AI agents are designed to act autonomously, solving problems and executing tasks in dynamic environments. A key feature in Autogen, enabling their adaptability is AutoGen’s code executors. This feature along with LLMs enables AI agents to generate, evaluate, and execute code in real-time. This capability bridges the gap between static AI models and actionable intelligence. By automating workflows, performing data analysis, and debugging complex systems, it transforms agents from mere thinkers into effective doers. In this article, we will learn more about code executors in AutoGen and how to implement them.
Table of Contents
- Types of Code Executors in AutoGen
- How to Build AI Agents with Code Executors in AutoGen?
- Pre-requisites
- Building an AI Agent Using Command Line Executor
- Building an ML Model Using Jupyter Code Executor
- Building an AI Agent Using Custom Executor
- Conclusion
- Frequently Asked Questions
Types of Code Executors in AutoGen
AutoGen has three kinds of code executors that can be used for different purposes.
- Command Line Executor: It allows AI agents to run the code in the command line. It will save each code block to a separate file and execute that file. This executor is ideal for automating tasks like file management, script execution, or handling external tools. It provides flexibility and low-level control in a workflow.
- Jupyter Code Executor: It enables agents to execute Python code within a Jupyter-like environment. Here, you can define variables in one code block and reuse them in subsequent blocks. One advantage of this setup is that when an error occurs, only the specific block of code with the error needs to be re-executed, rather than the entire script.
- Custom Code Executor: It gives developers the ability to create specialized code execution logic. For example, the custom code executor can access variables defined in the environment without explicitly providing them to the LLM.
These Code Executors can be run on both the host machine (local) as well as the Docker containers.
Also Read: 4 Steps to Build Multi-Agent Nested Chats with AutoGen
How to Build AI Agents with Code Executors in AutoGen?
Now let’s learn how you can use these different code executors in AutoGen:
Pre-requisites
Before building AI agents, ensure you have the necessary API keys for the required LLMs.
Load the .env file with the API keys needed.
from dotenv import load_dotenv load_dotenv(./env)
Key Libraries Required
autogen-agentchat – 0.2.38
jupyter_kernel_gateway-3.0.1
Building an AI Agent Using Command Line Executor
Let’s build an AI agent to know the offers and discounts available on an e-commerce website using the command line executor. Here are the steps to follow.
1. Import the necessary libraries.
from autogen import ConversableAgent, AssistantAgent, UserProxyAgent from autogen.coding import LocalCommandLineCodeExecutor, DockerCommandLineCodeExecutor
2. Define the agents.
user_proxy = UserProxyAgent( name="User", llm_config=False, is_termination_msg=lambda msg: msg.get("content") is not None and "TERMINATE" in msg["content"], human_input_mode="TERMINATE", code_execution_config=False ) code_writer_agent = ConversableAgent( name="CodeWriter", system_message="""You are a Python developer. You use your coding skill to solve problems. Once the task is done, returns 'TERMINATE'.""", llm_config={"config_list": [{"model": "gpt-4o-mini"}]}, ) local_executor = LocalCommandLineCodeExecutor( timeout=15, work_dir='./code files') local_executor_agent = ConversableAgent( "local_executor_agent", llm_config=False, code_execution_config={"executor": local_executor}, human_input_mode="ALWAYS", )
We are using the ‘local_executor’ in the code_execution_config of the local_executor_agent.
3. Define the messages which are used to initialize the chat.
messages = ["""To check whether there are any offers or discounts available on a given e-commerce website - https://www.flipkart.com/ Follow these steps, 1. download the html page of the given URL 2. we only need html content, so remove any CSS, JavaScript, and Image tags content 3. save the remaining html content. """ , "read the text and list all the offers and discounts available"] # Intialize the chat chat_result = local_executor_agent.initiate_chat( code_writer_agent, message=messages[0], )
It will ask for human input after each message from the codeWriter agent. You just need to press the ‘Enter’ key to execute the code written by the agent. We can also any further instructions if there is any problem with the code.
