###こんにちは、みんな。
AI テクノロジーを使用してモザイクを除去することを考えたことはありますか? これまで使用していた AI 技術は、顔認識であれ OCR 認識であれ、少なくとも手動で認識できるため、この問題を慎重に考えるのは非常に困難です。しかし、モザイク画像を渡された場合、元に戻すことはできるのでしょうか?#乱数をピクセルとして生成したノイズ マップ
#モデルは乱数から通常のアバターを生成
Pix2pix モデルの変換
CycleGAN モデル
データ セット
事前トレーニング モデル
Pix2pix モデル
python gan<span style="color: rgb(215, 58, 73); margin: 0px; padding: 0px; background: none 0% 0% / auto repeat scroll padding-box border-box rgba(0, 0, 0, 0);">/</span>infer<span style="color: rgb(0, 92, 197); margin: 0px; padding: 0px; background: none 0% 0% / auto repeat scroll padding-box border-box rgba(0, 0, 0, 0);">.py</span> <br><span style="color: rgb(106, 115, 125); margin: 0px; padding: 0px; background: none 0% 0% / auto repeat scroll padding-box border-box rgba(0, 0, 0, 0);">--init_model output/pix2pix/checkpoints/110/ </span><br><span style="color: rgb(106, 115, 125); margin: 0px; padding: 0px; background: none 0% 0% / auto repeat scroll padding-box border-box rgba(0, 0, 0, 0);">--dataset_dir /home/aistudio/ </span><br><span style="color: rgb(106, 115, 125); margin: 0px; padding: 0px; background: none 0% 0% / auto repeat scroll padding-box border-box rgba(0, 0, 0, 0);">--image_size 256 </span><br><span style="color: rgb(106, 115, 125); margin: 0px; padding: 0px; background: none 0% 0% / auto repeat scroll padding-box border-box rgba(0, 0, 0, 0);">--n_samples 1 </span><br><span style="color: rgb(106, 115, 125); margin: 0px; padding: 0px; background: none 0% 0% / auto repeat scroll padding-box