1. Python キャッシュ
① キャッシュ機能
キャッシュは、最近使用したデータや頻繁に使用したデータをメモリに保存するためにアプリケーションで使用できる最適化テクノロジです。この方法でデータにアクセスする速度は、ディスク ファイルを直接読み取るよりもはるかに高速です。
Feedly に似た、さまざまなソースからニュースを取得し、それを集約して表示するニュース集約 Web サイトを構築するとします。ユーザーがニュースを閲覧すると、バックグラウンド プログラムが記事をダウンロードし、ユーザーの画面に表示します。キャッシュ技術が使用されていない場合、ユーザーが同じ記事を複数回閲覧するために切り替えた場合、その記事を複数回ダウンロードする必要があり、非効率的で不親切です。
より良いアプローチは、各記事を取得した後にコンテンツをデータベースなどにローカルに保存することです。その後、ユーザーが次回同じ記事を開いたときに、バックグラウンド プログラムがコンテンツを開くことができます。ソース ファイルを再度ダウンロードする代わりに、ローカル ストレージに保存します。これは、キャッシュと呼ばれる手法です。
② Python 辞書を使用してキャッシュを実装する
ニュース集約 Web サイトを例に挙げると、記事コンテンツを毎回ダウンロードする必要はありませんが、最初に記事コンテンツが存在するかどうかを確認してください。キャッシュ データ コンテンツに対応するメッセージがあり、コンテンツがない場合にのみ、サーバーは記事をダウンロードします。
次のサンプル プログラムでは、Python 辞書を使用して、記事の URL をキーとして、そのコンテンツを値として使用してキャッシュを実装しています。実行後、get_article 関数が 2 回目に実行されると、それが確認できます。サーバーにダウンロードさせずに結果を返すだけです:
import requests cache = dict() def get_article_from_server(url): print("Fetching article from server...") response = requests.get(url) return response.text def get_article(url): print("Getting article...") if url not in cache: cache[url] = get_article_from_server(url) return cache[url] get_article("https://www.escapelife.site/love-python.html") get_article("https://www.escapelife.site/love-python.html")
このコードをcache.py ファイルに保存し、リクエスト ライブラリをインストールして、スクリプトを実行します:
# 安装依赖 $ pip install requests # 执行脚本 $ python python_caching.py Getting article... Fetching article from server... Getting article...
get_article() を 2 回呼び出しています (17 行目と 18 行目) 「サーバーから記事を取得しています…」という文字列ですが、それでも 1 回しか出力されません。これは、記事に初めてアクセスした後、その URL とコンテンツがキャッシュ ディクショナリに入れられ、2 回目からはコードがサーバーからアイテムを再度取得する必要がないために発生します。
③ キャッシュに辞書を使用するデメリット
上記のキャッシュ実装には非常に大きな問題があります。つまり、辞書の内容が無限に増大するということです。のユーザーが継続的に記事を閲覧すると、バックグラウンド プログラムが辞書に保存する必要のあるコンテンツを詰め込み続け、サーバーのメモリが混雑し、最終的にはアプリケーションがクラッシュします。
上記のキャッシュ実装には非常に大きな問題があります。つまり、辞書の内容が無限に増大することになります。つまり、多数のユーザーが継続的に記事を閲覧すると、バックグラウンド プログラムは引き続き辞書にデータを追加し、保存する必要のあるコンテンツを詰め込むとサーバーのメモリがいっぱいになり、最終的にはアプリケーションがクラッシュします。
上記の問題を解決するには、どの記事をメモリに残し、どの記事を削除するかを決定する戦略が必要です。これらのキャッシュ戦略は、実際には、キャッシュされた情報の管理に使用されるアルゴリズムです。新しいアイテムを入れるスペースを確保するためにどのアイテムを破棄するかを選択します。
もちろん、キャッシュを管理するためのアルゴリズムを実装する必要はありません。必要なのは、さまざまな戦略を使用してキャッシュから項目を削除し、キャッシュが最大サイズを超えて大きくならないようにすることだけです。 。一般的な 5 つのキャッシュ アルゴリズムは次のとおりです。
キャッシュ戦略 | 英語名 | 消去条件 | これが最も役立つ場合 |