Here are the questions we have asked and the output at the end.
As we can see, with the mentioned questions, we can get a list of offers and discounts from an e-commerce website.
Also Read: Hands-on Guide to Building Multi-Agent Chatbots with AutoGen
Building an ML Model Using Jupyter Code Executor
By using this, we can access the variables defined in one code block from another code block, unlike the command line executor.
Now, let’s try to build an ML model using this.
1. Import the additional methods.
from autogen.coding.jupyter import LocalJupyterServer, DockerJupyterServer, JupyterCodeExecutor from pathlib import Path
2. Initialize the jupyter server and output directory.
server = LocalJupyterServer() output_dir = Path("coding") output_dir.mkdir()
Note that LocalJupyterServer may not function on Windows due to a bug. In this case, you can use the DockerJupyterServer instead or use the EmbeddedIPythonCodeExecutor.
3. Define the executor agent and writer agent with a custom system message.
jupyter_executor_agent = ConversableAgent( name="jupyter_executor_agent", llm_config=False, code_execution_config={ "executor": JupyterCodeExecutor(server, output_dir=output_dir), }, human_input_mode="ALWAYS", ) code_writer_system_message = """ You have been given coding capability to solve tasks using Python code in a stateful IPython kernel. You are responsible for writing the code, and the user is responsible for executing the code. When you write Python code, put the code in a markdown code block with the language set to Python. For example: ```python x = 3 ``` You can use the variable `x` in subsequent code blocks. ```python print(x) ``` Always use print statements for the output of the code. Write code incrementally and leverage the statefulness of the kernel to avoid repeating code. Import libraries in a separate code block. Define a function or a class in a separate code block. Run code that produces output in a separate code block. Run code that involves expensive operations like download, upload, and call external APIs in a separate code block. When your code produces an output, the output will be returned to you. Because you have limited conversation memory, if your code creates an image, the output will be a path to the image instead of the image itself.""" code_writer_agent = ConversableAgent( "code_writer", system_message=code_writer_system_message, llm_config={"config_list": [{"model": "gpt-4o"}]}, human_input_mode="TERMINATE", )
4. Define the initial message and initialize the chat
message = "read the datasets/user_behavior_dataset.csv and print what the data is about" chat_result = jupyter_executor_agent.initiate_chat( code_writer_agent, message=message, ) # Once the chat is completed we can stop the server. server.stop()
5. Once the chat is completed we can stop the server.
We can print the messages as follows
for chat in chat_result.chat_history[:]: if chat['name'] == 'code_writer' and 'TERMINATE' not in chat['content']: print("--------agent-----------") print(chat['content']) if chat['name'] == 'jupyter_executor_agent' and 'exitcode' not in chat['content']: print("--------user------------") print(chat['content'])
Here’s the sample
As we can see, we can get the code generated by the agent and also the results after executing the code.
Also Read: Building Agentic Chatbots Using AutoGen
Building an AI Agent Using Custom Executor
Now, let’s try to create a custom executor that can run the code in the same jupyter notebook where we are creating this executor. So, we can read a CSV file, and then ask an agent to build an ML model on the already imported file.
Here’s how we’ll do it.
1. Import the necessary libraries.
import pandas as pd from typing import List from IPython import get_ipython from autogen.coding import CodeBlock, CodeExecutor, CodeExtractor, CodeResult, MarkdownCodeExtractor
