa konsep utama yang akan menjadi penting untuk persediaan pengesanan dan pengelogan yang diedarkan kami.
Pengenalan kepada Pengesanan Teragih
Pengesanan teragih ialah kaedah menjejak permintaan semasa ia mengalir melalui pelbagai perkhidmatan dalam sistem teragih. Ia menyediakan cara untuk memahami kitaran hayat penuh permintaan, termasuk:
- Laluan permintaan melalui sistem
- Perkhidmatan dan sumber yang berinteraksi dengannya
- Masa yang diluangkan dalam setiap perkhidmatan
Surih biasanya terdiri daripada satu atau lebih rentang. Span mewakili unit kerja atau operasi. Ia menjejaki operasi khusus yang dibuat oleh permintaan, merekodkan masa operasi bermula dan tamat, serta data lain.
Memahami Projek OpenTelemetry dan Komponennya
OpenTelemetry ialah rangka kerja pemerhatian untuk perisian asli awan. Ia menyediakan satu set API, perpustakaan, ejen dan perkhidmatan pengumpul tunggal untuk menangkap jejak dan metrik yang diedarkan daripada aplikasi anda. Komponen utama termasuk:
- API : Menyediakan jenis data teras dan operasi untuk pengesanan dan metrik.
- SDK : Melaksanakan API, menyediakan cara untuk mengkonfigurasi dan menyesuaikan tingkah laku.
- Perpustakaan Instrumen : Menyediakan instrumentasi automatik untuk rangka kerja dan perpustakaan yang popular.
- Pengumpul : Menerima, memproses dan mengeksport data telemetri.
Gambaran Keseluruhan Amalan Terbaik Pembalakan dalam Sistem Teragih
Pengelogan berkesan dalam sistem teragih memerlukan pertimbangan yang teliti:
- Pengelogan Berstruktur : Gunakan format berstruktur yang konsisten (mis., JSON) untuk entri log bagi memudahkan penghuraian dan analisis.
- ID Korelasi : Sertakan pengecam unik dalam entri log untuk menjejak permintaan merentas perkhidmatan.
- Maklumat Kontekstual : Sertakan konteks yang berkaitan (cth., ID pengguna, ID pesanan) dalam entri log.
- Tahap Log : Gunakan tahap log yang sesuai (DEBUG, INFO, AMARAN, RALAT) secara konsisten merentas perkhidmatan.
- Log Berpusat : Agregat log daripada semua perkhidmatan di lokasi pusat untuk analisis yang lebih mudah.
Pengenalan kepada Timbunan ELK (Elasticsearch, Logstash, Kibana).
Timbunan ELK ialah pilihan popular untuk pengurusan log:
- Elasticsearch : Enjin carian dan analitik yang diedarkan dan RESTful yang mampu mengendalikan volum data yang besar.
- Logstash : Saluran paip pemprosesan data sebelah pelayan yang menyerap data daripada berbilang sumber, mengubahnya dan menghantarnya ke Elasticsearch.
- Kibana : Lapisan visualisasi yang berfungsi di atas Elasticsearch, menyediakan antara muka pengguna untuk mencari, melihat dan berinteraksi dengan data.
Konsep Pengagregatan dan Analisis Log
Pengagregatan log melibatkan pengumpulan data log daripada pelbagai sumber dan menyimpannya di lokasi berpusat. Ini membolehkan:
- Pencarian dan analisis log yang lebih mudah merentas pelbagai perkhidmatan
- Kaitan peristiwa merentas komponen sistem yang berbeza
- Storan jangka panjang dan pengarkiban data log
Analisis log melibatkan pengekstrakan cerapan bermakna daripada data log, yang boleh termasuk:
- Mengenal pasti corak dan trend
- Mengesan anomali dan ralat
- Memantau kesihatan dan prestasi sistem
- Menyokong analisis punca punca semasa tindak balas insiden
Dengan mengambil kira konsep ini, mari kita teruskan untuk melaksanakan pengesanan teragih dalam sistem pemprosesan pesanan kami.
3. Melaksanakan Pengesanan Teragih dengan OpenTelemetry
Mari mulakan dengan melaksanakan pengesanan teragih dalam sistem pemprosesan pesanan kami menggunakan OpenTelemetry.
Menyediakan OpenTelemetry dalam Perkhidmatan Go kami
Pertama, kami perlu menambah OpenTelemetry pada perkhidmatan Go kami. Tambahkan kebergantungan berikut pada fail go.mod anda:
require ( go.opentelemetry.io/otel v1.7.0 go.opentelemetry.io/otel/exporters/jaeger v1.7.0 go.opentelemetry.io/otel/sdk v1.7.0 go.opentelemetry.io/otel/trace v1.7.0 )
Seterusnya, mari sediakan pembekal pengesan dalam fungsi utama kami:
package main import ( "log" "go.opentelemetry.io/otel" "go.opentelemetry.io/otel/attribute" "go.opentelemetry.io/otel/exporters/jaeger" "go.opentelemetry.io/otel/sdk/resource" tracesdk "go.opentelemetry.io/otel/sdk/trace" semconv "go.opentelemetry.io/otel/semconv/v1.4.0" ) func initTracer() func() { exporter, err := jaeger.New(jaeger.WithCollectorEndpoint(jaeger.WithEndpoint("http://jaeger:14268/api/traces"))) if err != nil { log.Fatal(err) } tp := tracesdk.NewTracerProvider( tracesdk.WithBatcher(exporter), tracesdk.WithResource(resource.NewWithAttributes( semconv.SchemaURL, semconv.ServiceNameKey.String("order-processing-service"), attribute.String("environment", "production"), )), ) otel.SetTracerProvider(tp) return func() { if err := tp.Shutdown(context.Background()); err != nil { log.Printf("Error shutting down tracer provider: %v", err) } } } func main() { cleanup := initTracer() defer cleanup() // Rest of your main function... }
Ini menyediakan penyedia pengesan yang mengeksport jejak ke Jaeger, bahagian belakang pengesanan teragih yang popular.