---|---|---|---|
先入れ先出しアルゴリズム (FIFO) | 先入れ先出しアルゴリズム | 最も古いエントリを削除する | 新しいエントリが再利用される可能性が最も高くなります |
後入れ先出しアルゴリズム (LIFO) | 後入れ先出し | 最新のエントリを削除する | 古いエントリは再利用される可能性が最も高い |
最近使用されていないアルゴリズム(LRU) | 最も最近使用されていないエントリ | 最も最近使用されていないエントリを削除します | 最も最近使用されたエントリは再利用される可能性が最も高くなります |
最近使用された使用法アルゴリズム (MRU) | 最近使用されたエントリ | 最近使用されたエントリを削除します | 最近使用されなかったエントリは再利用される可能性が最も高くなります |
最も頻繁に使用されないアルゴリズム (LFU) | 最も頻繁に使用されない | 最も頻繁にアクセスされないエントリを削除します | ヒット率の高いアイテム再利用される可能性が高くなります |
上記の 5 つのキャッシュ アルゴリズムを読んだ後、LRU と LFU を見て少し混乱しませんか? その主な理由は、対応する中国語の説明ではその本当の意味を理解するのが難しいからです。英語で。 LRU アルゴリズムと LFU アルゴリズムの違いは次のとおりです:
LRU にはアクセス時間に基づく削除ルールがあり、データの履歴アクセス記録に基づいてデータを削除します。最近、将来アクセスされる確率も高くなります;
アクセス数に基づく LFU 除外ルール、データの過去のアクセス頻度に基づいてデータを除外します。過去に何度もアクセスされたデータであれば、今後もアクセスされるでしょうし、頻度も高くなります;
たとえば、ノードとページとして 10 分が使用された場合必要なページ方向が 2 1 2 4 2 3 4 の場合、スケジューリングは 1 分ごとに実行され、ページ 4 を調整するとページ フォールト割り込みが発生します。LRU アルゴリズムが使用されている場合は、ページ 1 を変更する必要があります (ページ 1 は変更されていません)。ただし、LFU アルゴリズムが使用されている場合は、ページ 3 を変更する必要があります (ページ 3 は 10 分以内にのみ使用されます)。使用は 1 回です)。
2. LRU アルゴリズムを深く理解する
① LRU キャッシュの特性を確認する
LRU 戦略を使用して実装されたキャッシュは、次の順序に従ってソートされます。エントリがアクセスされるたびに、LRU アルゴリズムによってエントリがキャッシュの先頭に移動されます。このように、アルゴリズムはリストの一番下を見て、長期間使用されていないエントリを迅速に特定できます。
以下に示すように、ユーザーはネットワークから最初の記事の LRU ポリシー ストレージ レコードを要求します。
記事が配信される前にキャッシュされる方法ユーザーへ 最寄りのスロットに保管しますか?以下に示すように、ユーザーが 2 番目の記事をリクエストすると、2 番目の記事が最上位に保存されます。つまり、2 番目の記事が最新の位置を占め、最初の記事がリストの下に押し出されます。
LRU ポリシーは、使用されているオブジェクトが新しいほど、将来も使用される可能性が高いと想定しているため、オブジェクトをキャッシュ内に最長期間保持しようとします。エントリが削除される場合、最初のドキュメントのキャッシュ保存レコードが最初に削除されます。
② LRU キャッシュの構造を表示する
Python で LRU キャッシュを実装する 1 つの方法は、二重リンク リスト (二重リンク リスト) とハッシュ マップ (ハッシュ マップ) を使用することです。二重リンクリストの先頭 この要素は最も最近使用されたエントリを指し、その末尾は最も最近使用されたエントリを指します。 LRU キャッシュ実装の論理構造は次のとおりです。
ハッシュ マップを使用すると、各エントリを二重リンク リスト内の特定の場所にマッピングできるため、キャッシュ内の各エントリが項目アクセスであること。この戦略は非常に高速であり、最も最近使用されていないアイテムへのアクセスとキャッシュの更新は両方とも O(1) 操作です。
Python 3.2 以降、Python には LRU 戦略を実装する @lru_cache デコレーターが追加されました。今後は、このデコレーターを使用して関数を装飾し、その計算結果をキャッシュできるようになります。
3. lru_cache デコレータを使用する
① @lru_cache デコレータの実装原理
アプリケーションの高速応答を実現する方法は数多くありますが、キャッシュを使用するのは非常に一般的な方法です。 。キャッシュを正しく使用すると、応答が速くなり、コンピューティング リソースへの余分な負荷が軽減されます。
Python の functools モジュールには、キャッシュ用の @lru_cache デコレータが付属しており、最も最近使用されていない (LRU) 戦略を使用して関数の計算結果をキャッシュできます。これはシンプルですが強力なテクノロジです:
@lru_cache デコレータを実装する;
LRU 戦略がどのように機能するかを理解する;
@ lru_cache デコレータを使用してパフォーマンスを向上させる;
@lru_cache デコレータの機能を拡張し、特定の時間が経過すると有効期限が切れるようにします。
以前に実装されたキャッシュ スキームと同様に、Python の @lru_cache デコレータ ストレージもストレージ オブジェクトとして辞書を使用します。関数の内容は、関数の呼び出し (関数のパラメーターを含む) で構成される辞書キーにキャッシュされます。つまり、デコレーターが適切に動作するには、これらの関数のパラメーターがハッシュ可能である必要があります。
② フィボナッチ数列
誰もがフィボナッチ数列の計算方法を知っているはずです。一般的な解決策は、再帰を使用することです:
-
0, 1, 1, 2, 3, 5, 8, 13, 21, 34 ……;
- ##2 は前の 2 つの項目の合計です ->( 1 1);
- 3 は前の 2 つの項目の合計です -> (1 2);
- 5 は前の 2 つの項目の合計です -> ;(2 3)。
递归的计算简洁并且直观,但是由于存在大量重复计算,实际运行效率很低,并且会占用较多的内存。但是这里并不是需要关注的重点,只是来作为演示示例而已:
# 匿名函数 fib = lambda n: 1 if n <= 1 else fib(n-1) + fib(n-2) # 将时间复杂度降低到线性 fib = lambda n, a=1, b=1: a if n == 0 else fib(n-1, b, a+b) # 保证了匿名函数的匿名性 fib = lambda n, fib: 1 if n <= 1 else fib(n-1, fib) + fib(n-2, fib)
③ 使用 @lru_cache 缓存输出结果
使用 @lru_cache 装饰器来缓存的话,可以将函数调用结果存储在内存中,以便再次请求时直接返回结果:
from functools import lru_cache @lru_cache def fib(n): if n==1 or n==2: return 1 else: return fib(n-1) + fib(n-2)
④ 限制 @lru_cache 装饰器大小
Python 的 @lru_cache 装饰器提供了一个 maxsize 属性,该属性定义了在缓存开始淘汰旧条目之前的最大条目数,默认情况下,maxsize 设置为 128。
如果将 maxsize 设置为 None 的话,则缓存将无限期增长,并且不会驱逐任何条目。
from functools import lru_cache @lru_cache(maxsize=16) def fib(n): if n==1 or n==2: return 1 else: return fib(n-1) + fib(n-2)
# 查看缓存列表 >>> print(steps_to.cache_info()) CacheInfo(hits=52, misses=30, maxsize=16, currsize=16)
⑤ 使用 @lru_cache 实现 LRU 缓存
就像在前面实现的缓存解决方案一样,@lru_cache 在底层使用一个字典,它将函数的结果缓存在一个键下,该键包含对函数的调用,包括提供的参数。这意味着这些参数必须是可哈希的,才能让 decorator 工作。
示例:玩楼梯:
想象一下,你想通过一次跳上一个、两个或三个楼梯来确定到达楼梯中的一个特定楼梯的所有不同方式,到第四个楼梯有多少条路?所有不同的组合如下所示:
可以这样描述,为了到达当前的楼梯,你可以从下面的一个、两个或三个楼梯跳下去,将能够到达这些点的跳跃组合的数量相加,便能够获得到达当前位置的所有可能方法。
例如到达第四个楼梯的组合数量将等于你到达第三、第二和第一个楼梯的不同方式的总数。如下所示,有七种不同的方法可以到达第四层楼梯:
注意给定阶梯的解是如何建立在较小子问题的答案之上的,在这种情况下,为了确定到达第四个楼梯的不同路径,可以将到达第三个楼梯的四种路径、到达第二个楼梯的两种路径以及到达第一个楼梯的一种路径相加。 这种方法称为递归,下面是一个实现这个递归的函数:
def steps_to(stair): if stair == 1: # You can reach the first stair with only a single step # from the floor. return 1 elif stair == 2: # You can reach the second stair by jumping from the # floor with a single two-stair hop or by jumping a single # stair a couple of times. return 2 elif stair == 3: # You can reach the third stair using four possible # combinations: # 1. Jumping