2. Define the executor that can extract and run the code from jupyter cells.
class NotebookExecutor(CodeExecutor): @property def code_extractor(self) -> CodeExtractor: # Extact code from markdown blocks. return MarkdownCodeExtractor() def __init__(self) -> None: # Get the current IPython instance running in this notebook. self._ipython = get_ipython() def execute_code_blocks(self, code_blocks: List[CodeBlock]) -> CodeResult: log = "" for code_block in code_blocks: result = self._ipython.run_cell("%%capture --no-display cap\n" + code_block.code) log += self._ipython.ev("cap.stdout") log += self._ipython.ev("cap.stderr") if result.result is not None: log += str(result.result) exitcode = 0 if result.success else 1 if result.error_before_exec is not None: log += f"\n{result.error_before_exec}" exitcode = 1 if result.error_in_exec is not None: log += f"\n{result.error_in_exec}" exitcode = 1 if exitcode != 0: break return CodeResult(exit_code=exitcode, output=log)
3. Define the agents.
code_writer_agent = ConversableAgent( name="CodeWriter", system_message="You are a helpful AI assistant.\n" "You use your coding skill to solve problems.\n" "You have access to a IPython kernel to execute Python code.\n" "You can suggest Python code in Markdown blocks, each block is a cell.\n" "The code blocks will be executed in the IPython kernel in the order you suggest them.\n" "All necessary libraries have already been installed.\n" "Add return or print statements to the code to get the output\n" "Once the task is done, returns 'TERMINATE'.", llm_config={"config_list": [{"model": "gpt-4o-mini"}]}, ) code_executor_agent = ConversableAgent( name="CodeExecutor", llm_config=False, code_execution_config={"executor": NotebookExecutor()}, is_termination_msg=lambda msg: "TERMINATE" in msg.get("content", "").strip().upper(), human_input_mode="ALWAYS" )
4. Read the file and initiate the chat with the file.
df = pd.read_csv('datasets/mountains_vs_beaches_preferences.csv') chat_result = code_executor_agent.initiate_chat( code_writer_agent, message="What are the column names in the dataframe defined above as df?", )
5. We can print the chat history as follows:
for chat in chat_result.chat_history[:]: if chat['name'] == 'CodeWriter' and 'TERMINATE' not in chat['content']: print("--------agent-----------") print(chat['content']) if chat['name'] == 'CodeExecutor' and 'exitcode' not in chat['content']: print("--------user------------") print(chat['content'])
As we can see again, we can get the code generated by the agent and also the results after executing the code.
Conclusion
AutoGen’s code executors provide flexibility and functionality for AI agents to perform real-world tasks. The command line executor enables script execution, while the Jupyter code executor supports iterative development. Custom executors, on the other hand, allow developers to create tailored workflows.
These tools empower AI agents to transition from problem solvers to solution implementers. Developers can use these features to build intelligent systems that deliver actionable insights and automate complex processes.
Frequently Asked Questions
Q1. What is the primary purpose of Code Executors in AutoGen?A. Code Executors in AutoGen allow AI agents to generate, execute, and evaluate code in real time. This enables agents to automate tasks, perform data analysis, debug systems, and implement dynamic workflows.
Q2. What are the differences between Command Line and Jupyter Code Executors?A. The Command Line Executor saves and executes code as separate files, ideal for tasks like file management and script execution. The Jupyter Code Executor operates in a stateful environment, allowing reuse of variables and selective re-execution of code blocks, making it more suitable for iterative coding tasks like building ML models.
Q3. Can Code Executors be used with Docker containers?A. Yes, both the Command Line Executor and Jupyter Code Executor can be configured to run on Docker containers, providing a flexible environment for execution.
Q4. What is the advantage of using a Custom Code Executor?A. Custom Code Executors allow developers to define specialized execution logic, such as running code within the same Jupyter notebook. This is useful for tasks requiring a high level of integration or customization.
Q5. What are the prerequisites for using Code Executors in AutoGen?A. Before using Code Executors, ensure you have the necessary API keys for your preferred LLMs. You should also have the required libraries, such as `autogen-agentchat` and `jupyter_kernel_gateway`, installed in your environment.
