초기화
# 检查是否已经安装以及版本号 >>> import sqlalchemy >>> sqlalchemy.__version__ ’1.1.4‘
>>> from sqlalchemy.ext.declarative import declarative_base # model都是要继承自Base >>> Base = declarative_base() >>> from sqlalchemy import Column, Integer, String >>> class User(Base): ... __tablename__ = 'users' # 指定数据表名 ... ... id = Column(Integer, primary_key=True) ... name = Column(String(50)) ... fullname = Column(String(50)) ... password = Column(String(50)) ... ... def __repr__(self): ... return "<user>" % ( ... self.name, self.fullname, self.password) # 查看创建的数据表结构 >>> User.__table__ Table('users', MetaData(bind=None), Column('id', Integer(), table=<users>, primary_key=True, nullable=False), Column('name', String(length=50), table=<users>), Column('fullname', String(length=50), table=<users>), Column('password', String(length=50), table=<users>), schema=None)</users></users></users></users></user>
데이터 테이블 정식 생성
>>> from sqlalchemy import create_engine # 连接到mysql >>> engine = create_engine("mysql://root:root@localhost:3306/python?charset=utf8", encoding="utf-8", echo=True) # 正式创建数据表 >>> Base.metadata.create_all(engine) CREATE TABLE users ( id INTEGER NOT NULL AUTO_INCREMENT, name VARCHAR(50), fullname VARCHAR(50), password VARCHAR(50), PRIMARY KEY (id) )
세션 생성
다음 작업은 모두 세션 개체 작업을 통해 수행됩니다
>>> from sqlalchemy.orm import sessionmaker >>> Session = sessionmaker(bind=engine) >>> session = Session()
개체 추가 및 업데이트
사용자 개체 추가
>>> ed_user = User(name='ed', fullname='Ed Jones', password='edspassword') >>> session.add(ed_user)
질의하고 filter_by
를 사용하여 필터링하고 first
첫 번째 쿼리된 개체만 나열
>>> our_user = session.query(User).filter_by(name='ed').first() BEGIN (implicit) INSERT INTO users (name, fullname, password) VALUES (?, ?, ?) ('ed', 'Ed Jones', 'edspassword') SELECT users.id AS users_id, users.name AS users_name, users.fullname AS users_fullname, users.password AS users_password FROM users WHERE users.name = ? LIMIT ? OFFSET ? ('ed', 1, 0) >>> our_user <user> >>> ed_user is our_user True</user>
, 한 번에 여러 개체 추가 add_all
>>> session.add_all([ ... User(name='wendy', fullname='Wendy Williams', password='foobar'), ... User(name='mary', fullname='Mary Contrary', password='xxg527'), ... User(name='fred', fullname='Fred Flinstone', password='blah')])세션은 매우 똑똑합니다. 예를 들어 Ed Jones가 수정되었다는 것을 알고 있습니다.
