제목: 동시 프로그래밍에서 발생하는 Python 문제 및 솔루션
소개:
현대 컴퓨터 시스템에서 동시 프로그래밍을 사용하면 멀티 코어 프로세서의 성능을 최대한 활용하고 프로그램의 실행 효율성을 향상시킬 수 있습니다. 널리 사용되는 프로그래밍 언어인 Python에는 강력한 동시 프로그래밍 기능도 있습니다. 그러나 동시 프로그래밍에서는 종종 몇 가지 문제가 발생합니다. 이 기사에서는 동시 프로그래밍에서 몇 가지 일반적인 Python 문제를 소개하고 특정 코드 예제와 함께 해당 솔루션을 제공합니다.
1. GIL(Global Interpreter Lock)
- 문제 개요:
Python에서 GIL(Global Interpreter Lock)은 여러 스레드에서 실행되는 Python 프로그램에 대한 제한 사항입니다. GIL은 동시 프로그램이 멀티 코어 프로세서에서 실제로 병렬로 실행되는 것을 방지하여 Python 동시 프로그램의 성능에 영향을 미칩니다. - 해결책:
(1) 다중 프로세스 간에 진정한 병렬 실행을 달성하려면 다중 스레드 대신 다중 프로세스를 사용하십시오.
(2) Cython과 같은 도구를 사용하여 C 확장 모듈을 작성하여 GIL 제한을 우회합니다.
샘플 코드:
import multiprocessing def compute(num): result = num * 2 return result if __name__ == '__main__': pool = multiprocessing.Pool() numbers = [1, 2, 3, 4, 5] results = pool.map(compute, numbers) print(results)
2. 스레드 안전성
- 문제 개요:
멀티 스레드 환경에서 여러 스레드가 동시에 공유 리소스에 액세스하면 데이터 경합(데이터 경합)과 같은 스레드 안전 문제가 발생할 수 있습니다. 경주), 프로그램에 문제가 발생했습니다. - 해결책:
(1) 하나의 스레드만 동시에 공유 리소스에 액세스할 수 있도록 하려면 뮤텍스(Mutex)를 사용하십시오.
(2) 스레딩 모듈의 대기열 대기열과 같은 스레드로부터 안전한 데이터 구조를 사용합니다.
샘플 코드:
import threading import time class Counter: def __init__(self): self.value = 0 self.lock = threading.Lock() def increment(self): with self.lock: old_value = self.value time.sleep(1) # 模拟耗时操作 self.value = old_value + 1 if __name__ == '__main__': counter = Counter() threads = [] for _ in range(5): t = threading.Thread(target=counter.increment) threads.append(t) t.start() for t in threads: t.join() print(counter.value)
3. 동시 데이터 공유
- 문제 개요:
멀티 스레드 또는 다중 프로세스 프로그램에서 데이터 공유는 매우 일반적인 요구 사항이지만 데이터 일관성 및 경쟁과 같은 문제도 발생합니다. 경쟁 조건으로. - 해결책:
(1) 스레딩 모듈의 대기열 대기열과 같은 스레드로부터 안전한 데이터 구조를 사용하여 서로 다른 스레드/프로세스 간의 데이터 공유를 조정합니다.
(2) 큐, 파이프 등과 같은 프로세스 간 통신(IPC) 메커니즘을 사용합니다.
샘플 코드:
import multiprocessing def consumer(queue): while True: item = queue.get() if item == 'end': break print(f'consume {item}') def producer(queue): for i in range(5): print(f'produce {i}') queue.put(i) queue.put('end') if __name__ == '__main__': queue = multiprocessing.Queue() p1 = multiprocessing.Process(target=consumer, args=(queue,)) p2 = multiprocessing.Process(target=producer, args=(queue,)) p1.start() p2.start() p1.join() p2.join()
결론:
이 문서에서는 특정 코드 예제를 사용하여 동시 프로그래밍에서 일반적인 Python 문제를 분석하여 해당 솔루션을 제공합니다. 동시 프로그래밍은 프로그램 운영의 효율성을 향상시키는 중요한 수단입니다. 동시 프로그래밍의 문제를 올바르게 해결하면 프로그램의 동시성 기능과 성능이 크게 향상됩니다.
위 내용은 동시 프로그래밍에서 발생하는 Python 문제와 해결 방법의 상세 내용입니다. 자세한 내용은 PHP 중국어 웹사이트의 기타 관련 기사를 참조하세요!
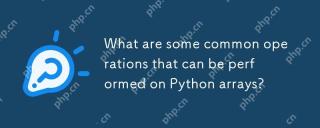
PythonArraysSupportVariousOperations : 1) SlicingExtractsSubsets, 2) 추가/확장 어드먼트, 3) 삽입 값 삽입 ATSpecificPositions, 4) retingdeletesElements, 5) 분류/ReversingChangesOrder 및 6) ListsompectionScreateNewListSbasedOnsistin
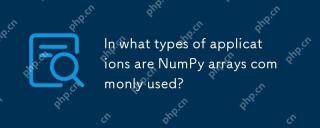
NumpyArraysareSentialplosplicationSefficationSefficientNumericalcomputationsanddatamanipulation. Theyarcrucialindatascience, MachineLearning, Physics, Engineering 및 Financeduetotheiribility에 대한 handlarge-scaledataefficivally. forexample, Infinancialanyaly
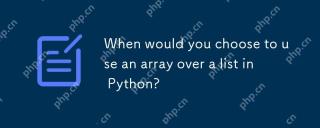
UseanArray.ArrayOveralistInpyThonWhendealingwithhomogeneousData, Performance-CriticalCode, OrinterFacingwithCcode.1) HomogeneousData : ArraysSaveMemorywithtypepletement.2) Performance-CriticalCode : arraysofferbetterporcomanceFornumericalOperations.3) Interf
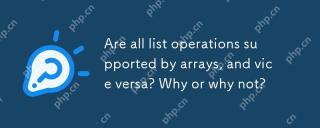
아니요, NOTALLLISTOPERATIONARESUPPORTEDBYARRARES, andVICEVERSA.1) ArraySDONOTSUPPORTDYNAMICOPERATIONSLIKEPENDORINSERTWITHUTRESIGING, WHITHIMPACTSPERFORMANCE.2) ListSDONOTEECONSTANTTIMECOMPLEXITEFORDITITICCESSLIKEARRAYSDO.
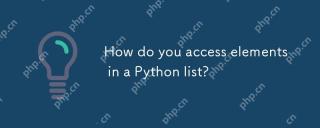
ToaccesselementsInapyThonlist, 사용 인덱싱, 부정적인 인덱싱, 슬라이스, 오리 화.
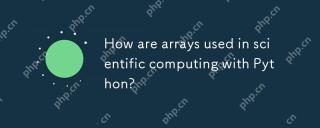
Arraysinpython, 특히 비밀 복구를위한 ArecrucialInscientificcomputing.1) theaRearedFornumericalOperations, DataAnalysis 및 MachinELearning.2) Numpy'SimplementationIncensuressuressurations thanpythonlists.3) arraysenablequick
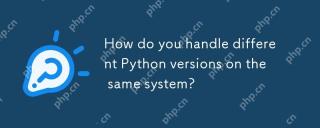
Pyenv, Venv 및 Anaconda를 사용하여 다양한 Python 버전을 관리 할 수 있습니다. 1) PYENV를 사용하여 여러 Python 버전을 관리합니다. Pyenv를 설치하고 글로벌 및 로컬 버전을 설정하십시오. 2) VENV를 사용하여 프로젝트 종속성을 분리하기 위해 가상 환경을 만듭니다. 3) Anaconda를 사용하여 데이터 과학 프로젝트에서 Python 버전을 관리하십시오. 4) 시스템 수준의 작업을 위해 시스템 파이썬을 유지하십시오. 이러한 도구와 전략을 통해 다양한 버전의 Python을 효과적으로 관리하여 프로젝트의 원활한 실행을 보장 할 수 있습니다.
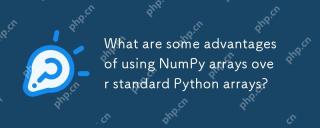
Numpyarrayshaveseveraladvantagesstandardpythonarrays : 1) thearemuchfasterduetoc 기반 간증, 2) thearemorememory-refficient, 특히 withlargedatasets 및 3) wepferoptizedformationsformationstaticaloperations, 만들기, 만들기