以上がAutogenのコードエグゼキューターを使用して複雑なタスクを達成しますの詳細内容です。詳細については、PHP 中国語 Web サイトの他の関連記事を参照してください。
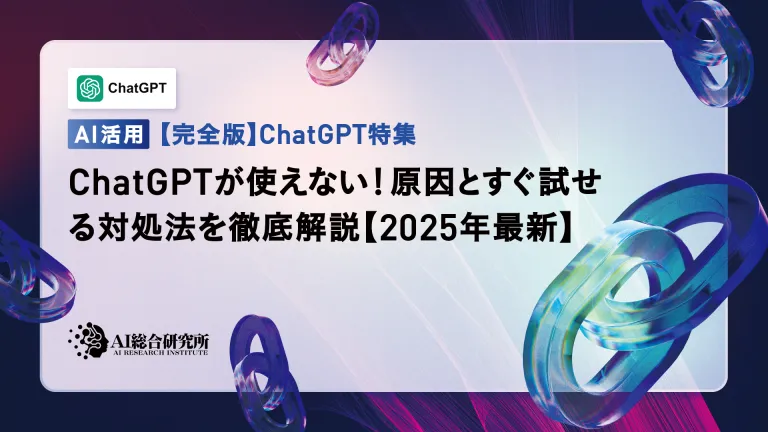
ChatGptはアクセスできませんか?この記事では、さまざまな実用的なソリューションを提供しています!多くのユーザーは、ChatGPTを毎日使用する場合、アクセス不能や応答が遅いなどの問題に遭遇する可能性があります。この記事では、さまざまな状況に基づいてこれらの問題を段階的に解決するように導きます。 ChatGPTのアクセス不能性と予備的なトラブルシューティングの原因 まず、問題がOpenaiサーバー側にあるのか、ユーザー自身のネットワークまたはデバイスの問題にあるのかを判断する必要があります。 以下の手順に従って、トラブルシューティングしてください。 ステップ1:OpenAIの公式ステータスを確認してください OpenAIステータスページ(status.openai.com)にアクセスして、ChatGPTサービスが正常に実行されているかどうかを確認してください。赤または黄色のアラームが表示されている場合、それは開くことを意味します
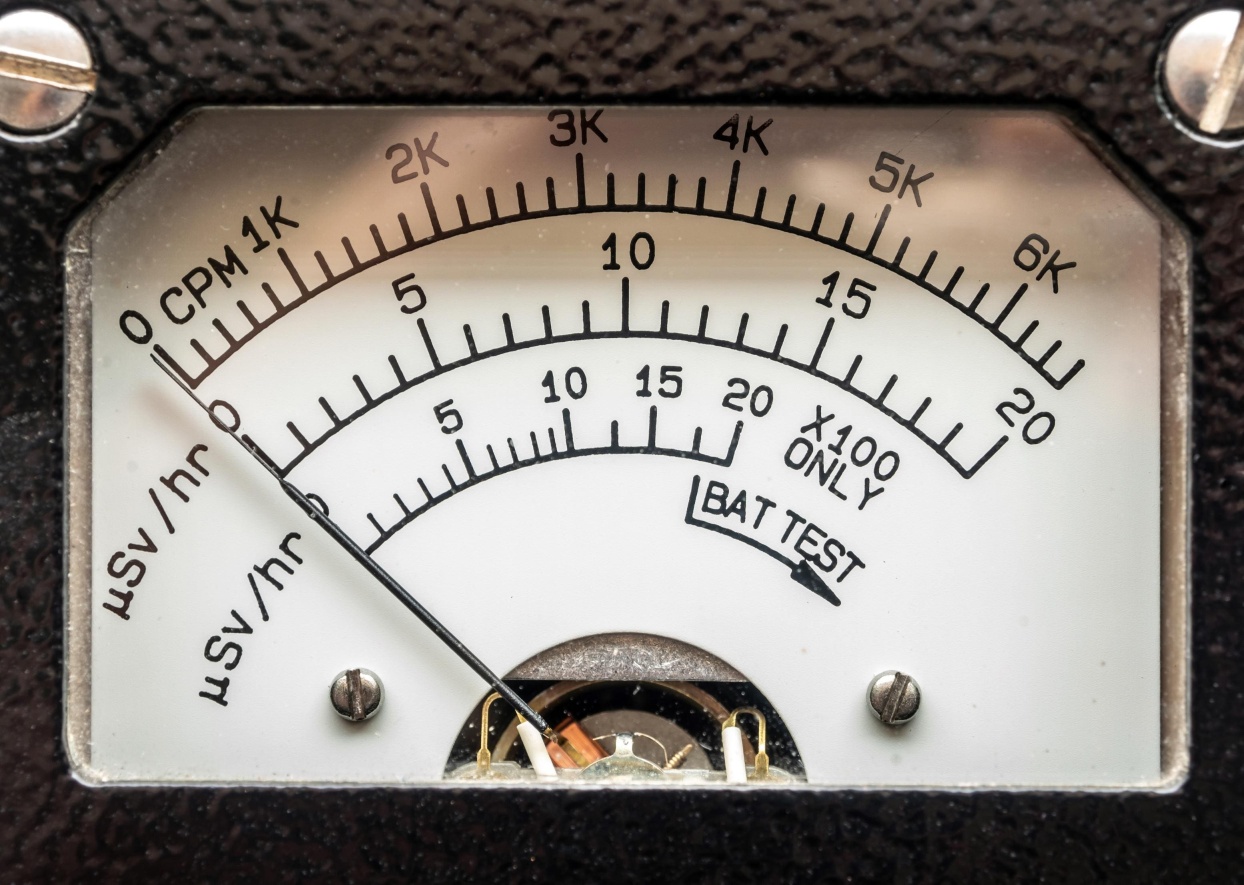
2025年5月10日、MIT物理学者のMax Tegmarkは、AI Labsが人工的なスーパーインテリジェンスを解放する前にOppenheimerの三位一体計算をエミュレートすべきだとGuardianに語った。 「私の評価では、「コンプトン定数」、競争が