border-box rgba(0, 0, 0, 0);">--crop_size 256 </span><br><span style="color: rgb(106, 115, 125); margin: 0px; padding: 0px; background: none 0% 0% / auto repeat scroll padding-box border-box rgba(0, 0, 0, 0);">--model_net Pix2pix </span><br><span style="color: rgb(106, 115, 125); margin: 0px; padding: 0px; background: none 0% 0% / auto repeat scroll padding-box border-box rgba(0, 0, 0, 0);">--net_G unet_256 </span><br><span style="color: rgb(106, 115, 125); margin: 0px; padding: 0px; background: none 0% 0% / auto repeat scroll padding-box border-box rgba(0, 0, 0, 0);">--test_list /home/aistudio/test_list.txt </span><br><span style="color: rgb(106, 115, 125); margin: 0px; padding: 0px; background: none 0% 0% / auto repeat scroll padding-box border-box rgba(0, 0, 0, 0);">--output ./infer_result/pix2pix/</span>CycleGAN モデル
python gan<span style="color: rgb(215, 58, 73); margin: 0px; padding: 0px; background: none 0% 0% / auto repeat scroll padding-box border-box rgba(0, 0, 0, 0);">/</span>infer<span style="color: rgb(0, 92, 197); margin: 0px; padding: 0px; background: none 0% 0% / auto repeat scroll padding-box border-box rgba(0, 0, 0, 0);">.py</span> <br><span style="color: rgb(106, 115, 125); margin: 0px; padding: 0px; background: none 0% 0% / auto repeat scroll padding-box border-box rgba(0, 0, 0, 0);">--init_model output/cyclegan/checkpoints/48/ </span><br><span style="color: rgb(106, 115, 125); margin: 0px; padding: 0px; background: none 0% 0% / auto repeat