이 기사에서는 phidata와 Ollama 로컬 LLM을 사용하여 웹 검색, 재무 분석, 추론 및 검색 증강 생성을 위한 AI 에이전트를 만드는 방법을 살펴보겠습니다. 코드는 llama3.2 모델을 사용합니다. 다른 모델을 사용하려면 사용하려는 모델을 다운로드하고 코드에서 model_id 변수를 바꿔야 합니다.
피다타(Phidata)란 무엇입니까?
에이전트 시스템을 구축, 제공, 모니터링하기 위한 오픈 소스 플랫폼입니다.
https://www.phidata.com/
올라마란 무엇인가요?
Ollama는 로컬 LLM(대형 언어 모델)의 배포 및 사용을 단순화하도록 설계된 플랫폼 및 도구 세트입니다.
https://ollama.ai/
이 글에서는 llama3.2 모델을 사용하겠습니다.
ollama pull llama3.2
UV 란 무엇입니까?
Rust로 작성된 매우 빠른 Python 패키지 및 프로젝트 관리자입니다.
https://github.com/astral-sh/uv
uv를 사용하고 싶지 않다면 uv 대신 pip를 사용해도 됩니다. 그런 다음 uv add 대신 pip install을 사용해야 합니다.
UV 설치 방법
https://docs.astral.sh/uv/getting-started/installation/
프로젝트 폴더 생성
pip를 사용하기로 결정했다면 프로젝트 폴더를 생성해야 합니다.
uv init phidata-ollama
종속성 설치
uv add phidata ollama duckduckgo-search yfinance pypdf lancedb tantivy sqlalchemy
이 글에서는 phidata와 Ollama를 이용해 5개의 AI 에이전트를 만들어 보겠습니다.
참고: 시작하기 전에 ollama Serve를 실행하여 ollama 서버가 실행 중인지 확인하세요.
웹 검색 에이전트 만들기
우리가 만들 첫 번째 에이전트는 DuckDuckGo 검색 엔진을 사용하는 웹 검색 에이전트입니다.
from phi.agent import Agent from phi.model.ollama import Ollama from phi.tools.duckduckgo import DuckDuckGo model_id = "llama3.2" model = Ollama(id=model_id) web_agent = Agent( name="Web Agent", model=model, tools=[DuckDuckGo()], instructions=["Always include sources"], show_tool_calls=True, markdown=True, ) web_agent.print_response("Tell me about OpenAI Sora?", stream=True)
출력:
┏━ Message ━━━━━━━━━━━━━━━━━━━━━━━━━━━━━━━━━━━━━━━━━━━━━━━━━━━━━━━━━━━━━━━┓ ┃ ┃ ┃ Tell me about OpenAI Sora? ┃ ┃ ┃ ┗━━━━━━━━━━━━━━━━━━━━━━━━━━━━━━━━━━━━━━━━━━━━━━━━━━━━━━━━━━━━━━━━━━━━━━━━━┛ ┏━ Response (12.0s) ━━━━━━━━━━━━━━━━━━━━━━━━━━━━━━━━━━━━━━━━━━━━━━━━━━━━━━┓ ┃ ┃ ┃ ┃ ┃ • Running: duckduckgo_news(query=OpenAI Sora) ┃ ┃ ┃ ┃ OpenAI's Sora is a video-generating model that has been trained on ┃ ┃ copyrighted content, which has raised concerns about its legality. ┃ ┃ According to TechCrunch, it appears that OpenAI trained Sora on game ┃ ┃ content, which could be a problem. Additionally, MSN reported that the ┃ ┃ model doesn't feel like the game-changer it was supposed to be. ┃ ┃ ┃ ┃ In other news, Yahoo reported that when asked to generate gymnastics ┃ ┃ videos, Sora produces horrorshow videos with whirling and morphing ┃ ┃ limbs. A lawyer told ExtremeTech that it's "overwhelmingly likely" that ┃ ┃ copyrighted materials are included in Sora's training dataset. ┃ ┃ ┃ ┃ Geeky Gadgets reviewed OpenAI's Sora, stating that while it is included ┃ ┃ in the 0/month Pro Plan, its standalone value for video generation ┃ ┃ is less clear compared to other options. ┃ ┃ ┃ ┗━━━━━━━━━━━━━━━━━━━━━━━━━━━━━━━━━━━━━━━━━━━━━━━━━━━━━━━━━━━━━━━━━━━━━━━━━┛
금융 대리인 만들기
우리가 만들 두 번째 에이전트는 yfinance 도구를 사용할 금융 에이전트입니다.
from phi.agent import Agent from phi.model.ollama import Ollama from phi.tools.yfinance import YFinanceTools model_id = "llama3.2" model = Ollama(id=model_id) finance_agent = Agent( name="Finance Agent", model=model, tools=[YFinanceTools(stock_price=True, analyst_recommendations=True, company_info=True, company_news=True)], instructions=["Use tables to display data"], show_tool_calls=True, markdown=True, ) finance_agent.print_response("Summarize analyst recommendations for NVDA", stream=True)
출력:
