With the new age of problem-solving augmented by Large Language Models (LLMs), only a handful of problems remain that have subpar solutions. Most classification problems (at a PoC level) can be solved by leveraging LLMs at 70–90% Precision/F1 with just good prompt engineering techniques, as well as adaptive in-context-learning (ICL) examples.
What happens when you want to consistently achieve performancehigher than that — when prompt engineering no longer suffices?
The classification conundrum
Text classification is one of the oldest and most well-understood examples of supervised learning. Given this premise, it shouldreallynot be hard to build robust, well-performing classifiers that handle a large number of input classes, right…?
Welp. It is.
It actually has to do a lot more with the ‘constraints’ that the algorithm is generally expected to work under:
- low amount of training data per class
- high classification accuracy (that plummets as you add more classes)
- possible addition ofnew classesto an existing subset of classes
- quick training/inference
- cost-effectiveness
- (potentially) really large number of training classes
- (potentially) endlessrequiredretraining ofsomeclasses due to data drift, etc.
Ever tried building a classifier beyond a few dozen classes under these conditions? (I mean, even GPT could probably do a great job up to ~30 text classes with just a few samples…)
Considering you take the GPT route — If you have more than a couple dozen classes or a sizeable amount of data to be classified, you are gonna have to reach deep into your pockets with the system prompt, user prompt, few shot example tokens that you will need to classifyone sample.That is after making peace with the throughput of the API, even if you are running async queries.
In applied ML, problems like these are generally tricky to solve since they don’t fully satisfy the requirements of supervised learning or aren’t cheap/fast enough to be run via an LLM. This particular pain point is what the R.E.D algorithm addresses: semi-supervised learning, when the training data per class is not enough to build (quasi)traditional classifiers.
The R.E.D. algorithm
R.E.D: Recursive Expert Delegationis a novel framework that changes how we approach text classification. This is an applied ML paradigm — i.e., there is nofundamentally differentarchitecture to what exists, but its a highlight reel of ideas that work best to build something that is practical and scalable.
In this post, we will be working through a specific example where we have a large number of text classes (100–1000), each class only has few samples (30–100), and there are a non-trivial number of samples to classify (10,000–100,000). We approach this as asemi-supervised learningproblem via R.E.D.
Let’s dive in.
How it works
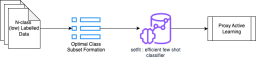
Instead of having a single classifier classify between a large number of classes, R.E.D. intelligently:
- Divides and conquers— Break the label space (large number of input labels) into multiple subsets of labels. This is a greedy label subset formation approach.
- Learns efficiently— Trains specialized classifiers for each subset. This step focuses on building a classifier that oversamples on noise, where noise is intelligently modeled as data fromother subsets.
- Delegates to an expert— Employes LLMs as expert oracles for specific label validation and correction only, similar to having a team of domain experts. Using an LLM as a proxy, it empirically ‘mimics’howa human expert validates an output.
- Recursive retraining— Continuously retrains with fresh samples added back from the expert until there are no more samples to be added/a saturation from information gain is achieved
The intuition behind it is not very hard to grasp:Active Learningemploys humans as domain experts to consistently ‘correct’ or ‘validate’ the outputs from an ML model, with continuous training. This stops when the model achieves acceptable performance. We intuit and rebrand the same, with a few clever innovations that will be detailed in a research pre-print later.
Let’s take a deeper look…
Greedy subset selection with least similar elements
When the number of input labels (classes) is high, the complexity of learning a linear decision boundary between classes increases. As such, the quality of the classifier deteriorates as the number of classes increases. This is especially true when the classifier does not have enoughsamplesto learn from — i.e. each of the training classes has only a few samples.
This is very reflective of a real-world scenario, and the primary motivation behind the creation of R.E.D.