# 可以直接修改ed_user对象 >>> ed_user.password = 'f8s7ccs' # session会自动知道哪些数据被修改了 >>> session.dirty IdentitySet([<user>]) # session也可以知道哪些对象被新建了 >>> session.new IdentitySet([<user>, <user>, <user>])</user></user></user></user>가 데이터베이스를 변경했기 때문에 자연스럽게 done
, commit
문에서 객체 1개를 업데이트하고 객체 3개를 생성한 것을 볼 수 있습니다. echo
>>> session.commit() UPDATE users SET password=? WHERE users.id = ? ('f8s7ccs', 1) INSERT INTO users (name, fullname, password) VALUES (?, ?, ?) ('wendy', 'Wendy Williams', 'foobar') INSERT INTO users (name, fullname, password) VALUES (?, ?, ?) ('mary', 'Mary Contrary', 'xxg527') INSERT INTO users (name, fullname, password) VALUES (?, ?, ?) ('fred', 'Fred Flinstone', 'blah') COMMIT >>> ed_user.id BEGIN (implicit) SELECT users.id AS users_id, users.name AS users_name, users.fullname AS users_fullname, users.password AS users_password FROM users WHERE users.id = ? (1,) 1롤백세션이 트랜잭션 내에서 작동하기 때문에 때때로 실수로 일부 삭제 작업을 수행하여 롤백할 수 있습니다. 먼저 ed_user의 사용자 이름을
으로 변경한 다음 새 사용자를 추가합니다. 하지만 현재로서는 아직 Edwardo
이 없다는 점을 기억하세요. commit
>>> ed_user.name = 'Edwardo' and we’ll add another erroneous user, fake_user: >>> fake_user = User(name='fakeuser', fullname='Invalid', password='12345') >>> session.add(fake_user) Querying the session, we can see that they’re flushed into the current transaction:쿼리하고
>>> session.query(User).filter(User.name.in_(['Edwardo', 'fakeuser'])).all() UPDATE users SET name=? WHERE users.id = ? ('Edwardo', 1) INSERT INTO users (name, fullname, password) VALUES (?, ?, ?) ('fakeuser', 'Invalid', '12345') SELECT users.id AS users_id, users.name AS users_name, users.fullname AS users_fullname, users.password AS users_password FROM users WHERE users.name IN (?, ?) ('Edwardo', 'fakeuser') [<user>, <user>]</user></user>롤백을 확인하면 ed_user의 이름이
으로 돌아왔고 ed
이 fake_user
세션에서 쫓겨났음을 알 수 있습니다.
>>> session.rollback() ROLLBACK >>> ed_user.name BEGIN (implicit) SELECT users.id AS users_id, users.name AS users_name, users.fullname AS users_fullname, users.password AS users_password FROM users WHERE users.id = ? (1,) u'ed' >>> fake_user in session False issuing a SELECT illustrates the changes made to the database:이때 다시 쿼리해보면 가짜 사용자가 사라진 것이 확실합니다.
사용자 이름이 ed
ed
>>> session.query(User).filter(User.name.in_(['ed', 'fakeuser'])).all() SELECT users.id AS users_id, users.name AS users_name, users.fullname AS users_fullname, users.password AS users_password FROM users WHERE users.name IN (?, ?) ('ed', 'fakeuser') [<user>]</user>
Edwordo
커팅으로 다시 변경되었습니다. >query 연산에 해당하는 count() 연산의 경우
>>> session.query(User).filter(User.name.like('%ed')).count() 2 >>> from sqlalchemy import func >>> session.query(func.count(User.name), User.name).group_by(User.name).all() [(1, u'ed'), (1, u'fred'), (1, u'mary'), (1, u'wendy')]
Querying
객체query
는 Query
메소드를 사용하여 생성할 수 있습니다. 사용자 ID별로 정렬하여
>>> for instance in session.query(User).order_by(User.id): ... print(instance.name, instance.fullname) ed Ed Jones wendy Wendy Williams mary Mary Contrary fred Fred Flinstone
쿼리 방법에 따라 세션에서 ORM으로 계측된 설명자를 매개변수로 받을 수도 있습니다. Query에서 반환된 튜플은 KeyedTuple 클래스에서 제공하는 명명된 튜플이며 일반 Python 객체와 매우 유사하게 처리될 수 있습니다. 이름은 속성의 속성 이름 및 클래스의 클래스 이름과 동일합니다.