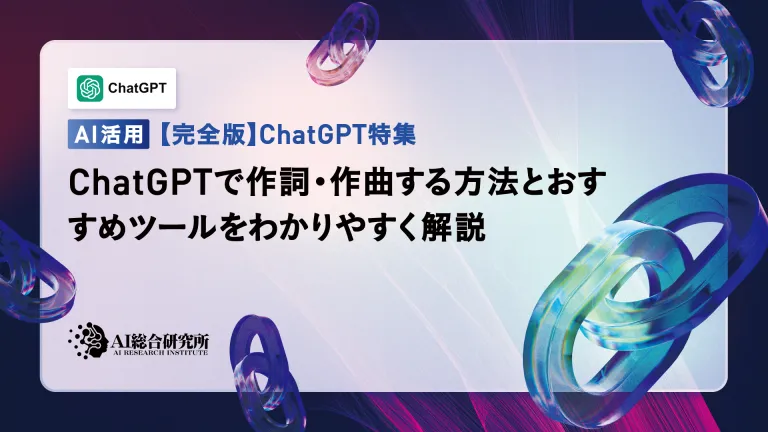
AI Music Creation Technologyは、1日ごとに変化しています。この記事では、ChatGPTなどのAIモデルを例として使用して、AIを使用して音楽の作成を支援し、実際のケースで説明する方法を詳細に説明します。 Sunoai、Hugging Face、PythonのMusic21 Libraryを通じて音楽を作成する方法を紹介します。 これらのテクノロジーを使用すると、誰もがオリジナルの音楽を簡単に作成できます。ただし、AIに生成されたコンテンツの著作権問題は無視できないことに注意する必要があります。使用する際には注意する必要があります。 音楽分野でのAIの無限の可能性を一緒に探りましょう! Openaiの最新のAIエージェント「Openai Deep Research」が紹介します。 [chatgpt] ope
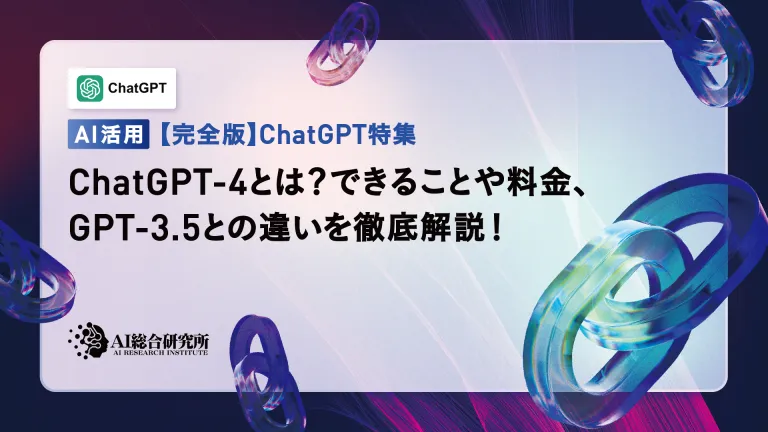
ChATGPT-4の出現により、AIアプリケーションの可能性が大幅に拡大しました。 GPT-3.5と比較して、CHATGPT-4は大幅に改善されました。強力なコンテキスト理解能力を備えており、画像を認識して生成することもできます。普遍的なAIアシスタントです。それは、ビジネス効率の改善や創造の支援など、多くの分野で大きな可能性を示しています。ただし、同時に、その使用における予防策にも注意を払わなければなりません。 この記事では、ChATGPT-4の特性を詳細に説明し、さまざまなシナリオの効果的な使用方法を紹介します。この記事には、最新のAIテクノロジーを最大限に活用するためのスキルが含まれています。参照してください。 Openaiの最新のAIエージェント、「Openai Deep Research」の詳細については、以下のリンクをクリックしてください