Some ways of improving a classifier’s performance under these constraints:
- Restrictthe number of classes a classifier needs to classify between
- Make the decision boundary between classes clearer, i.e., train the classifier onhighly dissimilar classes
Greedy Subset Selection does exactly this — since the scope of the problem is Text Classification, we form embeddings of the training labels, reduce their dimensionality via UMAP, then formSsubsets from them. Each of theSsubsets has elements asntraining labels. We pick training labels greedily, ensuring that every label we pick for the subset is the most dissimilar label w.r.t. the other labels that exist in the subset:
import numpy as np from sklearn.metrics.pairwise import cosine_similarity def avg_embedding(candidate_embeddings): return np.mean(candidate_embeddings, axis=0) def get_least_similar_embedding(target_embedding, candidate_embeddings): similarities = cosine_similarity(target_embedding, candidate_embeddings) least_similar_index = np.argmin(similarities) # Use argmin to find the index of the minimum least_similar_element = candidate_embeddings[least_similar_index] return least_similar_element def get_embedding_class(embedding, embedding_map): reverse_embedding_map = {value: key for key, value in embedding_map.items()} return reverse_embedding_map.get(embedding) # Use .get() to handle missing keys gracefully def select_subsets(embeddings, n): visited = {cls: False for cls in embeddings.keys()} subsets = [] current_subset = [] while any(not visited[cls] for cls in visited): for cls, average_embedding in embeddings.items(): if not current_subset: current_subset.append(average_embedding) visited[cls] = True elif len(current_subset) >= n: subsets.append(current_subset.copy()) current_subset = [] else: subset_average = avg_embedding(current_subset) remaining_embeddings = [emb for cls_, emb in embeddings.items() if not visited[cls_]] if not remaining_embeddings: break # handle edge case least_similar = get_least_similar_embedding(target_embedding=subset_average, candidate_embeddings=remaining_embeddings) visited_class = get_embedding_class(least_similar, embeddings) if visited_class is not None: visited[visited_class] = True current_subset.append(least_similar) if current_subset: # Add any remaining elements in current_subset subsets.append(current_subset) return subsets
the result of this greedy subset sampling is all the training labels clearly boxed into subsets, where each subset has at most onlynclasses. This inherently makes the job of a classifier easier, compared to the originalSclasses it would have to classify between otherwise!
Semi-supervised classification with noise oversampling
Cascade this after the initial label subset formation — i.e., this classifier is only classifying between a givensubsetof classes.
Picture this: when you have low amounts of training data, you absolutely cannot create a hold-out set that is meaningful for evaluation. Should you do it at all? How do you know if your classifier is working well?
We approached this problem slightly differently — we defined the fundamental job of a semi-supervised classifier to bepre-emptive classification of a sample. This means that regardless of what a sample gets classified as it will be ‘verified’ and ‘corrected’ at a later stage: this classifier only needs to identify what needs to be verified.
As such, we created a design for how it would treat its data:
- n+1classes, where the last class isnoise
- noise:data from classes that are NOT in the current classifier’s purview. The noise class is oversampled to be 2x the average size of the data for the classifier’s labels
Oversampling on noise is a faux-safety measure, to ensure that adjacent data that belongs to another class is most likely predicted as noise instead of slipping through for verification.
How do you check if this classifier is working well — in our experiments, we define this as the number of ‘uncertain’ samples in a classifier’s prediction. Using uncertainty sampling and information gain principles, we were effectively able to gauge if a classifier is ‘learning’ or not, which acts as a pointer towards classification performance. This classifier is consistently retrained unless there is an inflection point in the number of uncertain samples predicted, or there is only a delta of information being added iteratively by new samples.
Proxy active learning via an LLM agent
This is the heart of the approach — using an LLM as a proxy for a human validator. The human validator approach we are talking about is Active Labelling
Let’s get an intuitive understanding of Active Labelling:
- Use an ML model to learn on a sample input dataset, predict on a large set of datapoints
- For the predictions given on the datapoints, a subject-matter expert (SME) evaluates ‘validity’ of predictions
- Recursively, new ‘corrected’ samples are added as training data to the ML model
- The ML model consistently learns/retrains, and makes predictions until the SME is satisfied by the quality of predictions
For Active Labelling to work, there are expectations involved for an SME:
- when we expect a human expert to ‘validate’ an output sample, the expert understands what the task is
- a human expert will use judgement to evaluate ‘what else’ definitely belongs to a labelLwhen deciding if a new sample should belong toL
Given these expectations and intuitions, we can ‘mimic’ these using an LLM:
- give the LLM an ‘understanding’ of what each label means. This can be done by using a larger model tocritically evaluate the relationship between {label: data mapped to label} for all labels. In our experiments, this was done using a32B variant of DeepSeekthat was self-hosted.