Menginstrumenkan Aliran Kerja Pemprosesan Pesanan kami dengan Jejak
Sekarang, mari tambahkan pengesanan pada aliran kerja pemprosesan pesanan kami. Kita akan mulakan dengan fungsi CreateOrder:
import ( "context" "go.opentelemetry.io/otel" "go.opentelemetry.io/otel/attribute" "go.opentelemetry.io/otel/trace" ) func CreateOrder(ctx context.Context, order Order) error { tr := otel.Tracer("order-processing") ctx, span := tr.Start(ctx, "CreateOrder") defer span.End() span.SetAttributes(attribute.Int64("order.id", order.ID)) span.SetAttributes(attribute.Float64("order.total", order.Total)) // Validate order if err := validateOrder(ctx, order); err != nil { span.RecordError(err) span.SetStatus(codes.Error, "Order validation failed") return err } // Process payment if err := processPayment(ctx, order); err != nil { span.RecordError(err) span.SetStatus(codes.Error, "Payment processing failed") return err } // Update inventory if err := updateInventory(ctx, order); err != nil { span.RecordError(err) span.SetStatus(codes.Error, "Inventory update failed") return err } span.SetStatus(codes.Ok, "Order created successfully") return nil }
Ini mencipta rentang baharu untuk fungsi CreateOrder dan menambah atribut yang berkaitan. Ia juga mewujudkan rentang kanak-kanak untuk setiap langkah utama dalam proses.
Menyebarkan Konteks Merentasi Sempadan Perkhidmatan
Apabila membuat panggilan ke perkhidmatan lain, kami perlu menyebarkan konteks jejak. Berikut ialah contoh cara melakukan ini dengan klien HTTP:
import ( "net/http" "go.opentelemetry.io/contrib/instrumentation/net/http/otelhttp" ) func callExternalService(ctx context.Context, url string) error { client := http.Client{Transport: otelhttp.NewTransport(http.DefaultTransport)} req, err := http.NewRequestWithContext(ctx, "GET", url, nil) if err != nil { return err } _, err = client.Do(req) return err }
Ini menggunakan pakej otelhttp untuk menyebarkan konteks surih secara automatik dalam pengepala HTTP.
Mengendalikan Operasi Asynchronous dan Pekerjaan Latar Belakang
Untuk operasi tak segerak, kami perlu memastikan kami menghantar konteks jejak dengan betul. Berikut ialah contoh menggunakan kumpulan pekerja:
func processOrderAsync(ctx context.Context, order Order) { tr := otel.Tracer("order-processing") ctx, span := tr.Start(ctx, "processOrderAsync") defer span.End() workerPool <p>Ini mencipta rentang baharu untuk operasi async dan menyerahkannya kepada fungsi pekerja.</p> <h3> Mengintegrasikan OpenTelemetry dengan Aliran Kerja Sementara </h3> <p>Untuk menyepadukan OpenTelemetry dengan aliran kerja Temporal, kita boleh menggunakan pakej go.opentelemetry.io/contrib/instrumentation/go.temporal.io/temporal/oteltemporalgrpc:<br> </p> <pre class="brush:php;toolbar:false">import ( "go.temporal.io/sdk/client" "go.temporal.io/sdk/worker" "go.opentelemetry.io/contrib/instrumentation/go.temporal.io/temporal/oteltemporalgrpc" ) func initTemporalClient() (client.Client, error) { return client.NewClient(client.Options{ HostPort: "temporal:7233", ConnectionOptions: client.ConnectionOptions{ DialOptions: []grpc.DialOption{ grpc.WithUnaryInterceptor(oteltemporalgrpc.UnaryClientInterceptor()), grpc.WithStreamInterceptor(oteltemporalgrpc.StreamClientInterceptor()), }, }, }) } func initTemporalWorker(c client.Client, taskQueue string) worker.Worker { w := worker.New(c, taskQueue, worker.Options{ WorkerInterceptors: []worker.WorkerInterceptor{ oteltemporalgrpc.WorkerInterceptor(), }, }) return w }
Ini menyediakan pelanggan dan pekerja Temporal dengan instrumentasi OpenTelemetry.
Mengeksport Jejak ke Bahagian Belakang (cth., Jaeger)
Kami telah pun menyediakan Jaeger sebagai bahagian belakang jejak kami dalam fungsi initTracer. Untuk menggambarkan jejak kami, kami perlu menambahkan Jaeger pada docker-compose.yml kami:
services: # ... other services ... jaeger: image: jaegertracing/all-in-one:1.35 ports: - "16686:16686" - "14268:14268" environment: - COLLECTOR_OTLP_ENABLED=true
Kini anda boleh mengakses UI Jaeger di http://localhost:16686 untuk melihat dan menganalisis jejak anda.
Dalam bahagian seterusnya, kami akan menyediakan pengelogan berpusat menggunakan tindanan ELK untuk melengkapkan persediaan pengesanan teragih kami.
