線形回帰
1. 線形回帰関数
尤度関数の定義: 指定された結合サンプル値X (不明な) パラメータに関する次の関数
##尤度関数: このようなパラメータは正確には何ですかデータと組み合わせたときの真の値
2. 線形回帰尤度関数
勾配降下法タスク、ロジスティック回帰目的関数に変換
#シグモイド関数
def sigmoid(z): return 1 / (1 + np.exp(-z))
予測関数
def model(X, theta): return sigmoid(np.dot(X, theta.T))
目的関数
def cost(X, y, theta): left = np.multiply(-y, np.log(model(X, theta))) right = np.multiply(1 - y, np.log(1 - model(X, theta))) return np.sum(left - right) / (len(X))
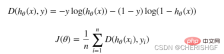
def gradient(X, y, theta): grad = np.zeros(theta.shape) error = (model(X, theta)- y).ravel() for j in range(len(theta.ravel())): #for each parmeter term = np.multiply(error, X[:,j]) grad[0, j] = np.sum(term) / len(X) return grad
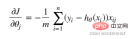
STOP_ITER = 0 STOP_COST = 1 STOP_GRAD = 2 def stopCriterion(type, value, threshold): # 设定三种不同的停止策略 if type == STOP_ITER: # 设定迭代次数 return value > threshold elif type == STOP_COST: # 根据损失值停止 return abs(value[-1] - value[-2]) < threshold elif type == STOP_GRAD: # 根据梯度变化停止 return np.linalg.norm(value) < threshold
サンプルの再シャッフル
import numpy.random #洗牌 def shuffleData(data): np.random.shuffle(data) cols = data.shape[1] X = data[:, 0:cols-1] y = data[:, cols-1:] return X, y
勾配降下ソリューション
def descent(data, theta, batchSize, stopType, thresh, alpha): # 梯度下降求解 init_time = time.time() i = 0 # 迭代次数 k = 0 # batch X, y = shuffleData(data) grad = np.zeros(theta.shape) # 计算的梯度 costs = [cost(X, y, theta)] # 损失值 while True: grad = gradient(X[k:k + batchSize], y[k:k + batchSize], theta) k += batchSize # 取batch数量个数据 if k >= n: k = 0 X, y = shuffleData(data) # 重新洗牌 theta = theta - alpha * grad # 参数更新 costs.append(cost(X, y, theta)) # 计算新的损失 i += 1 if stopType == STOP_ITER: value = i elif stopType == STOP_COST: value = costs elif stopType == STOP_GRAD: value = grad if stopCriterion(stopType, value, thresh): break return theta, i - 1, costs, grad, time.time() - init_time
完了code
import numpy as np import pandas as pd import matplotlib.pyplot as plt import os import numpy.random import time def sigmoid(z): return 1 / (1 + np.exp(-z)) def model(X, theta): return sigmoid(np.dot(X, theta.T)) def cost(X, y, theta): left = np.multiply(-y, np.log(model(X, theta))) right = np.multiply(1 - y, np.log(1 - model(X, theta))) return np.sum(left - right) / (len(X)) def gradient(X, y, theta): grad = np.zeros(theta.shape) error = (model(X, theta) - y).ravel() for j in range(len(theta.ravel())): # for each parmeter term = np.multiply(error, X[:, j]) grad[0, j] = np.sum(term) / len(X) return grad STOP_ITER = 0 STOP_COST = 1 STOP_GRAD = 2 def stopCriterion(type, value, threshold): # 设定三种不同的停止策略 if type == STOP_ITER: # 设定迭代次数 return value > threshold elif type == STOP_COST: # 根据损失值停止 return abs(value[-1] - value[-2]) < threshold elif type == STOP_GRAD: # 根据梯度变化停止 return np.linalg.norm(value) < threshold # 洗牌 def shuffleData(data): np.random.shuffle(data) cols = data.shape[1] X = data[:, 0:cols - 1] y = data[:, cols - 1:] return