T 分布確率的近傍埋め込み (t-SNE) は、視覚化のための教師なし機械学習アルゴリズムであり、非線形次元削減テクノロジを使用し、データ ポイントと特徴間の関係に基づいています。は、高次元空間と低次元空間におけるこれらの条件付き確率 (または類似性) の差を最小限に抑えて、低次元空間のデータ ポイントを完全に表現しようとします。
したがって、t-SNE は、2 次元または 3 次元の低次元空間に高次元データを埋め込んで可視化することが得意です。 t-SNE は、ガウス分布の代わりにヘビーテール分布を使用して低次元空間内の 2 点間の類似性を計算することに注意してください。これは、混雑と最適化の問題の解決に役立ちます。また、外れ値は t-SNE には影響しません。
t-SNE アルゴリズムのステップ
1. 高次元空間内の隣接する点間のペアごとの類似性を見つけます。
2. 高次元空間内の点のペアごとの類似性に基づいて、高次元空間内の各点を低次元マップにマッピングします。
3. カルバック・ライブラー発散 (KL 発散) に基づく勾配降下法を使用して、条件付き確率分布間の不一致を最小限に抑える低次元のデータ表現を見つけます。
4. Student-t 分布を使用して、低次元空間内の 2 点間の類似性を計算します。
MNIST データ セットに t-SNE を実装するための Python コード
インポート モジュール
# Importing Necessary Modules. import numpy as np import pandas as pd import matplotlib.pyplot as plt from sklearn.manifold import TSNE from sklearn.preprocessing import StandardScaler
データの読み取り
# Reading the data using pandas df = pd.read_csv('mnist_train.csv') # print first five rows of df print(df.head(4)) # save the labels into a variable l. l = df['label'] # Drop the label feature and store the pixel data in d. d = df.drop("label", axis = 1)
データ プレ- 処理
# Data-preprocessing: Standardizing the data from sklearn.preprocessing import StandardScaler standardized_data = StandardScaler().fit_transform(data) print(standardized_data.shape)
出力
# TSNE # Picking the top 1000 points as TSNE # takes a lot of time for 15K points data_1000 = standardized_data[0:1000, :] labels_1000 = labels[0:1000] model = TSNE(n_components = 2, random_state = 0) # configuring the parameters # the number of components = 2 # default perplexity = 30 # default learning rate = 200 # default Maximum number of iterations # for the optimization = 1000 tsne_data = model.fit_transform(data_1000) # creating a new data frame which # help us in plotting the result data tsne_data = np.vstack((tsne_data.T, labels_1000)).T tsne_df = pd.DataFrame(data = tsne_data, columns =("Dim_1", "Dim_2", "label")) # Plotting the result of tsne sn.FacetGrid(tsne_df, hue ="label", size = 6).map( plt.scatter, 'Dim_1', 'Dim_2').add_legend() plt.show()
以上がt-SNEアルゴリズムの原理とPythonコード実装の詳細な説明の詳細内容です。詳細については、PHP 中国語 Web サイトの他の関連記事を参照してください。
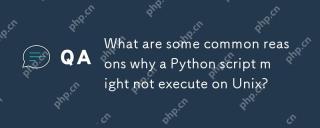
PythonスクリプトがUNIXシステムで実行できない理由には、次のものが含まれます。1)CHMOD XYOUR_SCRIPT.PYを使用して実行権限を付与する不十分な権限。 2)shebangラインが正しくないか欠落している場合、#!/usr/bin/envpythonを使用する必要があります。 3)環境可変設定が誤っていない場合、OS.Environデバッグを印刷できます。 4)間違ったPythonバージョンを使用して、Shebangラインまたはコマンドラインでバージョンを指定できます。 5)仮想環境を使用して依存関係を分離する依存関係の問題。 6)構文エラー、python-mpy_compileyour_script.pyを使用して検出します。
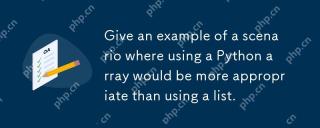
Pythonアレイの使用は、リストよりも大量の数値データの処理に適しています。 1)配列を保存するメモリを保存します。2)アレイは数値的な値で動作するのが高速です。3)アレイフォースタイプの一貫性、4)アレイはCアレイと互換性がありますが、リストほど柔軟で便利ではありません。
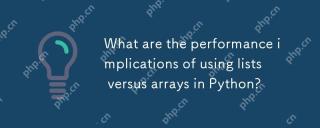
listSareのより良い前提条件とmixdatatypes、whilearraysares優れたスナリカル計算砂の砂を大きくしたデータセット。
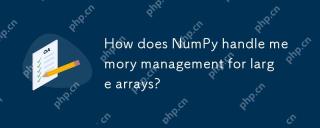
numpymanagesmemoryforlargearrayseffictificleusing biews、copies、andmemory-mappedfiles.1)rewsinging withotingcopying、directmodifying theoriginalArray.2)copiescanbecreatedwithcopy()methodforpreservingdata.3)Memory-MapplehandLemassiutasedatasetasedatasetasetasetasetasetasedas
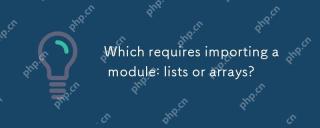
listsinpythondonotrequireimportingamodule、whilearrays fromthearraymoduledoneedanimport.1)listsarebuiltin、versatile、andcanholdmixeddatypes.2)araysaremoremory-efficient-fornumerumerumerumerumerumerdatabutでき、対象となるンドベフェフサメタイプ。
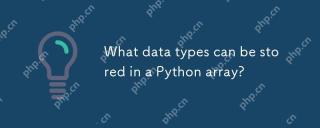
Pythonlistscanstoreanydatatype,arraymodulearraysstoreonetype,andNumPyarraysarefornumericalcomputations.1)Listsareversatilebutlessmemory-efficient.2)Arraymodulearraysarememory-efficientforhomogeneousdata.3)NumPyarraysareoptimizedforperformanceinscient
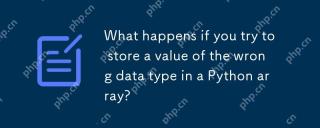
heouttemptemptostoreavure ofthewrongdatatypeinapythonarray、yure counteractypeerror.thisduetothearraymodule'sstricttypeeencultionyを使用します
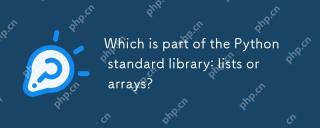
PythonListSarePartOfThestAndardarenot.liestareBuilting-in、versatile、forStoringCollectionsのpythonlistarepart。