all the way from the floor # 2. Jumping two stairs, then one # 3. Jumping one stair, then two # 4. Jumping one stair three times return 4 else: # You can reach your current stair from three different places: # 1. From three stairs down # 2. From two stairs down # 2. From one stair down # # If you add up the number of ways of getting to those # those three positions, then you should have your solution. return ( steps_to(stair - 3) + steps_to(stair - 2) + steps_to(stair - 1) ) print(steps_to(4))
将此代码保存到一个名为 stairs.py 的文件中,并使用以下命令运行它:
$ python stairs.py 7
太棒了,这个代码适用于 4 个楼梯,但是数一下要走多少步才能到达楼梯上更高的地方呢?将第 33 行中的楼梯数更改为 30,并重新运行脚本:
$ python stairs.py 53798080
可以看到结果超过 5300 万个组合,这可真的有点多。
时间代码:
当找到第 30 个楼梯的解决方案时,脚本花了相当多的时间来完成。要获得基线,可以度量代码运行的时间,要做到这一点,可以使用 Python 的 timeit module,在第 33 行之后添加以下代码:
setup_code = "from __main__ import steps_to" 36stmt = "steps_to(30)" 37times = repeat(setup=setup_code, stmt=stmt, repeat=3, number=10) 38print(f"Minimum execution time: {min(times)}")
还需要在代码的顶部导入 timeit module:
from timeit import repeat
以下是对这些新增内容的逐行解释:
第 35 行导入 steps_to() 的名称,以便 time.com .repeat() 知道如何调用它;
第 36 行用希望到达的楼梯数(在本例中为 30)准备对函数的调用,这是将要执行和计时的语句;
第 37 行使用设置代码和语句调用 time.repeat(),这将调用该函数 10 次,返回每次执行所需的秒数;
第 38 行标识并打印返回的最短时间。 现在再次运行脚本:
$ python stairs.py 53798080 Minimum execution time: 40.014977024000004
可以看到的秒数取决于特定硬件,在我的系统上,脚本花了 40 秒,这对于 30 级楼梯来说是相当慢的。
使用记忆来改进解决方案:
这种递归实现通过将其分解为相互构建的更小的步骤来解决这个问题,如下所示是一个树,其中每个节点表示对 steps_to() 的特定调用:
注意需要如何使用相同的参数多次调用 steps_to(),例如 steps_to(5) 计算两次,steps_to(4) 计算四次,steps_to(3) 计算七次,steps_to(2) 计算六次,多次调用同一个函数会增加不必要的计算周期,结果总是相同的。
为了解决这个问题,可以使用一种叫做记忆的技术,这种方法将函数的结果存储在内存中,然后在需要时引用它,从而确保函数不会为相同的输入运行多次,这个场景听起来像是使用 Python 的 @lru_cache 装饰器的绝佳机会。
只要做两个改变,就可以大大提高算法的运行时间:
从 functools module 导入 @lru_cache 装饰器;
使用 @lru_cache 装饰 steps_to()。
下面是两个更新后的脚本顶部的样子:
from functools import lru_cache from timeit import repeat @lru_cache def steps_to(stair): if stair == 1:
运行更新后的脚本产生如下结果:
$ python stairs.py 53798080 Minimum execution time: 7.999999999987184e-07
缓存函数的结果会将运行时从 40 秒降低到 0.0008 毫秒,这是一个了不起的进步。@lru_cache 装饰器存储了每个不同输入的 steps_to() 的结果,每次代码调用带有相同参数的函数时,它都直接从内存中返回正确的结果,而不是重新计算一遍答案,这解释了使用 @lru_cache 时性能的巨大提升。
⑥ 解包 @lru_cache 的功能
有了@lru_cache 装饰器,就可以将每个调用和应答存储在内存中,以便以后再次请求时进行访问,但是在内存耗尽之前,可以节省多少次调用呢?