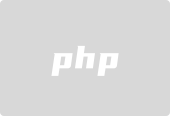
- Instead of predicting what is the correct label,leverage the LLM to identify if a prediction is ‘valid’ or ‘invalid’ only(i.e., LLM only has to answer a binary query).
- Reinforce the idea of what other valid samples for the label look like,i.e., for every pre-emptively predicted label for a sample, dynamically sourcecclosest samples in its training (guaranteed valid) set when prompting for validation.
The result? A cost-effective framework that relies on a fast, cheap classifier to make pre-emptive classifications, and an LLM that verifies these using (meaning of the label + dynamically sourced training samples that are similar to the current classification):
import math def calculate_uncertainty(clf, sample): predicted_probabilities = clf.predict_proba(sample.reshape(1, -1))[0] # Reshape sample for predict_proba uncertainty = -sum(p * math.log(p, 2) for p in predicted_probabilities) return uncertainty def select_informative_samples(clf, data, k): informative_samples = [] uncertainties = [calculate_uncertainty(clf, sample) for sample in data] # Sort data by descending order of uncertainty sorted_data = sorted(zip(data, uncertainties), key=lambda x: x[1], reverse=True) # Get top k samples with highest uncertainty for sample, uncertainty in sorted_data[:k]: informative_samples.append(sample) return informative_samples def proxy_label(clf, llm_judge, k, testing_data): #llm_judge - any LLM with a system prompt tuned for verifying if a sample belongs to a class. Expected output is a bool : True or False. True verifies the original classification, False refutes it predicted_classes = clf.predict(testing_data) # Select k most informative samples using uncertainty sampling informative_samples = select_informative_samples(clf, testing_data, k) # List to store correct samples voted_data = [] # Evaluate informative samples with the LLM judge for sample in informative_samples: sample_index = testing_data.tolist().index(sample.tolist()) # changed from testing_data.index(sample) because of numpy array type issue predicted_class = predicted_classes[sample_index] # Check if LLM judge agrees with the prediction if llm_judge(sample, predicted_class): # If correct, add the sample to voted data voted_data.append(sample) # Return the list of correct samples with proxy labels return voted_data
By feeding the valid samples (voted_data) to our classifier under controlled parameters, we achieve the ‘recursive’ part of our algorithm:
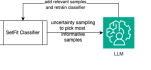
By doing this, we were able to achieve close-to-human-expert validation numbers on controlled multi-class datasets. Experimentally, R.E.D. scales up to1,000 classes while maintaining a competent degree of accuracyalmost on par with human experts (90%+ agreement).
I believe this is a significant achievement in applied ML, and has real-world uses for production-grade expectations of cost, speed, scale, and adaptability. The technical report, publishing later this year, highlights relevant code samples as well as experimental setups used to achieve given results.
All images, unless otherwise noted, are by the author
Interested in more details? Reach out to me over Medium or email for a chat!
위 내용은 R.E.D. : 전문가 대표단으로 텍스트 분류 스케일링의 상세 내용입니다. 자세한 내용은 PHP 중국어 웹사이트의 기타 관련 기사를 참조하세요!