X, y def descent(data, theta, batchSize, stopType, thresh, alpha): # 梯度下降求解 init_time = time.time() i = 0 # 迭代次数 k = 0 # batch X, y = shuffleData(data) grad = np.zeros(theta.shape) # 计算的梯度 costs = [cost(X, y, theta)] # 损失值 while True: grad = gradient(X[k:k + batchSize], y[k:k + batchSize], theta) k += batchSize # 取batch数量个数据 if k >= n: k = 0 X, y = shuffleData(data) # 重新洗牌 theta = theta - alpha * grad # 参数更新 costs.append(cost(X, y, theta)) # 计算新的损失 i += 1 if stopType == STOP_ITER: value = i elif stopType == STOP_COST: value = costs elif stopType == STOP_GRAD: value = grad if stopCriterion(stopType, value, thresh): break return theta, i - 1, costs, grad, time.time() - init_time def runExpe(data, theta, batchSize, stopType, thresh, alpha): # import pdb # pdb.set_trace() theta, iter, costs, grad, dur = descent(data, theta, batchSize, stopType, thresh, alpha) name = "Original" if (data[:, 1] > 2).sum() > 1 else "Scaled" name += " data - learning rate: {} - ".format(alpha) if batchSize == n: strDescType = "Gradient" # 批量梯度下降 elif batchSize == 1: strDescType = "Stochastic" # 随机梯度下降 else: strDescType = "Mini-batch ({})".format(batchSize) # 小批量梯度下降 name += strDescType + " descent - Stop: " if stopType == STOP_ITER: strStop = "{} iterations".format(thresh) elif stopType == STOP_COST: strStop = "costs change < {}".format(thresh) else: strStop = "gradient norm < {}".format(thresh) name += strStop print("***{}\nTheta: {} - Iter: {} - Last cost: {:03.2f} - Duration: {:03.2f}s".format( name, theta, iter, costs[-1], dur)) fig, ax = plt.subplots(figsize=(12, 4)) ax.plot(np.arange(len(costs)), costs, 'r') ax.set_xlabel('Iterations') ax.set_ylabel('Cost') ax.set_title(name.upper() + ' - Error vs. Iteration') return theta path = 'data' + os.sep + 'LogiReg_data.txt' pdData = pd.read_csv(path, header=None, names=['Exam 1', 'Exam 2', 'Admitted']) positive = pdData[pdData['Admitted'] == 1] negative = pdData[pdData['Admitted'] == 0] # 画图观察样本情况 fig, ax = plt.subplots(figsize=(10, 5)) ax.scatter(positive['Exam 1'], positive['Exam 2'], s=30, c='b', marker='o', label='Admitted') ax.scatter(negative['Exam 1'], negative['Exam 2'], s=30, c='r', marker='x', label='Not Admitted') ax.legend() ax.set_xlabel('Exam 1 Score') ax.set_ylabel('Exam 2 Score') pdData.insert(0, 'Ones', 1) # 划分训练数据与标签 orig_data = pdData.values cols = orig_data.shape[1] X = orig_data[:, 0:cols - 1] y = orig_data[:, cols - 1:cols] # 设置初始参数0 theta = np.zeros([1, 3]) # 选择的梯度下降方法是基于所有样本的 n = 100 runExpe(orig_data, theta, n, STOP_ITER, thresh=5000, alpha=0.000001) runExpe(orig_data, theta, n, STOP_COST, thresh=0.000001, alpha=0.001) runExpe(orig_data, theta, n, STOP_GRAD, thresh=0.05, alpha=0.001) runExpe(orig_data, theta, 1, STOP_ITER, thresh=5000, alpha=0.001) runExpe(orig_data, theta, 1, STOP_ITER, thresh=15000, alpha=0.000002) runExpe(orig_data, theta, 16, STOP_ITER, thresh=15000, alpha=0.001) from sklearn import preprocessing as pp # 数据预处理 scaled_data = orig_data.copy() scaled_data[:, 1:3] = pp.scale(orig_data[:, 1:3]) runExpe(scaled_data, theta, n, STOP_ITER, thresh=5000, alpha=0.001) runExpe(scaled_data, theta, n, STOP_GRAD, thresh=0.02, alpha=0.001) theta = runExpe(scaled_data, theta, 1, STOP_GRAD, thresh=0.002 / 5, alpha=0.001) runExpe(scaled_data, theta, 16, STOP_GRAD, thresh=0.002 * 2, alpha=0.001) # 设定阈值 def predict(X, theta): return [1 if x >= 0.5 else 0 for x in model(X, theta)] # 计算精度 scaled_X = scaled_data[:, :3] y = scaled_data[:, 3] predictions = predict(scaled_X, theta) correct = [1 if ((a == 1 and b == 1) or (a == 0 and b == 0)) else 0 for (a, b) in zip(predictions, y)] accuracy = (sum(map(int, correct)) % len(correct)) print('accuracy = {0}%'.format(accuracy))
ロジスティック回帰の長所と短所
利点
- 形式はシンプルで、モデルの解釈性は非常に優れています。特徴量の重みから、さまざまな特徴量が最終結果に及ぼす影響を確認できます。特定の特徴量の重み値が比較的高い場合、この特徴量は最終結果に大きな影響を与えます。
- モデルはうまく機能します。 (ベースラインとして) エンジニアリングでは許容されます。特徴量エンジニアリングが適切に行われていれば、その効果はそれほど悪くはなく、特徴量エンジニアリングを並行して開発できるため、開発のスピードが大幅に向上します。
- トレーニング速度が速くなります。分類する場合、計算量は特徴の数にのみ関係します。さらに、ロジスティック回帰の分散最適化 sgd は比較的成熟しており、ヒープ マシンによってトレーニング速度をさらに向上させることができるため、短期間でモデルの複数のバージョンを反復することができます。
- リソース、特にメモリをほとんど消費しません。各次元の特徴量のみを保存する必要があるためです。
- 出力結果を便利に調整します。ロジスティック回帰では、出力が各サンプルの確率スコアであるため、最終的な分類結果を簡単に得ることができます。また、これらの確率スコアを簡単に切り捨てる、つまり、しきい値を分割することができます(あるしきい値を超えるものは 1 つのカテゴリに分類され、一定の閾値未満のものを 1 つのカテゴリに分類します。一定の閾値を 1 つのカテゴリとします)。
- デメリット
- 精度はあまり高くありません。形式が非常に単純であるため (線形モデルに非常に似ている)、データの真の分布を当てはめるのは困難です。
データの不均衡の問題に対処するのは困難です。例: 正と負のサンプルの比率が 10000:1 であるなど、正と負のサンプルが非常にアンバランスである問題を扱う場合、すべてのサンプルを正であると予測する場合、損失関数の値を作成することもできます。より小さい。しかし、分類器としては、陽性サンプルと陰性サンプルを区別する能力はあまり良くありません。
非線形データの処理はさらに面倒です。ロジスティック回帰は、他の手法を導入しないと、線形分離可能なデータのみを処理でき、さらには二項分類問題も処理できます。
ロジスティック回帰自体は特徴をフィルタリングできません。場合によっては、gbdt を使用して特徴をフィルタリングし、ロジスティック回帰を使用します。
以上がPython でロジスティック回帰を解くために勾配降下法を実装する方法の詳細内容です。詳細については、PHP 中国語 Web サイトの他の関連記事を参照してください。
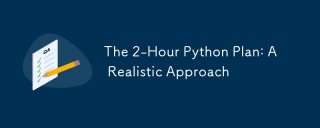
2時間以内にPythonの基本的なプログラミングの概念とスキルを学ぶことができます。 1.変数とデータ型、2。マスターコントロールフロー(条件付きステートメントとループ)、3。機能の定義と使用を理解する4。
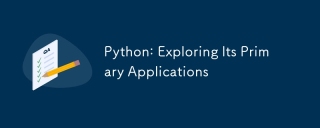
Pythonは、Web開発、データサイエンス、機械学習、自動化、スクリプトの分野で広く使用されています。 1)Web開発では、DjangoおよびFlask Frameworksが開発プロセスを簡素化します。 2)データサイエンスと機械学習の分野では、Numpy、Pandas、Scikit-Learn、Tensorflowライブラリが強力なサポートを提供します。 3)自動化とスクリプトの観点から、Pythonは自動テストやシステム管理などのタスクに適しています。
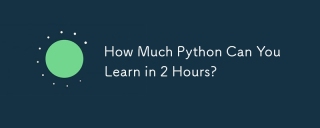
2時間以内にPythonの基本を学ぶことができます。 1。変数とデータ型を学習します。2。ステートメントやループの場合などのマスター制御構造、3。関数の定義と使用を理解します。これらは、簡単なPythonプログラムの作成を開始するのに役立ちます。
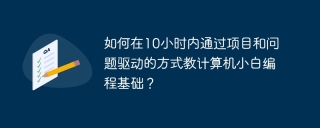
10時間以内にコンピューター初心者プログラミングの基本を教える方法は?コンピューター初心者にプログラミングの知識を教えるのに10時間しかない場合、何を教えることを選びますか...
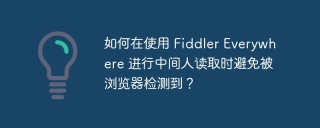
fiddlereveryversings for the-middleの測定値を使用するときに検出されないようにする方法
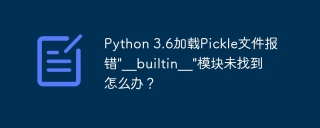
Python 3.6のピクルスファイルのロードレポートエラー:modulenotFounderror:nomodulenamed ...
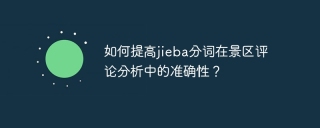
風光明媚なスポットコメント分析におけるJieba Wordセグメンテーションの問題を解決する方法は?風光明媚なスポットコメントと分析を行っているとき、私たちはしばしばJieba Wordセグメンテーションツールを使用してテキストを処理します...