Python 的 @lru_cache 装饰器提供了一个 maxsize 属性,它定义了在缓存开始清除旧条目之前的最大条目数,缺省情况下,maxsize 设置为 128,如果将 maxsize 设置为 None,那么缓存将无限增长,并且不会驱逐任何条目。如果在内存中存储大量不同的调用,这可能会成为一个问题。
如下是 @lru_cache 使用 maxsize 属性:
from functools import lru_cache from timeit import repeat @lru_cache(maxsize=16) def steps_to(stair): if stair == 1:
在本例中,将缓存限制为最多 16 个条目,当一个新调用传入时,decorator 的实现将会从现有的 16 个条目中删除最近最少使用的条目,为新条目腾出位置。
要查看添加到代码中的新内容会发生什么,可以使用 @lru_cache 装饰器提供的 cache_info() 来检查命中和未命中的次数以及当前缓存的大小。为了清晰起见,删除乘以函数运行时的代码,以下是修改后的最终脚本:
from functools import lru_cache from timeit import repeat @lru_cache(maxsize=16) def steps_to(stair): if stair == 1: # You can reach the first stair with only a single step # from the floor. return 1 elif stair == 2: # You can reach the second stair by jumping from the # floor with a single two-stair hop or by jumping a single # stair a couple of times. return 2 elif stair == 3: # You can reach the third stair using four possible # combinations: # 1. Jumping all the way from the floor # 2. Jumping two stairs, then one # 3. Jumping one stair, then two # 4. Jumping one stair three times return 4 else: # You can reach your current stair from three different places: # 1. From three stairs down # 2. From two stairs down # 2. From one stair down # # If you add up the number of ways of getting to those # those three positions, then you should have your solution. return ( steps_to(stair - 3) + steps_to(stair - 2) + steps_to(stair - 1) ) print(steps_to(30)) print(steps_to.cache_info())
如果再次调用脚本,可以看到如下结果:
$ python stairs.py 53798080 CacheInfo(hits=52, misses=30, maxsize=16, currsize=16)
可以使用 cache_info() 返回的信息来了解缓存是如何执行的,并对其进行微调,以找到速度和存储之间的适当平衡。下面是 cache_info() 提供的属性的详细说明:
hits=52 是 @lru_cache 直接从内存中返回的调用数,因为它们存在于缓存中;
misses =30 是被计算的不是来自内存的调用数,因为试图找到到达第 30 级楼梯的台阶数,所以每次调用都在第一次调用时错过了缓存是有道理的;
maxsize =16 是用装饰器的 maxsize 属性定义的缓存的大小;
currsize =16 是当前缓存的大小,在本例中它表明缓存已满。
如果需要从缓存中删除所有条目,那么可以使用 @lru_cache 提供的 cache_clear()。
四、添加缓存过期
假设想要开发一个脚本来监视 Real Python 并在任何包含单词 Python 的文章中打印字符数。真正的 Python 提供了一个 Atom feed,因此可以使用 feedparser 库来解析提要,并使用请求库来加载本文的内容。
如下是监控脚本的实现:
import feedparser import requests import ssl import time if hasattr(ssl, "_create_unverified_context"): ssl._create_default_https_context = ssl._create_unverified_context def get_article_from_server(url): print("Fetching article from server...") response = requests.get(url) return response.text def monitor(url): maxlen = 45 while True: print("\nChecking feed...") feed = feedparser.parse(url) for entry in feed.entries[:5]: if "python" in entry.title.lower(): truncated_title = ( entry.title[:maxlen] + "..." if len(entry.title) > maxlen else entry.title ) print( "Match found:", truncated_title, len(get_article_from_server(entry.link)), ) time.sleep(5) monitor("https://realpython.com/atom.xml")
将此脚本保存到一个名为 monitor.py 的文件中,安装 feedparser 和请求库,然后运行该脚本,它将持续运行,直到在终端窗口中按 Ctrl+C 停止它:
$ pip install feedparser requests $ python monitor.py Checking feed... Fetching article from server... The Real Python Podcast – Episode #28: Using ... 29520 Fetching article from server... Python Community Interview With David Amos 54256 Fetching article from server... Working With Linked Lists in Python 37099 Fetching article from server... Python Practice Problems: Get Ready for Your ... 164888 Fetching article from server... The Real Python Podcast – Episode #27: Prepar... 30784 Checking feed... Fetching article from server... The Real Python Podcast – Episode #28: Using ... 29520 Fetching article from server... Python Community Interview With David Amos 54256 Fetching article from server... Working With Linked Lists in Python 37099 Fetching article from server... Python Practice Problems: Get Ready for Your ... 164888 Fetching article from server... The Real Python Podcast – Episode #27: Prepar... 30784
代码解释:
第 6 行和第 7 行:当 feedparser 试图访问通过 HTTPS 提供的内容时,这是一个解决方案;
第 16 行:monitor() 将无限循环;
第 18 行:使用 feedparser,代码从真正的 Python 加载并解析提要;
第 20 行:循环遍历列表中的前 5 个条目;
第 21 到 31 行:如果单词 python 是标题的一部分,那么代码将连同文章的长度一起打印它;
第 33 行:代码在继续之前休眠了 5 秒钟;
第 35 行:这一行通过将 Real Python 提要的 URL 传递给 monitor() 来启动监视过程。
每当脚本加载一篇文章时,“Fetching article from server…”的消息就会打印到控制台,如果让脚本运行足够长的时间,那么将看到这条消息是如何反复显示的,即使在加载相同的链接时也是如此。
这是一个很好的机会来缓存文章的内容,并避免每五秒钟访问一次网络,可以使用 @lru_cache 装饰器,但是如果文章的内容被更新,会发生什么呢?第一次访问文章时,装饰器将存储文章的内容,并在以后每次返回相同的数据;如果更新了帖子,那么监视器脚本将永远无法实现它,因为它将提取存储在缓存中的旧副本。要解决这个问题,可以将缓存条目设置为过期。
from functools import lru_cache, wraps from datetime import datetime, timedelta def timed_lru_cache(seconds: int, maxsize: int = 128): def wrapper_cache(func): func = lru_cache(maxsize=maxsize)(func) func.lifetime = timedelta(seconds=seconds) func.expiration = datetime.utcnow() + func.lifetime @wraps(func) def wrapped_func(*args, **kwargs): if datetime.utcnow() >= func.expiration: func.cache_clear() func.expiration = datetime.utcnow() + func.lifetime return func(*args, **kwargs) return wrapped_func return wrapper_cache @timed_lru_cache(10) def get_article_from_server(url): ...