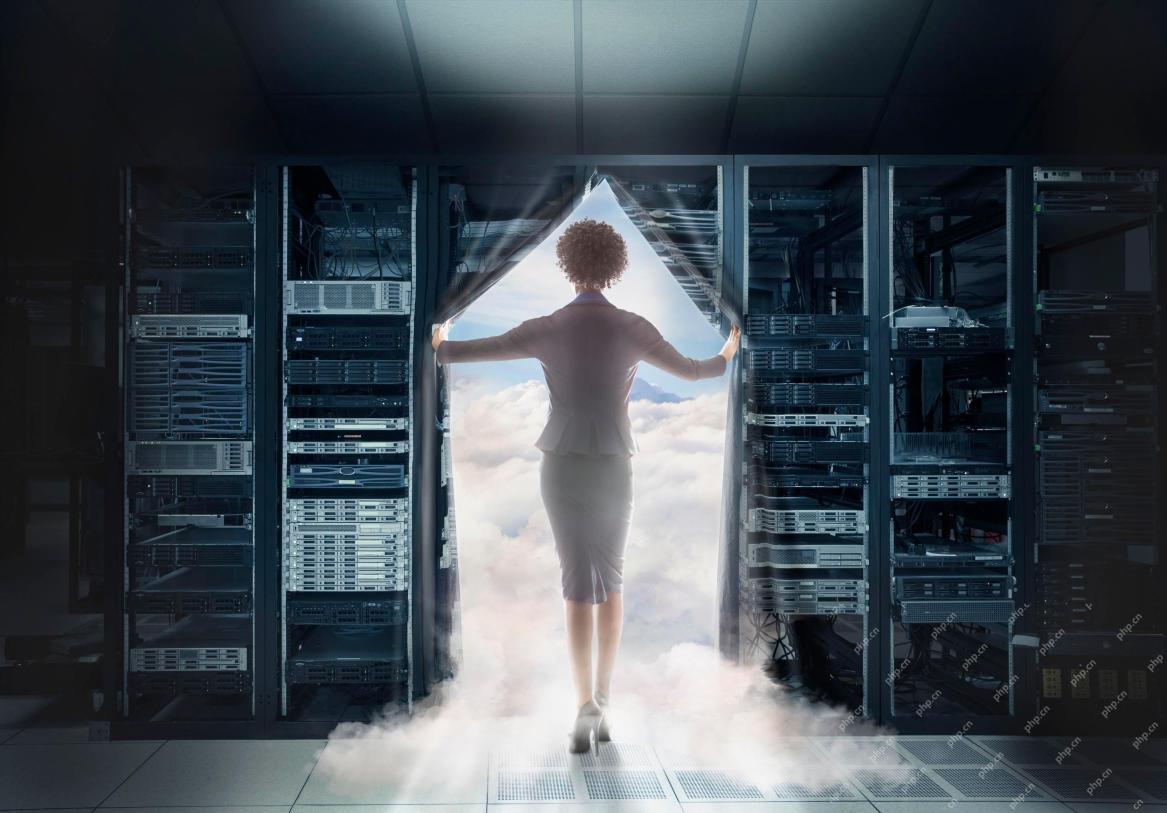
존 롤스 (John Rawls)의 1971 년 책 The Justice의 이론에서 그는 오늘날의 AI 디자인의 핵심으로 취해야 할 사고 실험을 제안하고 의사 결정 : 무지의 베일을 제안했다. 이 철학은 형평성을 이해하기위한 간단한 도구를 제공하며 리더 가이 이해를 사용하여 AI를 공평한 방식으로 설계하고 구현할 수있는 청사진을 제공합니다. 새로운 사회에 대한 규칙을 만들고 있다고 상상해보십시오. 그러나 전제가 있습니다.이 사회에서 어떤 역할을할지 미리 알 수 없습니다. 당신은 부자 또는 가난하거나 건강하거나 장애가있을 수 있으며 다수 또는 소수의 소수에 속할 수 있습니다. 이 "무지의 베일"하에 운영되면 규칙 제조업체가 스스로 이익을 얻는 결정을 내리지 못하게합니다. 반대로, 사람들은 대중을 공식화하도록 더 동기를 부여받을 것입니다
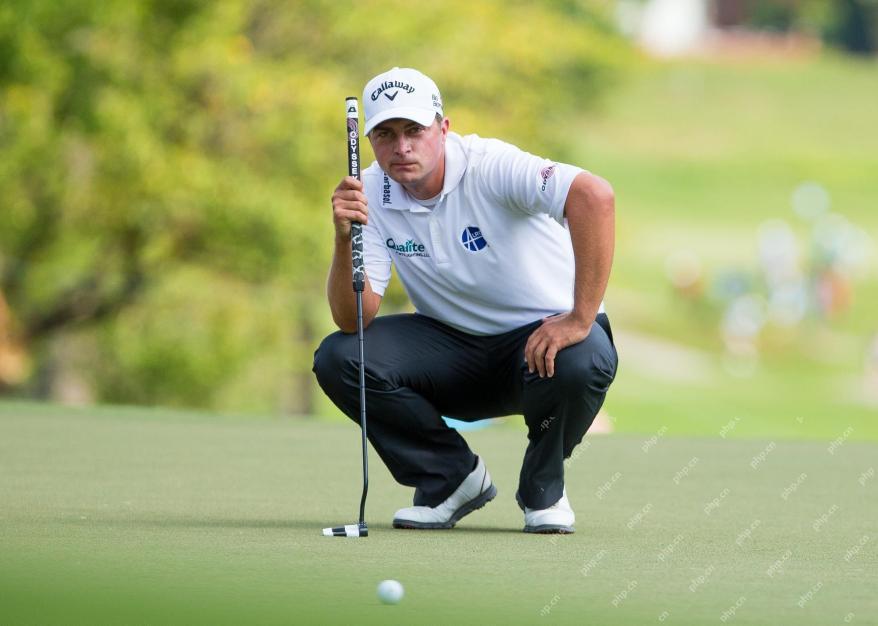
수많은 회사들이 로봇 프로세스 자동화 (RPA)를 전문으로하며, 반복적 인 작업과 같은 반복적 인 작업 (어디서나 자동화, 파란색 프리즘 등)를 제공하는 봇을 제공합니다. 한편, 프로세스 마이닝, 오케스트레이션 및 지능형 문서 처리 Speciali
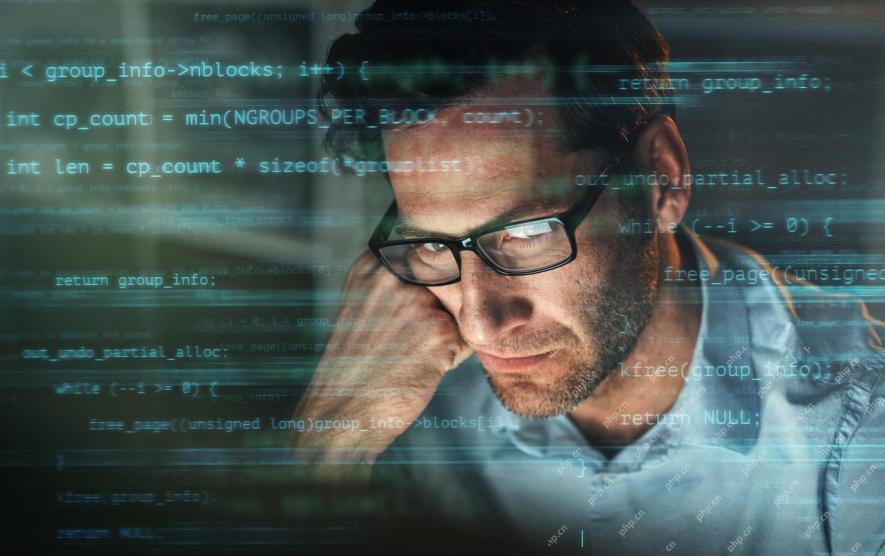