4. Menyediakan Pembalakan Berpusat dengan Tindanan ELK
Sekarang kami telah mengedarkan pengesanan di tempatnya, mari sediakan pembalakan berpusat menggunakan tindanan ELK (Elasticsearch, Logstash, Kibana).
Memasang dan Mengkonfigurasi Elasticsearch
Pertama, mari tambahkan Elasticsearch pada docker-compose.yml kami:
services: # ... other services ... elasticsearch: image: docker.elastic.co/elasticsearch/elasticsearch:7.14.0 environment: - discovery.type=single-node - "ES_JAVA_OPTS=-Xms512m -Xmx512m" ports: - "9200:9200" volumes: - elasticsearch_data:/usr/share/elasticsearch/data volumes: elasticsearch_data: driver: local
Ini menyediakan contoh Elasticsearch satu nod untuk tujuan pembangunan.
Setting up Logstash for Log Ingestion and Processing
Next, let’s add Logstash to our docker-compose.yml:
services: # ... other services ... logstash: image: docker.elastic.co/logstash/logstash:7.14.0 volumes: - ./logstash/pipeline:/usr/share/logstash/pipeline ports: - "5000:5000/tcp" - "5000:5000/udp" - "9600:9600" depends_on: - elasticsearch
Create a Logstash pipeline configuration file at ./logstash/pipeline/logstash.conf:
input { tcp { port => 5000 codec => json } } filter { if [trace_id] { mutate { add_field => { "[@metadata][trace_id]" => "%{trace_id}" } } } } output { elasticsearch { hosts => ["elasticsearch:9200"] index => "order-processing-logs-%{+YYYY.MM.dd}" } }
This configuration sets up Logstash to receive JSON logs over TCP, process them, and forward them to Elasticsearch.
Configuring Kibana for Log Visualization
Now, let’s add Kibana to our docker-compose.yml:
services: # ... other services ... kibana: image: docker.elastic.co/kibana/kibana:7.14.0 ports: - "5601:5601" environment: ELASTICSEARCH_URL: http://elasticsearch:9200 ELASTICSEARCH_HOSTS: '["http://elasticsearch:9200"]' depends_on: - elasticsearch
You can access the Kibana UI at http://localhost:5601 once it’s up and running.
Implementing Structured Logging in our Go Services
To send structured logs to Logstash, we’ll use the logrus library. First, add it to your go.mod:
go get github.com/sirupsen/logrus
Now, let’s set up a logger in our main function:
import ( "github.com/sirupsen/logrus" "gopkg.in/sohlich/elogrus.v7" ) func initLogger() *logrus.Logger { log := logrus.New() log.SetFormatter(&logrus.JSONFormatter{}) hook, err := elogrus.NewElasticHook("elasticsearch:9200", "warning", "order-processing-logs") if err != nil { log.Fatalf("Failed to create Elasticsearch hook: %v", err) } log.AddHook(hook) return log } func main() { log := initLogger() // Rest of your main function... }
This sets up a JSON formatter for our logs and adds an Elasticsearch hook to send logs directly to Elasticsearch.
Sending Logs from our Services to the ELK Stack
Now, let’s update our CreateOrder function to use structured logging:
func CreateOrder(ctx context.Context, order Order) error { tr := otel.Tracer("order-processing") ctx, span := tr.Start(ctx, "CreateOrder") defer span.End() logger := logrus.WithFields(logrus.Fields{ "order_id": order.ID, "trace_id": span.SpanContext().TraceID().String(), }) logger.Info("Starting order creation") // Validate order if err := validateOrder(ctx, order); err != nil { logger.WithError(err).Error("Order validation failed") span.RecordError(err) span.SetStatus(codes.Error, "Order validation failed") return err } // Process payment if err := processPayment(ctx, order); err != nil { logger.WithError(err).Error("Payment processing failed") span.RecordError(err) span.SetStatus(codes.Error, "Payment processing failed") return err } // Update inventory if err := updateInventory(ctx, order); err != nil { logger.WithError(err).Error("Inventory update failed") span.RecordError(err) span.SetStatus(codes.Error, "Inventory update failed") return err } logger.Info("Order created successfully") span.SetStatus(codes.Ok, "Order created successfully") return nil }
This code logs each step of the order creation process, including any errors that occur. It also includes the trace ID in each log entry, which will be crucial for correlating logs with traces.
5. Correlating Logs, Traces, and Metrics
Now that we have both distributed tracing and centralized logging set up, let’s explore how to correlate this information for a unified view of system behavior.
Implementing Correlation IDs Across Logs and Traces
We’ve already included the trace ID in our log entries. To make this correlation even more powerful, we can add a custom field to our spans that includes the log index:
span.SetAttributes(attribute.String("log.index", "order-processing-logs-"+time.Now().Format("2006.01.02")))
This allows us to easily jump from a span in Jaeger to the corresponding logs in Kibana.
Adding Trace IDs to Log Entries
We’ve already added trace IDs to our log entries in the previous section. This allows us to search for all log entries related to a particular trace in Kibana.