scroll padding-box border-box rgba(0, 0, 0, 0);">--dataset_dir /home/aistudio/ </span><br><span style="color: rgb(106, 115, 125); margin: 0px; padding: 0px; background: none 0% 0% / auto repeat scroll padding-box border-box rgba(0, 0, 0, 0);">--image_size 256 </span><br><span style="color: rgb(106, 115, 125); margin: 0px; padding: 0px; background: none 0% 0% / auto repeat scroll padding-box border-box rgba(0, 0, 0, 0);">--n_samples 1 </span><br><span style="color: rgb(106, 115, 125); margin: 0px; padding: 0px; background: none 0% 0% / auto repeat scroll padding-box border-box rgba(0, 0, 0, 0);">--crop_size 256 </span><br><span style="color: rgb(106, 115, 125); margin: 0px; padding: 0px; background: none 0% 0% / auto repeat scroll padding-box border-box rgba(0, 0, 0, 0);">--input_style A </span><br><span style="color: rgb(106, 115, 125); margin: 0px; padding: 0px; background: none 0% 0% / auto repeat scroll padding-box border-box rgba(0, 0, 0, 0);">--test_list /home/aistudio/test_list.txt </span><br><span style="color: rgb(106, 115, 125); margin: 0px; padding: 0px; background: none 0% 0% / auto repeat scroll padding-box border-box rgba(0, 0, 0, 0);">--model_net CycleGAN </span><br><span style="color: rgb(106, 115, 125); margin: 0px; padding: 0px; background: none 0% 0% / auto repeat scroll padding-box border-box rgba(0, 0, 0, 0);">--net_G resnet_9block </span><br><span style="color: rgb(106, 115, 125); margin: 0px; padding: 0px; background: none 0% 0% / auto repeat