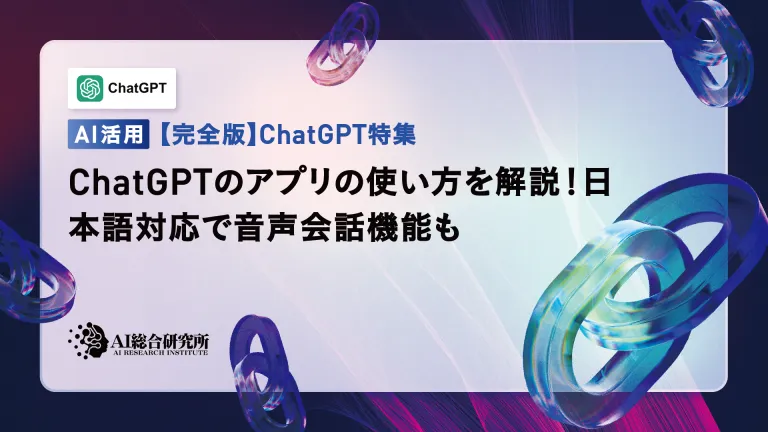
ChatGPTアプリ:AIアシスタントで創造性を解き放つ!初心者向けガイド ChatGPTアプリは、文章作成、翻訳、質問応答など、多様なタスクに対応する革新的なAIアシスタントです。創作活動や情報収集にも役立つ、無限の可能性を秘めたツールです。 この記事では、ChatGPTスマホアプリのインストール方法から、音声入力機能やプラグインといったアプリならではの機能、そしてアプリ利用上の注意点まで、初心者にも分かりやすく解説します。プラグインの制限やデバイス間の設定同期についてもしっかりと触れていきま
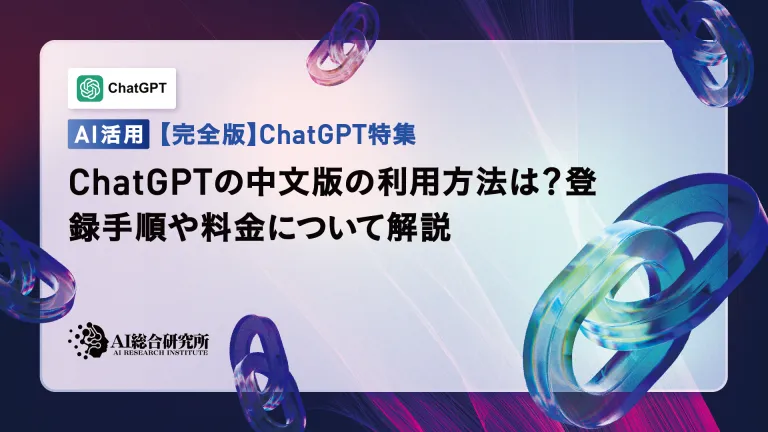
Chatgpt中国語版:中国語のAIの対話の新しい体験のロックを解除する ChatGptは世界中で人気がありますが、中国語版も提供していることをご存知ですか?この強力なAIツールは、毎日の会話をサポートするだけでなく、プロのコンテンツを処理し、簡素化された伝統的な中国語と互換性があります。中国のユーザーであろうと、中国語を学んでいる友人であろうと、あなたはそれから利益を得ることができます。 この記事では、アカウント設定、中国語の迅速な単語入力、フィルターの使用、さまざまなパッケージの選択を含むChatGpt中国語のバージョンの使用方法を詳細に紹介し、潜在的なリスクと対応戦略を分析します。さらに、ChatGpt中国語版を他の中国のAIツールと比較して、その利点とアプリケーションシナリオをよりよく理解するのに役立ちます。 Openaiの最新のAIインテリジェンス