┏━ Message ━━━━━━━━━━━━━━━━━━━━━━━━━━━━━━━━━━━━━━━━━━━━━━━━━━━━━━━━━━━━━━━┓ ┃ ┃ ┃ Summarize analyst recommendations for NVDA ┃ ┃ ┃ ┗━━━━━━━━━━━━━━━━━━━━━━━━━━━━━━━━━━━━━━━━━━━━━━━━━━━━━━━━━━━━━━━━━━━━━━━━━┛ ┏━ Response (3.9s) ━━━━━━━━━━━━━━━━━━━━━━━━━━━━━━━━━━━━━━━━━━━━━━━━━━━━━━━┓ ┃ ┃ ┃ ┃ ┃ • Running: get_analyst_recommendations(symbol=NVDA) ┃ ┃ ┃ ┃ Based on the analyst recommendations, here is a summary: ┃ ┃ ┃ ┃ • The overall sentiment is bullish, with 12 strong buy and buy ┃ ┃ recommendations. ┃ ┃ • There are no strong sell or sell recommendations. ┃ ┃ • The average price target for NVDA is around 0-0. ┃ ┃ • Analysts expect NVDA to continue its growth trajectory, driven by ┃ ┃ its strong products and services in the tech industry. ┃ ┃ ┃ ┃ Please note that these recommendations are subject to change and may ┃ ┃ not reflect the current market situation. It's always a good idea to do ┃ ┃ your own research and consult with a financial advisor before making ┃ ┃ any investment decisions. ┃ ┃ ┃ ┗━━━━━━━━━━━━━━━━━━━━━━━━━━━━━━━━━━━━━━━━━━━━━━━━━━━━━━━━━━━━━━━━━━━━━━━━━┛
상담원 팀 만들기
우리가 만들 세 번째 에이전트는 DuckDuckGo 검색 엔진과 YFinance 도구를 사용할 에이전트 팀입니다.
from phi.agent import Agent from phi.model.ollama import Ollama from phi.tools.duckduckgo import DuckDuckGo from phi.tools.yfinance import YFinanceTools web_instructions = 'Always include sources' finance_instructions = 'Use tables to display data' model_id = "llama3.2" model = Ollama(id=model_id) web_agent = Agent( name="Web Agent", role="Search the web for information", model=model, tools=[DuckDuckGo()], instructions=[web_instructions], show_tool_calls=True, markdown=True, ) finance_agent = Agent( name="Finance Agent", role="Get financial data", model=model, tools=[YFinanceTools(stock_price=True, analyst_recommendations=True, company_info=True)], instructions=[finance_instructions], show_tool_calls=True, markdown=True, ) agent_team = Agent( model=model, team=[web_agent, finance_agent], instructions=[web_instructions, finance_instructions], show_tool_calls=True, markdown=True, ) agent_team.print_response("Summarize analyst recommendations and share the latest news for NVDA", stream=True)
추론 에이전트 생성
우리가 만들 네 번째 에이전트는 작업을 사용할 추론 에이전트입니다.
from phi.agent import Agent from phi.model.ollama import Ollama model_id = "llama3.2" model = Ollama(id=model_id) task = ( "Three missionaries and three cannibals want to cross a river." "There is a boat that can carry up to two people, but if the number of cannibals exceeds the number of missionaries, the missionaries will be eaten." ) reasoning_agent = Agent(model=model, reasoning=True, markdown=True, structured_outputs=True) reasoning_agent.print_response(task, stream=True, show_full_reasoning=True)
출력:
┏━ Message ━━━━━━━━━━━━━━━━━━━━━━━━━━━━━━━━━━━━━━━━━━━━━━━━━━━━━━━━━━━━━━━┓ ┃ ┃ ┃ Three missionaries and three cannibals want to cross a river.There is a ┃ ┃ boat that can carry up to two people, but if the number of cannibals ┃ ┃ exceeds the number of missionaries, the missionaries will be eaten. ┃ ┃ ┃ ┗━━━━━━━━━━━━━━━━━━━━━━━━━━━━━━━━━━━━━━━━━━━━━━━━━━━━━━━━━━━━━━━━━━━━━━━━━┛ [Reasoning steps and output as in the original document]
RAG 에이전트 만들기
우리가 만들 다섯 번째 에이전트는 PDF 지식 베이스와 LanceDB 벡터 DB를 사용하는 RAG 에이전트입니다.