scroll padding-box border-box rgba(0, 0, 0, 0);">--g_base_dims 32 </span><br><span style="color: rgb(106, 115, 125); margin: 0px; padding: 0px; background: none 0% 0% / auto repeat scroll padding-box border-box rgba(0, 0, 0, 0);">--output ./infer_result/cyclegan/</span>トレーニング後、gan/infer.py ファイルを実行できます。この2機種のモザイク除去効果を比較してみます。 2. 既製のツールモデルを自分でトレーニングしたくない場合は、ここにある既製のプロジェクトを共有してください。これも、Pix2pix と CycleGAN を参照した、セマンティック セグメンテーションと画像変換に基づいています。 プロジェクト アドレス: https://github.com/HypoX64/DeepMosaics/blob/master/README_CN.mdWindows ユーザー向けに、作者は GUI を含むインストール不要のソフトウェア パッケージを提供していますインターフェース。
UI インターフェイス
コーディング
デコーディング
効果はまだ良好ですが、想像したほど完璧ではありません。ダウンロードして実行して試すことができます。
以上がAIはモザイクを除去できるのか?の詳細内容です。詳細については、PHP 中国語 Web サイトの他の関連記事を参照してください。
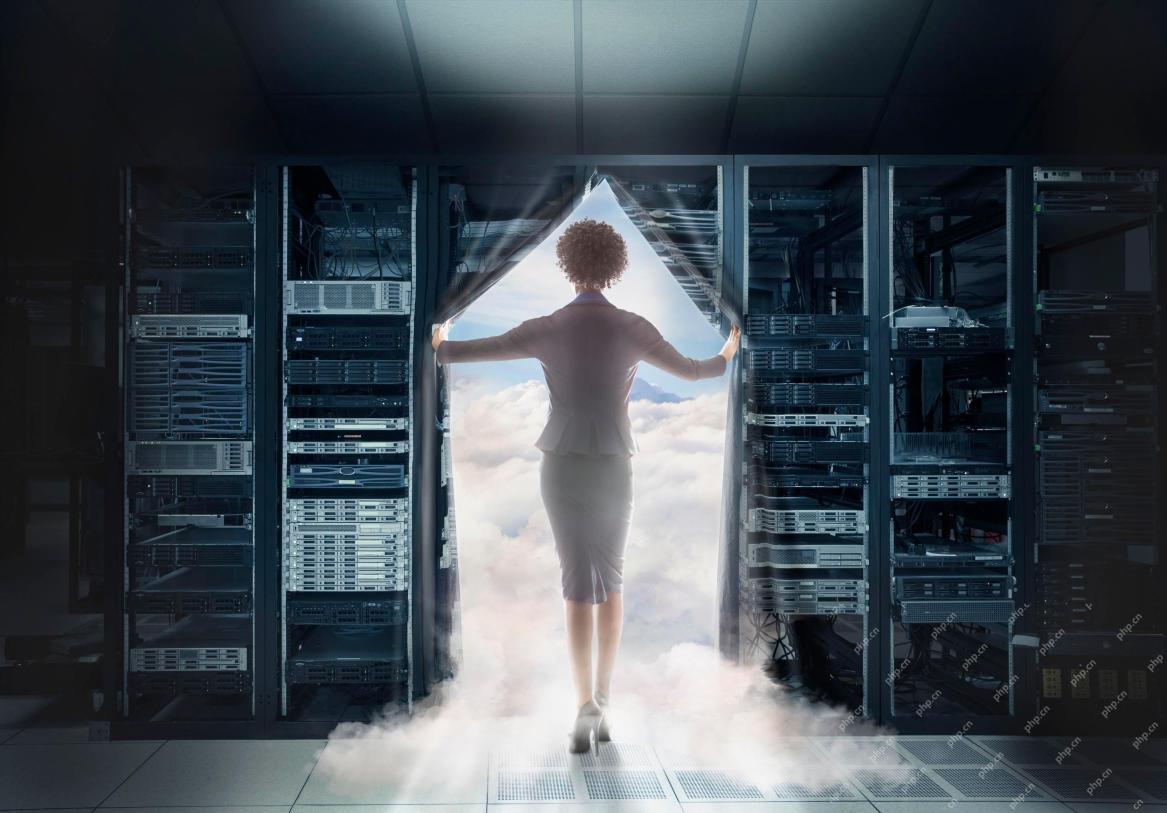
ジョン・ロールズの独創的な1971年の著書「正義の理論」で、彼は私たちが今日のAIデザインの核となり、意思決定を使用するべきであるという思考実験を提案しました:無知のベール。この哲学は、公平性を理解するための簡単なツールを提供し、リーダーがこの理解を使用してAIを公平に設計および実装するための青写真を提供します。 あなたが新しい社会のルールを作っていると想像してください。しかし、前提があります。この社会でどのような役割を果たすかは事前にわかりません。過半数または限界少数派に属している、金持ちまたは貧弱、健康、または障害者になることがあります。この「無知のベール」の下で活動することで、ルールメーカーが自分自身に利益をもたらす決定を下すことができません。それどころか、人々はより公衆を策定する意欲があります
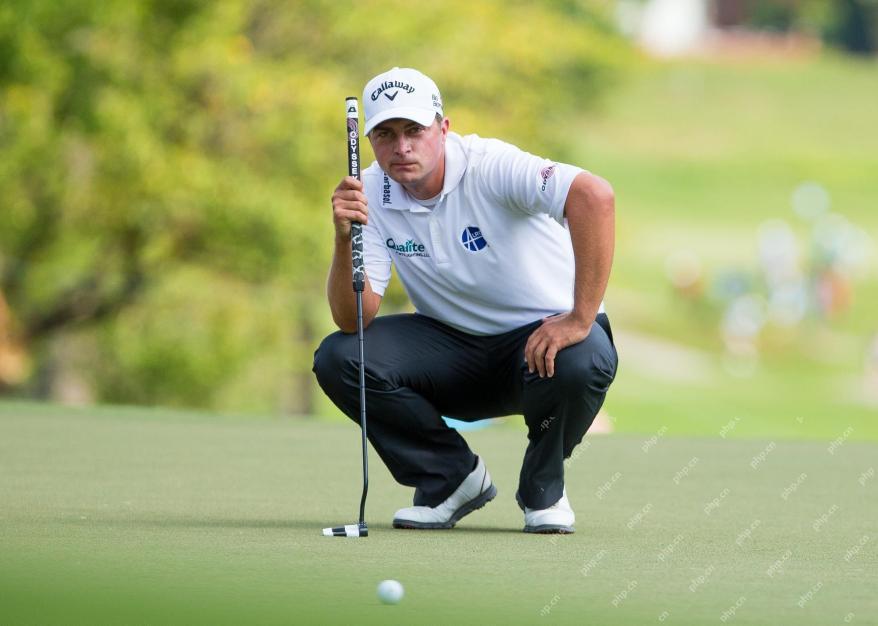
ロボットプロセスオートメーション(RPA)を専門とする多くの企業は、繰り返しタスクを自動化するためのボットを提供しています。 一方、プロセスマイニング、オーケストレーション、インテリジェントドキュメント処理スペシャル
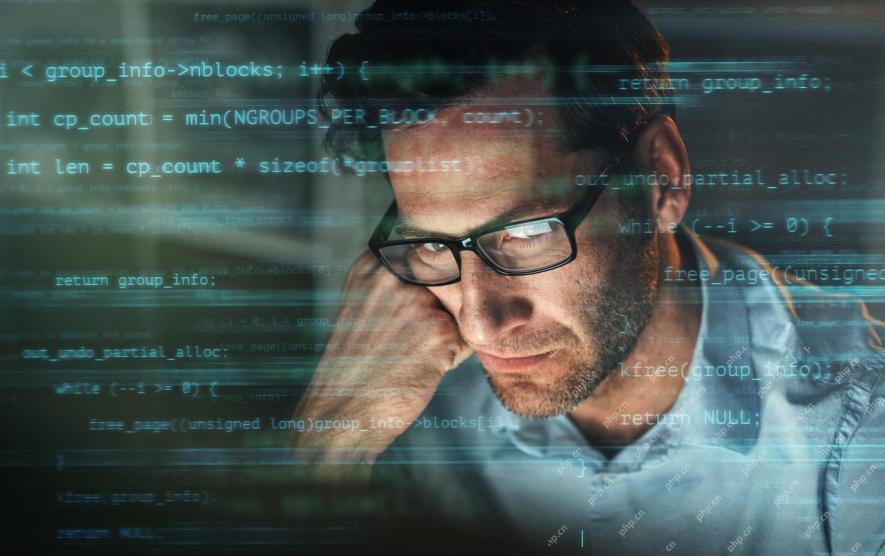
AIの未来は、単純な単語の予測と会話シミュレーションを超えて動いています。 AIエージェントは出現しており、独立したアクションとタスクの完了が可能です。 このシフトは、AnthropicのClaudeのようなツールですでに明らかです。 AIエージェント:研究a
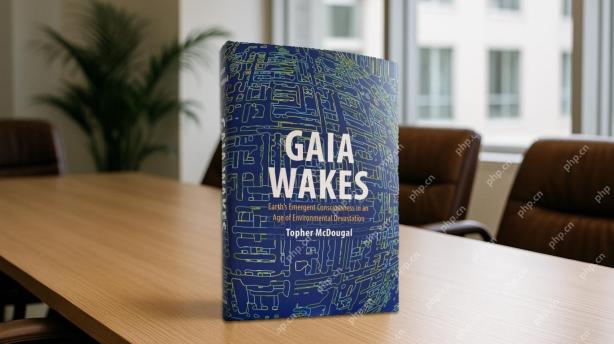