AI의 미래는 간단한 단어 예측과 대화 시뮬레이션을 넘어서고 있습니다. AI 에이전트는 새로운 행동 및 작업 완료가 가능합니다. 이러한 변화는 이미 Anthropic의 Claude와 같은 도구에서 분명합니다. AI 요원 : 연구 a
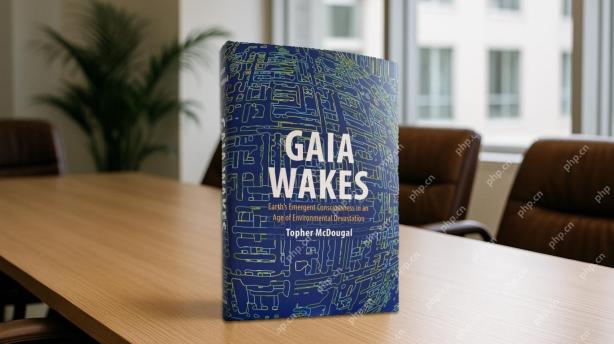
빠른 기술 발전은 미래의 업무에 대한 미래 지향적 인 관점을 필요로합니다. AI가 단순한 생산성 향상을 초월하고 사회적 구조를 형성하기 시작하면 어떻게됩니까? Topher McDougal의 다가오는 책인 Gaia Wakes :
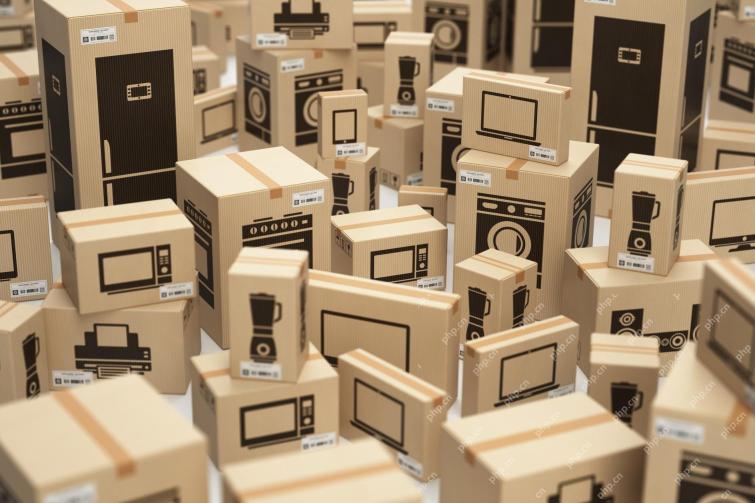
조화 시스템 (HS)과 같은 시스템의 "HS 8471.30"과 같은 복잡한 코드를 포함하는 제품 분류는 국제 무역 및 국내 판매에 중요합니다. 이 코드는 올바른 세금 신청을 보장하여 모든 inv에 영향을 미칩니다
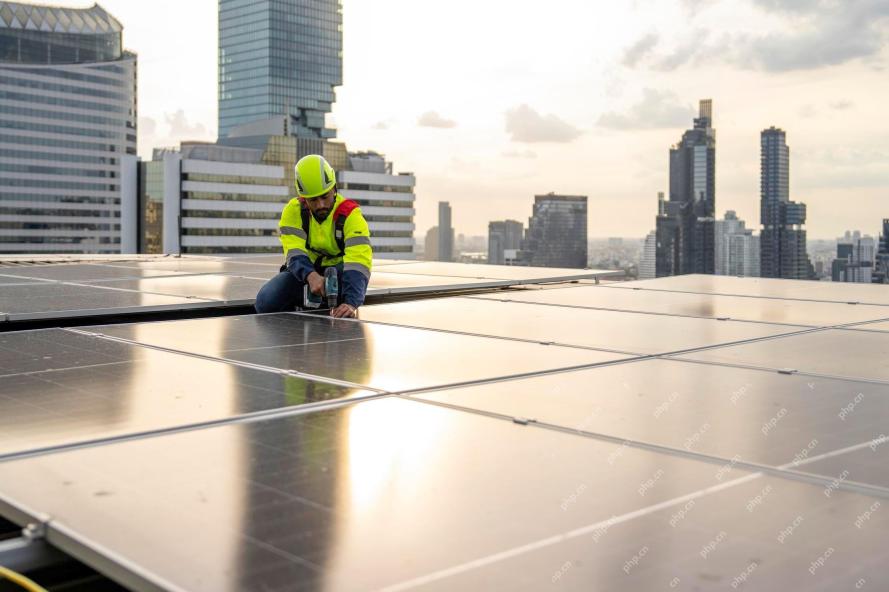
데이터 센터 및 기후 기술 투자의 에너지 소비의 미래 이 기사는 AI가 주도하는 데이터 센터의 에너지 소비 급증과 기후 변화에 미치는 영향을 탐구 하고이 과제를 해결하기 위해 혁신적인 솔루션 및 정책 권장 사항을 분석합니다. 