Linking Metrics to Traces Using Exemplars
To link our Prometheus metrics to traces, we can use exemplars. Here’s an example of how to do this:
import ( "github.com/prometheus/client_golang/prometheus" "github.com/prometheus/client_golang/prometheus/promauto" "go.opentelemetry.io/otel/trace" ) var ( orderProcessingDuration = promauto.NewHistogramVec( prometheus.HistogramOpts{ Name: "order_processing_duration_seconds", Help: "Duration of order processing in seconds", Buckets: prometheus.DefBuckets, }, []string{"status"}, ) ) func CreateOrder(ctx context.Context, order Order) error { // ... existing code ... start := time.Now() // ... process order ... duration := time.Since(start) orderProcessingDuration.WithLabelValues("success").Observe(duration.Seconds(), prometheus.Labels{ "trace_id": span.SpanContext().TraceID().String(), }) // ... rest of the function ... }
This adds the trace ID as an exemplar to our order processing duration metric.
Creating a Unified View of System Behavior
With logs, traces, and metrics all correlated, we can create a unified view of our system’s behavior:
- In Grafana, create a dashboard that includes both Prometheus metrics and Elasticsearch logs.
- Use the trace ID to link from a metric to the corresponding trace in Jaeger.
- From Jaeger, use the log index attribute to link to the corresponding logs in Kibana.
This allows you to seamlessly navigate between metrics, traces, and logs, providing a comprehensive view of your system’s behavior and making it easier to debug issues.
6. Log Aggregation and Analysis
With our logs centralized in Elasticsearch, let’s explore some strategies for effective log aggregation and analysis.
Designing Effective Log Aggregation Strategies
- Use Consistent Log Formats : Ensure all services use the same log format (in our case, JSON) with consistent field names.
- Include Relevant Context : Always include relevant context in logs, such as order ID, user ID, and trace ID.
- Use Log Levels Appropriately : Use DEBUG for detailed information, INFO for general information, WARN for potential issues, and ERROR for actual errors.
- Aggregate Logs by Service : Use different Elasticsearch indices or index patterns for different services to allow for easier analysis.
Implementing Log Sampling for High-Volume Services
For high-volume services, logging every event can be prohibitively expensive. Implement log sampling to reduce the volume while still maintaining visibility:
func shouldLog() bool { return rand.Float32() <h3> Creating Kibana Dashboards for Log Analysis </h3> <p>In Kibana, create dashboards that provide insights into your system’s behavior. Some useful visualizations might include:</p> <ol> <li>Number of orders created over time</li> <li>Distribution of order processing times</li> <li>Error rate by service</li> <li>Most common error types</li> </ol> <h3> Implementing Alerting Based on Log Patterns </h3> <p>Use Kibana’s alerting features to set up alerts based on log patterns. For example:</p> <ol> <li>Alert when the error rate exceeds a certain threshold</li> <li>Alert on specific error messages that indicate critical issues</li> <li>Alert when order processing time exceeds a certain duration</li> </ol> <h3> Using Machine Learning for Anomaly Detection in Logs </h3> <p>Elasticsearch provides machine learning capabilities that can be used for anomaly detection in logs. You can set up machine learning jobs in Kibana to detect:</p> <ol> <li>Unusual spikes in error rates</li> <li>Abnormal patterns in order creation</li> <li>Unexpected changes in log volume</li> </ol> <p>These machine learning insights can help you identify issues before they become critical problems.</p> <p>In the next sections, we’ll cover best practices for logging in a microservices architecture and explore some advanced OpenTelemetry techniques.</p> <h2> 7. Best Practices for Logging in a Microservices Architecture </h2> <p>When implementing logging in a microservices architecture, there are several best practices to keep in mind to ensure your logs are useful, manageable, and secure.</p> <h3> Standardizing Log Formats Across Services </h3> <p>Consistency in log formats across all your services is crucial for effective log analysis. In our Go services, we can create a custom logger that enforces a standard format:<br> </p> <pre class="brush:php;toolbar:false">import ( "github.com/sirupsen/logrus" ) type StandardLogger struct { *logrus.Logger ServiceName string } func NewStandardLogger(serviceName string) *StandardLogger { logger := logrus.New() logger.SetFormatter(&logrus.JSONFormatter{ FieldMap: logrus.FieldMap{ logrus.FieldKeyTime: "timestamp", logrus.FieldKeyLevel: "severity", logrus.FieldKeyMsg: "message", }, }) return &StandardLogger{ Logger: logger, ServiceName: serviceName, } } func (l *StandardLogger) WithFields(fields logrus.Fields) *logrus.Entry { return l.Logger.WithFields(logrus.Fields{ "service": l.ServiceName, }).WithFields(fields) }
This logger ensures that all log entries include a “service” field and use consistent field names.