代码解释:
第 4 行:@timed_lru_cache 装饰器将支持缓存中条目的生命周期(以秒为单位)和缓存的最大大小;
第 6 行:代码用 lru_cache 装饰器包装了装饰函数,这允许使用 lru_cache 已经提供的缓存功能;
第 7 行和第 8 行:这两行用两个表示缓存生命周期和它将过期的实际日期的属性来修饰函数;
第 12 到 14 行:在访问缓存中的条目之前,装饰器检查当前日期是否超过了过期日期,如果是这种情况,那么它将清除缓存并重新计算生存期和过期日期。
请注意,当条目过期时,此装饰器如何清除与该函数关联的整个缓存,生存期适用于整个缓存,而不适用于单个项目,此策略的更复杂实现将根据条目的单个生存期将其逐出。
在程序中,如果想要实现不同缓存策略,可以查看 cachetools 这个库,该库提供了几个集合和修饰符,涵盖了一些最流行的缓存策略。
使用新装饰器缓存文章:
现在可以将新的 @timed_lru_cache 装饰器与监视器脚本一起使用,以防止每次访问时获取文章的内容。为了简单起见,把代码放在一个脚本中,可以得到以下结果:
import feedparser import requests import ssl import time from functools import lru_cache, wraps from datetime import datetime, timedelta if hasattr(ssl, "_create_unverified_context"): ssl._create_default_https_context = ssl._create_unverified_context def timed_lru_cache(seconds: int, maxsize: int = 128): def wrapper_cache(func): func = lru_cache(maxsize=maxsize)(func) func.lifetime = timedelta(seconds=seconds) func.expiration = datetime.utcnow() + func.lifetime @wraps(func) def wrapped_func(*args, **kwargs): if datetime.utcnow() >= func.expiration: func.cache_clear() func.expiration = datetime.utcnow() + func.lifetime return func(*args, **kwargs) return wrapped_func return wrapper_cache @timed_lru_cache(10) def get_article_from_server(url): print("Fetching article from server...") response = requests.get(url) return response.text def monitor(url): maxlen = 45 while True: print("\nChecking feed...") feed = feedparser.parse(url) for entry in feed.entries[:5]: if "python" in entry.title.lower(): truncated_title = ( entry.title[:maxlen] + "..." if len(entry.title) > maxlen else entry.title ) print( "Match found:", truncated_title, len(get_article_from_server(entry.link)), ) time.sleep(5) monitor("https://realpython.com/atom.xml")
请注意第 30 行如何使用 @timed_lru_cache 装饰 get_article_from_server() 并指定 10 秒的有效性。在获取文章后的 10 秒内,任何试图从服务器访问同一篇文章的尝试都将从缓存中返回内容,而不会到达网络。
运行脚本并查看结果:
$ python monitor.py Checking feed... Fetching article from server... Match found: The Real Python Podcast – Episode #28: Using ... 29521 Fetching article from server... Match found: Python Community Interview With David Amos 54254 Fetching article from server... Match found: Working With Linked Lists in Python 37100 Fetching article from server... Match found: Python Practice Problems: Get Ready for Your ... 164887 Fetching article from server... Match found: The Real Python Podcast – Episode #27: Prepar... 30783 Checking feed... Match found: The Real Python Podcast – Episode #28: Using ... 29521 Match found: Python Community Interview With David Amos 54254 Match found: Working With Linked Lists in Python 37100 Match found: Python Practice Problems: Get Ready for Your ... 164887 Match found: The Real Python Podcast – Episode #27: Prepar... 30783 Checking feed... Match found: The Real Python Podcast – Episode #28: Using ... 29521 Match found: Python Community Interview With David Amos 54254 Match found: Working With Linked Lists in Python 37100 Match found: Python Practice Problems: Get Ready for Your ... 