from phi.agent import Agent from phi.model.openai import OpenAIChat from phi.embedder.openai import OpenAIEmbedder from phi.embedder.ollama import OllamaEmbedder from phi.model.ollama import Ollama from phi.knowledge.pdf import PDFUrlKnowledgeBase from phi.vectordb.lancedb import LanceDb, SearchType model_id = "llama3.2" model = Ollama(id=model_id) embeddings = OllamaEmbedder().get_embedding("The quick brown fox jumps over the lazy dog.") knowledge_base = PDFUrlKnowledgeBase( urls=["https://phi-public.s3.amazonaws.com/recipes/ThaiRecipes.pdf"], vector_db=LanceDb( table_name="recipes", uri="tmp/lancedb", search_type=SearchType.vector, embedder=OllamaEmbedder(), ), ) knowledge_base.load() agent = Agent( model=model, knowledge=knowledge_base, show_tool_calls=True, markdown=True, ) agent.print_response("Please tell me how to make green curry.", stream=True)
출력:
uv run rag_agent.py WARNING model "openhermes" not found, try pulling it first WARNING model "openhermes" not found, try pulling it first INFO Creating collection INFO Loading knowledge base INFO Reading: https://phi-public.s3.amazonaws.com/recipes/ThaiRecipes.pdf WARNING model "openhermes" not found, try pulling it first WARNING model "openhermes" not found, try pulling it first WARNING model "openhermes" not found, try pulling it first WARNING model "openhermes" not found, try pulling it first WARNING model "openhermes" not found, try pulling it first WARNING model "openhermes" not found, try pulling it first WARNING model "openhermes" not found, try pulling it first WARNING model "openhermes" not found, try pulling it first WARNING model "openhermes" not found, try pulling it first WARNING model "openhermes" not found, try pulling it first WARNING model "openhermes" not found, try pulling it first WARNING model "openhermes" not found, try pulling it first WARNING model "openhermes" not found, try pulling it first WARNING model "openhermes" not found, try pulling it first INFO Added 14 documents to knowledge base WARNING model "openhermes" not found, try pulling it first ERROR Error searching for documents: list index out of range ┏━ Message ━━━━━━━━━━━━━━━━━━━━━━━━━━━━━━━━━━━━━━━━━━━━━━━━━━━━━━━━━━━━━━━┓ ┃ ┃ ┃ Please tell me how to make green curry. ┃ ┃ ┃ ┗━━━━━━━━━━━━━━━━━━━━━━━━━━━━━━━━━━━━━━━━━━━━━━━━━━━━━━━━━━━━━━━━━━━━━━━━━┛ ┏━ Response (5.4s) ━━━━━━━━━━━━━━━━━━━━━━━━━━━━━━━━━━━━━━━━━━━━━━━━━━━━━━━┓ ┃ ┃ ┃ ┃ ┃ • Running: search_knowledge_base(query=green curry recipe) ┃ ┃ ┃ ┃ ┏━━━━━━━━━━━━━━━━━━━━━━━━━━━━━━━━━━━━━━━━━━━━━━━━━━━━━━━━━━━━━━━━━━━━━┓ ┃ ┃ ┃ Green Curry Recipe ┃ ┃ ┃ ┗━━━━━━━━━━━━━━━━━━━━━━━━━━━━━━━━━━━━━━━━━━━━━━━━━━━━━━━━━━━━━━━━━━━━━┛ ┃ ┃ ┃ ┃ ** Servings: 4-6 people** ┃ ┃ ┃ ┃ Ingredients: ┃ ┃ ┃ ┃ • 2 tablespoons vegetable oil ┃ ┃ • 2 cloves garlic, minced ┃ ┃ • 1 tablespoon grated fresh ginger ┃ ┃ • 2 tablespoons Thai red curry paste ┃ ┃ • 2 cups coconut milk ┃ ┃ • 1 cup mixed vegetables (such as bell peppers, bamboo shoots, and ┃ ┃ Thai eggplant) ┃ ┃ • 1 pound boneless, skinless chicken breasts or thighs, cut into ┃ ┃ bite-sized pieces ┃ ┃ • 2 tablespoons fish sauce ┃ ┃ • 1 tablespoon palm sugar ┃ ┃ • 1/4 teaspoon ground white pepper ┃ ┃ • Salt to taste ┃ ┃ • Fresh basil leaves for garnish ┃ ┃ ┃ ┃ Instructions: ┃ ┃ ┃ ┃ 1 Prepare the curry paste: In a blender or food processor, combine the ┃ ┃ curry paste, garlic, ginger, fish sauce, palm sugar, and white ┃ ┃ pepper. Blend until smooth. ┃ ┃ 2 