How do I use MongoDB's query language to retrieve data efficiently?
To use MongoDB's query language efficiently for data retrieval, you need to understand and apply the following concepts:
-
Basic Query Syntax: MongoDB uses a JSON-like syntax for querying data. For example, to find documents where the field
name
equals "John", you would use:db.collection.find({ name: "John" })
-
Operators: MongoDB provides a wide range of query operators such as
$eq
,$gt
,$lt
,$in
, and$or
. These allow for more complex and efficient queries. For instance, to find documents where the fieldage
is greater than 18 and less than 30, you could use:db.collection.find({ age: { $gt: 18, $lt: 30 } })
-
Projection: You can use projections to limit the amount of data returned from a query, reducing bandwidth and improving performance. For example, to retrieve only the
name
andemail
fields, you would use:db.collection.find({}, { name: 1, email: 1, _id: 0 })
-
Pagination: Efficiently handling large result sets involves using pagination. You can use
skip()
andlimit()
methods to retrieve results in manageable chunks:db.collection.find().skip(10).limit(10)
- Indexing: While not part of the query syntax itself, indexing is critical for efficient querying. MongoDB can use indexes to speed up queries by avoiding full collection scans. Always ensure that your queries can utilize indexes effectively.
By combining these elements, you can tailor your MongoDB queries to be as efficient as possible for your specific use cases.
What are the best practices for optimizing MongoDB queries to improve retrieval speed?
Optimizing MongoDB queries to enhance retrieval speed involves several best practices:
- Use Appropriate Indexes: Ensure that your queries can use indexes effectively. Indexes can drastically reduce the time required to retrieve data, especially for large collections.
-
Avoid Using
$or
: The$or
operator can be slow because MongoDB may not be able to use indexes efficiently for multiple conditions. Instead, use$in
where possible, or split the query into multiple indexed queries. -
Minimize the Use of
skip()
: Theskip()
method can be slow for large offsets. When paginating through large datasets, consider using range queries or a cursor-based pagination strategy. - Use Covered Queries: A covered query is one where all the fields in the query and the projection are covered by an index. This can significantly improve performance as MongoDB does not need to scan the document collection.
-
Limit and Sort Appropriately: Use
limit()
to constrain the number of documents returned andsort()
in conjunction with indexes to efficiently sort the results. - Regularly Analyze and Optimize: Use MongoDB’s profiling and explain tools to analyze queries and make necessary optimizations.
- Denormalization: In some cases, denormalizing your data can improve query performance by reducing the need for complex joins and lookups.
By implementing these best practices, you can significantly improve the speed and efficiency of your MongoDB queries.
How can I use indexes effectively in MongoDB to enhance query performance?
Using indexes effectively in MongoDB is key to enhancing query performance. Here are some strategies:
-
Create Indexes on Frequently Queried Fields: If you often query by certain fields, create indexes on these fields. For example, if you frequently search by
username
, you should create an index on theusername
field:db.collection.createIndex({ username: 1 })
-
Compound Indexes: Use compound indexes when your queries involve multiple fields. For example, if you commonly query by both
lastName
andfirstName
, a compound index would be beneficial:db.collection.createIndex({ lastName: 1, firstName: 1 })
-
Indexing for Sorting and Ranging: If you sort or use range queries on certain fields, index them to improve performance. For example, if you sort by
createdAt
, index this field:db.collection.createIndex({ createdAt: 1 })
- Sparse Indexes: Use sparse indexes for fields that are not present in every document. This can save space and improve performance for queries that filter on these fields.
-
Text Indexes: For full-text search capabilities, create text indexes on fields that contain text data:
db.collection.createIndex({ description: "text" })
-
Monitor and Adjust Indexes: Regularly use the
explain()
method to see how queries are using indexes and adjust them based on performance metrics. For instance:db.collection.find({ username: "john" }).explain()