>>> for name, fullname in session.query(User.name, User.fullname): ... print(name, fullname) ed Ed Jones wendy Wendy Williams mary Mary Contrary fred Fred Flinstone
모든 ColumnElement 파생 객체에서 사용할 수 있는
구문과 하나에 매핑되는 모든 클래스 속성(예: User.name)을 사용하여 개별 열 표현식의 이름을 제어할 수 있습니다.>>> for row in session.query(User, User.name).all(): ... print(row.User, row.name) <user> ed <user> wendy <user> mary <user> fred</user></user></user></user>query() 호출에 여러 엔터티가 존재한다고 가정할 때 User와 같은 전체 엔터티에 부여된 이름은
:label()
>>> for row in session.query(User.name.label('name_label')).all(): ... print(row.name_label) ed wendy mary fredQuery의 기본 작업을 사용하여 제어할 수 있습니다. 가장 편리하게 Python 배열 슬라이스를 사용하고 일반적으로
:aliased()
>>> from sqlalchemy.orm import aliased >>> user_alias = aliased(User, name='user_alias') >>> for row in session.query(user_alias, user_alias.name).all(): ... print(row.user_alias) <user> <user> <user> <user></user></user></user></user>와 함께 LIMIT 및 OFFSET 실행을 포함합니다. Query 객체는 완전히 생성적입니다. 즉, 대부분의 메소드 호출은 추가 기준이 적용될 수 있는 새로운 Query 객체를 반환합니다. 예를 들어 이름이 "ed"이고 이름이 "Ed Jones"인 사용자를 쿼리하려면
를 두 번 호출하면 ORDER BY
:
>>> for u in session.query(User).order_by(User.id)[1:3]: ... print(u) <user> <user> and filtering results, which is accomplished either with filter_by(), which uses keyword arguments: >>> for name, in session.query(User.name).\ ... filter_by(fullname='Ed Jones'): ... print(name) ed >>> for name, in session.query(User.name).\ ... filter(User.fullname=='Ed Jones'): ... print(name) ed</user></user>
를 사용하여 기준을 조인할 수 있습니다. 가장 일반적으로 사용되는 연산자filter()
>>> for user in session.query(User).\ ... filter(User.name=='ed').\ ... filter(User.fullname=='Ed Jones'): ... print(user) <user> Common Filter Operators</user>
AND
관계 구축객체 간의 관계를 생성하려면 주소 테이블 아래에 새 관계를 생성해 보겠습니다. 다음 작업은 Django의 ORM보다 더 번거롭습니다. 동시에 두 클래스의 관계를 설정해야 합니다filter()
equals: query.filter(User.name == 'ed') not equals: query.filter(User.name != 'ed') LIKE: query.filter(User.name.like('%ed%')) IN: query.filter(User.name.in_(['ed', 'wendy', 'jack'])) # works with query objects too: query.filter(User.name.in_( session.query(User.name).filter(User.name.like('%ed%')) )) NOT IN: query.filter(User.name.in_(['ed', 'wendy', 'jack'])) IS NULL: query.filter(User.name == None) # alternatively, if pep8/linters are a concern query.filter(User.name.is_(None)) IS NOT NULL: query.filter(User.name != None) # alternatively, if pep8/linters are a concern query.filter(User.name.isnot(None)) AND: # use and_() from sqlalchemy import and_ query.filter(and_(User.name == 'ed', User.fullname == 'Ed Jones')) # or send multiple expressions to .filter() query.filter(User.name == 'ed', User.fullname == 'Ed Jones') # or chain multiple filter()/filter_by() calls query.filter(User.name == 'ed').filter(User.fullname == 'Ed Jones') Note Make sure you use and_() and not the Python and operator! OR: from sqlalchemy import or_ query.filter(or_(User.name == 'ed', User.name == 'wendy')) Note Make sure you use or_() and not the Python or operator! MATCH: query.filter(User.name.match('wendy')) Note match() uses a database-specific MATCH or CONTAINS function; its behavior will vary by backend and is not available on some backends such as SQLite.관련 개체 작업이제 사용자를 생성했으며 이에 해당하는 빈 주소 컬렉션도 생성됩니다. . 컬렉션 유형은 집합/사전과 같은 모든 법적 유형일 수 있지만(자세한 내용은 컬렉션 액세스 사용자 정의 참조) 기본 컬렉션은 목록입니다. 이제 다른 사용자 Jack을 만들어 보겠습니다
>>> from sqlalchemy import ForeignKey
>>> from sqlalchemy.orm import relationship
>>> class Address(Base):
... __tablename__ = 'addresses'
... id = Column(Integer, primary_key=True)
... email_address = Column(String(50), nullable=False)
... user_id = Column(Integer, ForeignKey('users.id'))
...
... user = relationship("User", back_populates="addresses") # 将地址表和用户表关联
...
