저자: Shijunjun
1. 소개
최근 Privasea에서 시작한 얼굴 NFT 캐스팅 프로젝트가 큰 인기를 끌고 있습니다! 얼핏 보면 매우 간단해 보이지만, 사용자는 IMHUMAN(나는 인간입니다) 모바일 애플리케이션에 자신의 얼굴을 입력하고 NFT에 얼굴 데이터를 전송할 수 있습니다. 온체인 얼굴 데이터 + NFT를 통해 프로젝트는 4월 말 출시 이후 20W+ NFT 채굴량 이상의 NFT 채굴량을 확보할 수 있게 되었습니다. 인기는 분명합니다. 저도 많이 헷갈려요, 왜죠? 얼굴 데이터의 크기에 관계없이 블록체인에 업로드할 수 있나요? 내 얼굴 정보가 도난당하나요? 프리바시는 무엇을 하나요? 잠깐만요, 프로젝트 자체와 프로젝트 파티 Privasea를 계속 연구하여 알아 보겠습니다. 키워드: NFT, AI, FHE(Fully Homomorphic Encryption), DePIN2. Web2에서 Web3까지-인간-기계 대결은 멈추지 않습니다
먼저 NFT 캐스팅의 목적을 설명하겠습니다. 프로젝트 자체를, 이 프로젝트가 단순히 얼굴 데이터를 NFT로 변환하는 것이라고 생각했다면, 완전히 틀린 생각입니다. 위에서 언급한 프로젝트의 앱 이름 IMHUMAN(나는 인간입니다) 이 이 문제를 잘 설명했습니다. 사실 이 프로젝트의 목표는 얼굴 인식을 통해 화면 앞에 있는지 확인하는 것입니다. 실제 사람입니다. . Akamai에서 제공한 2024Q1보고서(부록 참조)에 따르면 Bot(사람이 보내는 HTTP 요청 및 기타 작업을 시뮬레이션할 수 있는 자동화된 프로그램)가 인터넷 트래픽의 무려 42.1%를 차지하는 것으로 나타났습니다. 그 중 악성 트래픽이 전체 인터넷 트래픽의 27.5%를 차지합니다. 악성 봇은 중앙 집중식 서비스 제공업체에 응답 지연이나 다운타임과 같은 치명적인 결과를 초래하여 실제 사용자의 경험에 영향을 미칠 수 있습니다. 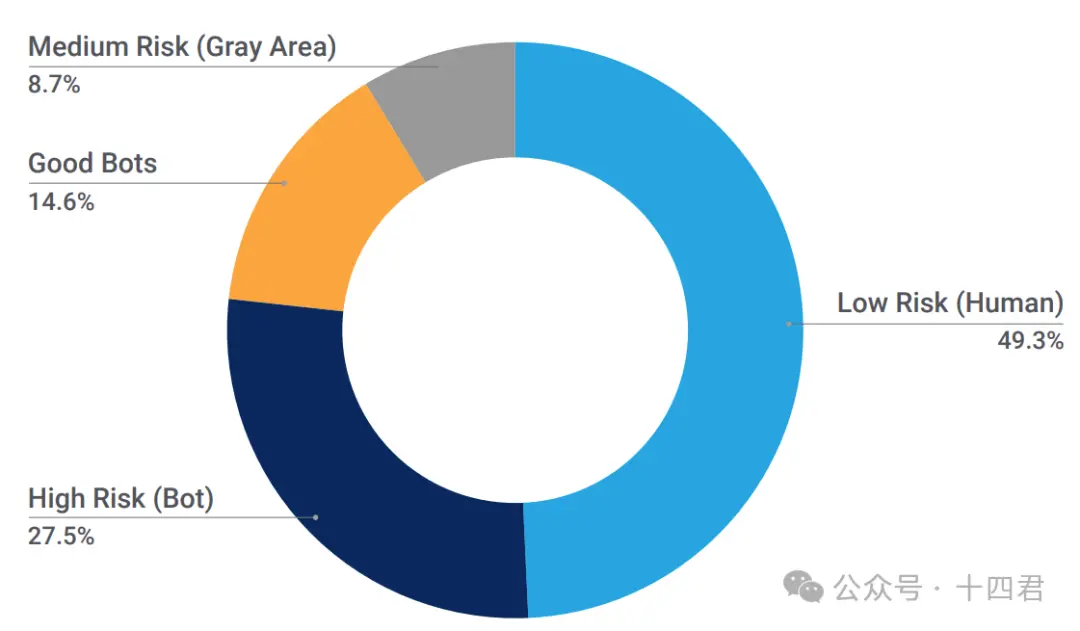
티켓 잡기 시나리오를 예로 들어 보겠습니다. 티켓을 잡기 위해 여러 가상 계정을 생성하면 사기꾼이 티켓 잡기 성공 확률을 크게 높일 수 있으며 일부는 Next to the에 자동화된 프로그램을 직접 배포하기도 합니다. 서비스 제공업체의 전산실에서 티켓 구매가 거의 지연 없이 이루어집니다.