By strategically planning and maintaining your indexes, you can greatly enhance the performance of your MongoDB queries.
What tools or methods can I use to analyze and troubleshoot slow MongoDB queries?
To analyze and troubleshoot slow MongoDB queries, you can utilize the following tools and methods:
-
MongoDB Profiler: MongoDB’s built-in profiler can log slow queries, which helps identify performance bottlenecks. You can enable the profiler to capture queries that exceed a certain execution time threshold:
db.setProfilingLevel(2, { slowms: 100 })
-
Explain() Method: The
explain()
method provides detailed information about the query execution plan, including index usage and execution time. Use it to analyze how your queries are being processed:db.collection.find({ field: "value" }).explain()
- MongoDB Compass: This GUI tool offers visual query performance analysis, showing execution statistics and index usage, which can be particularly helpful for developers who prefer a graphical interface.
- MongoDB Atlas Performance Advisor: If you're using MongoDB Atlas, the Performance Advisor can automatically analyze your queries and provide recommendations for index creation and optimization.
- Database Profiler and Logs: Regularly review the MongoDB server logs to identify and troubleshoot slow operations. You can configure MongoDB to log queries that exceed certain time thresholds.
- Third-Party Monitoring Tools: Tools like Datadog, New Relic, and Prometheus can monitor MongoDB performance and help identify slow queries in real-time.
-
Query Plan Cache: MongoDB caches query plans, which can help optimize repeated queries. Use the
planCacheListPlans
command to review cached plans:db.collection.getPlanCache().listPlans()
By leveraging these tools and methods, you can effectively analyze and troubleshoot slow MongoDB queries, ensuring optimal database performance.
以上がMongoDBのクエリ言語を使用してデータを効率的に取得するにはどうすればよいですか?の詳細内容です。詳細については、PHP 中国語 Web サイトの他の関連記事を参照してください。
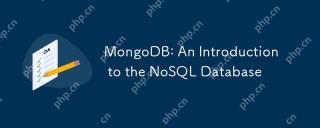
MongoDBは、複雑で構造化されていないデータの処理に適したBSON形式を使用してデータを保存するドキュメントベースのNOSQLデータベースです。 1)そのドキュメントモデルは柔軟で、頻繁に変化するデータ構造に適しています。 2)MongoDBは、WiredTigerストレージエンジンとクエリオプティマイザーを使用して、効率的なデータ操作とクエリをサポートします。 3)基本操作には、ドキュメントの挿入、クエリ、更新、削除が含まれます。 4)高度な使用法には、複雑なデータ分析に集約フレームワークを使用することが含まれます。 5)一般的なエラーには、接続の問題、クエリのパフォーマンスの問題、およびデータの一貫性の問題が含まれます。 6)パフォーマンスの最適化とベストプラクティスには、インデックスの最適化、データモデリング、シャード、キャッシュ、監視、チューニングが含まれます。
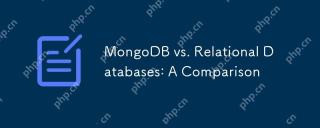
MongoDBは、柔軟なデータモデルと高いスケーラビリティを必要とするシナリオに適していますが、リレーショナルデータベースは、複雑なクエリとトランザクション処理を使用するアプリケーションにより適しています。 1)Mongodbのドキュメントモデルは、迅速な反復現代アプリケーション開発に適応します。 2)リレーショナルデータベースは、テーブル構造とSQLを通じて複雑なクエリと金融システムをサポートします。 3)MongoDBは、大規模なデータ処理に適したシャードを介して水平スケーリングを実現します。 4)リレーショナルデータベースは垂直拡張に依存しており、クエリとインデックスを最適化する必要があるシナリオに適しています。
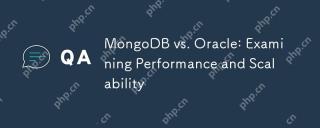
MongoDBは、高いスケーラビリティと柔軟性の要件に適したパフォーマンスとスケーラビリティが優れています。 Oracleは、厳格なトランザクション制御と複雑なクエリを要求する上で優れたパフォーマンスを発揮します。 1.MongoDBは、大規模なデータと高い並行性シナリオに適した、シャードテクノロジーを通じて高いスケーラビリティを実現します。 2。Oracleは、構造化されたデータとトランザクション制御のニーズに適したパフォーマンスを改善するために、オプティマイザーと並列処理に依存しています。
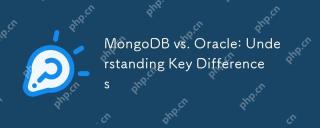
MongoDBは、大規模な構造化されていないデータの処理に適しており、Oracleはトランザクションの一貫性を必要とするエンタープライズレベルのアプリケーションに適しています。 1.MongoDBは、ユーザーの動作データの処理に適した柔軟性と高性能を提供します。 2。Oracleは、その安定性と強力な機能で知られており、金融システムに適しています。 3.MongoDBはドキュメントモデルを使用し、Oracleはリレーショナルモデルを使用します。 4.MongoDBはソーシャルメディアアプリケーションに適していますが、Oracleはエンタープライズレベルのアプリケーションに適しています。
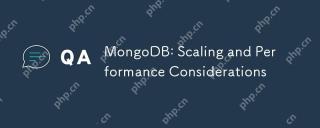
MongoDBのスケーラビリティとパフォーマンスの考慮事項には、水平スケーリング、垂直スケーリング、パフォーマンスの最適化が含まれます。 1.システム容量を改善するために、シャードテクノロジーを通じて水平拡張が達成されます。 2。垂直拡張により、ハードウェアリソースを増やすことでパフォーマンスが向上します。 3.パフォーマンスの最適化は、インデックスの合理的な設計と最適化されたクエリ戦略を通じて達成されます。
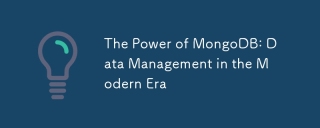
MongoDBは、柔軟性とスケーラビリティが最新のデータ管理において非常に重要であるため、NOSQLデータベースです。ドキュメントストレージを使用し、大規模で可変データの処理に適しており、強力なクエリとインデックスの機能を提供します。
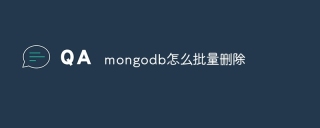
次の方法を使用して、MongoDBでドキュメントを削除できます。1。オペレーターの$は、削除するドキュメントのリストを指定します。 2。正規表現は、基準を満たすドキュメントと一致します。 3. $ exists演算子は、指定されたフィールドを使用してドキュメントを削除します。 4。sing()およびremove()メソッドは、最初にドキュメントを取得して削除します。これらの操作はトランザクションを使用できず、一致するすべてのドキュメントを削除する場合があるため、使用する場合は注意してください。
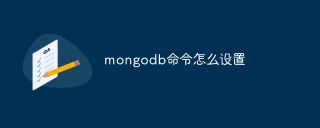
MongoDBデータベースをセットアップするには、コマンドライン(使用およびdb.createcollection())またはMongoシェル(Mongo、Use、DB.CreateCollection())を使用できます。その他の設定オプションには、データベースの表示(DBSの表示)、コレクションの表示(コレクションの表示)、データベースの削除(db.dropdatabase())、db。& collection_name& gt; drop())、挿入文書(db; lt; lt; lt; collection