Heat oil in a pan: Heat the oil in a large skillet or Dutch oven ┃ ┃ over medium-high heat. ┃ ┃ 3 Add the curry paste: Pour the blended curry paste into the hot oil ┃ ┃ and stir constantly for 1-2 minutes, until fragrant. ┃ ┃ 4 Add coconut milk: Pour in the coconut milk and bring the mixture to ┃ ┃ a simmer. ┃ ┃ 5 Add vegetables and chicken: Add the mixed vegetables and chicken ┃ ┃ pieces to the pan. Stir gently to combine. ┃ ┃ 6 Reduce heat and cook: Reduce the heat to medium-low and let the ┃ ┃ curry simmer, uncovered, for 20-25 minutes or until the chicken is ┃ ┃ cooked through and the sauce has thickened. ┃ ┃ 7 Season with salt and taste: Season the curry with salt to taste. ┃ ┃ Serve hot garnished with fresh basil leaves. ┃ ┃ ┃ ┃ Tips and Variations: ┃ ┃ ┃ ┃ • Adjust the level of spiciness by using more or less Thai red curry ┃ ┃ paste. ┃ ┃ • Add other protein sources like shrimp, tofu, or tempeh for a ┃ ┃ vegetarian or vegan option. ┃ ┃ • Experiment with different vegetables, such as zucchini or carrots, ┃ ┃ to add variety. ┃ ┃ ┃ ┃ Tools Used: Python ┃ ┃ ┃ ┗━━━━━━━━━━━━━━━━━━━━━━━━━━━━━━━━━━━━━━━━━━━━━━━━━━━━━━━━━━━━━━━━━━━━━━━━━┛
결론
이 기사에서는 phidata와 Ollama 로컬 LLM을 사용하여 웹 검색, 금융 분석, 추론, 검색 증강 생성을 위한 AI 에이전트를 만드는 방법을 살펴보았습니다.
위 내용은 phidata 및 Ollama를 사용하여 I 에이전트 구축의 상세 내용입니다. 자세한 내용은 PHP 중국어 웹사이트의 기타 관련 기사를 참조하세요!
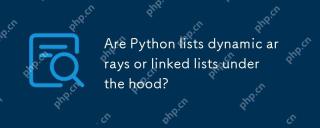
pythonlistsareimplementedesdynamicarrays, notlinkedlists.1) thearestoredIntIguousUousUousUousUousUousUousUousUousUousInSeripendExeDaccess, LeadingSpyTHOCESS, ImpactingEperformance
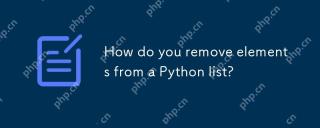
PythonoffersfourmainmethodstoremoveElementsfromalist : 1) 제거 (값) 제거 (값) removesthefirstoccurrencefavalue, 2) pop (index) 제거 elementatAspecifiedIndex, 3) delstatemeveselementsByindexorSlice, 4) RemovesAllestemsfromTheChmetho
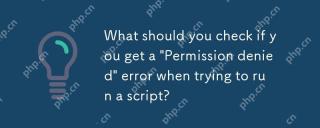
Toresolvea "permissionDenied"오류가 발생할 때 오류가 발생합니다.
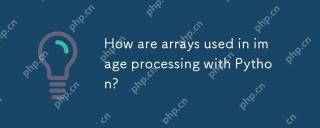
arraysarecrucialinpythonimageProcessingAstheyenableantureficient -manipulationand analysysofimagedata.1) ImagesAreconTortonumpyArrays, withGrayScaleImages2DarraysAndColorImagesS3darrays.2) arraysallowforvectorizedoperations, inablingastAdmentments bri
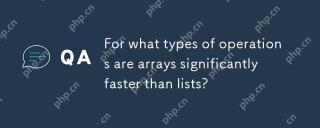
ArraysareSareSareStificerTanlistSforoperationsbenefitingfrom DirectMemoryAccessandfixed-sizestructures.1) AccessingElements : ArraysprovideConstant-timeaccessduetocontiguousUousUousSougues.2) 반복 : ArraysleAgeCachelocalityFasterItertion.3) Mem
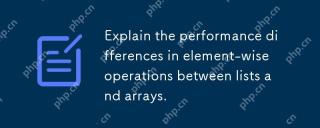
ArraysareBetterForElement-WiseOperationsDuetOfasterAcccessandoptimizedimmentations.1) ArraysHaveCecontIguousMemoryFordirectAccess, 향상
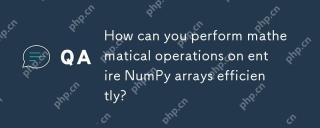
Numpy에서 전체 배열의 수학적 작업은 벡터화 된 작업을 통해 효율적으로 구현 될 수 있습니다. 1) 추가 (ARR 2)와 같은 간단한 연산자를 사용하여 배열에서 작업을 수행하십시오. 2) Numpy는 기본 C 언어 라이브러리를 사용하여 컴퓨팅 속도를 향상시킵니다. 3) 곱셈, 분할 및 지수와 같은 복잡한 작업을 수행 할 수 있습니다. 4) 배열 모양이 호환되도록 방송 작업에주의를 기울이십시오. 5) NP.Sum ()과 같은 Numpy 함수를 사용하면 성능을 크게 향상시킬 수 있습니다.
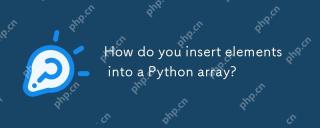
Python에는 요소를 목록에 삽입하는 두 가지 주요 방법이 있습니다. 1) 삽입 (인덱스, 값) 메소드를 사용하여 지정된 인덱스에 요소를 삽입 할 수 있지만 큰 목록의 시작 부분에서 삽입하는 것은 비효율적입니다. 2) Append (value) 메소드를 사용하여 목록 끝에 요소를 추가하여 매우 효율적입니다. 대형 목록의 경우 Append ()를 사용하거나 Deque 또는 Numpy Array를 사용하여 성능을 최적화하는 것이 좋습니다.