164887 Match found: The Real Python Podcast – Episode #27: Prepar... 30783 Checking feed... Fetching article from server... Match found: The Real Python Podcast – Episode #28: Using ... 29521 Fetching article from server... Match found: Python Community Interview With David Amos 54254 Fetching article from server... Match found: Working With Linked Lists in Python 37099 Fetching article from server... Match found: Python Practice Problems: Get Ready for Your ... 164888 Fetching article from server... Match found: The Real Python Podcast – Episode #27: Prepar... 30783
请注意,代码在第一次访问匹配的文章时是如何打印“Fetching article from server…”这条消息的。之后,根据网络速度和计算能力,脚本将从缓存中检索文章一两次,然后再次访问服务器。
该脚本试图每 5 秒访问这些文章,缓存每 10 秒过期一次。对于实际的应用程序来说,这些时间可能太短,因此可以通过调整这些配置来获得显著的改进。
五、@lru_cache 装饰器的官方实现
简单理解,其实就是一个装饰器:
def lru_cache(maxsize=128, typed=False): if isinstance(maxsize, int): if maxsize < 0: maxsize = 0 elif callable(maxsize) and isinstance(typed, bool): user_function, maxsize = maxsize, 128 wrapper = _lru_cache_wrapper(user_function, maxsize, typed, _CacheInfo) return update_wrapper(wrapper, user_function) elif maxsize is not None: raise TypeError('Expected first argument to be an integer, a callable, or None') def decorating_function(user_function): wrapper = _lru_cache_wrapper(user_function, maxsize, typed, _CacheInfo) return update_wrapper(wrapper, user_function) return decorating_function
_CacheInfo = namedtuple("CacheInfo", ["hits", "misses", "maxsize", "currsize"]) def _lru_cache_wrapper(user_function, maxsize, typed, _CacheInfo): sentinel = object() # unique object used to signal cache misses make_key = _make_key # build a key from the function arguments PREV, NEXT, KEY, RESULT = 0, 1, 2, 3 # names for the link fields cache = {} # 存储也使用的字典 hits = misses = 0 full = False cache_get = cache.get cache_len = cache.__len__ lock = RLock() # 因为双向链表的更新不是线程安全的所以需要加锁 root = [] # 双向链表 root[:] = [root, root, None, None] # 初始化双向链表 if maxsize == 0: def wrapper(*args, **kwds): # No caching -- just a statistics update nonlocal misses misses += 1 result = user_function(*args, **kwds) return result elif maxsize is None: def wrapper(*args, **kwds): # Simple caching without ordering or size limit nonlocal hits, misses key = make_key(args, kwds, typed) result = cache_get(key, sentinel) if result is not sentinel: hits += 1 return result misses += 1 result = user_function(*args, **kwds) cache[key] = result return result else: def wrapper(*args, **kwds): # Size limited caching that tracks accesses by recency nonlocal root, hits, misses, full key = make_key(args, kwds, typed) with lock: link = cache_get(key) if link is not None: # Move the link to the front of the circular queue link_prev, link_next, _key, result = link link_prev[NEXT] = link_next link_next[PREV] = link_prev last = root[PREV] last[NEXT] = root[PREV] = link link[PREV] = last link[NEXT] = root hits += 1 return result misses += 1 result = user_function(*args, **kwds) with lock: if key in cache: pass elif full: oldroot = root oldroot[KEY] = key oldroot[RESULT] = result root = oldroot[NEXT] oldkey = root[KEY] oldresult = root[RESULT] root[KEY] = root[RESULT] = None del cache[oldkey] cache[key] = oldroot else: last = root[PREV] link = [last, root, key, result] last[NEXT] = root[PREV] = cache[key] = link full = (cache_len() >= maxsize) return result def cache_info(): """Report cache statistics""" with lock: return _CacheInfo(hits, misses, maxsize, cache_len()) def cache_clear(): """Clear the cache and cache statistics""" nonlocal hits, misses, full with lock: cache.clear() root[:] = [root, root, None, None] hits = misses = 0 full = False wrapper.cache_info = cache_info wrapper.cache_clear = cache_clear return wrapper
以上がPython がキャッシュに LRU キャッシュ戦略を使用する方法の詳細内容です。詳細については、PHP 中国語 Web サイトの他の関連記事を参照してください。
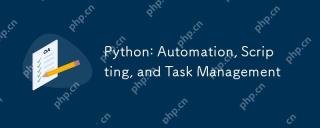
Pythonは、自動化、スクリプト、およびタスク管理に優れています。 1)自動化:OSやShutilなどの標準ライブラリを介してファイルバックアップが実現されます。 2)スクリプトの書き込み:Psutilライブラリを使用してシステムリソースを監視します。 3)タスク管理:スケジュールライブラリを使用してタスクをスケジュールします。 Pythonの使いやすさと豊富なライブラリサポートにより、これらの分野で優先ツールになります。
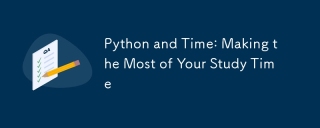
限られた時間でPythonの学習効率を最大化するには、PythonのDateTime、時間、およびスケジュールモジュールを使用できます。 1. DateTimeモジュールは、学習時間を記録および計画するために使用されます。 2。時間モジュールは、勉強と休息の時間を設定するのに役立ちます。 3.スケジュールモジュールは、毎週の学習タスクを自動的に配置します。
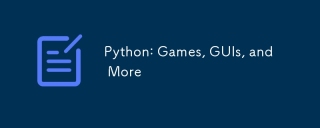
PythonはゲームとGUI開発に優れています。 1)ゲーム開発は、2Dゲームの作成に適した図面、オーディオ、その他の機能を提供し、Pygameを使用します。 2)GUI開発は、TKINTERまたはPYQTを選択できます。 TKINTERはシンプルで使いやすく、PYQTは豊富な機能を備えており、専門能力開発に適しています。
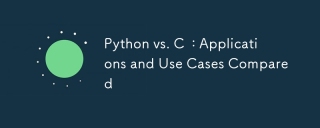
Pythonは、データサイエンス、Web開発、自動化タスクに適していますが、Cはシステムプログラミング、ゲーム開発、組み込みシステムに適しています。 Pythonは、そのシンプルさと強力なエコシステムで知られていますが、Cは高性能および基礎となる制御機能で知られています。
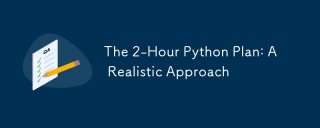
2時間以内にPythonの基本的なプログラミングの概念とスキルを学ぶことができます。 1.変数とデータ型、2。マスターコントロールフロー(条件付きステートメントとループ)、3。機能の定義と使用を理解する4。
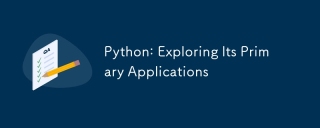
Pythonは、Web開発、データサイエンス、機械学習、自動化、スクリプトの分野で広く使用されています。 1)Web開発では、DjangoおよびFlask Frameworksが開発プロセスを簡素化します。 2)データサイエンスと機械学習の分野では、Numpy、Pandas、Scikit-Learn、Tensorflowライブラリが強力なサポートを提供します。 3)自動化とスクリプトの観点から、Pythonは自動テストやシステム管理などのタスクに適しています。
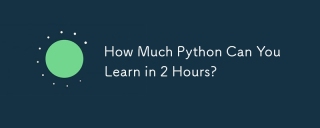
2時間以内にPythonの基本を学ぶことができます。 1。変数とデータ型を学習します。2。ステートメントやループの場合などのマスター制御構造、3。関数の定義と使用を理解します。これらは、簡単なPythonプログラムの作成を開始するのに役立ちます。
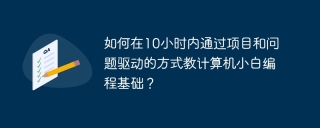
10時間以内にコンピューター初心者プログラミングの基本を教える方法は?コンピューター初心者にプログラミングの知識を教えるのに10時間しかない場合、何を教えることを選びますか...


ホットAIツール

Undresser.AI Undress
リアルなヌード写真を作成する AI 搭載アプリ

AI Clothes Remover
写真から衣服を削除するオンライン AI ツール。

Undress AI Tool
脱衣画像を無料で

Clothoff.io
AI衣類リムーバー

AI Hentai Generator
AIヘンタイを無料で生成します。

人気の記事

ホットツール

AtomエディタMac版ダウンロード
最も人気のあるオープンソースエディター

Safe Exam Browser
Safe Exam Browser は、オンライン試験を安全に受験するための安全なブラウザ環境です。このソフトウェアは、あらゆるコンピュータを安全なワークステーションに変えます。あらゆるユーティリティへのアクセスを制御し、学生が無許可のリソースを使用するのを防ぎます。

ゼンドスタジオ 13.0.1
強力な PHP 統合開発環境

SublimeText3 英語版
推奨: Win バージョン、コードプロンプトをサポート!

メモ帳++7.3.1
使いやすく無料のコードエディター