急速な技術の進歩は、仕事の未来に関する将来の見通しの視点を必要とします。 AIが単なる生産性向上を超えて、私たちの社会構造の形成を開始するとどうなりますか? Topher McDougalの今後の本、Gaia Wakes:
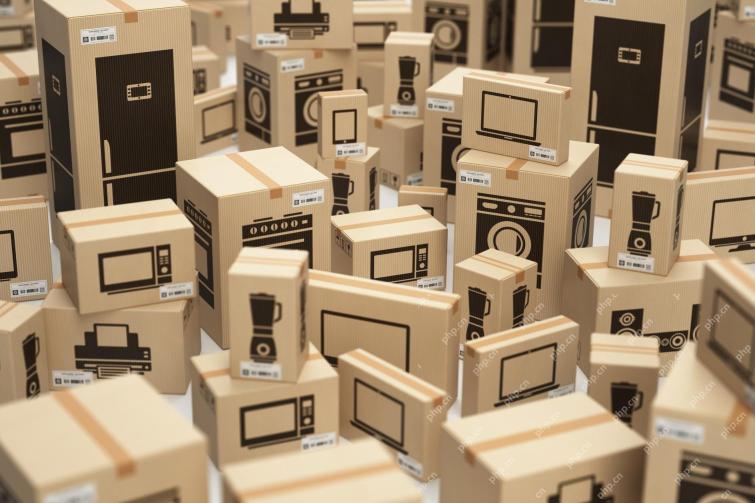
多くの場合、Harmonized System(HS)などのシステムからの「HS 8471.30」などの複雑なコードを含む製品分類は、国際貿易と国内販売に不可欠です。 これらのコードは、すべてのINVに影響を与える正しい税申請を保証します
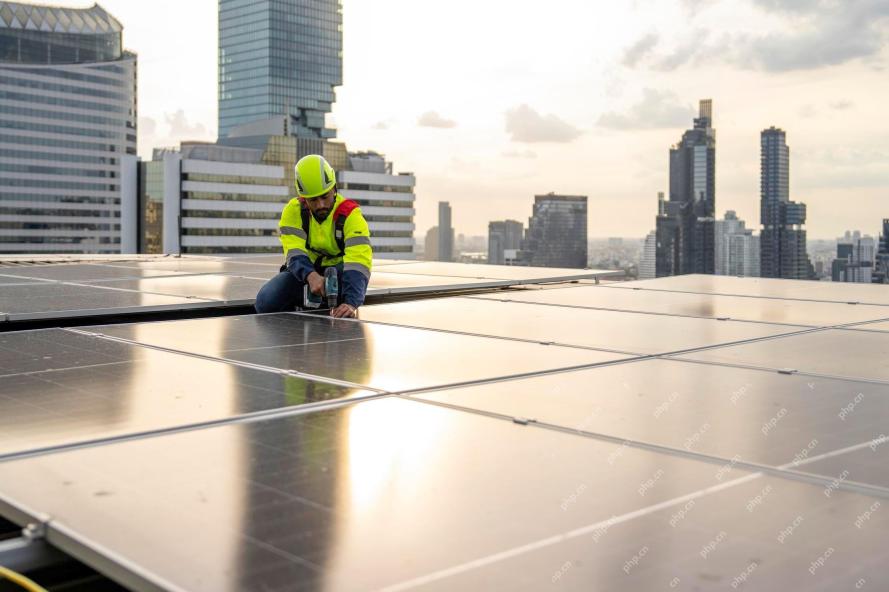
データセンターと気候技術投資におけるエネルギー消費の将来 この記事では、AIが推進するデータセンターのエネルギー消費の急増と気候変動への影響を調査し、この課題に対処するための革新的なソリューションと政策の推奨事項を分析します。 エネルギー需要の課題:大規模で超大規模なデータセンターは、数十万の普通の北米の家族の合計に匹敵する巨大な力を消費し、新たなAIの超大規模なセンターは、これよりも数十倍の力を消費します。 2024年の最初の8か月で、Microsoft、Meta、Google、Amazonは、AIデータセンターの建設と運用に約1,250億米ドルを投資しました(JP Morgan、2024)(表1)。 エネルギー需要の成長は、挑戦と機会の両方です。カナリアメディアによると、迫り来る電気
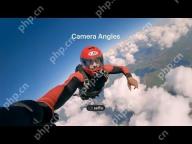
生成AIは、映画とテレビの制作に革命をもたらしています。 LumaのRay 2モデル、滑走路のGen-4、OpenaiのSora、GoogleのVEO、その他の新しいモデルは、前例のない速度で生成されたビデオの品質を向上させています。これらのモデルは、複雑な特殊効果と現実的なシーンを簡単に作成できます。短いビデオクリップやカメラ認知モーション効果も達成されています。これらのツールの操作と一貫性を改善する必要がありますが、進歩の速度は驚くべきものです。 生成ビデオは独立した媒体になりつつあります。アニメーション制作が得意なモデルもあれば、実写画像が得意なモデルもあります。 AdobeのFireflyとMoonvalleyのMAであることは注目に値します
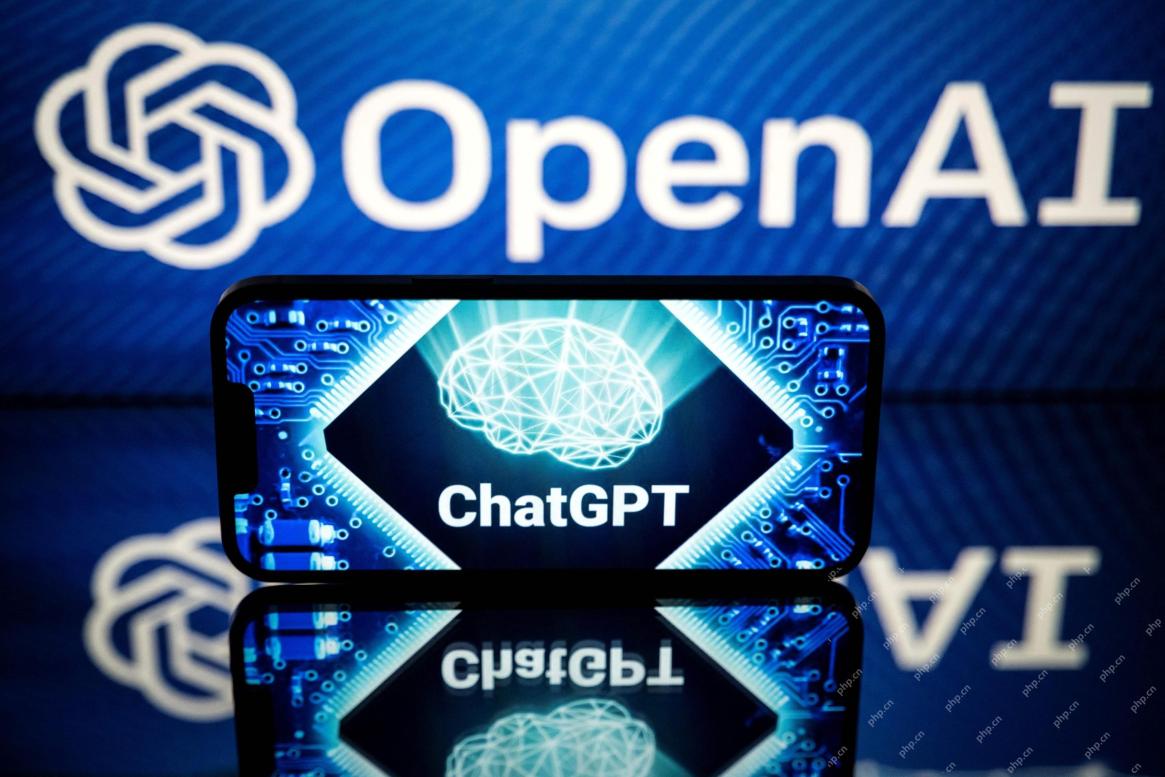
ChatGptユーザーエクスペリエンスは低下します:それはモデルの劣化ですか、それともユーザーの期待ですか? 最近、多数のCHATGPT有料ユーザーがパフォーマンスの劣化について不満を述べています。 ユーザーは、モデルへの応答が遅く、答えが短い、助けの欠如、さらに多くの幻覚を報告しました。一部のユーザーは、ソーシャルメディアに不満を表明し、ChatGptは「お世辞になりすぎて」、重要なフィードバックを提供するのではなく、ユーザービューを検証する傾向があることを指摘しています。 これは、ユーザーエクスペリエンスに影響を与えるだけでなく、生産性の低下やコンピューティングリソースの無駄など、企業の顧客に実際の損失をもたらします。 パフォーマンスの劣化の証拠 多くのユーザーは、特にGPT-4などの古いモデル(今月末にサービスから廃止される)で、ChatGPTパフォーマンスの大幅な分解を報告しています。 これ