에너지 수요의 과제 : 대규모 및 초대형 스케일 데이터 센터는 수십만 명의 일반 북미 가족의 합과 비슷한 대규모 전력을 소비하며, AI 초반 규모 센터는 이보다 수십 배 더 많은 힘을 소비합니다. 2024 년 첫 8 개월 동안 Microsoft, Meta, Google 및 Amazon은 AI 데이터 센터의 건설 및 운영에 약 1,250 억 달러를 투자했습니다 (JP Morgan, 2024) (표 1). 에너지 수요 증가는 도전이자 기회입니다. 카나리아 미디어에 따르면 다가오는 전기
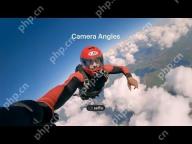
생성 AI는 영화 및 텔레비전 제작을 혁신하고 있습니다. Luma의 Ray 2 모델과 활주로의 Gen-4, Openai의 Sora, Google의 VEO 및 기타 새로운 모델은 전례없는 속도로 생성 된 비디오의 품질을 향상시키고 있습니다. 이 모델은 복잡한 특수 효과와 현실적인 장면을 쉽게 만들 수 있으며 짧은 비디오 클립과 카메라로 인식 된 모션 효과조차도 달성되었습니다. 이러한 도구의 조작과 일관성은 여전히 개선되어야하지만 진행 속도는 놀랍습니다. 생성 비디오는 독립적 인 매체가되고 있습니다. 일부 모델은 애니메이션 제작에 능숙하고 다른 모델은 라이브 액션 이미지에 능숙합니다. Adobe 's Firefly와 Moonvalley's MA가
