テキスト ドキュメントの類似性の計算方法
ペアごとの類似性の計算
2 つのテキスト ドキュメント間の類似性を判断する最も一般的な方法は、テキスト ドキュメントを次のように変換することです。 TF-IDF (Term Frequency-Inverse Document Frequency) ベクトルを計算し、コサイン類似度を使用してそれらを比較します。このアプローチは、情報検索に関する教科書で説明されており、「情報検索の概要」で詳しく説明されています。
Gensim や scikit-learn などの Python ライブラリは、TF-IDF 変換とコサイン類似度計算の実装を提供します。 scikit-learn を使用すると、次のコード スニペットでコサイン類似度の計算が実行されます。
<code class="python">from sklearn.feature_extraction.text import TfidfVectorizer # Extract documents from text files documents = [open(f).read() for f in text_files] # Create a TF-IDF vectorizer tfidf = TfidfVectorizer().fit_transform(documents) # Calculate pairwise cosine similarity pairwise_similarity = tfidf * tfidf.T</code>
プレーン テキスト ドキュメントの場合:
<code class="python">corpus = ["I'd like an apple", "An apple a day keeps the doctor away", "Never compare an apple to an orange", "I prefer scikit-learn to Orange", "The scikit-learn docs are Orange and Blue"] # Create a TF-IDF vectorizer with minimum frequency and exclusion of stop words vect = TfidfVectorizer(min_df=1, stop_words="english") # Apply TF-IDF transformation tfidf = vect.fit_transform(corpus) # Calculate pairwise cosine similarity pairwise_similarity = tfidf * tfidf.T </code>
結果の解釈
pairwise_similarity は各行と列がコーパス内のドキュメントを表すスパース行列。スパース行列を NumPy 配列に変換すると、各セルが 2 つの対応するドキュメント間の類似性を表していることがわかります。
たとえば、「scikit-learn ドキュメントはオレンジとブルーです」に最も類似したドキュメントを特定するには、次の場所を見つけます。コーパス内のインデックスを取得し、np.fill_diagonal():
<code class="python">import numpy as np arr = pairwise_similarity.toarray() np.fill_diagonal(arr, np.nan) input_doc = "The scikit-learn docs are Orange and Blue" input_idx = corpus.index(input_doc) result_idx = np.nanargmax(arr[input_idx]) print(corpus[result_idx])</code>
で対角線 (自己相似性を表す) をマスクした後、np.nanargmax を対応する行に適用します。大規模なデータセットの場合は、スパース行列はメモリを節約します。あるいは、pairwise_similarity.shape を使用して自己類似性と argmax() を直接マスクすることを検討してください。
<code class="python">n, _ = pairwise_similarity.shape pairwise_similarity[np.arange(n), np.arange(n)] = -1.0 pairwise_similarity[input_idx].argmax() </code>
以上がTF-IDF とコサイン類似度を使用してテキスト ドキュメント間の類似性を計算するにはどうすればよいですか?の詳細内容です。詳細については、PHP 中国語 Web サイトの他の関連記事を参照してください。
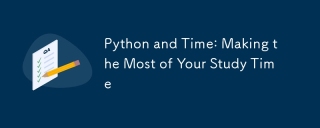
限られた時間でPythonの学習効率を最大化するには、PythonのDateTime、時間、およびスケジュールモジュールを使用できます。 1. DateTimeモジュールは、学習時間を記録および計画するために使用されます。 2。時間モジュールは、勉強と休息の時間を設定するのに役立ちます。 3.スケジュールモジュールは、毎週の学習タスクを自動的に配置します。
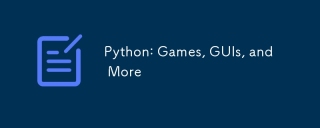
PythonはゲームとGUI開発に優れています。 1)ゲーム開発は、2Dゲームの作成に適した図面、オーディオ、その他の機能を提供し、Pygameを使用します。 2)GUI開発は、TKINTERまたはPYQTを選択できます。 TKINTERはシンプルで使いやすく、PYQTは豊富な機能を備えており、専門能力開発に適しています。
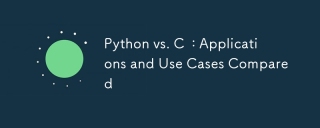
Pythonは、データサイエンス、Web開発、自動化タスクに適していますが、Cはシステムプログラミング、ゲーム開発、組み込みシステムに適しています。 Pythonは、そのシンプルさと強力なエコシステムで知られていますが、Cは高性能および基礎となる制御機能で知られています。
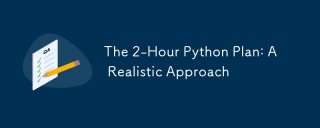
2時間以内にPythonの基本的なプログラミングの概念とスキルを学ぶことができます。 1.変数とデータ型、2。マスターコントロールフロー(条件付きステートメントとループ)、3。機能の定義と使用を理解する4。
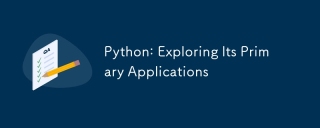
Pythonは、Web開発、データサイエンス、機械学習、自動化、スクリプトの分野で広く使用されています。 1)Web開発では、DjangoおよびFlask Frameworksが開発プロセスを簡素化します。 2)データサイエンスと機械学習の分野では、Numpy、Pandas、Scikit-Learn、Tensorflowライブラリが強力なサポートを提供します。 3)自動化とスクリプトの観点から、Pythonは自動テストやシステム管理などのタスクに適しています。
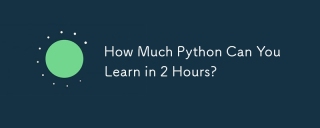
2時間以内にPythonの基本を学ぶことができます。 1。変数とデータ型を学習します。2。ステートメントやループの場合などのマスター制御構造、3。関数の定義と使用を理解します。これらは、簡単なPythonプログラムの作成を開始するのに役立ちます。
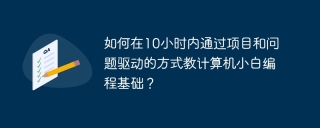
10時間以内にコンピューター初心者プログラミングの基本を教える方法は?コンピューター初心者にプログラミングの知識を教えるのに10時間しかない場合、何を教えることを選びますか...
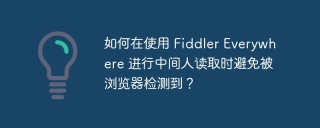
fiddlereveryversings for the-middleの測定値を使用するときに検出されないようにする方法