ホットAIツール

Undresser.AI Undress
リアルなヌード写真を作成する AI 搭載アプリ

AI Clothes Remover
写真から衣服を削除するオンライン AI ツール。

Undress AI Tool
脱衣画像を無料で

Clothoff.io
AI衣類リムーバー

Video Face Swap
完全無料の AI 顔交換ツールを使用して、あらゆるビデオの顔を簡単に交換できます。

人気の記事

ホットツール

SublimeText3 中国語版
中国語版、とても使いやすい

Safe Exam Browser
Safe Exam Browser は、オンライン試験を安全に受験するための安全なブラウザ環境です。このソフトウェアは、あらゆるコンピュータを安全なワークステーションに変えます。あらゆるユーティリティへのアクセスを制御し、学生が無許可のリソースを使用するのを防ぎます。
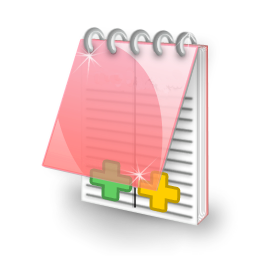
EditPlus 中国語クラック版
サイズが小さく、構文の強調表示、コード プロンプト機能はサポートされていません

SublimeText3 Linux 新バージョン
SublimeText3 Linux 最新バージョン

SecLists
SecLists は、セキュリティ テスターの究極の相棒です。これは、セキュリティ評価中に頻繁に使用されるさまざまな種類のリストを 1 か所にまとめたものです。 SecLists は、セキュリティ テスターが必要とする可能性のあるすべてのリストを便利に提供することで、セキュリティ テストをより効率的かつ生産的にするのに役立ちます。リストの種類には、ユーザー名、パスワード、URL、ファジング ペイロード、機密データ パターン、Web シェルなどが含まれます。テスターはこのリポジトリを新しいテスト マシンにプルするだけで、必要なあらゆる種類のリストにアクセスできるようになります。

ホットトピック