일반 유저들은 이런 하이테크 유저들을 상대로 승리할 확률이 거의 없습니다.
서비스 제공자도 이에 대해 약간의 노력을 기울였습니다. 클라이언트 측에서는 Web2 시나리오에서 인간과 기계를 구별하기 위해 실명 인증, 행동 확인 코드 및 기타 방법이 도입되었습니다. 차단은 WAF 정책 및 기타 수단을 통해 수행됩니다.
이 문제가 해결될 수 있나요?
분명히 그렇지 않습니다. 부정 행위로 인한 이점이 엄청나기 때문입니다.
동시에 인간과 기계의 대결은 계속되고 있으며 치터와 테스터 모두 끊임없이 무기고를 업그레이드하고 있습니다.
최근 AI의 급속한 발전을 활용하여 클라이언트의 행동 검증 코드는 다양한 시각적 모델에 의해 거의 차원적으로 축소되었으며 심지어 인간보다 더 빠르고 정확한 인식 기능을 갖추고 있습니다. 이로 인해 검증자는 수동적으로 업그레이드하여 초기 사용자 행동 특징 감지(이미지 확인 코드)에서 생체 인식 기능 감지(지각적 확인: 클라이언트 환경 모니터링, 장치 지문 등)로 점진적으로 전환합니다. 일부 고위험 작업에는 업그레이드가 필요할 수 있습니다. 생물학적 특징 감지(지문, 얼굴 인식)까지.
Web3의 경우 인간-기계 감지도 강력한 요구 사항입니다.
일부 프로젝트 에어드랍의 경우 사기꾼이 여러 개의 가짜 계정을 만들어 마녀 공격을 시작할 수 있습니다. 이때 실제 인물을 식별해야 합니다.
Web3의 재정적 특성으로 인해 계정 로그인, 화폐 인출, 거래, 이체 등 일부 고위험 작업의 경우 실제 사람만이 사용자인지 확인해야 할 뿐만 아니라 계정 소유자이므로 얼굴 인식이 필수가 됩니다.
수요는 확실하지만, 이를 어떻게 구현하느냐가 문제입니다. 우리 모두 알고 있듯이, 분산화는 Web3의 원래 의도입니다. Web3에서 얼굴 인식을 구현하는 방법을 논의할 때 실제로 더 깊은 질문은 Web3가 AI 시나리오에 어떻게 적응해야 합니까?
- 어떻게 해야 합니까? 분산형 기계 학습 컴퓨팅 네트워크를 구축하시겠습니까?
- 사용자 데이터의 개인 정보가 유출되지 않도록 하는 방법은 무엇입니까?
- 네트워크 운영 등을 유지하는 방법은 무엇입니까?