... def __repr__(self):
... return "<address>" % self.email_address
# 在用户表中还要重新设置一次
>>> User.addresses = relationship(
... "Address", order_by=Address.id, back_populates="user")
>>> Base.metadata.create_all(engine)</address>
User 개체에 Address 개체를 자유롭게 추가할 수 있습니다. 이 경우 전체 목록을 직접 할당하면 됩니다.이제 우리는 사용자 Jack을 일부 주소와 연결
>>> jack = User(name='jack', fullname='Jack Bean', password='gjffdd') >>> jack.addresses []양방향 관계를 사용할 때 한 방향으로 추가된 요소는 자동으로 다른 방향에서 표시됩니다. 이 동작은 속성 변경 시 이벤트를 기반으로 발생하며 Python에서 평가됩니다. SQL 사용:
이제 주소 개체를 통해 사용자 개체에 액세스할 수 있습니다
>>> jack.addresses = [ ... Address(email_address='jack@google.com'), ... Address(email_address='j25@yahoo.com')]
Jack Bean을 데이터베이스에 추가하고 커밋하고 해당 주소 컬렉션의 두 주소 멤버는 모두 있습니다. 캐스케이딩이라는 프로세스를 사용하여 한 번에 세션에 추가됩니다.
다음
데이터베이스에 저장
>>> jack.addresses[1] <address> >>> jack.addresses[1].user <user></user> </address>
Jack을 쿼리하면 아직 Jack이 반환되지 않습니다. Jack의 주소:
>>> session.add(jack) >>> session.commit() sqlalchemy.engine.base.Engine INSERT INTO addresses (email_address, user_id) VALUES (%s, %s) sqlalchemy.engine.base.Engine ('jack@google.com', 5L) sqlalchemy.engine.base.Engine INSERT INTO addresses (email_address, user_id) VALUES (%s, %s) sqlalchemy.engine.base.Engine ('j25@yahoo.com', 5L) sqlalchemy.engine.base.Engine COMMIT
주소 컬렉션에 액세스했을 때 SQL이 갑자기 실행되었습니다. 이는 지연 로딩 관계의 예입니다. 이제 주소 컬렉션이 로드되어 일반 목록처럼 작동합니다. 이 컬렉션의 로딩을 약간 최적화하는 방법입니다.commit
>>> session.delete(jack) >>> session.query(User).filter_by(name='jack').count() 0 So far, so good. How about Jack’s Address objects ? >>> session.query(Address).filter( ... Address.email_address.in_(['jack@google.com', 'j25@yahoo.com']) ... ).count() 2
Uh oh, they’re still there ! Analyzing the flush SQL, we can see that the user_id column of each address was set to NULL, but the rows weren’t deleted
. SQLAlchemy doesn’t assume that deletes cascade
, you have to tell it to do so. Configuring delete/delete-orphan Cascade
. We will configure cascade options on the User.addresses relationship
to change the behavior. While SQLAlchemy allows you to add new attributes and relationships to mappings at any point in time, in this case the existing relationship needs to be removed, so we need to tear down the mappings completely and start again - we’ll close the Session:
直接close来rollback,并不进行commit
>>> session.close() ROLLBACK
Use a new declarative_base():
>>> Base = declarative_base()
Next we’ll declare the User class, adding in the addresses relationship
including the cascade configuration (we’ll leave the constructor out too):
>>> class User(Base): ... __tablename__ = 'users' ... ... id = Column(Integer, primary_key=True) ... name = Column(String(50)) ... fullname = Column(String(50)) ... password = Column(String(50)) ... ... addresses = relationship("Address", back_populates='user', ... cascade="all, delete, delete-orphan") ... ... def __repr__(self): ... return "<user>" % ( ... self.name, self.fullname, self.password)</user>
Then we recreate Address, noting that in this case
we’ve created the Address.user relationship via the User class already:
>>> class Address(Base): ... __tablename__ = 'addresses' ... id = Column(Integer, primary_key=True) ... email_address = Column(String(50), nullable=False) ... user_id = Column(Integer, ForeignKey('users.id')) ... user = relationship("User", back_populates="addresses") ... ... def __repr__(self): ... return "<address>" % self.email_address</address>
Now when we load the user jack (below using get(), which loads by primary key), removing an address from the corresponding addresses collection will result in that Address being deleted:
# load Jack by primary key >>> jack = session.query(User).get(5) # remove one Address (lazy load fires off) >>> del jack.addresses[1] # only one address remains >>> session.query(Address).filter( ... Address.email_address.in_(['jack@google.com', 'j25@yahoo.com']) ... ).count() 1
Deleting Jack will delete both Jack and the remaining Address associated with the user:
>>> session.delete(jack) >>> session.query(User).filter_by(name='jack').count() 0 >>> session.query(Address).filter( ... Address.email_address.in_(['jack@google.com', 'j25@yahoo.com']) ... ).count() 0
Further detail on configuration of cascades is at Cascades. The cascade functionality can also integrate smoothly with the ON DELETE CASCADE functionality of the relational database. See Using Passive Deletes for details.