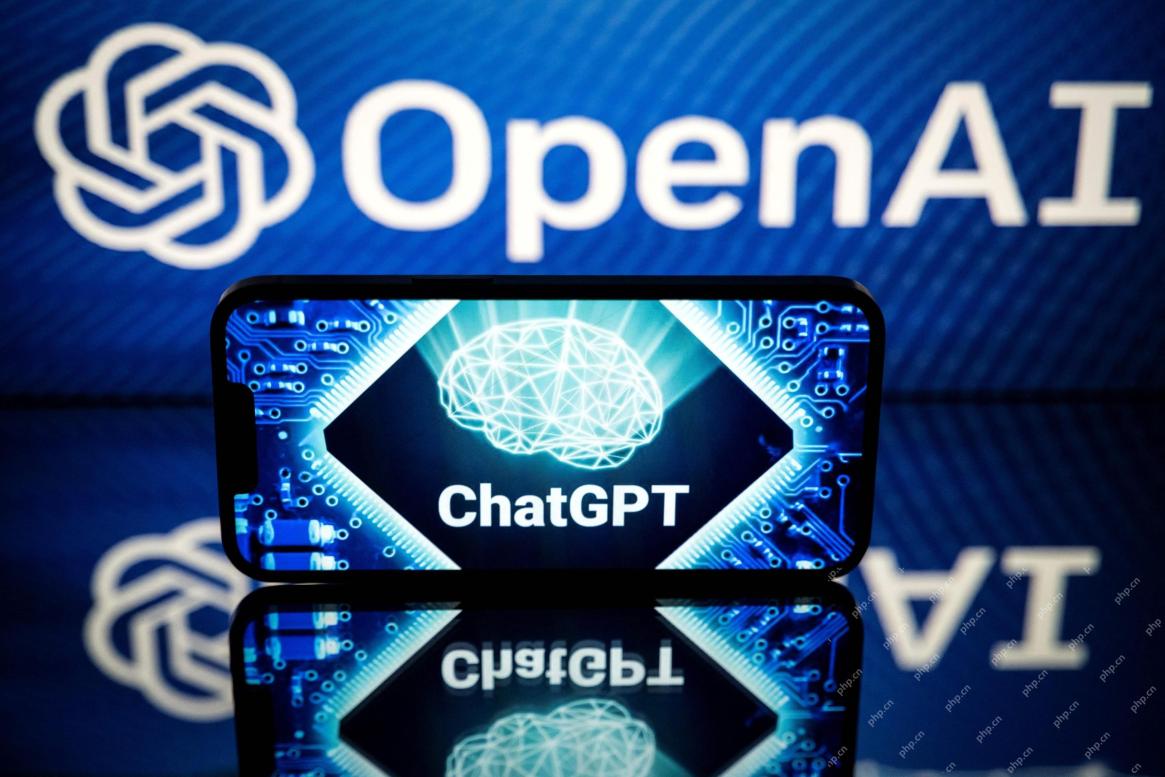
ChatGpt 사용자 경험 감소 : 모델 저하 또는 사용자 기대치입니까? 최근에, 많은 ChatGpt 유료 사용자가 성능 저하에 대해 불평하여 광범위한 관심을 끌었습니다. 사용자는 모델에 대한 느린 반응, 짧은 답변, 도움 부족 및 더 많은 환각을보고했습니다. 일부 사용자는 소셜 미디어에 대한 불만을 표명했으며 Chatgpt가“너무 아첨”이되었으며 중요한 피드백을 제공하기보다는 사용자보기를 확인하는 경향이 있습니다. 이는 사용자 경험에 영향을 줄뿐만 아니라 생산성 감소 및 컴퓨팅 리소스 낭비와 같은 회사 고객에게 실제 손실을 가져옵니다. 성능 저하의 증거 많은 사용자들이 ChatGpt 성능, 특히 GPT-4와 같은 이전 모델 (이번 달 말에 서비스에서 곧 중단 될 예정)에서 상당한 악화를보고했습니다. 이것


핫 AI 도구

Undresser.AI Undress
사실적인 누드 사진을 만들기 위한 AI 기반 앱

AI Clothes Remover
사진에서 옷을 제거하는 온라인 AI 도구입니다.

Undress AI Tool
무료로 이미지를 벗다

Clothoff.io
AI 옷 제거제

Video Face Swap
완전히 무료인 AI 얼굴 교환 도구를 사용하여 모든 비디오의 얼굴을 쉽게 바꾸세요!

인기 기사