3. Privasea AI NetWork - 프라이빗 컴퓨팅 + AI 탐색
이전 장의 끝 부분에서 언급한 문제에 대해 Privasea는 획기적인 솔루션을 제공했습니다. Privasea는 FHE(Fully Homomorphic Encryption)를 기반으로 합니다. )는 Web3에서 AI 시나리오의 개인 정보 보호 컴퓨팅 문제를 해결하기 위해 Privasea AI NetWork를 구축했습니다. FHE는 일반인의 관점에서 일반 텍스트와 암호문에 동일한 작업을 수행한 후 동일한 결과를 보장하는 암호화 기술입니다. Privasea는 애플리케이션 계층, 최적화 계층, 산술 계층 및 원본 계층으로 구분된 기존 THE를 최적화하고 캡슐화하여 HESea 라이브러리를 구성하여 이를 기계 학습 시나리오에 적용합니다. 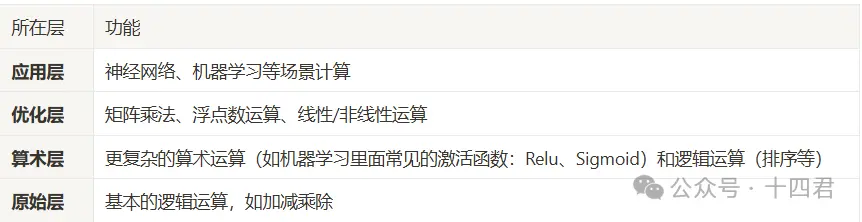
Privasea는 계층화된 구조를 통해 각 사용자의 고유한 요구 사항을 충족하기 위해 보다 구체적이고 맞춤화된 솔루션을 제공합니다. Privasea의 최적화된 패키징은 주로 애플리케이션 계층과 최적화 계층에 중점을 두고 있습니다. 다른 동형 라이브러리의 기본 솔루션과 비교할 때 이러한 맞춤형 계산은 1000배 이상의 가속화를 제공할 수 있습니다. 3.1 Privasea AI NetWork의 네트워크 아키텍처
Privasea AI NetWork의 아키텍처를 살펴보면: 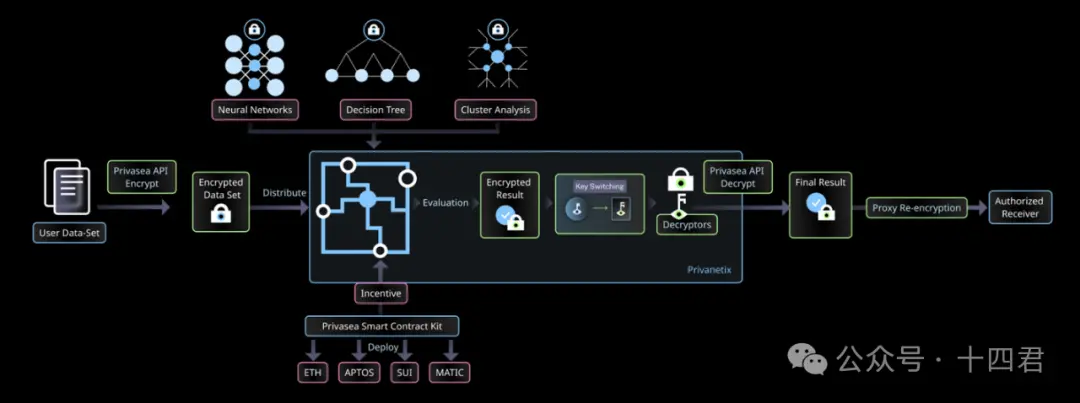
네트워크에는 총 4가지 역할이 있습니다. 데이터 소유자, Privanetix 노드, 암호 해독기, 결과 수신기 .
Data Owner: Privasea API를 통해 작업과 데이터를 안전하게 제출하는 데 사용됩니다.
Privanetix Node: 고급 HESea 라이브러리를 갖추고 블록체인 기반 인센티브 메커니즘과 통합된 전체 네트워크의 핵심입니다. 기본 데이터의 개인 정보를 보호하면서 안전하고 효율적인 계산을 수행할 수 있습니다. 계산 무결성과 기밀성을 보장합니다.
Decryptor: Privasea API를 통해 복호화된 결과를 얻고 결과를 확인합니다.
Result Receiver: 작업 결과는 데이터 소유자 및 작업 발급자가 지정한 사람에게 반환됩니다.
3.2 Privasea AI NetWork의 핵심 워크플로
다음은 Privasea AI NetWork의 일반적인 워크플로 다이어그램입니다.