backref
上面同时设置两个relationship太麻烦了,可以使用backref
from sqlalchemy import Integer, ForeignKey, String, Column from sqlalchemy.ext.declarative import declarative_base from sqlalchemy.orm import relationship Base = declarative_base() class User(Base): __tablename__ = 'user' id = Column(Integer, primary_key=True) name = Column(String) addresses = relationship("Address", backref="user") class Address(Base): __tablename__ = 'address' id = Column(Integer, primary_key=True) email = Column(String) user_id = Column(Integer, ForeignKey('user.id'))
The above configuration establishes a collection of Address objects on User called User.addresses
. It also establishes a .user
attribute on Address which will refer to the parent User object.
In fact, the backref keyword is only a common shortcut for placing a second relationship() onto the Address mapping, including the establishment of an event listener on both sides which will mirror attribute operations in both directions. The above configuration is equivalent to:
rom sqlalchemy import Integer, ForeignKey, String, Column from sqlalchemy.ext.declarative import declarative_base from sqlalchemy.orm import relationship Base = declarative_base() class User(Base): __tablename__ = 'user' id = Column(Integer, primary_key=True) name = Column(String) addresses = relationship("Address", back_populates="user") class Address(Base): __tablename__ = 'address' id = Column(Integer, primary_key=True) email = Column(String) user_id = Column(Integer, ForeignKey('user.id')) user = relationship("User", back_populates="addresses")
Above, we add a .user relationship to Address explicitly. On both relationships, the back_populates
directive tells each relationship about the other one, indicating that they should establish “bidirectional” behavior between each other. The primary effect of this configuration is that the relationship adds event handlers to both attributes which have the behavior of “when an append or set event occurs here, set ourselves onto the incoming attribute using this particular attribute name”. The behavior is illustrated as follows. Start with a User and an Address instance. The .addresses collection
is empty, and the .user attribute is None
:
>>> u1 = User() >>> a1 = Address() >>> u1.addresses [] >>> print(a1.user) None
However, once the Address is appended to the u1.addresses collection, both the collection and the scalar attribute have been populated:
>>> u1.addresses.append(a1) >>> u1.addresses [<__main__.address>] >>> a1.user <__main__.user></__main__.user></__main__.address>
This behavior of course works in reverse for removal operations as well, as well as for equivalent operations on both sides. Such as when .user is set again to None, the Address object is removed from the reverse collection:
>>> a1.user = None >>> u1.addresses []
The manipulation of the .addresses collection and the .user attribute occurs entirely in Python without any interaction with the SQL database. Without this behavior, the proper state would be apparent on both sides once the data has been flushed to the database, and later reloaded after a commit or expiration operation occurs. The backref/back_populates behavior has the advantage that common bidirectional operations can reflect the correct state without requiring a database round trip.