ホットAIツール

Undresser.AI Undress
リアルなヌード写真を作成する AI 搭載アプリ

AI Clothes Remover
写真から衣服を削除するオンライン AI ツール。

Undress AI Tool
脱衣画像を無料で

Clothoff.io
AI衣類リムーバー

AI Hentai Generator
AIヘンタイを無料で生成します。

人気の記事

ホットツール
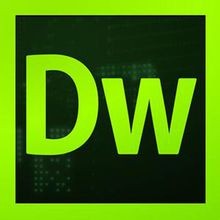
ドリームウィーバー CS6
ビジュアル Web 開発ツール

MantisBT
Mantis は、製品の欠陥追跡を支援するために設計された、導入が簡単な Web ベースの欠陥追跡ツールです。 PHP、MySQL、Web サーバーが必要です。デモおよびホスティング サービスをチェックしてください。

DVWA
Damn Vulnerable Web App (DVWA) は、非常に脆弱な PHP/MySQL Web アプリケーションです。その主な目的は、セキュリティ専門家が法的環境でスキルとツールをテストするのに役立ち、Web 開発者が Web アプリケーションを保護するプロセスをより深く理解できるようにし、教師/生徒が教室環境で Web アプリケーションを教え/学習できるようにすることです。安全。 DVWA の目標は、シンプルでわかりやすいインターフェイスを通じて、さまざまな難易度で最も一般的な Web 脆弱性のいくつかを実践することです。このソフトウェアは、

MinGW - Minimalist GNU for Windows
このプロジェクトは osdn.net/projects/mingw に移行中です。引き続きそこでフォローしていただけます。 MinGW: GNU Compiler Collection (GCC) のネイティブ Windows ポートであり、ネイティブ Windows アプリケーションを構築するための自由に配布可能なインポート ライブラリとヘッダー ファイルであり、C99 機能をサポートする MSVC ランタイムの拡張機能が含まれています。すべての MinGW ソフトウェアは 64 ビット Windows プラットフォームで実行できます。

SecLists
SecLists は、セキュリティ テスターの究極の相棒です。これは、セキュリティ評価中に頻繁に使用されるさまざまな種類のリストを 1 か所にまとめたものです。 SecLists は、セキュリティ テスターが必要とする可能性のあるすべてのリストを便利に提供することで、セキュリティ テストをより効率的かつ生産的にするのに役立ちます。リストの種類には、ユーザー名、パスワード、URL、ファジング ペイロード、機密データ パターン、Web シェルなどが含まれます。テスターはこのリポジトリを新しいテスト マシンにプルするだけで、必要なあらゆる種類のリストにアクセスできるようになります。