뜨거운 도구

스튜디오 13.0.1 보내기
강력한 PHP 통합 개발 환경
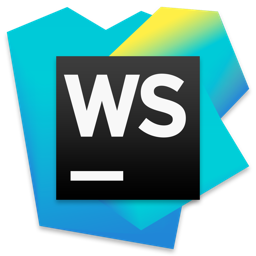
WebStorm Mac 버전
유용한 JavaScript 개발 도구

Eclipse용 SAP NetWeaver 서버 어댑터
Eclipse를 SAP NetWeaver 애플리케이션 서버와 통합합니다.

안전한 시험 브라우저
안전한 시험 브라우저는 온라인 시험을 안전하게 치르기 위한 보안 브라우저 환경입니다. 이 소프트웨어는 모든 컴퓨터를 안전한 워크스테이션으로 바꿔줍니다. 이는 모든 유틸리티에 대한 액세스를 제어하고 학생들이 승인되지 않은 리소스를 사용하는 것을 방지합니다.

mPDF
mPDF는 UTF-8로 인코딩된 HTML에서 PDF 파일을 생성할 수 있는 PHP 라이브러리입니다. 원저자인 Ian Back은 자신의 웹 사이트에서 "즉시" PDF 파일을 출력하고 다양한 언어를 처리하기 위해 mPDF를 작성했습니다. HTML2FPDF와 같은 원본 스크립트보다 유니코드 글꼴을 사용할 때 속도가 느리고 더 큰 파일을 생성하지만 CSS 스타일 등을 지원하고 많은 개선 사항이 있습니다. RTL(아랍어, 히브리어), CJK(중국어, 일본어, 한국어)를 포함한 거의 모든 언어를 지원합니다. 중첩된 블록 수준 요소(예: P, DIV)를 지원합니다.