ホットAIツール

Undresser.AI Undress
リアルなヌード写真を作成する AI 搭載アプリ

AI Clothes Remover
写真から衣服を削除するオンライン AI ツール。

Undress AI Tool
脱衣画像を無料で

Clothoff.io
AI衣類リムーバー

Video Face Swap
完全無料の AI 顔交換ツールを使用して、あらゆるビデオの顔を簡単に交換できます。

人気の記事

ホットツール

AtomエディタMac版ダウンロード
最も人気のあるオープンソースエディター
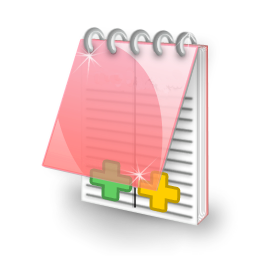
EditPlus 中国語クラック版
サイズが小さく、構文の強調表示、コード プロンプト機能はサポートされていません
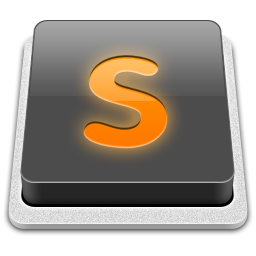
SublimeText3 Mac版
神レベルのコード編集ソフト(SublimeText3)

Safe Exam Browser
Safe Exam Browser は、オンライン試験を安全に受験するための安全なブラウザ環境です。このソフトウェアは、あらゆるコンピュータを安全なワークステーションに変えます。あらゆるユーティリティへのアクセスを制御し、学生が無許可のリソースを使用するのを防ぎます。

SAP NetWeaver Server Adapter for Eclipse
Eclipse を SAP NetWeaver アプリケーション サーバーと統合します。

ホットトピック