Implementing Contextual Logging
Contextual logging involves including relevant context with each log entry. In a microservices architecture, this often means including a request ID or trace ID that can be used to correlate logs across services:
func CreateOrder(ctx context.Context, logger *StandardLogger, order Order) error { tr := otel.Tracer("order-processing") ctx, span := tr.Start(ctx, "CreateOrder") defer span.End() logger := logger.WithFields(logrus.Fields{ "order_id": order.ID, "trace_id": span.SpanContext().TraceID().String(), }) logger.Info("Starting order creation") // ... rest of the function ... }
Handling Sensitive Information in Logs
It’s crucial to ensure that sensitive information, such as personal data or credentials, is not logged. You can create a custom log hook to redact sensitive information:
type SensitiveDataHook struct{} func (h *SensitiveDataHook) Levels() []logrus.Level { return logrus.AllLevels } func (h *SensitiveDataHook) Fire(entry *logrus.Entry) error { if entry.Data["credit_card"] != nil { entry.Data["credit_card"] = "REDACTED" } return nil } // In your main function: logger.AddHook(&SensitiveDataHook{})
Managing Log Retention and Rotation
In a production environment, you need to manage log retention and rotation to control storage costs and comply with data retention policies. While Elasticsearch can handle this to some extent, you might also want to implement log rotation at the application level:
import ( "gopkg.in/natefinch/lumberjack.v2" ) func initLogger() *logrus.Logger { logger := logrus.New() logger.SetOutput(&lumberjack.Logger{ Filename: "/var/log/myapp.log", MaxSize: 100, // megabytes MaxBackups: 3, MaxAge: 28, //days Compress: true, }) return logger }
Implementing Audit Logging for Compliance Requirements
For certain operations, you may need to maintain an audit trail for compliance reasons. You can create a separate audit logger for this purpose:
type AuditLogger struct { logger *logrus.Logger } func NewAuditLogger() *AuditLogger { logger := logrus.New() logger.SetFormatter(&logrus.JSONFormatter{}) // Set up a separate output for audit logs // This could be a different file, database, or even a separate Elasticsearch index return &AuditLogger{logger: logger} } func (a *AuditLogger) LogAuditEvent(ctx context.Context, event string, details map[string]interface{}) { span := trace.SpanFromContext(ctx) a.logger.WithFields(logrus.Fields{ "event": event, "trace_id": span.SpanContext().TraceID().String(), "details": details, }).Info("Audit event") } // Usage: auditLogger.LogAuditEvent(ctx, "OrderCreated", map[string]interface{}{ "order_id": order.ID, "user_id": order.UserID, })
8. Advanced OpenTelemetry Techniques
Now that we have a solid foundation for distributed tracing, let’s explore some advanced techniques to get even more value from OpenTelemetry.
Implementing Custom Span Attributes and Events
Custom span attributes and events can provide additional context to your traces:
func ProcessPayment(ctx context.Context, order Order) error { _, span := otel.Tracer("payment-service").Start(ctx, "ProcessPayment") defer span.End() span.SetAttributes( attribute.String("payment.method", order.PaymentMethod), attribute.Float64("payment.amount", order.Total), ) // Process payment... if paymentSuccessful { span.AddEvent("PaymentProcessed", trace.WithAttributes( attribute.String("transaction_id", transactionID), )) } else { span.AddEvent("PaymentFailed", trace.WithAttributes( attribute.String("error", "Insufficient funds"), )) } return nil }
Using OpenTelemetry’s Baggage for Cross-Cutting Concerns
Baggage allows you to propagate key-value pairs across service boundaries:
import ( "go.opentelemetry.io/otel/baggage" ) func AddUserInfoToBaggage(ctx context.Context, userID string) context.Context { b, _ := baggage.Parse(fmt.Sprintf("user_id=%s", userID)) return baggage.ContextWithBaggage(ctx, b) } func GetUserIDFromBaggage(ctx context.Context) string { if b := baggage.FromContext(ctx); b != nil { if v := b.Member("user_id"); v.Key() != "" { return v.Value() } } return "" }
Implementing Sampling Strategies for High-Volume Tracing
For high-volume services, tracing every request can be expensive. Implement a sampling strategy to reduce the volume while still maintaining visibility:
import ( "go.opentelemetry.io/otel/sdk/trace" "go.opentelemetry.io/otel/sdk/trace/sampling" ) sampler := sampling.ParentBased( sampling.TraceIDRatioBased(0.1), // Sample 10% of traces ) tp := trace.NewTracerProvider( trace.WithSampler(sampler), // ... other options ... )
Creating Custom OpenTelemetry Exporters
While we’ve been using Jaeger as our tracing backend, you might want to create a custom exporter for a different backend or for special processing:
type CustomExporter struct{} func (e *CustomExporter) ExportSpans(ctx context.Context, spans []trace.ReadOnlySpan) error { for _, span := range spans { // Process or send the span data as needed fmt.Printf("Exporting span: %s\n", span.Name()) } return nil } func (e *CustomExporter) Shutdown(ctx context.Context) error { // Cleanup logic here return nil } // Use the custom exporter: exporter := &CustomExporter{} tp := trace.NewTracerProvider( trace.WithBatcher(exporter), // ... other options ... )
Integrating OpenTelemetry with Existing Monitoring Tools
OpenTelemetry can be integrated with many existing monitoring tools. For example, to send traces to both Jaeger and Zipkin:
jaegerExporter, _ := jaeger.New(jaeger.WithCollectorEndpoint(jaeger.WithEndpoint("http://jaeger:14268/api/traces"))) zipkinExporter, _ := zipkin.New("http://zipkin:9411/api/v2/spans") tp := trace.NewTracerProvider( trace.WithBatcher(jaegerExporter), trace.WithBatcher(zipkinExporter), // ... other options ... )
These advanced techniques will help you get the most out of OpenTelemetry in your order processing system.
In the next sections, we’ll cover performance considerations, testing and validation strategies, and discuss some challenges and considerations when implementing distributed tracing and logging at scale.
9. Performance Considerations
When implementing distributed tracing and logging, it’s crucial to consider the performance impact on your system. Let’s explore some strategies to optimize performance.