ホットAIツール

Undresser.AI Undress
リアルなヌード写真を作成する AI 搭載アプリ

AI Clothes Remover
写真から衣服を削除するオンライン AI ツール。

Undress AI Tool
脱衣画像を無料で

Clothoff.io
AI衣類リムーバー

AI Hentai Generator
AIヘンタイを無料で生成します。

人気の記事

ホットツール

SecLists
SecLists は、セキュリティ テスターの究極の相棒です。これは、セキュリティ評価中に頻繁に使用されるさまざまな種類のリストを 1 か所にまとめたものです。 SecLists は、セキュリティ テスターが必要とする可能性のあるすべてのリストを便利に提供することで、セキュリティ テストをより効率的かつ生産的にするのに役立ちます。リストの種類には、ユーザー名、パスワード、URL、ファジング ペイロード、機密データ パターン、Web シェルなどが含まれます。テスターはこのリポジトリを新しいテスト マシンにプルするだけで、必要なあらゆる種類のリストにアクセスできるようになります。
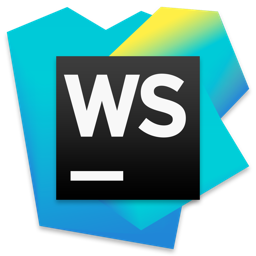
WebStorm Mac版
便利なJavaScript開発ツール
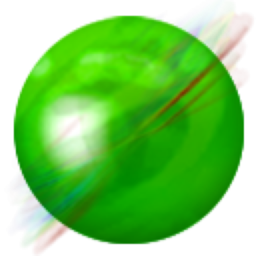
ZendStudio 13.5.1 Mac
強力な PHP 統合開発環境

Safe Exam Browser
Safe Exam Browser は、オンライン試験を安全に受験するための安全なブラウザ環境です。このソフトウェアは、あらゆるコンピュータを安全なワークステーションに変えます。あらゆるユーティリティへのアクセスを制御し、学生が無許可のリソースを使用するのを防ぎます。

MinGW - Minimalist GNU for Windows
このプロジェクトは osdn.net/projects/mingw に移行中です。引き続きそこでフォローしていただけます。 MinGW: GNU Compiler Collection (GCC) のネイティブ Windows ポートであり、ネイティブ Windows アプリケーションを構築するための自由に配布可能なインポート ライブラリとヘッダー ファイルであり、C99 機能をサポートする MSVC ランタイムの拡張機能が含まれています。すべての MinGW ソフトウェアは 64 ビット Windows プラットフォームで実行できます。