Remember, when the backref keyword is used on a single relationship, it’s exactly the same as if the above two relationships were created inpidually using back_populates on each.
mysql操作
检验一下我们上面的成果以及熟悉创建的mysql表的结构
地址表的结构
> SHOW CREATE TABLE addresses; +-----------+----------------+ | Table | Create Table | |-----------+----------------| | addresses | CREATE TABLE `addresses` ( `id` int(11) NOT NULL AUTO_INCREMENT, `email_address` varchar(50) NOT NULL, `user_id` int(11) DEFAULT NULL, PRIMARY KEY (`id`), KEY `user_id` (`user_id`), CONSTRAINT `addresses_ibfk_1` FOREIGN KEY (`user_id`) REFERENCES `users` (`id`) ) ENGINE=InnoDB AUTO_INCREMENT=5 DEFAULT CHARSET=utf8 | +-----------+----------------+ 1 row in set Time: 0.005s > DESC addresses; +---------------+-------------+--------+-------+-----------+----------------+ | Field | Type | Null | Key | Default | Extra | |---------------+-------------+--------+-------+-----------+----------------| | id | int(11) | NO | PRI | <null> | auto_increment | | email_address | varchar(50) | NO | | <null> | | | user_id | int(11) | YES | MUL | <null> | | +---------------+-------------+--------+-------+-----------+----------------+ 3 rows in set Time: 0.002s</null></null></null>
用户表的结构
> SHOW CREATE TABLE users; +---------+----------------+ | Table | Create Table | |---------+----------------| | users | CREATE TABLE `users` ( `id` int(11) NOT NULL AUTO_INCREMENT, `name` varchar(50) DEFAULT NULL, `fullname` varchar(50) DEFAULT NULL, `password` varchar(50) DEFAULT NULL, PRIMARY KEY (`id`) ) ENGINE=InnoDB AUTO_INCREMENT=6 DEFAULT CHARSET=utf8 | +---------+----------------+ 1 row in set Time: 0.002s > DESC users; +----------+-------------+--------+-------+-----------+----------------+ | Field | Type | Null | Key | Default | Extra | |----------+-------------+--------+-------+-----------+----------------| | id | int(11) | NO | PRI | <null> | auto_increment | | name | varchar(50) | YES | | <null> | | | fullname | varchar(50) | YES | | <null> | | | password | varchar(50) | YES | | <null> | | +----------+-------------+--------+-------+-----------+----------------+ 4 rows in set Time: 0.003s</null></null></null></null>
详细数据
> SELECT * FROM addresses; +------+-----------------+-----------+ | id | email_address | user_id | |------+-----------------+-----------| | 3 | jack@google.com | 5 | | 4 | j25@yahoo.com | 5 | +------+-----------------+-----------+ 2 rows in set Time: 0.002s > SELECT * FROM users; +------+--------+----------------+------------+ | id | name | fullname | password | |------+--------+----------------+------------| | 1 | ed | Ed Jones | f8s7ccs | | 2 | wendy | Wendy Williams | foobar | | 3 | mary | Mary Contrary | xxg527 | | 4 | fred | Fred Flinstone | blah | | 5 | jack | Jack Bean | gjffdd | +------+--------+----------------+------------+ 5 rows in set Time: 0.003s
知乎live设计模型
from sqlalchemy import Column, String, Integer, create_engine, SmallInteger from sqlalchemy.orm import sessionmaker from sqlalchemy.ext.declarative import declarative_base DB_URI = 'sqlite:///user.db' Base = declarative_base() engine = create_engine(DB_URI) Base.metadata.bind = engine Session = sessionmaker(bind=engine) session = Session()