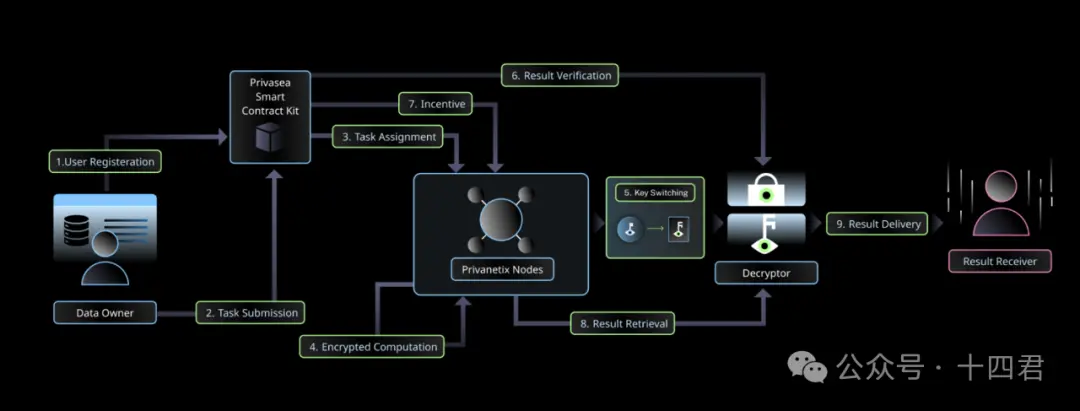
1단계: 사용자 등록: 데이터 소유자 합격 Privacy AI Network에서 등록 프로세스를 시작하는 데 필요한 인증 및 권한 부여 자격 증명을 제공하십시오. 이 단계에서는 승인된 사용자만 시스템에 액세스하고 네트워크 활동에 참여할 수 있도록 합니다.
2단계: 작업 제출: 계산 작업 및 입력 데이터를 제출합니다. 동시에 데이터 소유자는 승인된 암호 해독기와 결과 수신자를 지정합니다. 최종 결과에 액세스할 수 있습니다.
3단계: 작업 할당 : 네트워크에 배포된 블록체인 기반 스마트 계약은 가용성과 기능을 기반으로 컴퓨팅 작업을 적절한 Privanetix 노드에 할당합니다. 이러한 동적 할당 프로세스는 컴퓨팅 작업의 효율적인 리소스 할당 및 배포를 보장합니다.
4단계: 암호화된 계산: 지정된 Privanetix 노드는 암호화된 데이터를 수신하고 HESea 라이브러리를 사용하여 계산을 수행합니다. 이러한 계산은 민감한 데이터를 해독하지 않고도 수행할 수 있으므로 기밀성이 유지됩니다. 계산의 무결성을 추가로 확인하기 위해 Privanetix 노드는 이러한 단계에 대한 영지식 증명을 생성합니다.
STEP 5: Key Switching : After completing the calculation, the designated Privanetix node employs key switching technology to ensure that the final result is authorized and only accessible to the designated decryptor.
STEP 6: Result Verification: After completing the calculation, the Privanetix node transmits the encrypted result and the corresponding zero-knowledge proof back to the blockchain-based smart contract for future verification.励Step 7: Incentive mechanism
: Track the contribution of the PrivaNetix node, and allocate rewards Step 8: Result retrieval: The decryrator uses the Privasea API to access the encryption results. Their first priority is to verify the integrity of the computation, ensuring that the Privanetix node performed the computation as intended by the data owner.
STEP 9: Result Delivery : Share the decrypted results with designated result recipients pre-determined by the data owner.
In the core workflow of Privasea AI NetWork, an open API is exposed to users, which allows users to only pay attention to the input parameters and corresponding results without having to understand the complex operations within the network itself. There would be too much mental load. At the same time, end-to-end encryption prevents the data itself from being leaked without affecting data processing. PoW && PoS dual mechanism superpositionPrivasea recently launched WorkHeart NFT and StarFuel NFT through the dual mechanism of PoW and PoS to conduct network nodes Management and rewards Hand out . Purchase WorkHeart NFT and you will be qualified to become a Privanetix node to participate in network computing and obtain token income based on the PoW mechanism. StarFuel NFT is a node gainer (limited to 5000), which can be combined with WorkHeart, similar to PoS. The more tokens pledged to it, the greater the profit multiplier of the WorkHeartnode. In fact, this question is easier to answer. The essence of PoW is to reduce the node evil rate and maintain the stability of the network through the time cost of operation. Different from the large number of invalid calculations in BTC's random number verification, the actual work output (operation) of this privacy computing network node can be directly linked to the workload mechanism, which is naturally suitable for PoW. And PoS makes it easier to balance economic resources. In this way, WorkHeart NFT obtains income through the PoW mechanism, while StarFuel NFT increases the income multiple through the PoS mechanism, forming a multi-level and diversified incentive mechanism, allowing users to choose appropriate participation methods based on their own resources and strategies. The combination of the two mechanisms can optimize the revenue distribution structure and balance the importance of computing resources and economic resources in the network. 3.3 SummaryIt can be seen that Privatosea AI NetWork has built an
encrypted version of the machine learning system based on FHE. Thanks to the characteristics of FHE privacy computing, the computing tasks are subcontracted to various computing nodes (Privanetix) in a distributed environment, the validity of the results is verified through ZKP, and the dual mechanisms of PoW and PoS are used to provide computing results. Nodes reward or punish to maintain the operation of the network. It can be said that the design of Privasea AI NetWork is paving the way for privacy-preserving AI applications in various fields.4. FHE Homomorphic Encryption - the new holy grail of cryptography?