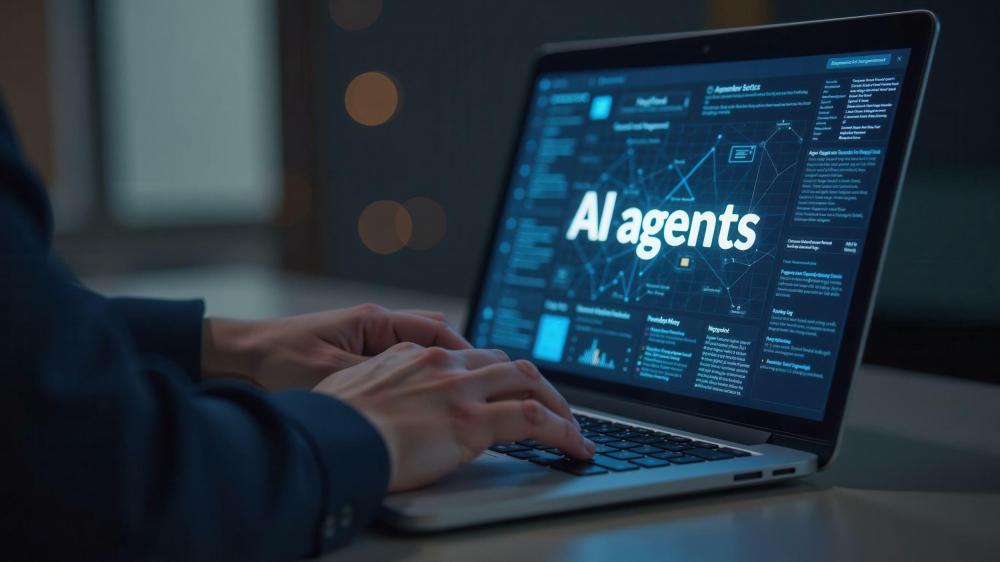
これらは、生成AIの分野で次の飛躍と考えることができ、ChatGptやその他の大規模なモデルのチャットボットを提供しました。単に質問に答えたり情報を生成したりするのではなく、彼らは私たちに代わって行動を起こすことができます。
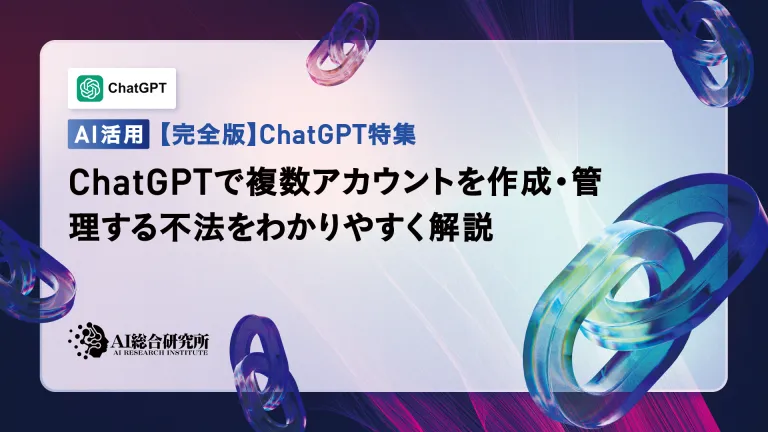
ChatGPTを活用した効率的な複数アカウント管理術|ビジネスとプライベートの使い分けも徹底解説! 様々な場面で活用されているChatGPTですが、複数アカウントの管理に頭を悩ませている方もいるのではないでしょうか。この記事では、ChatGPTの複数アカウント作成方法、利用上の注意点、そして安全かつ効率的な運用方法を詳しく解説します。ビジネス利用とプライベート利用の使い分け、OpenAIの利用規約遵守といった重要な点にも触れ、複数アカウントを安全に活用するためのガイドを提供します。 OpenAI


ホットAIツール

Undresser.AI Undress
リアルなヌード写真を作成する AI 搭載アプリ

AI Clothes Remover
写真から衣服を削除するオンライン AI ツール。

Undress AI Tool
脱衣画像を無料で

Clothoff.io
AI衣類リムーバー

Video Face Swap
完全無料の AI 顔交換ツールを使用して、あらゆるビデオの顔を簡単に交換できます。

人気の記事

ホットツール