正規表現を使用して、最初の閉じたタグと停止に一致する方法は? HTMLまたは他のマークアップ言語を扱う場合、しばしば正規表現が必要です...


ホットAIツール

Undresser.AI Undress
リアルなヌード写真を作成する AI 搭載アプリ

AI Clothes Remover
写真から衣服を削除するオンライン AI ツール。

Undress AI Tool
脱衣画像を無料で

Clothoff.io
AI衣類リムーバー

AI Hentai Generator
AIヘンタイを無料で生成します。

人気の記事

ホットツール

SublimeText3 Linux 新バージョン
SublimeText3 Linux 最新バージョン

ゼンドスタジオ 13.0.1
強力な PHP 統合開発環境

SublimeText3 中国語版
中国語版、とても使いやすい
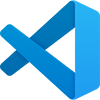
VSCode Windows 64 ビットのダウンロード
Microsoft によって発売された無料で強力な IDE エディター

mPDF
mPDF は、UTF-8 でエンコードされた HTML から PDF ファイルを生成できる PHP ライブラリです。オリジナルの作者である Ian Back は、Web サイトから「オンザフライ」で PDF ファイルを出力し、さまざまな言語を処理するために mPDF を作成しました。 HTML2FPDF などのオリジナルのスクリプトよりも遅く、Unicode フォントを使用すると生成されるファイルが大きくなりますが、CSS スタイルなどをサポートし、多くの機能強化が施されています。 RTL (アラビア語とヘブライ語) や CJK (中国語、日本語、韓国語) を含むほぼすべての言語をサポートします。ネストされたブロックレベル要素 (P、DIV など) をサポートします。