class User(Base): __tablename__ = 'live_user' id = Column(Integer, unique=True, primary_key=True, autoincrement=True) speaker_id = Column(String(40), index=True, unique=True) name = Column(String(40), index=True, nullable=False) gender = Column(SmallInteger, default=2) headline = Column(String(200)) avatar_url = Column(String(100), nullable=False) bio = Column(String(200)) description = Column(String()) @classmethod def add(cls, **kwargs): speaker_id = kwargs.get('speaker_id', None) if id is not None: r = session.query(cls).filter_by(speaker_id=speaker_id).first() if r: return r try: r = cls(**kwargs) session.add(r) session.commit() except: session.rollback() raise else: return r
Base.metadata.create_all()
接口分为2种:
http://www.php.cn/ (未结束)
http://www.php.cn/ (已结束)
elasticsearch-dsl-py相比elasticsearch-py做了各种封装,DSL也支持用类代表一个doc_type(类似数据库中的Table),实现ORM的效果。我们就用它来写Live模型:
from elasticsearch_dsl import DocType, Date, Integer, Text, Float, Boolean from elasticsearch_dsl.connections import connections from elasticsearch_dsl.query import SF, Q from config import SEARCH_FIELDS from .speaker import User, session connections.create_connection(hosts=['localhost'])
class Live(DocType): id = Integer() speaker_id = Integer() feedback_score = Float() # 评分 topic_names = Text(analyzer='ik_max_word') # 话题标签名字 seats_taken = Integer() # 参与人数 subject = Text(analyzer='ik_max_word') # 标题 amount = Float() # 价格(RMB) description = Text(analyzer='ik_max_word') status = Boolean() # public(True)/ended(False) starts_at = Date() outline = Text(analyzer='ik_max_word') # Live内容 speaker_message_count = Integer() tag_names = Text(analyzer='ik_max_word') liked_num = Integer() class Meta: index = 'live' @classmethod def add(cls, **kwargs): id = kwargs.pop('id', None) if id is None: return False live = cls(meta={'id': id}, **kwargs) live.save() return live
它允许我们用一种非常可维护的方法来组织字典:
In : from elasticsearch_dsl.query import Q In : Q('multi_match', subject='python').to_dict() Out: {'multi_match': {'subject': 'python'}}
In : from elasticsearch import Elasticsearch In : from elasticsearch_dsl import Search, Q In : s = Search(using=client, index='live') In : s = s.query('match', subject='python').query(~Q('match', description='量化')) In : s.execute() Out: <response:>]></response:>
上述例子表示从live这个索引(类似数据库中的Database)中找到subject字典包含python,但是description字段不包含量化的Live。
更多Python-SQLALchemy 相关文章请关注PHP中文网!
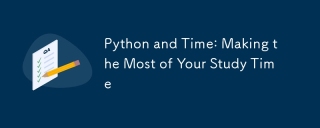
제한된 시간에 Python 학습 효율을 극대화하려면 Python의 DateTime, Time 및 Schedule 모듈을 사용할 수 있습니다. 1. DateTime 모듈은 학습 시간을 기록하고 계획하는 데 사용됩니다. 2. 시간 모듈은 학습과 휴식 시간을 설정하는 데 도움이됩니다. 3. 일정 모듈은 주간 학습 작업을 자동으로 배열합니다.
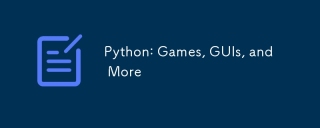
Python은 게임 및 GUI 개발에서 탁월합니다. 1) 게임 개발은 Pygame을 사용하여 드로잉, 오디오 및 기타 기능을 제공하며 2D 게임을 만드는 데 적합합니다. 2) GUI 개발은 Tkinter 또는 PYQT를 선택할 수 있습니다. Tkinter는 간단하고 사용하기 쉽고 PYQT는 풍부한 기능을 가지고 있으며 전문 개발에 적합합니다.
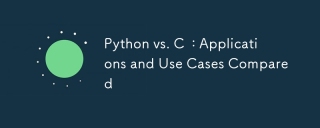
Python은 데이터 과학, 웹 개발 및 자동화 작업에 적합한 반면 C는 시스템 프로그래밍, 게임 개발 및 임베디드 시스템에 적합합니다. Python은 단순성과 강력한 생태계로 유명하며 C는 고성능 및 기본 제어 기능으로 유명합니다.