핫 AI 도구

Undresser.AI Undress
사실적인 누드 사진을 만들기 위한 AI 기반 앱

AI Clothes Remover
사진에서 옷을 제거하는 온라인 AI 도구입니다.

Undress AI Tool
무료로 이미지를 벗다

Clothoff.io
AI 옷 제거제

Video Face Swap
완전히 무료인 AI 얼굴 교환 도구를 사용하여 모든 비디오의 얼굴을 쉽게 바꾸세요!

인기 기사

뜨거운 도구

mPDF
mPDF는 UTF-8로 인코딩된 HTML에서 PDF 파일을 생성할 수 있는 PHP 라이브러리입니다. 원저자인 Ian Back은 자신의 웹 사이트에서 "즉시" PDF 파일을 출력하고 다양한 언어를 처리하기 위해 mPDF를 작성했습니다. HTML2FPDF와 같은 원본 스크립트보다 유니코드 글꼴을 사용할 때 속도가 느리고 더 큰 파일을 생성하지만 CSS 스타일 등을 지원하고 많은 개선 사항이 있습니다. RTL(아랍어, 히브리어), CJK(중국어, 일본어, 한국어)를 포함한 거의 모든 언어를 지원합니다. 중첩된 블록 수준 요소(예: P, DIV)를 지원합니다.

SecList
SecLists는 최고의 보안 테스터의 동반자입니다. 보안 평가 시 자주 사용되는 다양한 유형의 목록을 한 곳에 모아 놓은 것입니다. SecLists는 보안 테스터에게 필요할 수 있는 모든 목록을 편리하게 제공하여 보안 테스트를 더욱 효율적이고 생산적으로 만드는 데 도움이 됩니다. 목록 유형에는 사용자 이름, 비밀번호, URL, 퍼징 페이로드, 민감한 데이터 패턴, 웹 셸 등이 포함됩니다. 테스터는 이 저장소를 새로운 테스트 시스템으로 간단히 가져올 수 있으며 필요한 모든 유형의 목록에 액세스할 수 있습니다.
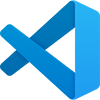
VSCode Windows 64비트 다운로드
Microsoft에서 출시한 강력한 무료 IDE 편집기

SublimeText3 중국어 버전
중국어 버전, 사용하기 매우 쉽습니다.
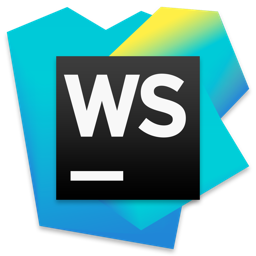
WebStorm Mac 버전
유용한 JavaScript 개발 도구