Optimizing Logging Performance in High-Throughput Systems
- Use Asynchronous Logging : Implement a buffered, asynchronous logger to minimize the impact on request processing:
type AsyncLogger struct { ch chan *logrus.Entry } func NewAsyncLogger(bufferSize int) *AsyncLogger { logger := &AsyncLogger{ ch: make(chan *logrus.Entry, bufferSize), } go logger.run() return logger } func (l *AsyncLogger) run() { for entry := range l.ch { entry.Logger.Out.Write(entry.Bytes()) } } func (l *AsyncLogger) Log(entry *logrus.Entry) { select { case l.ch <ol> <li> <strong>Log Sampling</strong> : For very high-throughput systems, consider sampling your logs: </li> </ol> <pre class="brush:php;toolbar:false">func (l *AsyncLogger) SampledLog(entry *logrus.Entry, sampleRate float32) { if rand.Float32() <h3> Managing the Performance Impact of Distributed Tracing </h3> <ol> <li> <strong>Use Sampling</strong> : Implement a sampling strategy to reduce the volume of traces: </li> </ol> <pre class="brush:php;toolbar:false">sampler := trace.ParentBased( trace.TraceIDRatioBased(0.1), // Sample 10% of traces ) tp := trace.NewTracerProvider( trace.WithSampler(sampler), // ... other options ... )
- Optimize Span Creation : Only create spans for significant operations to reduce overhead:
func ProcessOrder(ctx context.Context, order Order) error { ctx, span := tracer.Start(ctx, "ProcessOrder") defer span.End() // Don't create a span for this quick operation validateOrder(order) // Create a span for this potentially slow operation ctx, paymentSpan := tracer.Start(ctx, "ProcessPayment") err := processPayment(ctx, order) paymentSpan.End() if err != nil { return err } // ... rest of the function }
Implementing Buffering and Batching for Trace and Log Export
Use the OpenTelemetry SDK’s built-in batching exporter to reduce the number of network calls:
exporter, err := jaeger.New(jaeger.WithCollectorEndpoint(jaeger.WithEndpoint("http://jaeger:14268/api/traces"))) if err != nil { log.Fatalf("Failed to create Jaeger exporter: %v", err) } tp := trace.NewTracerProvider( trace.WithBatcher(exporter, trace.WithMaxExportBatchSize(100), trace.WithBatchTimeout(5 * time.Second), ), // ... other options ... )
Scaling the ELK Stack for Large-Scale Systems
- Use Index Lifecycle Management : Configure Elasticsearch to automatically manage index lifecycle:
PUT _ilm/policy/logs_policy { "policy": { "phases": { "hot": { "actions": { "rollover": { "max_size": "50GB", "max_age": "1d" } } }, "delete": { "min_age": "30d", "actions": { "delete": {} } } } } }
- Implement Elasticsearch Clustering : For large-scale systems, set up Elasticsearch in a multi-node cluster for better performance and reliability.
Implementing Caching Strategies for Frequently Accessed Logs and Traces
Use a caching layer like Redis to store frequently accessed logs and traces:
import ( "github.com/go-redis/redis/v8" ) func getCachedTrace(traceID string) (*Trace, error) { val, err := redisClient.Get(ctx, "trace:"+traceID).Bytes() if err == redis.Nil { // Trace not in cache, fetch from storage and cache it trace, err := fetchTraceFromStorage(traceID) if err != nil { return nil, err } redisClient.Set(ctx, "trace:"+traceID, trace, 1*time.Hour) return trace, nil } else if err != nil { return nil, err } var trace Trace json.Unmarshal(val, &trace) return &trace, nil }
10. Testing and Validation
Proper testing and validation are crucial to ensure the reliability of your distributed tracing and logging implementation.
Unit Testing Trace Instrumentation
Use the OpenTelemetry testing package to unit test your trace instrumentation:
import ( "testing" "go.opentelemetry.io/otel/sdk/trace/tracetest" ) func TestProcessOrder(t *testing.T) { sr := tracetest.NewSpanRecorder() tp := trace.NewTracerProvider(trace.WithSpanProcessor(sr)) otel.SetTracerProvider(tp) ctx := context.Background() err := ProcessOrder(ctx, Order{ID: "123"}) if err != nil { t.Errorf("ProcessOrder failed: %v", err) } spans := sr.Ended() if len(spans) != 2 { t.Errorf("Expected 2 spans, got %d", len(spans)) } if spans[0].Name() != "ProcessOrder" { t.Errorf("Expected span named 'ProcessOrder', got '%s'", spans[0].Name()) } if spans[1].Name() != "ProcessPayment" { t.Errorf("Expected span named 'ProcessPayment', got '%s'", spans[1].Name()) } }
Integration Testing for the Complete Tracing Pipeline
Set up integration tests that cover your entire tracing pipeline:
func TestTracingPipeline(t *testing.T) { // Start a test Jaeger instance jaeger := startTestJaeger() defer jaeger.Stop() // Initialize your application with tracing app := initializeApp() // Perform some operations that should generate traces resp, err := app.CreateOrder(Order{ID: "123"}) if err != nil { t.Fatalf("Failed to create order: %v", err) } // Wait for traces to be exported time.Sleep(5 * time.Second) // Query Jaeger for the trace traces, err := jaeger.QueryTraces(resp.TraceID) if err != nil { t.Fatalf("Failed to query traces: %v", err) } // Validate the trace validateTrace(t, traces[0]) }
Validating Log Parsing and Processing Rules
Test your Logstash configuration to ensure it correctly parses and processes logs:
input { generator { message => '{"timestamp":"2023-06-01T10:00:00Z","severity":"INFO","message":"Order created","order_id":"123","trace_id":"abc123"}' count => 1 } } filter { json { source => "message" } } output { stdout { codec => rubydebug } }
Run this configuration with logstash -f test_config.conf and verify the output.