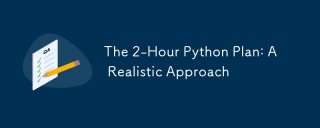
2 시간 이내에 Python의 기본 프로그래밍 개념과 기술을 배울 수 있습니다. 1. 변수 및 데이터 유형을 배우기, 2. 마스터 제어 흐름 (조건부 명세서 및 루프), 3. 기능의 정의 및 사용을 이해하십시오. 4. 간단한 예제 및 코드 스 니펫을 통해 Python 프로그래밍을 신속하게 시작하십시오.
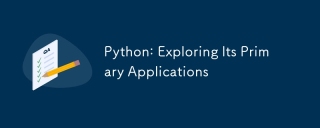
Python은 웹 개발, 데이터 과학, 기계 학습, 자동화 및 스크립팅 분야에서 널리 사용됩니다. 1) 웹 개발에서 Django 및 Flask 프레임 워크는 개발 프로세스를 단순화합니다. 2) 데이터 과학 및 기계 학습 분야에서 Numpy, Pandas, Scikit-Learn 및 Tensorflow 라이브러리는 강력한 지원을 제공합니다. 3) 자동화 및 스크립팅 측면에서 Python은 자동화 된 테스트 및 시스템 관리와 같은 작업에 적합합니다.
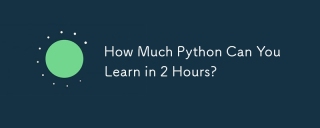
2 시간 이내에 파이썬의 기본 사항을 배울 수 있습니다. 1. 변수 및 데이터 유형을 배우십시오. 이를 통해 간단한 파이썬 프로그램 작성을 시작하는 데 도움이됩니다.
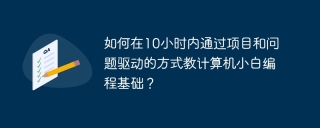
10 시간 이내에 컴퓨터 초보자 프로그래밍 기본 사항을 가르치는 방법은 무엇입니까? 컴퓨터 초보자에게 프로그래밍 지식을 가르치는 데 10 시간 밖에 걸리지 않는다면 무엇을 가르치기로 선택 하시겠습니까?
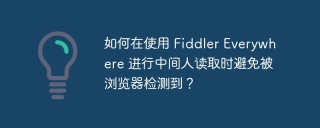
Fiddlerevery Where를 사용할 때 Man-in-the-Middle Reading에 Fiddlereverywhere를 사용할 때 감지되는 방법 ...


핫 AI 도구

Undresser.AI Undress
사실적인 누드 사진을 만들기 위한 AI 기반 앱

AI Clothes Remover
사진에서 옷을 제거하는 온라인 AI 도구입니다.

Undress AI Tool
무료로 이미지를 벗다

Clothoff.io
AI 옷 제거제

AI Hentai Generator
AI Hentai를 무료로 생성하십시오.

인기 기사

뜨거운 도구

Eclipse용 SAP NetWeaver 서버 어댑터
Eclipse를 SAP NetWeaver 애플리케이션 서버와 통합합니다.
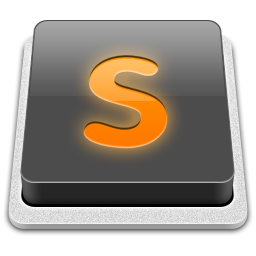
SublimeText3 Mac 버전
신 수준의 코드 편집 소프트웨어(SublimeText3)

Atom Editor Mac 버전 다운로드
가장 인기 있는 오픈 소스 편집기
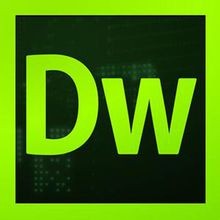
드림위버 CS6
시각적 웹 개발 도구
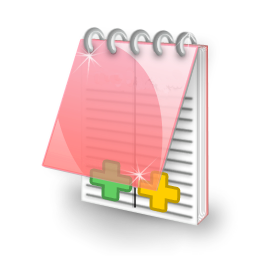
에디트플러스 중국어 크랙 버전
작은 크기, 구문 강조, 코드 프롬프트 기능을 지원하지 않음