핫 AI 도구

Undresser.AI Undress
사실적인 누드 사진을 만들기 위한 AI 기반 앱

AI Clothes Remover
사진에서 옷을 제거하는 온라인 AI 도구입니다.

Undress AI Tool
무료로 이미지를 벗다

Clothoff.io
AI 옷 제거제

Video Face Swap
완전히 무료인 AI 얼굴 교환 도구를 사용하여 모든 비디오의 얼굴을 쉽게 바꾸세요!

인기 기사

뜨거운 도구

PhpStorm 맥 버전
최신(2018.2.1) 전문 PHP 통합 개발 도구

Atom Editor Mac 버전 다운로드
가장 인기 있는 오픈 소스 편집기
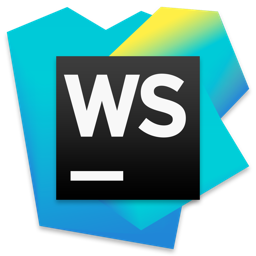
WebStorm Mac 버전
유용한 JavaScript 개발 도구
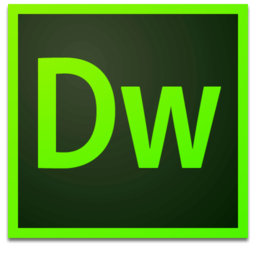
Dreamweaver Mac版
시각적 웹 개발 도구

SublimeText3 Linux 새 버전
SublimeText3 Linux 최신 버전