Load Testing and Observing Tracing Overhead
Perform load tests to understand the performance impact of tracing:
func BenchmarkWithTracing(b *testing.B) { // Initialize tracing tp := initTracer() defer tp.Shutdown(context.Background()) b.ResetTimer() for i := 0; i <p>Compare the results to understand the overhead introduced by tracing.</p> <h3> Implementing Trace and Log Monitoring for Quality Assurance </h3> <p>Set up monitoring for your tracing and logging systems:</p> <ol> <li>Monitor trace export errors</li> <li>Track log ingestion rates</li> <li>Alert on sudden changes in trace or log volume</li> <li>Monitor Elasticsearch, Logstash, and Kibana health</li> </ol> <h2> 11. Challenges and Considerations </h2> <p>As you implement and scale your distributed tracing and logging system, keep these challenges and considerations in mind:</p><h3> Menguruskan Kos Pengekalan dan Penyimpanan Data </h3>
- Laksanakan dasar pengekalan data yang mengimbangi keperluan pematuhan dengan kos storan
- Gunakan penyelesaian storan berperingkat, alihkan data lama ke pilihan storan yang lebih murah
- Semak dan optimumkan strategi pengekalan data anda dengan kerap
Memastikan Privasi dan Pematuhan Data dalam Log dan Jejak
- Laksanakan penutupan data yang mantap untuk maklumat sensitif
- Pastikan pematuhan terhadap peraturan seperti GDPR, termasuk hak untuk dilupakan
- Selalu audit log dan jejak anda untuk memastikan tiada data sensitif dikumpul secara tidak sengaja
Mengendalikan Versi dan Keserasian Ke Belakang dalam Data Surih
- Gunakan versi semantik untuk format data surih anda
- Laksanakan perubahan serasi ke belakang apabila boleh
- Apabila melanggar perubahan diperlukan, versi data surih anda dan kekalkan sokongan untuk berbilang versi semasa tempoh peralihan
Menangani Clock Skew dalam Cap Masa Surih Teragih
- Gunakan protokol penyegerakan masa seperti NTP merentas semua perkhidmatan anda
- Pertimbangkan untuk menggunakan jam logik sebagai tambahan kepada masa jam dinding
- Terapkan toleransi untuk sejumlah kecil jam yang condong dalam alat analisis surih anda
Melaksanakan Kawalan Akses dan Keselamatan untuk Tindanan ELK
- Gunakan pengesahan yang kukuh untuk Elasticsearch, Logstash dan Kibana
- Laksanakan kawalan akses berasaskan peranan (RBAC) untuk jenis pengguna yang berbeza
- Sulitkan data dalam transit dan dalam keadaan rehat
- Kemas kini dan tampal secara kerap semua komponen timbunan ELK anda
12. Langkah Seterusnya dan Pratonton Bahagian 6
Dalam siaran ini, kami telah membincangkan pengesanan dan pengelogan teragih yang komprehensif untuk sistem pemprosesan pesanan kami. Kami telah melaksanakan pengesanan dengan OpenTelemetry, menyediakan pengelogan berpusat dengan timbunan ELK, log dan jejak berkorelasi serta meneroka teknik dan pertimbangan lanjutan.
Dalam bahagian seterusnya dan akhir siri kami, kami akan menumpukan pada Kesediaan Pengeluaran dan Kebolehskalaan. Kami akan meliputi:
- Melaksanakan pengesahan dan kebenaran
- Mengendalikan pengurusan konfigurasi
- Melaksanakan pengehadan kadar dan pendikitan
- Mengoptimumkan untuk keselarasan tinggi
- Melaksanakan strategi caching
- Bersedia untuk penskalaan mendatar
- Menjalankan ujian prestasi dan pengoptimuman
Nantikan semasa kami memberikan sentuhan akhir pada sistem pemprosesan pesanan kami yang canggih, memastikan ia sedia untuk kegunaan pengeluaran secara berskala!
Perlukan Bantuan?
Adakah anda menghadapi masalah yang mencabar, atau memerlukan perspektif luaran tentang idea atau projek baharu? Saya boleh tolong! Sama ada anda ingin membina konsep bukti teknologi sebelum membuat pelaburan yang lebih besar, atau anda memerlukan panduan tentang isu yang sukar, saya sedia membantu.
Perkhidmatan yang Ditawarkan:
- Penyelesaian Masalah: Menangani isu yang rumit dengan penyelesaian yang inovatif.
- Perundingan: Memberikan nasihat pakar dan pandangan baharu tentang projek anda.
- Bukti Konsep: Membangunkan model awal untuk menguji dan mengesahkan idea anda.
Jika anda berminat untuk bekerja dengan saya, sila hubungi melalui e-mel di hungaikevin@gmail.com.
Mari jadikan cabaran anda sebagai peluang!
Atas ialah kandungan terperinci Melaksanakan Sistem Pemprosesan Pesanan: Pengesanan dan Pembalakan Bahagian Teragih. Untuk maklumat lanjut, sila ikut artikel berkaitan lain di laman web China PHP!