We can see in the last chapter that the security of Privatosea AI NetWork relies on its underlying FHE. With the continuous technological breakthroughs of ZAMA, the leader of the FHE track, FHE has even been given a new password by investors. Taking the title of Holy Grail, let’s compare it to ZKP and related solutions. 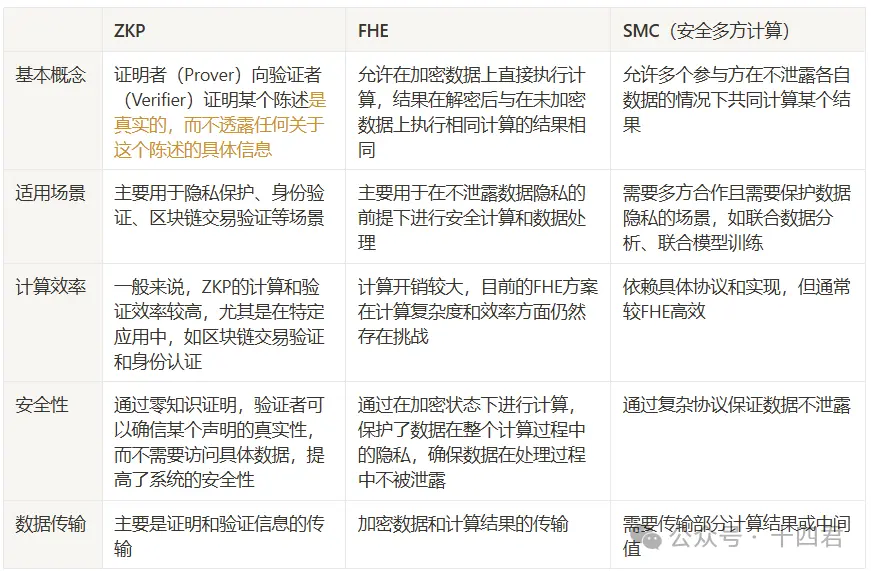
By comparison, you can see that the applicable scenarios of ZKP and FHE are quite different. FHE focuses on privacy calculation, while ZKP focuses on privacy verification. And SMC seems to have a greater overlap with FHE. The concept of SMC is secure joint computing, which solves the data privacy problem of individual computers that work together. 5. Limitations of FHE
FHE realizes the separation of data processing rights and data ownership, thereby preventing data leakage without affecting calculations. But at the same time, the sacrifice is computing speed. Encryption is like a double-edged sword. While it improves security, it also greatly reduces the computing speed. In recent years, various types of FHE performance improvement solutions have been proposed, some based on algorithm optimization and some relying on hardware acceleration.
- In terms of algorithm optimization, new FHE solutions such as CKKS and optimized bootstrap methods significantly reduce noise growth and computational overhead;
- In terms of hardware acceleration, customized GPU, FPGA and other hardware have significantly improved Performance of polynomial operations.
In addition, the application of hybrid encryption schemes is also being explored. By combining partially homomorphic encryption (PHE) and search encryption (SE), efficiency can be improved in specific scenarios. Despite this, FHE still has a large gap in performance from plaintext calculations. 6. Summary
Privasea not only provides users with a highly secure data processing environment through its unique architecture and relatively efficient privacy computing technology, but also opens a new chapter in the deep integration of Web3 and AI. Although the FHE it relies on at the bottom has a natural computing speed disadvantage, Privasea has recently reached a cooperation with ZAMA to jointly solve the problem of privacy computing. In the future, with continuous technological breakthroughs, Privasea is expected to unleash its potential in more fields and become an explorer of privacy computing and AI applications.
위 내용은 Privasea 심층 해석, NFT로 전송된 얼굴 데이터를 아직도 이런 방식으로 재생할 수 있나요?의 상세 내용입니다. 자세한 내용은 PHP 중국어 웹사이트의 기타 관련 기사를 참조하세요!