SublimeText3 Linux 新バージョン
SublimeText3 Linux 最新バージョン

SecLists
SecLists は、セキュリティ テスターの究極の相棒です。これは、セキュリティ評価中に頻繁に使用されるさまざまな種類のリストを 1 か所にまとめたものです。 SecLists は、セキュリティ テスターが必要とする可能性のあるすべてのリストを便利に提供することで、セキュリティ テストをより効率的かつ生産的にするのに役立ちます。リストの種類には、ユーザー名、パスワード、URL、ファジング ペイロード、機密データ パターン、Web シェルなどが含まれます。テスターはこのリポジトリを新しいテスト マシンにプルするだけで、必要なあらゆる種類のリストにアクセスできるようになります。
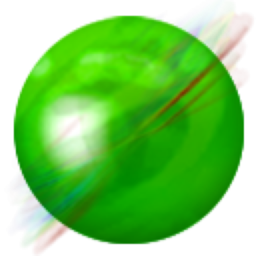
ZendStudio 13.5.1 Mac
強力な PHP 統合開発環境

DVWA
Damn Vulnerable Web App (DVWA) は、非常に脆弱な PHP/MySQL Web アプリケーションです。その主な目的は、セキュリティ専門家が法的環境でスキルとツールをテストするのに役立ち、Web 開発者が Web アプリケーションを保護するプロセスをより深く理解できるようにし、教師/生徒が教室環境で Web アプリケーションを教え/学習できるようにすることです。安全。 DVWA の目標は、シンプルでわかりやすいインターフェイスを通じて、さまざまな難易度で最も一般的な Web 脆弱性のいくつかを実践することです。このソフトウェアは、

メモ帳++7.3.1
使いやすく無料のコードエディター