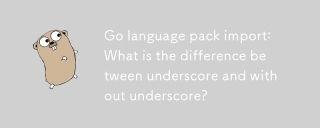
Artikel ini menerangkan mekanisme import pakej Go: Dinamakan import (mis., Import & quot; fmt & quot;) dan import kosong (mis., Import _ & quot; fmt & quot;). Dinamakan import membuat kandungan pakej boleh diakses, sementara import kosong hanya melaksanakan t
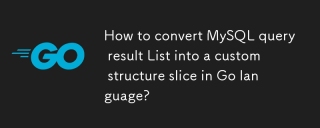
Artikel ini memperincikan penukaran yang cekap hasil pertanyaan MySQL ke dalam kepingan struct go. Ia menekankan menggunakan kaedah imbasan pangkalan data/SQL untuk prestasi optimum, mengelakkan parsing manual. Amalan terbaik untuk pemetaan medan struct menggunakan tag db dan robus
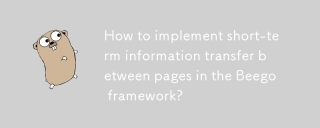
Artikel ini menerangkan fungsi Newflash () Beego untuk pemindahan data antara halaman dalam aplikasi web. Ia memberi tumpuan kepada menggunakan NewFlash () untuk memaparkan mesej sementara (kejayaan, kesilapan, amaran) antara pengawal, memanfaatkan mekanisme sesi. Limita
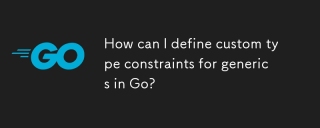
Artikel ini meneroka kekangan jenis adat Go untuk generik. Ia memperincikan bagaimana antara muka menentukan keperluan jenis minimum untuk fungsi generik, meningkatkan keselamatan jenis dan kebolehgunaan semula kod. Artikel ini juga membincangkan batasan dan amalan terbaik
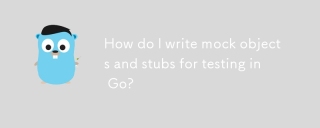
Artikel ini menunjukkan penciptaan dan stub di GO untuk ujian unit. Ia menekankan penggunaan antara muka, menyediakan contoh pelaksanaan mengejek, dan membincangkan amalan terbaik seperti menjaga mocks fokus dan menggunakan perpustakaan penegasan. Articl
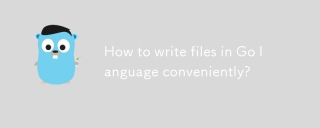
Artikel ini memperincikan penulisan fail yang cekap di GO, membandingkan OS.WriteFile (sesuai untuk fail kecil) dengan os.openfile dan buffered menulis (optimum untuk fail besar). Ia menekankan pengendalian ralat yang teguh, menggunakan penangguhan, dan memeriksa kesilapan tertentu.
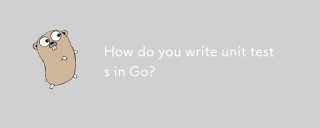
Artikel ini membincangkan ujian unit menulis di GO, meliputi amalan terbaik, teknik mengejek, dan alat untuk pengurusan ujian yang cekap.
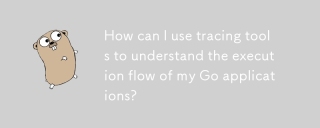
Artikel ini meneroka menggunakan alat pengesanan untuk menganalisis aliran pelaksanaan aplikasi GO. Ia membincangkan teknik instrumentasi manual dan automatik, membandingkan alat seperti Jaeger, Zipkin, dan OpenTelemetry, dan menonjolkan visualisasi data yang berkesan


Alat AI Hot

Undresser.AI Undress
Apl berkuasa AI untuk mencipta foto bogel yang realistik

AI Clothes Remover
Alat AI dalam talian untuk mengeluarkan pakaian daripada foto.

Undress AI Tool
Gambar buka pakaian secara percuma

Clothoff.io
Penyingkiran pakaian AI

AI Hentai Generator
Menjana ai hentai secara percuma.

Artikel Panas

Alat panas

DVWA
Damn Vulnerable Web App (DVWA) ialah aplikasi web PHP/MySQL yang sangat terdedah. Matlamat utamanya adalah untuk menjadi bantuan bagi profesional keselamatan untuk menguji kemahiran dan alatan mereka dalam persekitaran undang-undang, untuk membantu pembangun web lebih memahami proses mengamankan aplikasi web, dan untuk membantu guru/pelajar mengajar/belajar dalam persekitaran bilik darjah Aplikasi web keselamatan. Matlamat DVWA adalah untuk mempraktikkan beberapa kelemahan web yang paling biasa melalui antara muka yang mudah dan mudah, dengan pelbagai tahap kesukaran. Sila ambil perhatian bahawa perisian ini

Muat turun versi mac editor Atom
Editor sumber terbuka yang paling popular
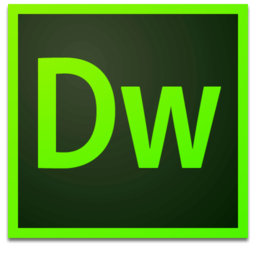
Dreamweaver Mac版
Alat pembangunan web visual

PhpStorm versi Mac
Alat pembangunan bersepadu PHP profesional terkini (2018.2.1).

SecLists
SecLists ialah rakan penguji keselamatan muktamad. Ia ialah koleksi pelbagai jenis senarai yang kerap digunakan semasa penilaian keselamatan, semuanya di satu tempat. SecLists membantu menjadikan ujian keselamatan lebih cekap dan produktif dengan menyediakan semua senarai yang mungkin diperlukan oleh penguji keselamatan dengan mudah. Jenis senarai termasuk nama pengguna, kata laluan, URL, muatan kabur, corak data sensitif, cangkerang web dan banyak lagi. Penguji hanya boleh menarik repositori ini ke mesin ujian baharu dan dia akan mempunyai akses kepada setiap jenis senarai yang dia perlukan.
